Comparison of topological properties of functional brain networks with graph theory in temporal lobe epilepsy with different duration of disease
Introduction
Temporal lobe epilepsy (TLE) is a focal disease, and its prevalence is 0.1% (1). However, a previous study showed that TLE could be considered as a neurological network disorder and is closely related to brain functions from the perspective of the occurrence of epilepsy and the spread of seizures (2). Recently, TLE-related cognition dysfunction has attracted widespread concerns and could cause equivalent or more significant harm than those caused by the epileptic seizures themselves (3,4). Previous studies showed that cognitive impairments in TLE patients ranged from inadequate attention, language, and memory to globally decreased cognition (5), and of which attention decline was most common (3,6). Alertness refers to maintaining a sensitive state to receive information network information, and the alertness network is one of the most critical attention networks, which was proved to be an essential factor for studying attention and cognitive psychology (7,8). The abnormality of the alertness network of TLE patients has attracted people’s attention (9,10); however, how the alertness of TLE patients changes and its underlying neural mechanism remains to be elucidated.
Epilepsy is a chronic disease. Many earlier studies have found that the imaging characteristics of epilepsy are associated with the duration of disease. Specifically, Cheung et al. (11) found that the speech and visual memory of TLE patients were negatively associated with the duration of disease. Further, Li et al. (12) confirmed that the functional connectivity (FC) in the hippocampus of TLE with hippocampal sclerosis was positively correlated with the duration of the disease. Haneef et al. (13) found that a longer epilepsy duration was associated with a lower betweenness centrality (BC). Haneef et al. (14) found that TLE showed reduced connectivity diversity as epilepsy progression. However, the characteristics of the TLE network have rarely explicitly been studied. In the well-known SANAD trial (15), the average duration of epilepsy tracked for newly diagnosed patients was five years. Rathouz et al. (16) found that childhood epilepsy was characterized by early (nearly onset and diagnosis) cognitive abnormalities that follow with overtime (up to 6 years), which persisted in a mostly unchanged manner with no signs of progress or recovery and could serve as a model for adult epilepsy. However, it is unknown whether the topological properties of the TLE network progress or recover with the increase of the duration of epilepsy, and the time of this change needs to be further studied.
Resting-state fMRI has been proved to can non-invasively measure the intrinsic or spontaneous activity of the human brain (17,18), and is widely applied on studying interregional functional connection patterns that connect healthy individuals with diseased people (19,20). Previous rs-fMRI reports showed lots of important topological properties of functional brain networks, including small-worldness characteristics for balancing functional separation and integration, important modular structures, and densely connected hubs using graph theory methods (21-23). A previous study showed that the graph-based network methods were not only beneficial for the architecture of the whole-brain network but also were valuable for further exploring the underlying mechanisms of various cognitive deficits (24). Recent studies also characterized the topological properties of brain networks under health and disease conditions using this method (25,26). Therefore, studies on rs-fMRI functional networks based on the graph theory are of great significance for exploring the topological mechanism of large-scale brain networks under health and disease conditions.
We hypothesized that TLE patients showed the brain network alterations of the topological properties with the extension of the duration of disease, and the time for these alterations might be 5 years. The brain network alterations might be the potential neural mechanisms of cognitive dysfunction. To further verify this hypothesis, the patterns of alterations in the functional brain network of TLE patients under the different duration of disease were systematically studied and compared with healthy participants and exploring the potential neural mechanisms of cognitive dysfunction in TLE patients.
We present the following article in accordance with the MDAR reporting checklist (available at http://dx.doi.org/10.21037/atm-20-6823).
Methods
Participants
From January 1, 20119, to October 1, 2019, 58 right-handed participants including 37 TLE patients (17 TLE patients with short duration of disease (≤5 years; TLE-SD) and 20 TLE patients with long duration of disease (>5 years; TLE-LD) and 21 healthy controls (HCs, matched with age, sex and education level) were included in this trial. All participants were recruited from the First Affiliated Hospital of Guangxi Medical University and the local community. We performed the diagnosis for TLE following the guidelines of the International Anti-Epilepsy Alliance (27,28). All participants received a comprehensive standard clinical assessment, including detailed medical history investigation, neurological examination, neuropsychological assessment, electroencephalogram (EEG) and brain magnetic resonance imaging (MRI) scanning. We listed detailed demographics and clinical characteristics of all subjects in Table 1.
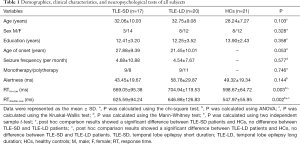
Full table
The inclusion criteria for participants were: (I) the symptoms of clinical seizures were shown as the origin of the temporal lobe; (II) EEG showed that epilepsy originated from the origin of the unilateral temporal lobe; (III) participants had no epileptic seizures within the last three months (IV) there was no identifiable lesion in the brain MRI; (V) the duration of the disease > one year; (VI) all participants have been regularly taking anti-epileptic drugs (AEDs).
The exclusion criteria for subjects were: (I) mental illness, alcohol or drug abuse histories; (II) combination with the cognitive-affected diseases including craniocerebral trauma, brain tumors, stroke, intracranial infection, multiple sclerosis, and Alzheimer’s diseases; (III) MRI scanning showed abnormalities in other structures except for hippocampal sclerosis; (IV) mini-mental state examination score ≤24; (V) left-handed; (VI) examination could not cooperate; (VII)Patients taking or taking topiramate or barbiturates.
The Ethics Committee of the First Affiliated Hospital of Guangxi Medical University approved this experimental protocol (No. 2020-KY-040). Each participant signed formal written consent. All procedures performed in this study involving human participants were in accordance with the Declaration of Helsinki (as revised in 2013).
Neuropsychological examination of alertness
Attention Network Test (ANT) was used to assess the alertness of each participant, as previously reported (29). This test requires the subject to sit in front of a computer and respond to different visual stimuli composed of cues and targets via pressing the right buttons. Meanwhile, this test also requires the subject to measure whether the center target arrow is left or right and react fast and accurately via pushing the left or right arrow buttons. Also, there can be a warning showed by an asterisk or no warning before the center target arrow appears. Based on the presence or absence and the position of the asterisk, four kinds of prompt conditions are: (I) no cue (NC), no asterisk appears before the target appears, and only one cross representing the fixed point that exists on the screen; (II) double cues (DC), the asterisk appears above and below the fixed cross; (III) center cue (CC), the asterisk appears in the center of the screen replacing the fixed cross and does not provide any information about the target location; (IV) space cue (SC), an asterisk appears above or below the cross to show where the arrow is about to appear. This test comprised 24 exercises and three sets of trails, each comprising 96 tests, and the entire test time was about 25 minutes. The correct rate and response time (RT) of each subject were recorded using computers. According to Fan’s ANT design principle (30), alertness was measured: alertness = RT no cue-RT double cues, and the more significant the difference, the higher the efficiency.
MRI data collection
The 3.0-T scanner (Philips, The Netherlands) was used to perform MRI, and Rs-fMRI and high-resolution T1-weighted anatomic imaging data were collected from each participant. The rs-fMRI scanning prolonged for 450 seconds, and 225 brain volumes were retrieved. During the scanning, headphones and cushions were used to diminish noise interferences and avoid head movements. The subjects needed to close their eyes, stay awake and relaxed, avoid thinking about anything special, and avoid head movement. And we used a magnetization-prepared rapid gradient echo sequence to collect the high-resolution T1-weighted images in sagittal orientation. Then, we used an axial T2 fluid-attenuated inversion recovery sequence to remove the clinically silent brain lesions. The rs-fMRI parameters were: TR/TE =2,000/30 ms, flip angle =90°, field of view (FOV) =220 mm × 220 mm, image matrix =64×62, slice thickness =3.5 mm, slice gap =0.5 mm, voxel size =3.44 mm × 3.44 mm × 4 mm, 41 slices. The parameters of T1-weighted structural images are: TR/TE = 7.8/3.46 ms, flip angle =9°, FOV =256 mm × 256 mm, image matrix =256×256, slice thickness =2 mm, voxel size =1.0 mm × 1.0 mm × 1.0 mm, 176 slices.
MRI data preprocessing
As previously described (31), we used GRETNA (http://www.nitrc.org/projects/gretna) software, which was based on MATLAB R 2013b(The Math Works, Natick, Massachusetts, USA) platform and SPM12(http://www.fil.ion.ucl.ac.uk/spm/software/spm12/) software, to preprocess the retrieved fMRI images, and the steps were: (I) removing the first 10 volumes of rest data to ensure magnetization equilibration; (II) correcting slice time; (III) realigning the remaining images to the mean volume for the correction of head movements; (IV) spatially normalizing the images to the Montreal Neurological Institute (MNI) template via the diffeomorphic anatomical registration using the exponentiated Lie algebra (DARTAL) technique (32); (V) to resample the images at a voxel size of 3×3×3 mm; (VI) spatially smoothing the normalized functional data with full width at half maxima (FWHM) Gaussian kernels of 4 mm; (VII) excluding linear and quadratic trends; (VIII) performing nuisance variable regression through regressing out mean signals for white matter, cerebrospinal fluid and Friston-24 head motion parameters; (IX) Finally, minimizing the influences of low-frequency drift and high-frequency physiological noises by using a band-pass (0.01–0.10 Hz) filter (33). All participants were included with our defined head motion threshold (the translation parameter <2 mm, the rotational motion parameter <2°).
Establishment of brain networks
GRETNA toolbox was used to set up the functional brain networks comprising nodes and edges. Each node stood for the brain region, and each edge stood for the connection between 2 nodes. The nodes and edges were defined according to the Brainnetome atlas (34). The Brainnetome Atlas is a fine-grained, cross-validated atlas that associates the anatomy of the brain with psychological functions and contains 210 cortical regions and 36 subcortical regions. For graph analysis, ROI was the node in the functional brain network. Then we averaged the fMRI time courses of all voxels in each ROI, and calculated the mean time series.
As previously described, functional parcellation could improve the reliability of the test-retest network (35). In this study, Pearson correlation coefficients between the regional time series of all pairs of brain regions were measured to assess the FC between regions, and then a correlation matrix (246×246) for every participant was derived. The Pearson correlation was not based on local correlation but had a higher retest network reliability (9). Next, each correlation matrix (Pearson correlation coefficient value) was set as a threshold into an undirected binarization matrix with a fixed sparsity (the sparsity threshold: 0.05–0.4; interval = 0.01) (36,37). A set of 246×246 binarization matrices were retrieved from each object using this threshold.
Graph theory analysis
According to the topology method, we used GRETNA software to calculate global and regional network metrics of the brain network. Global network metrics contained the small-world parameters (clustering coefficient, Cp; characteristic path length, Lp; normalized clustering coefficient γ, normalized characteristic path length λ, and small-worldness σ) and global network efficiency parameters (global efficiency, Eg, local efficiency, and Eloc) (38). The detailed descriptions of the above parameters were: (I) the Cp refers to the possibility of interconnection between neighbors of a node, and its value ranges from 0 to 1. It is a measurement for local network connectivity, and the denser connection, the high Cp; (II) the Lp is the least number of edges connecting two nodes in network. It is a measurement for global network connectivity, and the shorter distance, the lower Lp; (III) the γ quantifies the proportion of the Cp in the real network and the random network. The Cp and the normalized Cp quantify the brain networks; (IV) the λ quantifies the proportion of the Lp between the real network and the random network. The Lp and the normalized Lp represent the functional integration of the brain networks; (V) the σ quantifies the proportion of γ and λ, and it reflects the balance between functional segregation and integration; (VI) the Eg is the reciprocal of Lp, which reflects the average efficiency of the global network and represents the functional integration of the network; (VII) the Eloc refers to the information transfer efficiency of a local network formed by a node and all its neighbors, it is the reciprocal of the characteristic path length of the node, which reflects the average efficiency of the local network.
Nodal network metrics comprised BC, degree centrality (DC), and nodal efficiency (Ne). BC is the number of the shortest paths through a node divided by the number of all possible shortest paths through that node. It reflects the contribution of each node to the shortest path between all other point pairs. DC refers to the number of edges directly connected to a node, which reflects the number of neighbors of the node in the network. Ne refers to the inverse of the average of the shortest path length between a node and all other nodes. Reflects the ability of a node to spread information to other nodes in the network. The above 3 nodal parameters were together used to test the centrality of individual nodes in the network (12).
Finally, each network metric was used to calculate the area under the curve (AUC) over the sparsity. The AUC data have a summary scalar value for the topology characteristics of the brain network, regardless of the single threshold for each network. This method can explore the difference between groups of relative network organizations. The difference was sensitive to the changes of topological properties in brain disorders (39,40).
Statistical analysis
We used SPSS 18.0 to analyze the demographic, clinical, and neuropsychological data. For the data with a normal distribution and homogeneity of variance, we used ANOVA or independent samples t-test to analyze the difference between groups. If there was a significant difference, then the post hoc analysis was conducted. For the data without a normal distribution or variance, Kruskal-Wallis and Mann-Whitney tests were used. For the qualitative variables, the chi-square test was used. The statistical significance level was set at 0.05.
For network topology metrics, we employed univariate analysis of covariance (ANCOVA) to compare differences between the three groups, with age, sex, and education level as covariates (P<0.05, corrected with FDR). Paired post hoc t-tests were conducted on the metrics that detected the significant differences in ANCOVA. Then, a partial correlation analysis was conducted between the network metrics with differences between groups and alertness. We used GRETNA software for statistical analysis of all network parameters.
Validation analysis
For the graph-based network analysis, the node definition was proved to be an important factor (36,39,41). We further assessed whether the primary findings would be affected by distinct types of network establishment strategies. Segmenting brain regions according to functions could promote the functional homogeneity of voxels within a node and described regional brain activities more accurately (41).These type of templates were more suitable for measuring between-group differences in topological properties of brain functional network (42). Therefore, we use three functional templates, including the Dosenbach template of 160 ROIs (Dosenbach-160) (43), the Craddock template of 200 ROIs (Craddock-200) (44), and the Power template of 264 ROIs (Power-264) (45) to construct the network validating the results. We also used these three templates to calculate the global metrics, and then the statistical analysis was performed. Notably, we did not quantify the nodal metrics since it was challenging to match these nodes across different parcellation schemes because of their different spatial locations and network sizes.
Results
Demographic, clinical, and neuropsychological data
As shown in Table 1, the demographic, clinical, and alertness information were summarized and analyzed. The results showed that no differences were found among three groups in age, sex, and education level. Also, no differences in age of onset, seizure frequency, and the Monotherapy/Polytherapy of AEDs between TLE-SD and TLE-LD groups were observed. However, there were significant differences in the RTs of NC and DC among these three groups. Post hoc test showed that no difference between TLE-SD and TLE-LD patients were found, while there were significant differences between TLE-SD or TLE-LD patients and HCs. Also, the RTs in the TLE group were longer than those in the HCs group.
Global properties of the functional brain networks
It was found that all three groups had small-worldness attributes (γ>1, λ ≈ 1, σ>1) based on the previous report (46). The ANCOVA test results were shown in Table 2 and showed that marked differences in Lp, γ, λ, and σ were observed. Also, marked differences in Eg and Eloc were found. The post hoc comparisons showed that the γ, σ, Eg and Eloc were lower, but the Lp was higher in TLE-SD patients than those in HCs (all P<0.05, corrected with FWER). However, there was no difference between the remaining two groups. More detailed data about the global network properties of the TLE-SD and TLE-LD patients and HCs were presented in Figure 1 and Table 2.
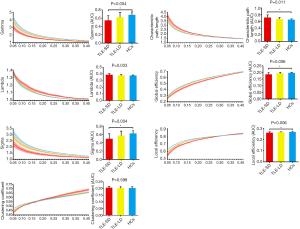
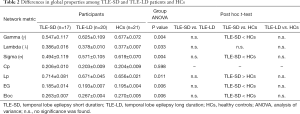
Full table
The global metric data were expressed as mean ± SD. ANOVA was conducted to compare the differences, and then post hoc two independent sample t-test between 2 groups was performed (using FWE correction), with age, sex, and education level as covariates.
Nodal properties of functional brain networks
ANCOVAs showed that there were marked differences in the right medial pre-frontal thalamus (mPFtha) and the right rostral temporal thalamus (rTtha) between DC and Ne groups (all P<0.05, FDR corrected) (Figure 2). Post hoc analysis showed that compared to HCs, the TLE-SD patients showed decreased Ne in the mPFtha and rTtha. However, no marked differences between the rest of the two pairs were observed, as showed by the post hoc analysis. Also, ANCOVAs did not reveal marked differences in BC.
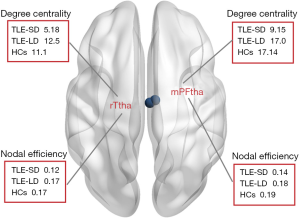
Correlation between network metrics and alertness
The results (Table 3 and Figure 3) showed a positive correlation between the Lp and alertness in TLE-SD patients. And the Eg and alertness in TLE-SD patients and the Eloc and alertness in TLE-SD patients were negatively correlated.
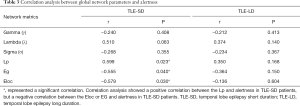
Full table
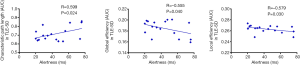
Besides, Pearson correlation analysis was also performed between the nodal network parameters with significant differences and alertness. The result showed only a negative correction between alertness and low Ne values for the mPFtha in the TLE-LD group (Figure 4).
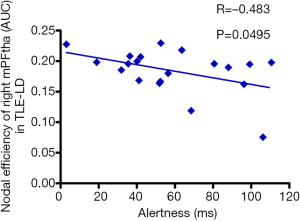
Validation results
We use three functional templates to construct the network and calculated the global metrics, respectively. When using Dosenbach-160 atlas to construct the network, ANCOVA showed marked differences in Lp, γ, σ, and Eg (all P<0.05; Table S1). Post hoc analysis showed that, compared with HCs, TLE-SD patients had lower γ and σ (all P<0.05, corrected with FWER). When using Power-264 atlas to construct the network, we retrieved comparable results (Table S2). When using Craddock-200 to construct the network, we retrieved comparable results to the Brainnetome atlas. However, post hoc analysis revealed that apart from the differences between TLE-SD patients and HCs, significant differences between TLE-SD and TLE-LD patients (TLE-SD patients had lower Eloc than TLE-LD patients) (Table S3).
Discussion
In current study, we investigated the topological alterations of functional brain networks in TLE patients with different durations of disease by using graph theory. The results were: (I) small-world characteristics were observed in both TLE patients and HCs; (II) the global-level finding was that the Eg and Eloc of brain functional networks in TLE-SD patients significantly decreased and tended toward random alterations; (III) the nodal-level finding was that TLE-SD patients had significantly reduced DC and Ne in the mPFtha and the rTtha; (IV) correction analysis showed that alertness was positively related to the Lp but negatively related to the Eg and Eloc in TLE-SD patients; and alertness was negatively correlated with the Ne of mPFtha in TLE-LD patients.
It was also found that the functional brain networks in 3 groups were all characteristic of small-world properties (47). Park et al. (48) reported that the duration of epilepsy was negatively correlated with the Eg in focal epilepsy patients. Paldino et al. (49) found that modularity and, to a lesser extent, path length and Eg were independently related to epilepsy duration in children with focal epilepsy. Our study found that the global topological properties with significant differences among the three groups included Gamma, Lambda, Sigma, Lp, Eg, and Eloc. In previous studies on TLE (50,51), it was found that the topological properties of whole brain functional networks in TLE patients was disrupted, such as the small-worldness, global efficiency and other parameters decreased,and our results were similar . However, post hoc analysis showed a significant difference only between TLE-SD and HCs, while there was no difference between TLE-LD and HCs, which means the functional network of TLE patients has a shorter duration rather than longer duration and is shifting to “randomization”. And the network “randomization” reflects that the local specialization and global integration are reducing. Our previous 2-year longitudinal study on TLE patients (52) found that TLE patients at follow-up showed decreased FC but enhanced amplitude of low-frequency fluctuation (ALFF) compared to HCs at baseline, which suggests that a compensatory reorganization mechanism may exist. Brain compensation represents the ability of the brain to enhance fMRI activity in task-specific networks and to recruit other cortical regions to be beneficial for behavioral performance (53). Many functional neuroimaging reports showed that many patients with neurodegenerative disorders, including Huntington’s disease (54,55), exhibited enhanced fMRI activity and recruited other brain regions to maintain a comparable level of behavioral performance with HCs. Our results showed that no marked differences in sex, age, education level, onset time, frequency of onset and single/multidrug treatment between TLE-SD and TLE-LD patients were observed, as showed by the demographic and clinical data. Therefore, we hypothesize that as the course of the disease prolongs, the brain will develop a modern compensation mechanism to restore Eg and information processing speed. This process is gradual since the course may be 5 years or more because of individual differences. In addition, for the study on the graph-based brain networks, the definition and selection of nodes are important to construct the functional brain networks. In our research, we use 3 different functional network templates to establish the network and calculate the global topological properties, and the results were consistent with our major results, which further validates the reliability of our results.
Our study showed that the significantly decreased nodes in DC and Ne were distributed in the right thalamus (mPFtha and rTtha). Kim et al. (56) found that the duration of disease and the strength of bilateral pre-frontal cortical thalamus function in patients with idiopathic generalized epilepsy were negatively correlated. Lee et al. (57) found thalamic nuclear atrophy in the TLE patients with HS. Morgan et al. (58) found that it was susceptible to TLE seizures for the anterior nucleus and pulvinar of the thalamus as subregions. Also, González et al. (59) found that patients with TLE showed impaired connectivity in thalamic arousal networks, and these disturbances could partially recover following successful epilepsy surgery. Our findings were like the earlier studies showing that the thalamus and its related networks played a crucial role in TLE progression.
Although our behavioral analysis showed that no significant differences in alertness between TLE-LD or TLE-SD patients and HCs were observed, and the RTs of NC and DC of patients were longer than those of HCs, suggesting that there was a potential impairment in the alertness of TLE patients, and which might be more severe in the TLE-LD patients. After analyzing the correlation between the different functional network characteristics and the alertness of TLE, we observed that in the TLE patients with short duration (<5 years), there was a positive correlation between alertness and the Lp, and a negative correlation between alertness and the Eg and Eloc; and there was a negative correlation between alertness and the Ne of mPFtha in the TLE patients with long duration (>5 years). It appears to be a counterintuitive finding. Dinkelacker et al. (60) found a negative correlation between the number of hippocampal-thalamus fiber connections in TLE patients and their performance in some executive tasks. Ellmore et al. (61) reported that the total number of fibers in the hippocampus of TLE patients decreased, but the fractional anisotropy increased. Bonilha et al. (62) found the limbic circuitry in TLE patients paradoxically increased, as showed by a higher Cp and a more substantial nodal degree of the ipsilateral hippocampus thalamus. Therefore, the reorganization of the limbic system might cause neuropsychological abnormalities in TLE. As for the results of the correlation analysis in our research, it is speculated that as the disease progresses, the global network has been reconstructed, and the alertness might be a function that multiple brain regions concurrently process information, rather than as a single group of neurons or a single neuronal circuit. And thalamus might be a critical region for the alertness networks.
There were some limitations in our study. Our study design limited the ability to show how the brain’s functional network dynamically reorganizes as the disease progresses. We also divided the disease into subgroups for five years, which is not strictly in line with reality because the functional reorganization of the brain is a gradual process and may change at other time points. And further longitudinal studies were needed. Because of the small sample size, TLE patients (such as lateralization of epilepsy areas) were not considered in subgroup analysis.
Further research enrolled more participants were needed to increase the reliability of results and to verify the current results. Many clinical factors, including the dose and type of anti-epileptic drugs, also had the potential influences on our findings. However, it is challenging to remove these factors because drug therapy must be taken to prevent the disease from worsening.
In conclusion, the rs-fMRI and graph theory analysis showed that the functional brain network efficiency of TLE patients decreased and was randomized. And as the disease progressed, compensatory reorganization might occur. Thalamus and its related networks might play a key role in TLE progression. A negative relationship between the alertness (neuropsychological testing) and the disruption of the topological properties of the functional network in TLE patients was observed. Our findings might be beneficial for a better understanding of the potential disease progression neuropathophysiological mechanisms of TLE.
Acknowledgments
Funding: This work was supported by the National Natural Science Foundation of China (81560223,81660225) and the Natural Science Foundation of Guangxi Province (2018GXNSFAA050149). The authors thank all participants who contributed to the present study.
Footnote
Reporting Checklist: The authors have completed the MDAR reporting checklist. Available at http://dx.doi.org/10.21037/atm-20-6823
Data Sharing Statement: Available at http://dx.doi.org/10.21037/atm-20-6823
Conflicts of Interest: All authors have completed the ICMJE uniform disclosure form (available at http://dx.doi.org/10.21037/atm-20-6823). The authors have no conflicts of interest to declare.
Ethical Statement: The authors are accountable for all aspects of the work in ensuring that questions related to the accuracy or integrity of any part of the work are appropriately investigated and resolved. All procedures performed in this study involving human participants were in accordance with the Declaration of Helsinki (as revised in 2013). All protocols were allowed by the Ethics Committee of the First Affiliated Hospital of Guangxi Medical University (No. 2020-KY-040). Formal written consent was retrieved from every subject.
Open Access Statement: This is an Open Access article distributed in accordance with the Creative Commons Attribution-NonCommercial-NoDerivs 4.0 International License (CC BY-NC-ND 4.0), which permits the non-commercial replication and distribution of the article with the strict proviso that no changes or edits are made and the original work is properly cited (including links to both the formal publication through the relevant DOI and the license). See: https://creativecommons.org/licenses/by-nc-nd/4.0/.
References
- Téllez-Zenteno JF, Hernandez-Ronquillo L. A review of the epidemiology of temporal lobe epilepsy. Epilepsy Res Treat 2012;2012:630853. [Crossref] [PubMed]
- Spencer SS. Neural networks in human epilepsy: evidence of and implications for treatment. Epilepsia 2002;43:219-27. [Crossref] [PubMed]
- Yang H, Zhang C, Liu C, et al. Brain network alteration in patients with temporal lobe epilepsy with cognitive impairment. Epilepsy Behav 2018;81:41-8. [Crossref] [PubMed]
- Shu Y, Zhu C, Zeng M, et al. The protective effect of carbenoxolone on gap junction damage in the hippocampal CA1 area of a temporal lobe epilepsy rat model. Ann Transl Med 2019;7:624. [Crossref] [PubMed]
- Oyegbile TO, Dow C, Jones J, et al. The nature and course of neuropsychological morbidity in chronic temporal lobe epilepsy. Neurology 2004;62:1736-42. [Crossref] [PubMed]
- Englot DJ, Gonzalez HFJ, Reynolds BB, et al. Relating structural and functional brainstem connectivity to disease measures in epilepsy. Neurology 2018;91:e67-77. [Crossref] [PubMed]
- Posner MI. Measuring alertness. Ann N Y Acad Sci 2008;1129:193-9. [Crossref] [PubMed]
- Sturm W, Willmes K. On the functional neuroanatomy of intrinsic and phasic alertness. Neuroimage 2001;14:S76-84. [Crossref] [PubMed]
- Liu J, Zhou X, Zhang Z, et al. Disrupted functional network in patients with temporal lobe epilepsy with impaired alertness. Epilepsy Behav 2019;101:106573. [Crossref] [PubMed]
- Zhou M, Jiang W, Zhong D, et al. Resting-state brain entropy in right temporal lobe epilepsy and its relationship with alertness. Brain Behav 2019;9:e01446. [Crossref] [PubMed]
- Cheung MC, Chan AS, Chan YL, et al. Effects of illness duration on memory processing of patients with temporal lobe epilepsy. Epilepsia 2006;47:1320-8. [Crossref] [PubMed]
- Li H, Fan W, Yang J, et al. Asymmetry in cross-hippocampal connectivity in unilateral mesial temporal lobe epilepsy. Epilepsy Res 2015;118:14-21. [Crossref] [PubMed]
- Haneef Z, Levin HS, Chiang S. Brain Graph Topology Changes Associated with Anti-Epileptic Drug Use. Brain Connect 2015;5:284-91. [Crossref] [PubMed]
- Haneef Z, Chiang S, Yeh HJ, et al. Functional connectivity homogeneity correlates with duration of temporal lobe epilepsy. Epilepsy Behav 2015;46:227-33. [Crossref] [PubMed]
- Hughes DM, Bonnett LJ, Czanner G, et al. Identification of patients who will not achieve seizure remission within 5 years on AEDs. Neurology 2018;91:e2035-44. [Crossref] [PubMed]
- Rathouz PJ, Zhao Q, Jones JE, et al. Cognitive development in children with new onset epilepsy. Dev Med Child Neurol 2014;56:635-41. [Crossref] [PubMed]
- Biswal B, Yetkin FZ, Haughton VM, et al. Functional connectivity in the motor cortex of resting human brain using echo-planar MRI. Magn Reson Med 1995;34:537-41. [Crossref] [PubMed]
- Fox MD, Raichle ME. Spontaneous fluctuations in brain activity observed with functional magnetic resonance imaging. Nat Rev Neurosci 2007;8:700-11. [Crossref] [PubMed]
- Biswal BB, Mennes M, Zuo XN, et al. Toward discovery science of human brain function. Proc Natl Acad Sci U S A 2010;107:4734-9. [Crossref] [PubMed]
- Kelly C, Biswal BB, Craddock RC, et al. Characterizing variation in the functional connectome: promise and pitfalls. Trends Cogn Sci 2012;16:181-8. [Crossref] [PubMed]
- Bullmore E, Sporns O. Complex brain networks: graph theoretical analysis of structural and functional systems. Nat Rev Neurosci 2009;10:186-98. [Crossref] [PubMed]
- He Y, Evans A. Graph theoretical modeling of brain connectivity. Curr Opin Neurol 2010;23:341-50. [PubMed]
- Liao X, Vasilakos AV, He Y. Small-world human brain networks: Perspectives and challenges. Neurosci Biobehav Rev 2017;77:286-300. [Crossref] [PubMed]
- Vaessen MJ, Jansen JF, Vlooswijk MC, et al. White matter network abnormalities are associated with cognitive decline in chronic epilepsy. Cereb Cortex 2012;22:2139-47. [Crossref] [PubMed]
- Bassett DS, Bullmore ET. Human brain networks in health and disease. Curr Opin Neurol 2009;22:340-7. [Crossref] [PubMed]
- Zhang LJ, Zheng G, Zhang L, et al. Disrupted small world networks in patients without overt hepatic encephalopathy: a resting state fMRI study. Eur J Radiol 2014;83:1890-9. [Crossref] [PubMed]
- Glauser T, Ben-Menachem E, Bourgeois B, et al. ILAE treatment guidelines: evidence-based analysis of antiepileptic drug efficacy and effectiveness as initial monotherapy for epileptic seizures and syndromes. Epilepsia 2006;47:1094-120. [Crossref] [PubMed]
- Scheffer IE, Berkovic S, Capovilla G, et al. ILAE classification of the epilepsies: Position paper of the ILAE Commission for Classification and Terminology. Epilepsia 2017;58:512-21. [Crossref] [PubMed]
- Fan J, McCandliss BD, Sommer T, et al. Testing the efficiency and independence of attentional networks. J Cogn Neurosci 2002;14:340-7. [Crossref] [PubMed]
- Fan J, McCandliss BD, Fossella J, et al. The activation of attentional networks. Neuroimage 2005;26:471-9. [Crossref] [PubMed]
- Wang J, Wang X, Xia M, et al. GRETNA: a graph theoretical network analysis toolbox for imaging connectomics. Front Hum Neurosci 2015;9:386. [PubMed]
- Ashburner J. A fast diffeomorphic image registration algorithm. Neuroimage 2007;38:95-113. [Crossref] [PubMed]
- Liu X, Duyn JH. Time-varying functional network information extracted from brief instances of spontaneous brain activity. Proc Natl Acad Sci U S A 2013;110:4392-7. [Crossref] [PubMed]
- Fan L, Li H, Zhuo J, et al. The Human Brainnetome Atlas: A New Brain Atlas Based on Connectional Architecture. Cereb Cortex 2016;26:3508-26. [Crossref] [PubMed]
- Gong Q, He Y. Depression, neuroimaging and connectomics: a selective overview. Biol Psychiatry 2015;77:223-35. [Crossref] [PubMed]
- Fornito A, Zalesky A, Bullmore ET. Network scaling effects in graph analytic studies of human resting-state FMRI data. Front Syst Neurosci 2010;4:22. [PubMed]
- Braun U, Plichta MM, Esslinger C, et al. Test-retest reliability of resting-state connectivity network characteristics using fMRI and graph theoretical measures. Neuroimage 2012;59:1404-12. [Crossref] [PubMed]
- Rubinov M, Sporns O. Complex network measures of brain connectivity: uses and interpretations. Neuroimage 2010;52:1059-69. [Crossref] [PubMed]
- Wang J, Wang L, Zang Y, et al. Parcellation-dependent small-world brain functional networks: a resting-state fMRI study. Hum Brain Mapp 2009;30:1511-23. [Crossref] [PubMed]
- Zhang J, Wang J, Wu Q, et al. Disrupted brain connectivity networks in drug-naive, first-episode major depressive disorder. Biol Psychiatry 2011;70:334-42. [Crossref] [PubMed]
- Wig GS, Schlaggar BL, Petersen SE. Concepts and principles in the analysis of brain networks. Ann N Y Acad Sci 2011;1224:126-46. [Crossref] [PubMed]
- Chen X, Liao X. Topological analyses of functional connectomics: A crucial role of global signal removal, brain parcellation, and null models. 2018;39:4545-64.
- Dosenbach NU, Nardos B, Cohen AL, et al. Prediction of individual brain maturity using fMRI. Science 2010;329:1358-61. [Crossref] [PubMed]
- Craddock RC, James GA, Holtzheimer PE 3rd, et al. A whole brain fMRI atlas generated via spatially constrained spectral clustering. Hum Brain Mapp 2012;33:1914-28. [Crossref] [PubMed]
- Power JD, Cohen AL, Nelson SM, et al. Functional network organization of the human brain. Neuron 2011;72:665-78. [Crossref] [PubMed]
- Watts DJ, Strogatz SH. Collective dynamics of 'small-world' networks. Nature 1998;393:440-2. [Crossref] [PubMed]
- Sporns O, Chialvo DR, Kaiser M, et al. Organization, development and function of complex brain networks. Trends Cogn Sci 2004;8:418-25. [Crossref] [PubMed]
- Park KM, Lee BI, Shin KJ, et al. Progressive topological disorganization of brain network in focal epilepsy. 2018;137:425-31.
- Paldino MJ, Zhang W, Chu ZD, et al. Metrics of brain network architecture capture the impact of disease in children with epilepsy. Neuroimage Clin 2016;13:201-8. [Crossref] [PubMed]
- Liu M, Chen Z, Beaulieu C, et al. Disrupted anatomic white matter network in left mesial temporal lobe epilepsy. Epilepsia 2014;55:674-82. [Crossref] [PubMed]
- Jiang W, Li J, Chen X, et al. Disrupted Structural and Functional Networks and Their Correlation with Alertness in Right Temporal Lobe Epilepsy: A Graph Theory Study. Front Neurol 2017;8:179. [Crossref] [PubMed]
- Zhang Z, Zhou X, Liu J, et al. Longitudinal assessment of resting-state fMRI in temporal lobe epilepsy: A two-year follow-up study. Epilepsy Behav 2020;103:106858. [Crossref] [PubMed]
- Barch DM, Burgess GC, Harms MP, et al. Function in the human connectome: task-fMRI and individual differences in behavior. Neuroimage 2013;80:169-89. [Crossref] [PubMed]
- Poudel GR, Stout JC, Dominguez DJ, et al. Functional changes during working memory in Huntington's disease: 30-month longitudinal data from the IMAGE-HD study. Brain Struct Funct 2015;220:501-12. [Crossref] [PubMed]
- D JF, Stout JC, Poudel G, et al. Multimodal imaging biomarkers in premanifest and early Huntington's disease: 30-month IMAGE-HD data. Br J Psychiatry 2016;208:571-8. [Crossref] [PubMed]
- Kim JB, Suh SI, Seo WK, et al. Altered thalamocortical functional connectivity in idiopathic generalized epilepsy. Epilepsia 2014;55:592-600. [Crossref] [PubMed]
- Lee HJ, Seo SA, Park KM. Quantification of thalamic nuclei in patients diagnosed with temporal lobe epilepsy and hippocampal sclerosis. Neuroradiology 2020;62:185-95. [Crossref] [PubMed]
- Morgan VL, Rogers BP, Abou-Khalil B. Segmentation of the thalamus based on BOLD frequencies affected in temporal lobe epilepsy. Epilepsia 2015;56:1819-27. [Crossref] [PubMed]
- González HFJ, Chakravorti S, Goodale SE, et al. Thalamic arousal network disturbances in temporal lobe epilepsy and improvement after surgery. J Neurol Neurosurg Psychiatry 2019;90:1109-16. [Crossref] [PubMed]
- Dinkelacker V, Valabregue R, Thivard L, et al. Hippocampal-thalamic wiring in medial temporal lobe epilepsy: Enhanced connectivity per hippocampal voxel. Epilepsia 2015;56:1217-26. [Crossref] [PubMed]
- Ellmore TM, Pieters TA, Tandon N. Dissociation between diffusion MR tractography density and strength in epilepsy patients with hippocampal sclerosis. Epilepsy Res 2011;93:197-203. [Crossref] [PubMed]
- Bonilha L, Nesland T, Martz GU, et al. Medial temporal lobe epilepsy is associated with neuronal fibre loss and paradoxical increase in structural connectivity of limbic structures. J Neurol Neurosurg Psychiatry 2012;83:903-9. [Crossref] [PubMed]
(English Language Editor: J. Chapnick)