Upregulation of STAT1-CCL5 axis is a biomarker of colon cancer and promotes the proliferation of colon cancer cells
Introduction
Colorectal cancer (CRC) was the third most commonly diagnosed cancer in men and women in 2019 (1). The statistical data of the American Cancer Society showed that CRC is the second leading cause of cancer-related death in the United States (1). Improvements in cancer prevention, early diagnosis, and treatment strategies leaded to a 35% decrease in CRC-associated mortality between 1990 and 2007 (2). Encouragingly, the advancements in precision medicine have further improved the outcomes of patients without compromising treatment effects. Nevertheless, a recent report presented an unsatisfactory 5-year survival rate for stage IV CRC patients of about 14% (3). With the developments of gene sequencing techniques, there is a growing body of evidence that indicates that some genetic alterations, such as those in KRAS, NRAS, epidermal growth factor receptor (EGFR), as well as APC, are closely related to carcinogenesis and poor clinical outcomes in CRC (4). For colon cancer patients, these gene expression statuses not only predict prognosis but also herald the efficacy of some regimens such as bevacizumab, cetuximab, and panitumumab (5). Therefore, investigating genic background differences between normal and colon cancer cells would be significant to identifying some key oncogenic pathways and understanding the malignant biological behaviors of cancer cells, and may also provide valuable information for the development of anti-cancer drugs.
In the present study, we calculated the correlation values of genes through a WGCNA-based systemic biology method and identified a novel signaling pathway associated with the development of colon cancer and the prognosis of patients. This study was based on dataset GSE29612. The raw gene expression data of GSE29621 were provided by Chen et al., and the pre-processed gene matrix was downloaded from the Gene Expression Omnibus (GEO) database (https://www.ncbi.nlm.nih.gov/geo) (6). GSE29612 contains the expression profiles of 65 colon cancer samples (tumor purity >90%, without genetic cancer syndromes and inflammatory bowel disease) (6).
Methods
Data cleaning and processing
Figure 1 shows the workflow followed in this study. The gene expression values were detected with the Affymetrix Genechip Human Genome U133 Plus 2.0 Array and were further transformed using the Robust Multi-array Average (RMA) algorithm. Then, based on the results of variance analysis, the top 50% of the variant genes (10,826 genes) were used in the following WGCNA analysis.
Constructing co-expression network
To construct the co-expression network, the gene expression matrices of 10,826 genes were extracted by WGCNA package (version of R software: 3.6.0). The analysis was performed as previously described (7). The connection strength between two nodes was calculated by adjacency matrix aij using the following formula:
[1]
In the formula, xi and xj represent the vectors of the expression data of genes i and j. Sij represents the Pearson’s correlation coefficients of xi and xj. aij reflects the network connection strength between two genes. In this study, β =5 was served as the soft-threshold value for constructing the scale-free network (scale-free R2 >0.85). In WGCNA analysis, the correlations between two connected genes as well as the correlations between multiple associated genes are vital parameters. A series of modules were generated by hierarchical clustering of weighted coefficient matrix. Then, topological overlap measure (TOM) was calculated using the following formula:
[2]
In this formula, Ai,j represents weighted adjacency matrix calculated by Ai,j=|cor(xi,xj)|β. TOM-based dissimilarity method (minimized gene group =50) was employed to perform average linkage hierarchical clustering. The DynamicTreeCut algorithm was used to distribute genes with similar expression patterns into the same module.
Identifying clinically significant modules
Two parameters, module gene significance (MS) and module eigengenes (MEs), were adopted to evaluate the correlations between the clinical traits of patients and clustered modules. As the first principal component, the expression levels of all the genes in one clustered module could be profiled with ME. Gene significance (GS) refers to the absolute values of P in the linear regression analysis. MS was calculated based on the average GS values of all the genes in each module.
KEGG pathway and GO term enrichment analysis
As previously described, the enrichment analysis was conducted using the online analysis tool G: Profiler (https://biit.cs.ut.ee/gprofiler/) (version: e98_eg45_p14_ce5b097). The significantly enriched KEGG pathways and GO terms were identified with <0.05 serving as the cutoff for determining the false positive rate.
Hub genes
Hub genes were screened out by calculating their connectivity with other genes (|cor.geneModuleMembership| ≥0.8). After that, the hub genes had to meet the criterion |cor.geneTraitSignificance| ≥0.2. To further investigate the role of hub genes in CRC, the gene expression matrix was downloaded from the GEO, the Cancer Cell Line Encyclopedia, The Cancer Genome Atlas (TCGA), and the Genotype-Tissue Expression (GTEx) databases. Comparisons of hub gene expression levels between tumor and normal tissues were performed using the online Gene Expression Profiling Interactive Analysis (GEPIA) tool (http://gepia.cancer-pku.cn/).
Cell counting
To evaluate the effect of two hub genes, STAT1 and CCL5, on colon cancer cells, colon cancer cell lines HCT-116, SW480, and SW620 were cultured in high-glucose Dulbecco’s Modified Eagle Medium (DMEM) supplemented with 10% or 1% fetal bovine serum (FBS) at 37 °C with 5% CO2. After the cells were incubated with STAT1 inhibitor Fludarabine (10 µM, Catalog No: HY-B0069, MCE) (8) and CCL5 neutralizing antibody (1 µg/mL, Catalog No: 12000-1-AP, Proteintech), the number of cells of the three cancer cell lines were counted with a cell counting chamber.
EdU assay
An EdU assay was conducted according to the manufacturer’s instructions (Cell-LightTM EdU Apollo 567 Kit, Catalog No: C10310-1, RIBOBIO) (9). Firstly, cells were seeded in 24-well plates at a density of 3×103 per well and incubated for 2 days. Next, the cells were stimulated for 12 h with or without 10% FBS. Then, 250 µL EdU medium was added to each well. After incubation lasting 2 h, the cells were fixed with 4% paraformaldehyde for 20 min. Subsequently, glycine solution was added to neutralize aldehydes. After the permeability of the cell membrane had been increased with 0.5% TritonX-100 in PBS, the cells were incubated with Apollo dyeing reaction solution in the dark for 30 min. Nuclei were counterstained with Hoechst 33342. Finally, fluorescent images were taken at 200× and the positive cells were counted in 5 fields per well.
Small-interfering RNA (Si-RNA) transfection
Si-RNA of STAT1 was purchased from RiboBio Co., Ltd. (Guangzhou). Tumor cells were seeded at a density of 2×106 cells in a 6-well plate on the day prior to transfection. The transfection was performed using Hieff Trans™ in vitro siRNA/miRNA Transfection Reagent (Yeasen Biotech Co., Ltd.) according to the manufacturer’s recommendations. Twenty picomole Si-RNA was required for each well.
Real-time PCR analysis
The primers of STAT1 were designed as follows: forward primer, 5'-CAGCTTGACTCAAAATTCCTGGA-3'; reverse primer, 5'- TGAAGATTACGCTTGCTTTTCCT-3'. The primers of CCL5 were designed as follows: forward primer, 5'-CCAGCAGTCGTCTTTGTCAC-3'; reverse primer, 5'-CTCTGGGTTGGCACACACTT-3'. Total mRNA was extracted using FastPure Cell/Tissue Total RNA Isolation Kit (Vazyme) according to the manufacturer’s protocol. Approximately 500 ng total mRNA was reversely transcribed to complementary DNA (cDNA) using HiScript II Q Select RT SuperMix for qPCR (Vazyme). Quantification reactions were performed with ABI 7500 platform. Samples were amplified using the following conditions: 95 °C for 30 s, 45 cycles of 95 °C for 15 s, 60 °C for 60 s. The expression fold changes were calculated with the formula: 2−∆∆CT (10).
CCK-8 assay
Cell viability was measured using CCK-8 cell proliferation kit (Dojindo). Ten µL CCK-8 reagent was added into 100 µL cell culture medium. After incubation for 2.5 hours in 37 °C, the absorbance values were measured at 450 nm with the microplate reader (Molecular Devices).
CFSE staining and flow cytometry
SW480 and SW620 were labeled with 2.5 µM CFSE (Invitrogen). Then, cells were seeded at the density of 1 × 106 per well in 6-well plates and cultured with RPMI-1640 media containing 10% FBS. After culture for 2 days, we performed flow cytometry assay to measure the ratio of proliferating cells (CFSE-low) with BD Celesta platform. The data of flow cytometry were analyzed with Flow Jo software (Flow Jo LLC, Ashland, USA).
Results
Constructing the co-expression network and identifying clinically significant modules
Patients’ clinical traits were summarized in Table 1. After Pearson’s correlation coefficients and average strength were calculated, the 65 samples of GSE29621 were clustered (Figure 2). Then, with the soft-thresholding power as β =5 (R2 >0.85), the co-expression analysis was conducted by generating a scale-free network. Based on an average hierarchical clustering algorithm, 26 modules were generated (Figure 3). Notably, the turquoise module possessed a strong correlation with CRC differentiation grade (R =0.53, P<0.0001) (Figure 4, Table 2). Thus, the turquoise module was identified as having clinical significance.
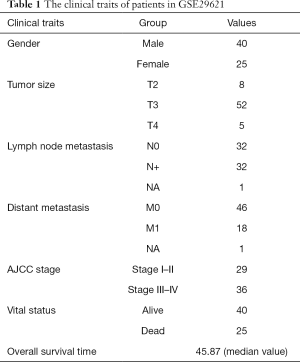
Full table
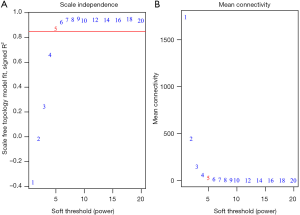
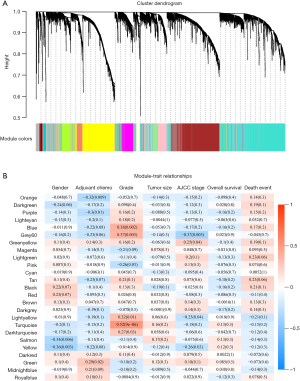
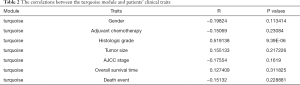
Full table
The results of KEGG pathway and GO term enrichment analyses
The turquoise module was a highly complicated gene panel comprising 1,723 genes. Therefore, the biological functions of the turquoise module were profiled through KEGG pathway analysis and GO term enrichment analysis. The results of enrichment analysis showed that the genes in the turquoise module were significantly enriched in inflammation-associated pathways such as the B-cell receptor signaling pathway, antigen processing and presentation, the chemokine signaling pathway, cytokine-cytokine receptor interaction, the NF-κB pathway, PD-L1 expression, and the PD-1 pathway in cancer (Figure 5). Furthermore, this panel of genes was remarkably enriched in extracellular cytokine release processes including in the cytokine-mediated signaling pathway, extracellular regions, and chemokine receptor activity. Cytoscape software (software version: 3.6.0) was employed to construct the interaction networks of GO terms (Figures 6 and 7).
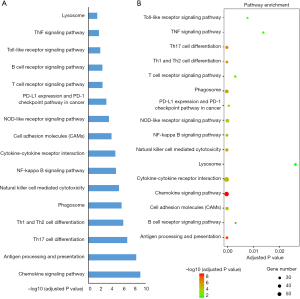
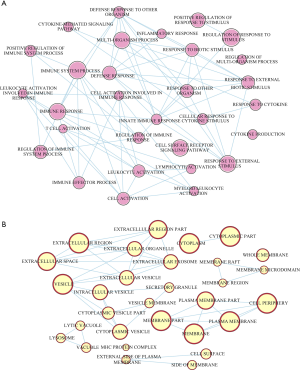
The identification and verification of hub genes
A total of 82 genes in the turquoise module met the criteria of |cor.geneModuleMembership| ≥0.8 and |cor.geneTraitSignificance| ≥0.2 and were subsequently screened out as hub genes. Among these 82 genes, two genes, STAT1 and CCL5, stood out. The results from the TCGA and GTEx databases showed that the mRNA levels of STAT1 and CCL5 were significantly elevated in CRC tissues compared with normal colon tissues (Figure 8). In addition, the correlation analysis of the TGCA, GEO, and Cancer Cell Line Encyclopedia databases indicated that the expression of STAT1 was closely correlated with that of CCL5 (P<0.0001) (Figure 9A,B,C,D). Moreover, in SW489 and SW620 cells, when STAT1 expression was inhibited by Si-STAT1, the level of CCL5 was decreased simultaneously (Figure 9E,F).
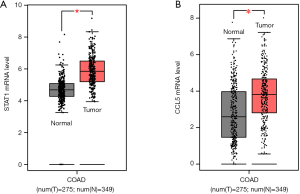
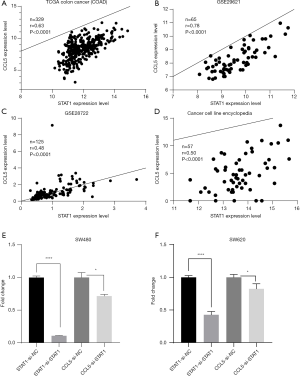
STAT1-CCL5 pathway promoting the proliferation of colon cancer cells
For the cancer cells cultured with medium containing 10% FBS, STAT1 inhibitor and CCL5-neutralizing antibody exhibited anti-proliferative activity. After the HCT-116, SW480, and SW620 cells underwent STAT1 inhibitor treatment at 10 µM, a significant decrease was observed in their numbers. Meanwhile, CCL5-neutralizing antibody at 1 µg/mL also decreased the number of cancer cells in the culture medium with 10% FBS (a decreasing trend was observed, but statistical significance was not reached in the HCT-116 cells) (Figure 10). The results of the EdU assay showed that treatment with STAT1 inhibitor remarkably reduced the number of EdU-positive cancer cells (Figure 11). Moreover, we used medium with 1% FBS to minimize the potential influence of growth factors from serum. Similar to the findings under the conditions of 10% FBS, blocking STAT1 and CCL5 inhibited the proliferation of three cancer cell types.
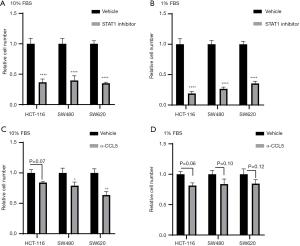
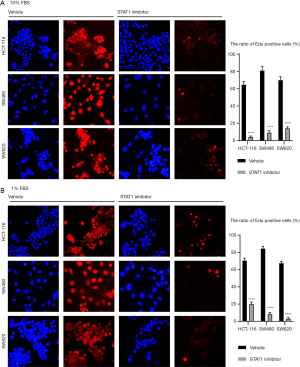
In addition, we used Si-STAT1 to explore the effect of STAT1 on colon cancer cell’s proliferation in SW480 and SW620 cells. The results of CFSE staining assay indicated that Si-STAT1 treatment significantly reduced the ratio of proliferating (CFSE-low) colon cancer cells (Figure 12A,B). The results of CCK-8 and cell number counting assays also demonstrated that Si-STAT1 impaired the proliferation of colon cancer cells (Figure 12C,D).
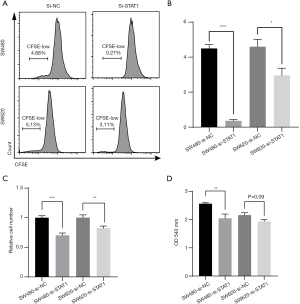
Discussion
Both sporadic CRC and colitis-associated cancer are well known to be related to inflammation. At some stages of cancer, the localized inflammation not only counteracts the anti-tumor immune response through an immunosuppressive effect, but it also participates in the proliferation, survival, and metastasis of CRC cells (11). Before the tumor lesion forms, cytokines from inflammatory cells may upregulate the generation of reactive nitrogen intermediates (RNI) and reactive oxygen species (ROS) (12). Terzic et al. found that intracellular RNI and ROS in pre-malignant cells silenced tumor suppressors through epigenetic alterations and promoted tumor initiation (11). Their study also showed that, apart from inducing the initiation of cancer cells, multiple pro-tumor cytokines could activate the receptors on colon cancer cells, which subsequently activated intracellular oncogenic signaling pathways or transcriptional factors such as NF-κB, AP-1, and STAT3 (11). These oncogenic signals regulate a wide range of biological processes including cell proliferation, survival, and apoptosis (13,14). Furthermore, Li et al. also found that the activation of these oncogenic signals enhanced chemokine secretion, recruited more inflammatory cells, and self-propagated localized inflammation (15). In advanced colon cancer, some proinflammatory cytokines including IL-1, TNF-α, and IL-6 activate transcriptional factors such as ZEB, Twist, and Snail, which promote epithelial-mesenchymal transition and increase the migratory properties of cancer cells (11). In this study, we comprehensively analyzed gene expression data from the TCGA, GTEx, GEO, and Cancer Cell Line Encyclopedia (CCLE) databases. We found that several tumor immune microenvironment-related signals played a key role in CRC.
The present WGCNA analysis was performed based on the GSE29621 dataset with the aim of identifying the co-expression modules associated with tumorigenesis or outcomes of CRC. To save computational memory, the most differentially expressed genes (in the top 50%) were used to construct a weighted co-expression network. Subsequently, the turquoise module was identified as a clinically significant module. Further enrichment analysis showed that the genes of the turquoise module were remarkably enriched in multiple inflammatory processes and pathways, such as cytokine pathways, chemokine signaling, and immunosuppressive pathways. These results were consistent with previous studies. Chronically localized inflammation not only induces the initiation of CRC, but also promotes the progression of CRC. Among all hub genes in the turquoise module, the expressions of two genes, STAT1 and CCL5, were highly positively correlated. Moreover, the mRNA levels of STAT1 and CCL5 were both significantly elevated in CRC compared with normal colon tissues. This finding indicated that STAT1 and CCL5 overexpression might be valuable biomarkers for predicting the progression of CRC.
STAT1 is a vital participator of innate immunity, protecting the host from infection by pathogens. As a downstream mediator of interferon receptors, STAT1 regulates the expression of multiple anti-pathogens and immunoregulatory genes (16). STAT1 is generally believed to have a bi-directional role in different cancer cells. In some cancers, STAT1 performs its anti-tumor role by enhancing immune surveillance and increasing the tumor-killing effect of natural killer (NK) cells (17). STAT1 has also been shown to suppress the proliferation of cancer cells via the upregulation of cyclin-dependent kinase inhibitor expression or the downregulation of c-myc expression (18,19). In other cancers, such as leukemia, STAT1 promotes leukemogenesis by inhibiting immune clearance (20). Moreover, in some solid tumors, STAT1 increases the expression of several interferon-inducible genes and repairs treatment-mediated DNA damage, and is highly related to chemotherapy resistance (21). Wang et al. reported that STAT1 exhibited pro-survival effect in CRC by increasing PI3K class IB signaling and decreasing the expression of programmed cell death protein 4 (22). Furthermore, when STAT1 signaling was blocked, they also observed that the tumorigenic potency was impaired and there was reduced sensitivity to the inhibition of translation initiation factor eIF4A (22). For some oncogenes, such as CUG2, oncogenic signaling pathway-mediated STAT1 activation significantly enhances the metastatic and drug-resistance properties of colon cancer cells (23). In our study, STAT1 inhibitor markedly suppressed the proliferation of multiple colon cancer cell lines, which suggested STAT1 might contribute to cancer progression.
CCL5, which is also known as RANTES, is a C-C chemokine secreted by immune cells, fibroblasts, and epithelial cells (24). CCL5 was originally found to be an inducer, recruiting leukocytes to inflammatory sites (24). Further investigations showed it to be overexpressed in multiple cancers (25,26). Elevated CCL5 promotes cancer development through regulating cell proliferation, the generation of matrix metalloproteinase, and angiogenesis (27,28). Recent studies demonstrated that increased CCL5 had a substantial influence during the development of CRC. In CRC, CCL5 not only promotes growth and metastasis, but also undermines immune surveillance by increasing the ratio of Tregs and myeloid-derived suppressor cells (29-31). Furthermore, CCL5-β-catenin-Slug has been shown to be a core pathway by which epithelial-mesenchymal transition of CRC is induced (32). In the present study, neutralizing CCL5 significantly decreased the proliferation of CRC, indicating that CCL5 might serve as a potential target for CRC treatment.
Previous studies have shown that STAT1-CCL5 axis is the downstream pathway of cancer-associated molecules such as interleukin 35 and interferon-induced protein 35 (33,34). In pancreatic ductal adenocarcinoma, the activated STAT1-CCL5 pathway promoted micro-vessel formation and monocyte infiltration (34). However, to our knowledge, the role of the STAT1-CCL5 axis in CRC has not been systemically studied. The results of our study show that increased levels of STAT1 and CCL5 might be potential biomarkers for CRC screening. The STAT1-CCL5 axis may be a vital regulator in the development of CRC through its promotion of cell proliferation.
Conclusions
In conclusion, using WGCNA big data mining, we screened out a clinically significant module. The genes of this module were remarkably enriched in inflammation and cytokine-associated pathways. Among all of the hub genes of the clinically significant module, the mRNA levels of STAT1 and CCL5 were significantly higher in CRC than in normal colon tissues. STAT1 expression was highly positively correlated with CCL5. The results of in vitro study showed that interfering with STAT1 and CCL5 could inhibit the proliferation of CRC cells. Our study indicated that the STAT1-CCL5 axis is an important modulator of CRC development through its regulation of cell proliferation. The levels of STAT1 and CCL5 may become valuable biomarkers for CRC screening.
Acknowledgments
Funding: This work was supported by the National Natural Science Foundation of China (No. 81874120, 81572608), Wuhan Science and Technology Bureau (No. 2017060201010170), National Cancer Center Climbing Foundation Key Project (NCC201816B046), and Henan Science and Technology Open Cooperation Project (No. 162106000021).
Footnote
Conflicts of Interest: All authors have completed the ICMJE uniform disclosure form (available at http://dx.doi.org/10.21037/atm-20-4428).The authors have no conflicts of interest to declare.
Ethical Statement: The authors are accountable for all aspects of the work in ensuring that questions related to the accuracy or integrity of any part of the work are appropriately investigated and resolved.
Open Access Statement: This is an Open Access article distributed in accordance with the Creative Commons Attribution-NonCommercial-NoDerivs 4.0 International License (CC BY-NC-ND 4.0), which permits the non-commercial replication and distribution of the article with the strict proviso that no changes or edits are made and the original work is properly cited (including links to both the formal publication through the relevant DOI and the license). See: https://creativecommons.org/licenses/by-nc-nd/4.0/.
References
- Siegel RL, Miller KD, Jemal A. Cancer statistics, 2019. CA Cancer J Clin 2019;69:7-34. [Crossref] [PubMed]
- Roselló S, Simón S, Cervantes A. Programmed colorectal cancer screening decreases incidence and mortality. Transl Gastroenterol Hepatol 2019;4:84. [Crossref] [PubMed]
- Mattiuzzi C, Sanchis-Gomar F, Lippi G. Concise update on colorectal cancer epidemiology. Ann Transl Med 2019;7:609. [Crossref] [PubMed]
- Das S, Ciombor KK, Haraldsdottir S, et al. Promising New Agents for Colorectal Cancer. Curr Treat Options Oncol 2018;19:29. [Crossref] [PubMed]
- He L, Wang F, Tian H, et al. The expression profile of RNA sensors in colorectal cancer and its correlation with cancer stages. Transl Cancer Res 2019;8:1351-63. [Crossref]
- Chen DT, Hernandez JM, Shibata D, et al. Complementary strand microRNAs mediate acquisition of metastatic potential in colonic adenocarcinoma. J Gastrointest Surg 2012;16:905-12; discussion 912-3. [Crossref] [PubMed]
- Langfelder P, Horvath S. WGCNA: an R package for weighted correlation network analysis. BMC Bioinformatics 2008;9:559. [Crossref] [PubMed]
- Liu J, Qu L, Meng L, et al. Topoisomerase inhibitors promote cancer cell motility via ROS-mediated activation of JAK2-STAT1-CXCL1 pathway. J Exp Clin Cancer Res 2019;38:370. [Crossref] [PubMed]
- Xu H, Jiao Y, Yi M, et al. EYA2 Correlates With Clinico-Pathological Features of Breast Cancer, Promotes Tumor Proliferation, and Predicts Poor Survival. Front Oncol 2019;9:26. [Crossref] [PubMed]
- Liu Q, Li A, Yu S, et al. DACH1 antagonizes CXCL8 to repress tumorigenesis of lung adenocarcinoma and improve prognosis. J Hematol Oncol 2018;11:53. [Crossref] [PubMed]
- Terzic J, Grivennikov S, Karin E, et al. Inflammation and colon cancer. Gastroenterology 2010;138:2101-14.e5. [Crossref] [PubMed]
- Meira LB, Bugni JM, Green SL, et al. DNA damage induced by chronic inflammation contributes to colon carcinogenesis in mice. J Clin Invest 2008;118:2516-25. [PubMed]
- Grivennikov S, Karin E, Terzic J, et al. IL-6 and Stat3 are required for survival of intestinal epithelial cells and development of colitis-associated cancer. Cancer Cell 2009;15:103-13. [Crossref] [PubMed]
- Bollrath J, Greten FR. IKK/NF-kappaB and STAT3 pathways: central signalling hubs in inflammation-mediated tumour promotion and metastasis. EMBO Rep 2009;10:1314-9. [Crossref] [PubMed]
- Li B, Vincent A, Cates J, et al. Low levels of tumor necrosis factor alpha increase tumor growth by inducing an endothelial phenotype of monocytes recruited to the tumor site. Cancer Res 2009;69:338-48. [Crossref] [PubMed]
- Stark GR, Darnell JE Jr. The JAK-STAT pathway at twenty. Immunity 2012;36:503-14. [Crossref] [PubMed]
- Lee CK, Rao DT, Gertner R, et al. Distinct requirements for IFNs and STAT1 in NK cell function. J Immunol 2000;165:3571-7. [Crossref] [PubMed]
- Ramana CV, Grammatikakis N, Chernov M, et al. Regulation of c-myc expression by IFN-gamma through Stat1-dependent and -independent pathways. Embo j 2000;19:263-72. [Crossref] [PubMed]
- Chin YE, Kitagawa M, Su WC, et al. Cell growth arrest and induction of cyclin-dependent kinase inhibitor p21 WAF1/CIP1 mediated by STAT1. Science 1996;272:719-22. [Crossref] [PubMed]
- Kovacic B, Stoiber D, Moriggl R, et al. STAT1 acts as a tumor promoter for leukemia development. Cancer Cell 2006;10:77-87. [Crossref] [PubMed]
- Khodarev NN, Roizman B, Weichselbaum RR. Molecular pathways: interferon/stat1 pathway: role in the tumor resistance to genotoxic stress and aggressive growth. Clin Cancer Res 2012;18:3015-21. [Crossref] [PubMed]
- Wang S, Darini C, Desaubry L, et al. STAT1 Promotes KRAS Colon Tumor Growth and Susceptibility to Pharmacological Inhibition of Translation Initiation Factor eIF4A. Mol Cancer Ther 2016;15:3055-63. [Crossref] [PubMed]
- Malilas W, Koh SS, Kim S, et al. Cancer upregulated gene 2, a novel oncogene, enhances migration and drug resistance of colon cancer cells via STAT1 activation. Int J Oncol 2013;43:1111-6. [Crossref] [PubMed]
- Kan JY, Wu DC, Yu FJ, et al. Chemokine (C-C Motif) Ligand 5 is Involved in Tumor-Associated Dendritic Cell-Mediated Colon Cancer Progression Through Non-Coding RNA MALAT-1. J Cell Physiol 2015;230:1883-94. [Crossref] [PubMed]
- Sugasawa H, Ichikura T, Tsujimoto H, et al. Prognostic significance of expression of CCL5/RANTES receptors in patients with gastric cancer. J Surg Oncol 2008;97:445-50. [Crossref] [PubMed]
- An G, Wu F, Huang S, et al. Effects of CCL5 on the biological behavior of breast cancer and the mechanisms of its interaction with tumorassociated macrophages. Oncol Rep 2019;42:2499-511. [PubMed]
- Soria G, Ben-Baruch A. The inflammatory chemokines CCL2 and CCL5 in breast cancer. Cancer Lett 2008;267:271-85. [Crossref] [PubMed]
- Aldinucci D, Colombatti A. The inflammatory chemokine CCL5 and cancer progression. Mediators Inflamm 2014;2014:292376.
- Chang LY, Lin YC, Mahalingam J, et al. Tumor-derived chemokine CCL5 enhances TGF-beta-mediated killing of CD8(+) T cells in colon cancer by T-regulatory cells. Cancer Res 2012;72:1092-102. [Crossref] [PubMed]
- Zhang Y, Lv D, Kim HJ, et al. A novel role of hematopoietic CCL5 in promoting triple-negative mammary tumor progression by regulating generation of myeloid-derived suppressor cells. Cell Res 2013;23:394-408. [Crossref] [PubMed]
- Cambien B, Richard-Fiardo P, Karimdjee BF, et al. CCL5 neutralization restricts cancer growth and potentiates the targeting of PDGFRbeta in colorectal carcinoma. PLoS One 2011;6:e28842. [Crossref] [PubMed]
- Chen K, Liu Q, Tsang LL, et al. Human MSCs promotes colorectal cancer epithelial-mesenchymal transition and progression via CCL5/beta-catenin/Slug pathway. Cell Death Dis 2017;8:e2819. [Crossref] [PubMed]
- Shirai K, Shimada T, Yoshida H, et al. Interferon (IFN)-induced protein 35 (IFI35) negatively regulates IFN-beta-phosphorylated STAT1-RIG-I-CXCL10/CCL5 axis in U373MG astrocytoma cells treated with polyinosinic-polycytidylic acid. Brain Res 2017;1658:60-7. [Crossref] [PubMed]
- Huang C, Li Z, Li N, et al. Interleukin 35 Expression Correlates With Microvessel Density in Pancreatic Ductal Adenocarcinoma, Recruits Monocytes, and Promotes Growth and Angiogenesis of Xenograft Tumors in Mice. Gastroenterology 2018;154:675-88. [Crossref] [PubMed]