Analysis of expression differences of immune genes in non-small cell lung cancer based on TCGA and ImmPort data sets and the application of a prognostic model
Introduction
As the most common type of tumor in the world (1), lung cancer has the highest mortality rate among all cancers, and accounts for 23% of the total number of tumor-related deaths, more than any other cancer (2). Around 75% to 80% of all lung cancer cases are non-small cell lung cancer (1,3).
With the development of modern molecular biology and tumor immunology, it has gradually been recognized that tumor occurrence and progression are not only related to the internal genetic background of cancer cells, but are also dependent on the interaction between the tumor and various systems within the body, especially the immune system (4,5).
Immune-related cells and factors are involved throughout the entire process of tumor occurrence, proliferation, and development, and have a significant influence on the tumor (6-8). Particularly with the development of monoclonal antibody drugs and adoptive immunotherapy, tumor immunity has become a hot spot in tumor research. To date, there has been no in-depth study into the relationship between the screening of immune-related molecules, their expression in lung cancer, and their effect on lung cancer prognosis (7). Therefore, it is necessary to explore the characteristics of immune-related molecules in lung cancer patients, and to evaluate the relationship between the components of the immune environment of lung cancer at the cell, molecule, and gene levels, to identify new targets that can predict the therapeutic effect and prognosis of lung cancer.
In recent years, with the development of gene expression profile chips and second-generation high-throughput sequencing technology, the quantity of lung cancer expression profile data has exploded, providing a basis for a comprehensive study of differentially expressed genes and their role in lung cancer prognosis (9,10). Based on big data from the TCGA and ImmPort databases, this study analyzed the differences in the expression of immune genes in NSCLC and clinical survival time, and constructed a prognostic model based on immune genes. The feasibility of the immune gene prognosis model in clinical application was verified.
Methods
Data download
The transcriptome data and clinical data of all NSCLC in TCGA-LUAD and TCGA-LUSC were downloaded through the Genomic Data Commons (GDC) Data Portal, and 594 clinical data were obtained. The immune gene data was downloaded through the ImmPort data portal, and 2,498 immune-related genes were obtained. Transcription factor data was downloaded from the Cistorm website.
Differential expression analysis
Differential gene expression analysis
All transcriptome data were analyzed for differences using the Wilcoxon test in R software. Genes with significant differences in expression between normal and tumor tissues were screened out. The screening criteria were |logFC| >1, FDR <0.05.
Analysis of immune gene differences
The differential genes were combined with the acquired immune gene data and analyzed in R software to screen out the differential immune genes from all the differential genes.
Construction and evaluation of immune gene prognosis model
The expression of immune genes was combined with survival time and survival status to carry out a survival analysis of the differential immune genes and clinical survival time, and to identify the immune genes that affect the prognosis of NSCLC. Based on these genes, an immune gene prognosis model was constructed. The ROC and risk score curves were drawn in R to evaluate the model.
Clinical correlation analysis
The prognosis-related immune genes were combined with clinical data, and the patient’s risk value was calculated based on the immune gene prognosis model. In addition, survival-related differential immune genes were also subjected to clinical correlation analysis.
Real-time polymerase chain reaction (PCR)
The original concentrations of specific transcripts were measured by real-time PCR using an ABI PRISM 7300 sequence detector (Applied Biosystems, Foster City, CA, USA). The primer sequences are provided in Table S1.
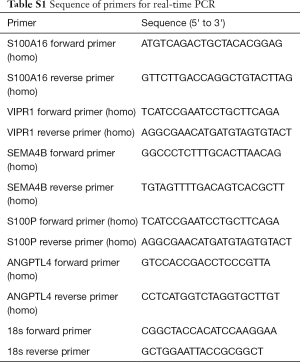
Full table
Ethics statement
All of the 29 patients (including lung cancer tissues and paired adjacent tissues) involved in this study underwent treatment at the Department of Thoracic Surgery, the First Affiliated Hospital of China Medical University (Shenyang, China). All clinical investigations were approved by the hospital’s Ethical Committee and all of the patients submitted signed consent forms.
Results
Differential gene expression analysis
After screening by Wilcoxon test, 6,778 differential genes met the conditions. All of the differential genes screened were intersected with immune genes, and 490 differential immune genes were obtained. The heat map showed distinct clustering of differentially immune genes in cancerous and adjacent tissues (Figure 1A) and the volcano plot suggested clustering of differentially immune genes of high or low expression level (Figure 1B).
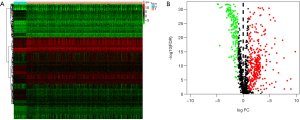
Prognostic related immune gene analysis
Survival analysis of differential immune genes and clinical survival time and survival status yielded 28 differential immune genes related to prognosis: S100P, S100A16, CRABP1, FURIN, FGF2, F2RL1, BIRC5, OAS1, PPARG, BTK, IGKV4-1, SEMA4B, SEMA7A, CX3CR1, CAT, GPI, IL11, INHA, INSL4, TNFSF12, TNFSF13, ADRB2, ANGPTL4, LGR4, TNFRSF11A, VIPR1, SHC3, and CBLC. Among these immune genes, 18 carry high risk, and the other 10 carry low risk (Figure 2). Survival analysis to identify prognosis-related immune genes found that 9 of the 28 immune genes were significantly related to patient survival: ANGPTL4, IL11, INHA, LGR4, S100A16, S100P, SEMA4B, SHC3, and VIPR1.
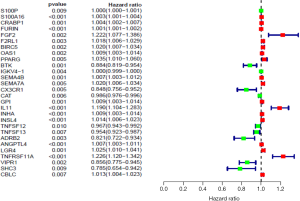
Immune gene prognostic model
The risk scores of prognosis-related immune genes were calculated with R software (Table 1) and combined with the patients’ clinical data, including survival time and survival status. A prognostic risk model based on the prognosis-related immune genes identified was constructed, and a survival curve was drawn. According to the calculated patient risk score, the patients were divided into a high-risk group and a low-risk group, which showed a significant difference in the survival prognosis of the two groups (P<0.05) (Figure 3A). The 5-year survival rate of the high-risk group was approximately 23.7% (95% CI: 16.25–34.7%) and the 5-year survival rate of the low-risk group was approximately 57.9% (95% CI: 48.7–68.9%). The AUC value was 0.723, which represented a higher true positive rate, thus proving the accuracy of the survival curve (Figure 3B).
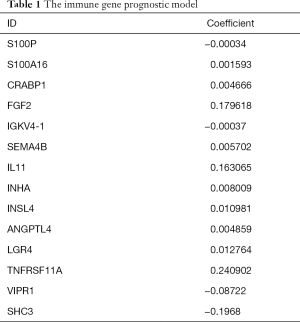
Full table
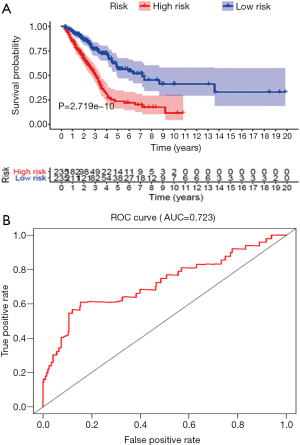
The abscissa from left to right indicates that the risk value increases gradually. After drawing the risk curve, the significant molecules were identified. The risk diagram, survival state diagram, and heat map based on the risk scores all showed that the higher the risk score, the worse the patient's prognosis (Figure 4).
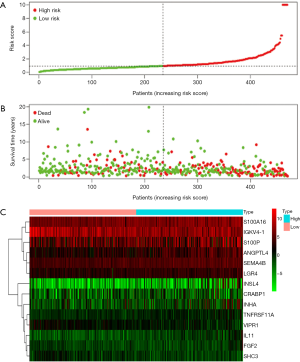
Clinical correlation analysis
Clinical correlation analysis of the survival-related differential immune genes revealed that in patients with lymph node metastasis, ANGPTL4 was positively correlated with T stage, S100a16 and SEMA4B were upregulated, and VIPR1 was downregulated. Further analysis revealed that VIPR1 was decreased in metastatic lung cancer compared to non-metastatic lung cancer (Figure 5).
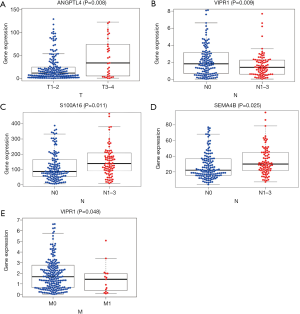
The survival analysis of the above genes revealed that the immune genes ANGPTL4, S100A16, SEMA4B, VIPR1, and S100P were significantly related to patient survival (Figure 6).
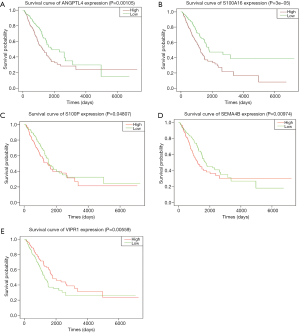
Validation of clinical samples
To verify the expression of the selected immune-related molecules in lung cancer and adjacent tissues, 29 cases of clinical samples were analyzed. The results showed that the level of S100A16 was elevated in lung cancer compared to the adjacent tissue, thus it could possibly act as an oncogene (Figure 7). In contrast, the expression of VIPR1 was found to be lower in lung cancer tissues than in the adjacent tissues, thus it could play a role as a tumor suppressor. S100A16 and VIPR1 exhibit potential to serve as clinical markers of lung cancer.
According to the pathology of 29 lung cancer patients, they were divided into two groups, well-differentiated lung cancer and poorly differentiated lung cancer, and analyzed the expressions of S100A16 and VIPR1. We found that the expression of these two molecules is closely related to the pathological differentiation of lung cancer, which holds potential for lung cancer prognosis. The results showed that S100A16 was elevated in poorly differentiated lung cancer than well-differentiated lung cancer (Figure 8). In contrast, the expression of VIPR1 was decreased in the poorly differentiated group compared to the well-differentiated group. Both S100A16 and VIPR1 exhibit potential to serve as clinical predictors of lung cancer.
Discussion
Over the past decade, it has been recognized that tumor occurrence and progression should not only be attributed to the internal genetic background of cancer cells, but also to the interaction the tumor has with various systems within the body, especially the immune system (11,12). Immune-related cells and factors are involved throughout the whole process of tumor occurrence, proliferation, and development, and significantly influence the tumor (13). Therefore, there is an increasing need to explore the characteristics of immune-related molecules and to evaluate the function of immune genes in lung cancer (14,15). By doing this, more immune mechanisms and early warning effects of immunotherapy can be revealed. In this study, our prognostic model and survival analysis screened out immune genes that are significantly related to the prognosis and survival of lung cancer patients. Our research could provide an effective reference for clinical treatment of lung cancer. Current research has confirmed that immune genes are related to various biological behaviors, such as tumor development, metastasis, and apoptosis (16-19). At the same time, these immune genes also can be regarded as a new target of immunotherapy.
In our study, we identified the important immune-related molecules in NSCLC. Through our analysis of biological information and the prognosis analysis of lung cancer patients, we preliminarily screened five immune genes with potential early warning significance. However, in the subsequent validation of clinical samples, we finally focused on two molecules, S100A16 and VIPR1. After a further literature search, we found these two molecules to have an important pathologic effect, despite having been rarely discussed in the study of NSCLC (20,21).
S100A16 is a member of the S100 family and a partner of the Annexin family of proteins. It has been reported to be significantly upregulated in many tumors, such as HCC and breast cancer (22,23). S100A16 expression has also been associated with poor prognosis in patients with breast cancer and with the promotion of the invasive activity of breast cancer cells via interaction with cytoskeleton dynamics. In addition, the upregulation of S100A16 has been found to promote epithelial-mesenchymal transition via the Notch1 pathway in breast cancer (22). Conversely, the downregulation of S100A16 expression in oral squamous cell carcinoma specimens has been associated with poor prognosis and differentiation grade (24). Furthermore, in oral squamous cell carcinoma, S100A16 has been demonstrated to contribute to a less aggressive tumor phenotype. These findings imply that S100A16 plays distinct roles in tumor development and progression, depending on the pathologic type of cancer (21,23). However, the role of S100A16 in NSCLC remains unclear. Our findings concluded that S100A16 is a promising candidate as a prognostic marker and molecular hub in NSCLC.
Vasoactive intestinal peptide (VIP), also known as PACAP, is an important neuropeptide that controls cell physiology and mainly functions via two receptor subtypes VAPC1 and VAPC2 (25,26). VIP is a small neuropeptide involved in the relaxation of smooth muscle, exocrine, endocrine, and hydration ion flux in intestinal epithelial cells (27,28). Research also showed that the reduction of VIP may be related to the occurrence of disease, and overexpression of VIP could inhibit inflammation, therefore reducing acute lung injury in mice (29). Other studies also found that the immune response and survival rate of VIP knockout mice increased (30). VIP or VPAC1 receptor antagonist could strengthen the ability of chemotherapy by killing breast cancer cells and improving anti-viral immunity (31). These findings all indicate that, because of its potential role in tumor development and progression, VIPR can be used in tumor diagnosis and as a treatment target.
The function of VIP/PACAP and its receptor (VPAC1 and VPAC2) in normal human tumors and their role in potential new treatments were summarized by Dr. Teddy Moody (32). Some studies have reported that VIPR1 inhibits the growth of several cancers, including prostate cancer, lymph blastoma, and medulloblastoma, which suggests that VIPR1 may significantly inhibit the growth and development of cancer cells (33,34). In the present study, overexpression of VIPR1 was found to reduce the proliferative capacity of H1299 cells (35). The experimental results are consistent with those of previous studies and indicate that VIPR1 plays an important role in the inhibition of LUAD cell growth. It may provide a novel target for drug intervention in patients with CAP expressing VIPR1. The results of our research are consistent with the results of current in-vivo experiments and further explain the important role of VIPR1 in lung cancer. Our findings lay a solid foundation for further study of the clinical transformation of lung cancer.
Conclusions
In conclusion, our results present two innovative molecules, S100A16 and VIPR1, which play an important role in NSCLC and hold potential as predictors of lung cancer prognosis. Our results also provide a new insight into the immune genes that mediate the malignant biological behavior of lung cancer.
Acknowledgments
Funding: This study was supported by Program Funded by Liaoning Province Education Administration (No. QN2019003), Jieping Wu Medical Foundation (No. 320.6750.19021), and 2018 China Medical University Young Backbone Support Program (No. QGZ2018011).
Footnote
Conflicts of Interest: All authors have completed the ICMJE uniform disclosure form (available at http://dx.doi.org/10.21037/atm.2020.04.38). The authors have no conflicts of interest to declare.
Ethical Statement: The authors are accountable for all aspects of the work in ensuring that questions related to the accuracy or integrity of any part of the work are appropriately investigated and resolved. All clinical investigations were approved by the hospital’s Ethical Committee and all of the patients submitted signed consent forms.
Open Access Statement: This is an Open Access article distributed in accordance with the Creative Commons Attribution-NonCommercial-NoDerivs 4.0 International License (CC BY-NC-ND 4.0), which permits the non-commercial replication and distribution of the article with the strict proviso that no changes or edits are made and the original work is properly cited (including links to both the formal publication through the relevant DOI and the license). See: https://creativecommons.org/licenses/by-nc-nd/4.0/.
References
- Torre LA, Siegel RL, Jemal A. Lung cancer statistics. Lung cancer and personalized medicine. 2016.
- Siegel RL, Miller KD, Jemal A. Cancer statistics, 2020. CA Cancer J Clin 2020;70:7-30. [Crossref] [PubMed]
- Tseng TS, Gross T, Celestin MD, et al. Knowledge and attitudes towards low dose computed tomography lung cancer screening and smoking among African Americans—a mixed method study. Transl Cancer Res 2019;8:S431-42. [Crossref]
- Weeden CE, Ah-Cann C, Asselin-Labat ML. Studying the immune landscape in lung cancer models: choosing the right experimental tools. Transl Lung Cancer Res 2018;7:S248-50. [Crossref] [PubMed]
- Ohyama K, Yoshimi H, Aibara N, et al. Immune complexome analysis reveals the specific and frequent presence of immune complex antigens in lung cancer patients: A pilot study. Int J Cancer 2017;140:370-80. [Crossref] [PubMed]
- Xu CH, Wei W, Yu CW, et al. Diagnosis value of serum soluble B7-H4 expression in non-small cell lung cancer. Clin Respir J 2018;12:134-9. [Crossref] [PubMed]
- Sato K, Akamatsu H, Murakami E, et al. Correlation between immune-related adverse events and efficacy in non-small cell lung cancer treated with nivolumab. Lung Cancer 2018;115:71-4. [Crossref] [PubMed]
- Bukh A, Aguado MT, Kimose HH, et al. Primary lung cancer. A controlled study of preoperative and postoperative levels of circulating immune complexes. Cancer 1988;61:2033-41. [Crossref] [PubMed]
- Bhattacharya S, Andorf S, Gomes L, et al. ImmPort: disseminating data to the public for the future of immunology. Immunol Res 2014;58:234-9. [Crossref] [PubMed]
- Zhu Y, Qiu P, Ji Y. TCGA-assembler: open-source software for retrieving and processing TCGA data. Nat Methods 2014;11:599-600. [Crossref] [PubMed]
- Ladbury CJ, Rusthoven CG, Camidge DR, et al. Impact of Radiation Dose to the Host Immune System on Tumor Control and Survival for Stage III Non-Small Cell Lung Cancer Treated with Definitive Radiation Therapy. Int J Radiat Oncol Biol Phys 2019;105:346-55. [Crossref] [PubMed]
- Mendes F, Antunes C, Abrantes AM, et al. Lung cancer: the immune system and radiation. Br J Biomed Sci 2015;72:78-84. [Crossref] [PubMed]
- Tian H, Chen Y, Zhao J, et al. Effects of siRNAs targeting CD97 immune epitopes on biological behavior in breast cancer cell line MDA-MB231. Zhejiang Da Xue Xue Bao Yi Xue Ban 2017;46:341-8. [PubMed]
- Giatromanolaki A, Koukourakis IM, Balaska K, et al. Programmed death-1 receptor (PD-1) and PD-ligand-1 (PD-L1) expression in non-small cell lung cancer and the immune-suppressive effect of anaerobic glycolysis. Med Oncol 2019;36:76. [Crossref] [PubMed]
- Zhang Y, Chen B, Wang L, et al. Systemic immune-inflammation index is a promising noninvasive marker to predict survival of lung cancer: A meta-analysis. Medicine (Baltimore) 2019;98:e13788. [Crossref] [PubMed]
- Bufalino C, Hepgul N, Aguglia E, et al. The role of immune genes in the association between depression and inflammation: a review of recent clinical studies. Brain Behav Immun 2013;31:31-47. [Crossref] [PubMed]
- Cortez MA, Anfossi S, Ramapriyan R, et al. Role of miRNAs in immune responses and immunotherapy in cancer. Genes Chromosomes Cancer 2019;58:244-53. [Crossref] [PubMed]
- Cohen EN, Gao H, Anfossi S, et al. Inflammation Mediated Metastasis: Immune Induced Epithelial-To-Mesenchymal Transition in Inflammatory Breast Cancer Cells. PLoS One 2015;10:e0132710. [Crossref] [PubMed]
- Yi L, Sun D, Han Q, et al. Interferon regulatory factor 3 mediates Poly(I:C)-induced innate immune response and apoptosis in non-small cell lung cancer. Int J Oncol 2018;52:1623-32. [PubMed]
- De Petris L, Orre LM, Kanter L, et al. Tumor expression of S100A6 correlates with survival of patients with stage I non-small-cell lung cancer. Lung Cancer 2009;63:410-7. [Crossref] [PubMed]
- Katono K, Sato Y, Kobayashi M, et al. S100A16, a promising candidate as a prognostic marker for platinum-based adjuvant chemotherapy in resected lung adenocarcinoma. Onco Targets Ther 2017;10:5273-9. [Crossref] [PubMed]
- Zhou W, Pan H, Xia T, et al. Up-regulation of S100A16 expression promotes epithelial-mesenchymal transition via Notch1 pathway in breast cancer. J Biomed Sci 2014;21:97. [Crossref] [PubMed]
- Tanaka M, Ichikawa-Tomikawa N, Shishito N, et al. Co-expression of S100A14 and S100A16 correlates with a poor prognosis in human breast cancer and promotes cancer cell invasion. BMC Cancer 2015;15:53. [Crossref] [PubMed]
- Sapkota D, Bruland O, Parajuli H, et al. S100A16 promotes differentiation and contributes to a less aggressive tumor phenotype in oral squamous cell carcinoma. BMC Cancer 2015;15:631. [Crossref] [PubMed]
- Walling LR, Butler JS. Structural Determinants for Antitoxin Identity and Insulation of Cross Talk between Homologous Toxin-Antitoxin Systems. J Bacteriol 2016;198:3287-95. [Crossref] [PubMed]
- Hannibal J, Georg B, Fahrenkrug J. PAC1- and VPAC2 receptors in light regulated behavior and physiology: Studies in single and double mutant mice. PLoS One 2017;12:e0188166. [Crossref] [PubMed]
- Szema AM, Forsyth E, Ying B, et al. NFATc3 and VIP in Idiopathic Pulmonary Fibrosis and Chronic Obstructive Pulmonary Disease. PLoS One 2017;12:e0170606. [Crossref] [PubMed]
- Duggan SN. Negotiating the complexities of exocrine and endocrine dysfunction in chronic pancreatitis. Proc Nutr Soc 2017;76:484-94. [Crossref] [PubMed]
- Loh DH, Kuljis DA, Azuma L, et al. Disrupted reproduction, estrous cycle, and circadian rhythms in female mice deficient in vasoactive intestinal peptide. J Biol Rhythms 2014;29:355-69. [Crossref] [PubMed]
- Alcolado NG, Conrad DJ, Poroca D, et al. Cystic fibrosis transmembrane conductance regulator dysfunction in VIP knockout mice. Am J Physiol Cell Physiol 2014;307:C195-207. [Crossref] [PubMed]
- Thakur ML, Zhang K, Berger A, et al. VPAC1 receptors for imaging breast cancer: a feasibility study. J Nucl Med 2013;54:1019-25. [Crossref] [PubMed]
- Moody TW, Nucheberenguer B, Jensen RT. VIP/PACAP, and their receptors and cancer. Curr Opin Endocrinol Diabetes 2016;23:38-47. [Crossref]
- Abad C, Jayaram B, Becquet L, et al. Erratum to: VPAC1 receptor (Vipr1)-deficient mice exhibit ameliorated experimental autoimmune encephalomyelitis, with specific deficits in the effector stage. J Neuroinflammation 2017;14:157. [Crossref] [PubMed]
- Abad C, Jayaram B, Becquet L, et al. VPAC1 receptor (Vipr1)-deficient mice exhibit ameliorated experimental autoimmune encephalomyelitis, with specific deficits in the effector stage. J Neuroinflammation 2016;13:169. [Crossref] [PubMed]
- Zhao L, Yu Z, Zhao B. Mechanism of VIPR1 gene regulating human lung adenocarcinoma H1299 cells. Med Oncol 2019;36:91. [Crossref] [PubMed]