Epigenetic landscape analysis of lncRNAs in acute myeloid leukemia with DNMT3A mutations
Introduction
DNA methylation, as one of the most important modification methods in epigenetics, plays a key role in the regulation of an organism’s life. The mutation frequency of DNA methyltransferase 3A (DNMT3A) has been found to be 22.1% in acute myeloid leukemia (AML) (1,2). It has been reported that AML cases with DNMT3A mutations are often accompanied by other genetic or epigenetic abnormalities, with nucleophosmin 1 (NPM1) mutations (nearly 69.6%) being the most frequent ones. Meanwhile, MLL abnormalities are likely exclusive to DNMT3A mutations. The NPM1 gene, which is also one of the most common mutation genes in AML, carries the normal karyotype, with an incidence of approximately 35. NPM1 mutation is considered to be a relatively favorable prognostic factor in young AML patients but indicates poor survival in older AML patients (3). Studies have shown that DNMT3A mutations could be a prognostic factor in NPM1-mutated AML with remission (4-6).
DNMT3A and DNA methyltransferase 3 B (DNMT3B) jointly control the modification of DNA. They are highly expressed in early mammalian embryos and are gradually down-regulated with cell differentiation, but their expression is low in mature adult tissues (7,8). Animal models have shown that when DNMT3A is knocked out during the embryonic stage, mice display growth retardation, dysplasia, and other phenomena, and die by 4 weeks of age (9). DNMT3A also plays an important role in maintaining the function of germline stem cells. Knock-out of DNMT3A in mouse germ stem cells can cause extensive hypomethylation in the mouse genome and lead to genetic imprinting disorder, spermatogenesis, development disorders, and embryo death in mice (10,11). DNMT3A is also a necessary protein for hematopoietic stem cells (HSCs) to maintain their normal proliferation and differentiation function (12,13). Studies have indicated that conditional knockout of DNMT3A in mouse HSCs impairs the self-renewal ability of HSCs and destroys their directional differentiation potential (14-16).
As a new mechanism of epigenetic litter control, ncRNA has become a topical issue in modern oncology research. Like many other solid tumors, the occurrence of hematological malignancies is a disorder of multiple intracellular molecules and multiple links. Studies have shown that long non-coding RNAs (lncRNAs) can control the development of tumors, and notch receptor 1 (NOTCH1) has been shown to regulate the expression of several lncRNAs in T-cell acute lymphoblastic leukemia (17). A total of 48 lncRNA patterns have been identified that can predict response to standardized therapy and overall survival in elderly patients with CN-AML (18). Hughes found that in AML patients with a CCAAT enhancer-binding Protein Alpha (CEBPA) mutation, the expression of lncRNA Urothelial Cancer-Associated 1 (UCA1) was specifically up-regulated, and cell proliferation could be maintained by inhibiting the expression of cell cycle regulator p27kip1 (19). The expression of lncRNA Nuclear Paraspeckle Assembly Transcript 1 (NEAT1) in acute promyelocytic leukemia (APL) samples was significantly down-regulated compared with a normal control group and was inhibited by the PML/RARa fusion gene (20). The expression profiles of lncRNAs are related to the recurrent mutation, clinical features, and prognosis of AML (21). Some of these lncRNAs may play a functional role in leukemia formation (22).
Adult leukemia with a DNMT3A mutation is mostly insensitive to chemotherapy and has a low remission rate, which is now known as a special subtype of acute leukemia. Thus, our study aimed to explore the specific lncRNAs expressed in DNMT3A-mutated leukemia based on a DNMT3A mutant mouse model, and analyze the clinical prognosis of its target genes.
Methods
The c-bio portal and metascape analysis
The cBioCancer Genomics Portal was used to analyze the genetic landscape of the DNMT3A mutation in The Cancer Genome Atlas (TCGA) AML samples. Detailed clinical information and genetic alternations of DNMT3A were provided by this cancer genomic dataset, including 225 tumor research papers. Functional analysis was performed using a web-based portal called Metascape. Clusters enriched terms into groups and were divided by functional annotation and the P value. Cytoscape software was used to visualize the network results.
Construction of Dnmt3aR878H/WTMx1Cre+ mice
Dnmt3a R878H conditional knock-in mice were generated through recombineering by targeting PL253 vectors, as previously reported (23). Polymerase chain reaction (PCR) analysis was performed with specific primers (Table 1) to detect the genotype of the offspring. Next, we activated the mutant Dnmt3a protein by intraperitoneal injection with pIpC 4 weeks after birth. The mice were bred based on animal care standards, and all operations were approved by the Committee on Animal Use for Research at the Shanghai Jiao Tong University School of Medicine (23). All experiments were performed following guidelines and regulations of the Shanghai Jiao Tong University School of Medicine, China.
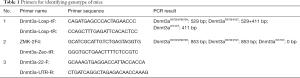
Full table
RNA-sequence
RNA was extracted from the bone marrow cells of Dnmt3aR878H/WTMx1Cre+ and Dnmt3aWT/WTMx1Cre+ mice using the standard protocol of TRIzol-isopropanol precipitation. The libraries were prepared according to the manufacturer’s instructions (Illumina’s TruSeq RNA Sample Preparation Kit, version 2) as follows:
- Step 1. Magnetic oligo-dT was utilized for pulling down the poly-A RNA from the bulk RNA of mice;
- Step 2. mRNA was segmented by metal-ion-catalyzed hydrolysis;
- Step 3. cDNA was synthesized for a-tailing and subsequent ligation of adapters.
The quality of samples was examined using a 2100 Bioanalyzer and then sequenced by Illumina MiSeq with a 200-bp paired-end. All the experiments were performed by colleagues at the Shanghai Institute of Hematology, Rui-Jin hospital of Shanghai Jiao Tong University School of Medicine.
Prediction of lncRNA
The Cuffcomparison method was used to compare Cufflinks splicing results without reference to annotations, and unknown annotations were obtained. To match the new transcripts and extract (i, u, x), 3 types of transcripts for prediction of lncRNA, we achieved the following parameters:
- Step 1. Transcription length ≥200 bp and exon ≥2;
- Step 2. Predicted ORF <300 bp;
- Step 3. Pfam, CPC, and CNCI prediction—the intersection of the prediction results. A CPC score <0 and CNCI score <0 were selected, with Pfam being significantly higher than that of other transcripts as potential lncRNA;
- Step 4. By comparing with unknown lncRNA, the same sequence of unknown lncRNA was removed.
i: A transfrag falling entirely within a reference intron; u: Unknown, intergenic transcript; x: Exonic overlap with reference on the opposite strand.
Prediction of lncRNA target genes
Trans-regulation and cis-regulation were used to predict target genes. We chose genes with a distance of less than 10 kb without lncRNA as the target gene for cis regulation. Trans-prediction uses a murine mRNA database. The blast was used to select the complementary analogous sequence, RNA plex (24) was used to calculate the complementary energy between the 2 sequences, and the sequences above the threshold were selected for further analysis.
OncoLnc and CCLE analysis
OncoLnc is a comprehensive interactive web server that contains the gene expression data of tumors and normal samples from the TCGA projects. The patients were divided into 2 groups according to the median expression of candidate genes. Next, we used Kaplan-Meier and log-rank methods to analyze the overall survival by the OncoLnc dataset (http://www.oncolnc.org/). The expression data of cell lines with DNMT3A mutation with/without NPM1 mutation was analyzed using the Broad Institute Cancer Cell Line Encyclopedia (CCLE) dataset.
Statistical analysis
The experiment was carried out in triplicate. The data for each sample was statistically analyzed using GraphPad Prism 8.0 (GraphPad Software Inc., San Diego, CA, USA). The P value was calculated by comparison of means (t-test).
Results
Genetic landscape of DNMT3A in acute myeloid leukemia
It is widely recognized that DNMT3A plays an important role in hematopoiesis and tumorigenesis. To explore the genetic landscape of DNMT3A in AML, we analyzed the DNMT3A alterations using cBioPortal for acute myeloid leukemia (OHSU, Nature 2018) and found that DNMT3A was altered in 114 samples from 531 patients with AML (21%). Among the DNMT3A alterations, the majority were missense mutations (putative driver, R882) and truncating mutations (putative driver) (Figure 1A). The genetic landscape also contained 1 unknown significant inframe mutation (Q573_A575del) and 15 missense mutations with unknown significance, including G543C, N879D, G543D, R309G, V657M, L3440, V657M, F751L, V716D, N879D, R659H, K826M, G570E, R326C, and F755S. The European Leukemia Net (ELN) risk classification (European leukemia net, 2017) and the mutation spectra of these AML patients were exhibited in Figure 1A. The mutation count of DNMT3A in patients for these 4 different mutation types was also displayed (Figure 1B). Then, the detailed cancer types with DNMT3A alternations were further analyzed. Among all these different types, the AML type with mutated NPM1 contained the most mutations, with a nearly 50% (47.65%) alteration frequency. The second and third types of AML were acute monoblastic/monocytic leukemia (26.67%) and AML NOS (26.58%), respectively. A certain percentage of DNMT3A alterations occurred in AML with RUNX1-RUNX1T1 (18.18%), therapy-related myeloid neoplasms (16.67%), AML with myelodysplasia-related changes (10.94%), AML with biallelic mutations of CEBPA (9.09%), AML with minimal differentiation (7.69%), AML with CBFB-MYH11 (7.14%), APL with PML-RARA (5.88%), and AML with recurrent genetic abnormalities (0%) (Figure 1C).
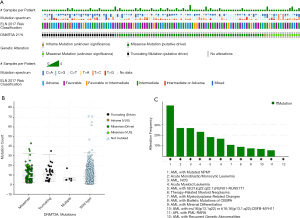
Construction of the Dnmt3a R878H mouse model
DNMT3A consists of 3 main domains: the PWWP domain, the ADD domain, and the C-terminal catalytic domain, with the vast majority of mutations occurring mainly in the C-terminal catalytic domain (Figure 2A). To further explore the functional mechanism of the putative driver missense mutation DNMT3A-R882H, we used a mouse model that was reported previously to express the mutant Dnmt3a R878H through Cre-mediated splicing endogenously. Mice with an IFN-inducible Mx1 promoter (Mx1Cre), which is specifically expressed in the hematopoietic system, were crossed with Dnmt3a mutant mice, and then we used pIpC to replace the endogenous Dnmt3a exon 23 and induce the expression of Dnmt3a R878H (Figure 2B). Finally, we used the Dnmt3aR878H/WTMx1-Cre+ mice and Dnmt3aWT/WTMx1-Cre+ mice for further functional analysis (Figure 2C).
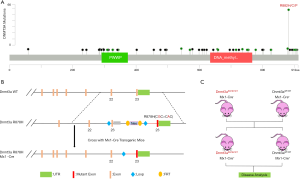
RNA-sequence of Dnmt3a R878H mice
To explore the function of the DNMT3A mutation in mice in detail, we performed RNA-seq of the BM cells of Dnmt3aR878H/WTMx1-Cre+ mice and Dnmt3aWT/WTMx1-Cre+ mice ON Miseq. TopHat’s spliced mapping algorithm was applied to map the genome of pre-processed reads. The covering distribution of the genome in each individual sample was obtained from a 1K window. The outermost circle in the graph in Figure 3 is the genome, and each circle in the graph represents the chromosome coverage of a sample (Figure 3A). Satisfaction analysis displayed that the number of genes detected increased with the increase in sequencing (Figure 3B). To compare the gene expression levels between different genes and different samples, reads of sequences were transformed into fragments per kilobase of the exon model per million mapped reads (FPKM) to standardize the gene expression levels. Next, we checked the relationship between samples using the correlation coefficient (Figure 3C) and principal component analysis (Figure 3D). Both these methods demonstrated that the similarity between the murine samples was high, with correlation coefficients being over 0.9, indicating the experimental reliability and rationality of the sample selection.
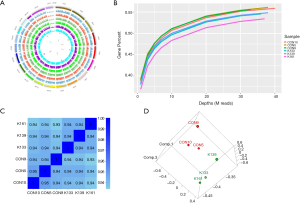
Prediction of new lncRNAs by RNA-Seq in mice
Numerous studies have shown that lncRNA plays an important role in tumorigenesis and development by regulating gene expression through chromatin modification and transcriptional regulation. In our study, Cuffcomparison was used to compare the splicing results of Cufflinks without reference to annotations, and new transcripts with unknown annotation matching were obtained. Three types of transcripts (i, u, x) were extracted to predict the lncRNAs. Then, we used the Perl script to find the corresponding gene on the chromosome of the new lncRNAs (Figure 4A). In addition, we compared the structure of the lncRNAs with that of the RNA. We observed no significant difference in transcription length (Figure 4B), exon number (Figure 4C), or expression level (Figure 4D) between the lncRNAs and RNAs.
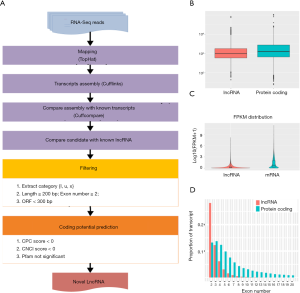
We further used Cuffdiff to analyze differential lncRNAs among samples and identified 23 differentially expressed lncRNAs in Dnmt3aR878H/WTMx1-Cre+ mice (Figure 5A and Table S1). The expression of these differentially expressed lncRNAs was shown using the expression value of the samples and the P values, as shown in Figure 5B and Table S1. The trans-regulation and cis-regulation systems were used to predict the target genes regulated by differential lncRNAs of the Dnmt3a mutation. In total, we found 11 candidate genes through the cis-regulation system and 113 target genes through the trans-regulation system (Figure 5C). Next, we examined differences in the expression levels of these candidate genes between Dnmt3aR878H/WTMx1-Cre+ mice and Dnmt3aWT/WTMx1-Cre+ mice. EdgeR was used to analyze the different genes among the samples and obtain the correction of the P value posterior multiple hypothesis tests. We then determined the P value threshold by controlling the false discovery rate (FDR). The scatter map of gene expression (Figure 5D) and the volcanic map of the differential genes (Figure 5E) showed the up-regulated genes (red plot) and down-regulated genes (green plot) in Dnmt3aR878H/WTMx1-Cre+ mice compared with Dnmt3aWT/WTMx1-Cre+ mice. Next, the prognostic values of these candidate genes were analyzed through the OncoLnc dataset, and 13 genes were found to be closely related to the prognosis of AML (Tables S2,S3). Taken together, the results above indicate that only 6 genes were both differentially expressed in Dnmt3aR878H/WTCre+ mice and associated with the prognosis of AML. Therefore, we chose only these 6 genes among the predicted candidate genes. The expressions of these differentially expressed candidate genes were further validated using the expression value of the murine samples (Figure 5F).
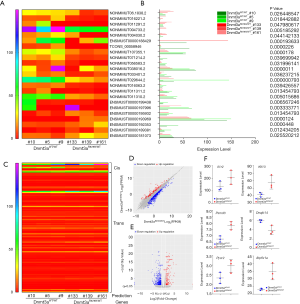
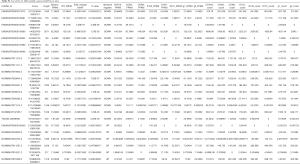
Full table

Full table
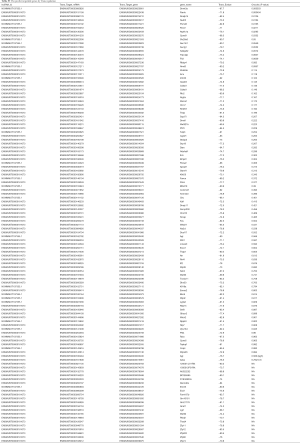
Full table
Prognosis and functional analysis of candidate genes in AML
We then explored the efficiency of these candidate genes in the survival of patients with AML. Oncolnc tools were used to analyze the survival of patients with AML. The Kaplan-Meier curve and log-rank test analyses revealed that increased IL1R2 (P=0.0022), KLF13 (P=0.0134), ATP6V1A (P=0.0295), PSMD3 (P=0.0165), and PYCR2 (P=0.0211) mRNA levels were significantly associated with poor prognosis in terms of overall survival (OS) of AML patients (Figure 6A). Decreased Dnaj heat shock protein family (Hsp40) member B14 (DNAJB14) mRNA levels also had a tendency to indicate a poor prognosis, with P=0.0126. Next, we chose two cell lines OCI-AML3 (harboring DNMT3A R882C mutation and NPM1 mutation) and OCI-AML2 (harboring DNMT3A R635W mutation) and attempted to explore the expression level of these dysregulated genes in human AML patients cell lines with NPM1 and/or DNMT3A mutations. The results based on CCLE showed that most of these dysregulated genes showed greater expression in OCI-AML3 cells compared with OCI-AML2 cells (Figure 6B). Functional analysis of these target genes was performed using Metascape tools, and the significant terms were identified and then hierarchically clustered into a tree based on κ-statistical similarities (Figure 6C,D, Table 2).
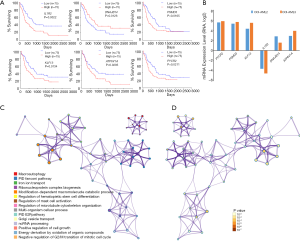
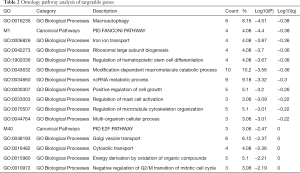
Full table
Discussion
DNMT3A gene mutations occur in a variety of hematopoietic diseases. The mutation rate in adult AML is more than 20%, and 10–15% in myelodysplastic syndrome (MDS). The loss of DNMT3A was clinically observed to be associated with a variety of hematological malignancies (25-27). However, the detailed mechanism of leukemogenesis associated with DNMT3A mutations was not clear.
Increasing evidence has shown that lncRNAs can promote or inhibit the growth of tumors by regulating or maintaining gene expression. Abnormal expression of lncRNA often leads to the occurrence, development, and metastasis of tumors (18). It has been reported that HOX antisense intergenic RNA (HOTAIR) exhibits a significant positive correlation with DNMT3A (28). The lncRNA H19 plays a crucial role in the initiation and progression of cancers, while the overexpression of H19 in AML patients has a strong positive association with DNMT3A mutations. Patients with a high expression level of H19 have shown a lower complete remission (CR) rate compared with those patients with low expression (29). Furthermore, the molecular dynamics analysis of lncRNA demonstrated that some lncRNAs could integrate with the promoter of the 5‘-UTR and recruit DNMT3A protein. The complex could then methylate the target genes, leading to epigenetic alternation (30). In this study, we focused on those lncRNAs related to hematological tumors to fully understand the mechanism of these lncRNAs in diseases; we hope our findings will lead to new ideas for the research, diagnosis, and prognosis of hematological tumors.
To further explore those lncRNAs regulated by DNMT3A mutations, we used the DNMT3A mutation conditional knock-in mice for correlation analysis. We analyzed the RNA-seq data using a special algorithm, detailed in section 2.4 of this article. Our data showed that considerable differences exist in gene expression and lncRNA expression between DNMT3A-mutant mice and wild mice. In addition, mutant mice showed significant heterogeneity compared with wild mice, which is consistent with previous reports (23). Twenty-three Dnmt3a mutation-specific lncRNAs were identified, 14 of which were novel lncRNAs and 9 of which were known lncRNAs in the database of murine lncRNAs. To explore the expression level of these lncRNAs in human AML, we checked the homologous lncRNAs by comparing the ID or whole-genome pairwise alignment (hg19/mm10, genome.UCSC.edu) of these lncRNAs in murine and human samples. Meanwhile, because of the rapid evolution and highly non-conservative characteristics of lncRNA, we were unable to match the murine lncRNAs with human lncRNAs, which prevented us from comprehensively evaluating the expression of these lncRNAs in databases in human AML patients (31).
According to this finding, we identified the differentially expressed lncRNAs in Dnmt3a mutant mice and predicted their downstream target genes based on cis- and trans-regulation. Indeed, more than 6 genes, including Il1r2, Klf13, Psmd3, Dnajb14, Pycr2, and Atp6v1a, were aberrantly regulated by the DNMT3A R878H mutation and suggested a poor prognosis. The functional analysis demonstrated that these genes are associated with macro-autophagy, iron ion transport, hematopoietic stem cell differentiation, and the cell cycle, which are, in turn, closely related to the occurrence and development of hematological malignancies.
Consistent with our result, the immune microenvironment-related gene, IL1R2, is known to be associated with a poor prognosis of AML and lung cancer in TCGA and Gene Expression Omnibus (GEO) datasets (32,33). In addition, a hormone-sensitive patient-derived xenograft (PDX) model showed that Krüppel-like factor 1 (KLF1) was related to the hormone sensitivity in acute lymphoblastic leukemia (34). ATP6V1A was a critical gene related to autophagy, which can induce autophagy through binding with a small-molecule compound EN6 and activating the mTOR signaling pathway (35). PSMD is considered an important cancer-related gene in the NF-kb pathway, which was shown to be consistently activated in Sezary Syndrome (36). Furthermore, some studies indicate that the single-nucleotide polymorphism (SNP) variations of PSMD3 in European individuals are related to the number of immune cells in peripheral blood and inflammatory diseases such as asthma (37). However, the roles of PYCR2 and DNAJB12 in hematopoietic malignancies have not been widely studied, although PYCR2 has been associated with proteomic subgrouping and reported to be involved in metabolic reprogramming of hepatocellular carcinoma with hepatitis B infection (38). As an ER J-protein, DNAJB14 accelerates the degradation of membrane proteins to maintain homeostasis (39). Our study is the first to report that the decreased expression of DNAJB14 is associated with the poor prognosis of AML.
Conclusions
In conclusion, we found the specific lncRNAs regulated by DNMT3A mutations using a DNMT3A R878H knock-in mouse model and predicted the candidate genes influenced by these lncRNAs. These findings might provide information relevant to the future development of novel therapeutics targeting these special lncRNAs and candidate genes for DNMT3A-mutated leukemic cells.
Acknowledgments
We would like to thank Editage for their English language editing services. We specially thank all of our colleagues at Shanghai Institute of Hematology for constructing the animal model and technical help.
Funding: This work was supported by the National Natural Science Foundation of China [Grant number 81570151], Shanghai Municipal Education Commission-Gaofeng Clinical Medicine [Grant number 20152507].
Footnote
Conflicts of Interest: The authors have no conflicts of interest to declare.
Ethical Statement: The authors are accountable for all aspects of the work in ensuring that questions related to the accuracy or integrity of any part of the work are appropriately investigated and resolved. All experiments were performed following guidelines and regulations of the Shanghai Jiao Tong University School of Medicine, China.
Open Access Statement: This is an Open Access article distributed in accordance with the Creative Commons Attribution-NonCommercial-NoDerivs 4.0 International License (CC BY-NC-ND 4.0), which permits the non-commercial replication and distribution of the article with the strict proviso that no changes or edits are made and the original work is properly cited (including links to both the formal publication through the relevant DOI and the license). See: https://creativecommons.org/licenses/by-nc-nd/4.0/.
References
- Yan XJ, Xu J, Gu ZH, et al. Exome sequencing identifies somatic mutations of DNA methyltransferase gene DNMT3A in acute monocytic leukemia. Nat Genet 2011;43:309-15. [Crossref] [PubMed]
- Linch DC, Hills RK, Burnett AK, et al. The clinical impact of mutant DNMT3A R882 variant allele frequency in acute myeloid leukaemia. Br J Haematol 2020. [Epub ahead of print]. [Crossref] [PubMed]
- Ostronoff F, Othus M, Lazenby M, et al. Prognostic significance of NPM1 mutations in the absence of FLT3-internal tandem duplication in older patients with acute myeloid leukemia: a SWOG and UK National Cancer Research Institute/Medical Research Council report. J Clin Oncol 2015;33:1157-64. [Crossref] [PubMed]
- Schnittger S, Haferlach C, Alpermann T, et al. DNMT3A is a Powerful Follow-up Marker in NPM1 mutated AML. Blood 2014.124.
- Bezerra MF, Lima AS, Pique-Borras MR, et al. Co-occurrence of DNMT3A, NPM1, FLT3 mutations identifies a subset of acute myeloid leukemia with adverse prognosis. Blood 2020. [Epub ahead of print]. [Crossref] [PubMed]
- Cappelli LV, Meggendorfer M, Dicker F, et al. DNMT3A mutations are over-represented in young adults with NPM1 mutated AML and prompt a distinct co-mutational pattern. Leukemia 2019;33:2741-6. [Crossref] [PubMed]
- Noh KM, Wang H, Kim HR, et al. Engineering of a Histone-Recognition Domain in Dnmt3a Alters the Epigenetic Landscape and Phenotypic Features of Mouse ESCs. Mol Cell 2018;69:533. [Crossref] [PubMed]
- Weinberg DN, Papillon-Cavanagh S, Chen H, et al. The histone mark H3K36me2 recruits DNMT3A and shapes the intergenic DNA methylation landscape. Nature 2019;573:281-6. [Crossref] [PubMed]
- Challen GA, Sun D, Jeong M, et al. Dnmt3a is essential for hematopoietic stem cell differentiation. Nat Genet 2011;44:23-31. [Crossref] [PubMed]
- Zhang X, Su J, Jeong M, et al. DNMT3A and TET2 compete and cooperate to repress lineage-specific transcription factors in hematopoietic stem cells. Nat Genet 2016;48:1014-23. [Crossref] [PubMed]
- Sanders MA, Chew E, Flensburg C, et al. MBD4 guards against methylation damage and germ line deficiency predisposes to clonal hematopoiesis and early-onset AML. Blood 2018;132:1526-34. [Crossref] [PubMed]
- Gu T, Lin X, Cullen SM, et al. DNMT3A and TET1 cooperate to regulate promoter epigenetic landscapes in mouse embryonic stem cells. Genome Biol 2018;19:88. [Crossref] [PubMed]
- Ketkar S, Verdoni AM, Smith AM, et al. Remethylation of Dnmt3a (-/-) hematopoietic cells is associated with partial correction of gene dysregulation and reduced myeloid skewing. Proc Natl Acad Sci U S A 2020;117:3123-34. [Crossref] [PubMed]
- Yang L, Rodriguez B, Mayle A, et al. DNMT3A Loss Drives Enhancer Hypomethylation in FLT3-ITD-Associated Leukemias. Cancer Cell 2016;30:363-5. [Crossref] [PubMed]
- Zhang X, Wang X, Wang XQD, et al. Dnmt3a loss and Idh2 neomorphic mutations mutually potentiate malignant hematopoiesis. Blood 2020. [Epub ahead of print]. [Crossref] [PubMed]
- Lu R, Wang J, Ren Z, et al. A Model System for Studying the DNMT3A Hotspot Mutation (DNMT3A(R882)) Demonstrates a Causal Relationship between Its Dominant-Negative Effect and Leukemogenesis. Cancer Res 2019;79:3583-94. [Crossref] [PubMed]
- Trimarchi T, Bilal E, Ntziachristos P, et al. Genome-wide mapping and characterization of Notch-regulated long noncoding RNAs in acute leukemia. Cell 2014;158:593-606. [Crossref] [PubMed]
- Garzon R, Volinia S, Papaioannou D, et al. Expression and prognostic impact of lncRNAs in acute myeloid leukemia. Proc Natl Acad Sci U S A 2014;111:18679-84. [Crossref] [PubMed]
- Hughes JM, Salvatori B, Giorgi FM, et al. CEBPA-regulated lncRNAs, new players in the study of acute myeloid leukemia. J Hematol Oncol 2014;7:69. [Crossref] [PubMed]
- Zeng C, Xu Y, Xu L, et al. Inhibition of long non-coding RNA NEAT1 impairs myeloid differentiation in acute promyelocytic leukemia cells. BMC Cancer 2014;14:693. [Crossref] [PubMed]
- Tsai CH, Yao CY, Tien FM, et al. Incorporation of long non-coding RNA expression profile in the 2017 ELN risk classification can improve prognostic prediction of acute myeloid leukemia patients. EBioMedicine 2019;40:240-50. [Crossref] [PubMed]
- Li Q, Dong C, Cui J, et al. Over-expressed lncRNA HOTAIRM1 promotes tumor growth and invasion through up-regulating HOXA1 and sequestering G9a/EZH2/Dnmts away from the HOXA1 gene in glioblastoma multiforme. J Exp Clin Cancer Res 2018;37:265. [Crossref] [PubMed]
- Dai YJ, Wang YY, Huang JY, et al. Conditional knockin of Dnmt3a R878H initiates acute myeloid leukemia with mTOR pathway involvement. Proc Natl Acad Sci U S A 2017;114:5237-42. [Crossref] [PubMed]
- Tafer H, Hofacker IL. RNAplex: a fast tool for RNARNA interaction search. Bioinformatics 2008;24:2657-63. [Crossref] [PubMed]
- Shlush LI, Zandi S, Mitchell A, et al. Identification of pre-leukaemic haematopoietic stem cells in acute leukaemia. Nature 2014;506:328-33. [Crossref] [PubMed]
- Bond J, Touzart A, Lepretre S, et al. DNMT3A mutation is associated with increased age and adverse outcome in adult T-cell acute lymphoblastic leukemia. Haematologica 2019;104:1617-25. [Crossref] [PubMed]
- Bartels S, Faisal M, Busche G, et al. Mutations associated with age-related clonal hematopoiesis in PMF patients with rapid progression to myelofibrosis. Leukemia 2019. [Epub ahead of print]. [Crossref] [PubMed]
- Zhang YY, Huang SH, Zhou HR, et al. Role of HOTAIR in the diagnosis and prognosis of acute leukemia. Oncol Rep 2016;36:3113-22. [Crossref] [PubMed]
- Zhang TJ, Zhou JD, Zhang W, et al. H19 overexpression promotes leukemogenesis and predicts unfavorable prognosis in acute myeloid leukemia. Clin Epigenetics 2018;10:47. [Crossref] [PubMed]
- Lister N, Shevchenko G, Walshe JL, et al. The molecular dynamics of long noncoding RNA control of transcription in PTEN and its pseudogene. Proc Natl Acad Sci U S A 2017;114:9942-7. [Crossref] [PubMed]
- Jiang YY, Zhu H, Zhang H. Nan Fang Yi Ke Da Xue Xue Bao 2018;38:731-5. [Analysis of orthologous lncRNAs in humans and mice and their species-specific epigenetic target genes]. [PubMed]
- Guo X, Zhang Y, Zheng L, et al. Global characterization of T cells in non-small-cell lung cancer by single-cell sequencing. Nat Med 2018;24:978-85. [Crossref] [PubMed]
- Yan H, Qu J, Cao W, et al. Identification of prognostic genes in the acute myeloid leukemia immune microenvironment based on TCGA data analysis. Cancer Immunol Immunother 2019;68:1971-8. [Crossref] [PubMed]
- Jing D, Bhadri VA, Beck D, et al. Opposing regulation of BIM and BCL2 controls glucocorticoid-induced apoptosis of pediatric acute lymphoblastic leukemia cells. Blood 2015;125:273-83. [Crossref] [PubMed]
- Chung CY, Shin HR, Berdan CA, et al. Covalent targeting of the vacuolar H(+)-ATPase activates autophagy via mTORC1 inhibition. Nat Chem Biol 2019;15:776-85. [Crossref] [PubMed]
- Caprini E, Cristofoletti C, Arcelli D, et al. Identification of key regions and genes important in the pathogenesis of sezary syndrome by combining genomic and expression microarrays. Cancer Res 2009;69:8438-46. [Crossref] [PubMed]
- Crosslin DR, McDavid A, Weston N, et al. Genetic variants associated with the white blood cell count in 13,923 subjects in the eMERGE Network. Hum Genet 2012;131:639-52. [Crossref] [PubMed]
- Gao Q, Zhu H, Dong L, et al. Integrated Proteogenomic Characterization of HBV-Related Hepatocellular Carcinoma. Cell 2019;179:561-77.e22. [Crossref] [PubMed]
- Goodwin EC, Motamedi N, Lipovsky A, et al. Expression of DNAJB12 or DNAJB14 causes coordinate invasion of the nucleus by membranes associated with a novel nuclear pore structure. PLoS One 2014;9:e94322. [Crossref] [PubMed]