Attitudes towards medical artificial intelligence talent cultivation: an online survey study
Introduction
Artificial intelligence (AI) holds great promise for the improvement of medicine. Medical AI has moved from theory toward application in real clinical practice in recent years (1,2). The advantages of medical AI include reduction of medical costs and improvement of diagnostic and therapeutic efficiency (3,4). AI can assist in the establishment of precise diagnoses and proper treatment strategy, presented as Watson (5) or Google’s DeepMind (5) providing advice on the best treatments for cancer and conducting genome analyses. Furthermore, AI has been used to predict genetic variations in low-grade gliomas (6), identify genetic phenotypes in small cell lung carcinoma (7), and automatically perform bone age assessment (8). These examples demonstrate the application of AI will be further extended to other areas in the future, leading to fundamental changes in the role of physicians and the way they practice medicine. Meanwhile, the development and utilization of AI programs in the medical field are currently entering the stage of commercialization calling for involvement of diverse academic backgrounds and occupations (9).
Talent cultivation, especially the specialized education in colleges and universities forms the footstone on the way towards the age of medical AI (10). Medical AI is rapidly emerging as a multidisciplinary branch of science, which involves foundational biomedical sciences, computer sciences, relevant laws and ethics, etc. The medical school curriculum as well as the graduate medical education within academic hospitals have not yet come to grips with educating students and trainees on this emerging technology around the world (11). Several opinions have pointed to the benefits and limitations associated with the use of AI in medicine (12,13), but the aspect related to formally educating the young generation has not been openly discussed. Some science and engineering institutes have set up independent AI college (14), however, fundamental medical courses and clinical application scenarios are still absent in the curriculum arrangements. A realistic and well-accepted scheme for talent cultivation on medical AI remains to be established.
Heated debates are maintained on educational reform concerning medical AI among educational, medical and relevant subject scholars. Recent reports have shown the interests, as well as concerns for career planning from medical students (15) and physicians (16) accompanied by development of AI. To date, no comprehensive survey was published to focus on the attitudes and formal suggestions on medical AI talent cultivation. Therefore, in this study, we conducted an on-line survey for both the medical and nonmedical public, to investigate their perception, willingness, understanding of the role for the issue. The respondents are also asked to provide detailed suggestions on course content and express their opinion on career planning. The information conveyed in the survey will be conductive to expand the talent pool effectively and accelerate the translational progress of AI in medicine.
Methods
Study design
The survey was designed to find out the intention and suggestion of medical AI teaching, as medical AI is considered as the promising application prospect while the talent cultivation and teaching plan remain uncertain. An electronic questionnaire was sent out using the WenJuanXing web-application (web link: www.wjx.cn) via social media. The questionnaire consisted of 7 items, including single-choice questions, multiple-choice questions, 3-point and 5-point Likert scale items, ranking question, and a narrative comment. Questions were designed to collect: (I) demographic information; (II) perception of medical AI; (III) willingness to participate in the medical AI related teaching activities; (IV) teaching content of medical AI; (V) the role of medical AI teaching; (VI) future career planning. According to the respondents’ profession (medical-related field or non-medical field), the questions were designed differently (http://cdn.amegroups.cn/static/application/f96fac204f567830f0f4c54990650073/atm.2019.12.149-1.pdf).
The respondents were informed that the information and results of questionnaire would be used to conduct statistical analysis and scientific publish. The anonymity of respondents was guaranteed. Once the respondents started to fill out the questionnaire, they had to answer all the questions, except the last narrative comment, to submit the survey.
Statistical analysis
Statistical analyses were performed by using distribution description, χ2 test and McNemar test. For the distribution description, we described demographic information of respondents, channels of knowing medical AI, the course type that medical AI should be set to, and the stage that medical AI should be involved in. When computing the proportion of multiple-choice question, the total number of respondents was divided by the number of each option. Besides, for the ranking questions, the ranking score was calculated by the total number of respondents multiplying the corresponding score (1st rank score: 3, 2nd rank score: 2, 3rd rank score: 1). For instance, if 80 respondents choose A as 1st option, the 1st rank score of A will be 80×3=240.
For the χ2 test, we reassign the respondents based on their education background, for those were “primary school and below”, “junior high school”, “high school”, “Specialized college”, or “Undergraduate/bachelor’s degree”, they were grouped to “Undergraduate or below”, and the rests were grouped to grouped to “Graduate or Phd”. Then we conducted three tests for each Likert scale to see if profession (“Medical-related”, “Non-medical”), gender (male, female), and education background (Undergraduate or below, Graduate or Phd) would have the significant difference.
For McNemar test, we tested the choice of future career if considering the influence of medical AI or not considering the influence for significant difference.
For all the tests, a P<0.05 was regarded as statistically significant. All analyses were performed using R 3.6.1.
Results
Over a 4-week period (Jul 13th, 2019–Aug 6th, 2019), a total of 714 students and professionals responded to the questionnaire. Of these, 4 respondents refused to upload their information and data to analyze, so the total number of analyzed respondents is 710. The proportion of respondents in the “Non-medical” group (42.25%) is approximate to the one in the “Medical-related” group (57.75%). As we targeted at students and professionals who were interested in medical AI, almost half of the respondents (49.58%) were at undergraduate level. Demographic characteristics of the Respondents was shown in Table 1, http://cdn.amegroups.cn/static/application/804b63ed0646e190ee5d2a438323fd48/atm.2019.12.149-2.pdf, Figure S1.
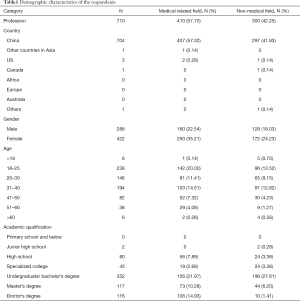
Full table
Perception of medical AI
To access the level of respondents’ perception of medical AI, respondents were asked to rate their agreement with 6 statements on 3-point Likert scale. When the perception level of medical AI increased, from “heard of the concept” to “been involved in the research”, the corresponding proportion of respondents in favor of “disagreement” became bigger, from 3.52% to 70.85% (http://cdn.amegroups.cn/static/application/804b63ed0646e190ee5d2a438323fd48/atm.2019.12.149-2.pdf). However, there was a significant difference between different education background when respondents rated their agreement (http://cdn.amegroups.cn/static/application/804b63ed0646e190ee5d2a438323fd48/atm.2019.12.149-2.pdf). As shown in Figure 1, the respondents in the “Undergraduate and below” group tended to disagree the statements while the rest tended to agree, as the level was increased. In the last statement, there was 35% of respondents in favor of “been involved in research on medical AI” in the “Graduate and Phd” group while only 11% agreement in the “Undergraduate and below” group. It is reasonable as most students will be involved in scientific research at their graduate or PhD stage. Moreover, when asked “I know medical AI through the following options”, there are 73.8% of respondents chose “Information from network and social platform” (http://cdn.amegroups.cn/static/application/804b63ed0646e190ee5d2a438323fd48/atm.2019.12.149-2.pdf). We might conclude that most of the respondents knew “medical AI” through internet and social platform thus lack of systematically study.
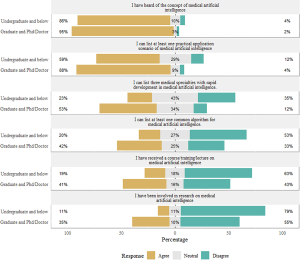
Willingness to participate in medical AI related activities
To access the level of respondents’ willingness to participate in medical AI related activities, the respondents were also asked to rate their agreement with 7 statements on 5-point Likert scale. As illustrated in Figure 2 and http://cdn.amegroups.cn/static/application/804b63ed0646e190ee5d2a438323fd48/atm.2019.12.149-2.pdf, as the level progressed, from “I am interested in medical AI” to “I believe that my current knowledge can support the study of medical AI”, more respondents tended to be in favor of disagree. Noticeably, before the fourth question “I am willing to undertake or assist in the education reform and related work on medical intelligence tutoring system”, the ratios of “agree” (combined the proportion of “strongly agree” and “somewhat agree”) were over 80%. When it came to the last question, “I believe that my current knowledge can support the study of medical AI courses”, the ratio of “agree” dropped to 56%. Besides, “Lack of teachers in the field of medical AI” was most selected in both “Medical-related” group (73.66%) and “Non-medical” group (62%) (http://cdn.amegroups.cn/static/application/804b63ed0646e190ee5d2a438323fd48/atm.2019.12.149-2.pdf). It seems that there was an urge for sophisticated lecturers regarding the medical AI.
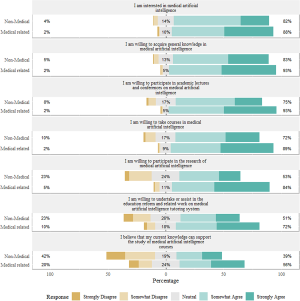
Teaching form/content
Since most respondents were in favor of statements, only a few statements showed the significance difference between “Medical-related” group and “Non-Medical” group (http://cdn.amegroups.cn/static/application/804b63ed0646e190ee5d2a438323fd48/atm.2019.12.149-2.pdf). As illustrated in Figure 3, the questions were designed to further explore the possible course type and the appropriate stage to introduce medical AI. Panel A showed that most of the respondents chose the “major elective course” as the proper course type. Panel B showed that “undergraduate” would be the appropriate stage to introduce the “medical AI”. Specifically, the undergraduate stage of medical-related group consisted of two parts: one is “after medical major courses” (with more votes), and the other is “before medical major courses”. On panel C, “AI application scenarios in the medical field” won more attention from both “Medical-related” group and “Non-medical” group. Despite respondents preferred to introduce the medical AI course sooner at the undergraduate stage as the major elective course, but most of respondents in the “Medical-related” group preferred that it should be arrange after medical major course. Furthermore, the practical content with application scenarios was most favored.
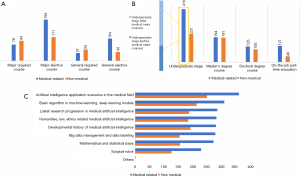
The role of medical AI teaching
More than half of the respondents disagreed that “Medical AI related content has been covered in other courses”, combining the proportion of “Strongly disagree” and “Somewhat disagree”. From Table 2, over 80% of respondents recognized the medical AI as “Conducive to promoting the development of medical AI diagnosis system” and “Effectively promote health care reform” (combining the proportion of “strong agree” and “Somewhat agree”).
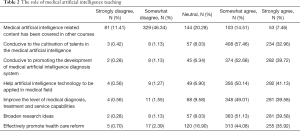
Full table
Career planning regrading medical AI
As shown in the Figure 4, http://cdn.amegroups.cn/static/application/804b63ed0646e190ee5d2a438323fd48/atm.2019.12.149-2.pdf, respondents showed tendency to work in the field of medical AI especially for those in the “Medical-related” group (61% for working full-time, 80% for engaging in some work related to the field). It is interesting to find out that although most respondents (58% in the “Non-medical” group, 78% in the “Medical-related” group) were in favor of the statement “AI can only play a supporting role in the future”, there were still certain respondents that remained the approval attitudes (34% in the “Non-medical” group, “33%” in the “Medical-related” group) when asked if they agreed on the statement “AI will replace human beings”. As illustrated in the Figure 5, “Surgeon” and “Ophthalmologist” remained the top 2 ranking place for ideal profession regardless of if the influence of medical AI was taken int account. Nevertheless, after taking the influence of medical AI into account, the score of conventionally considered as “most affected” profession increased such as “Clinical service guiding, care workers”, “Medical imaging doctor” and “Pharmacist”. Perhaps, those “most affected” profession might also have more medical AI application scenarios and those who would work in the profession, might have more chance to expose to the medical AI practice.
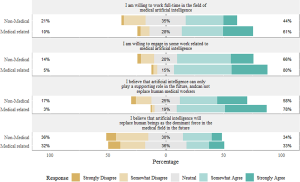
Narrative comment
As an optional question, the respondents were asked to provide additional comments on the implementation of medical AI. A total of 87 comments were received. Some respondents mentioned that medical AI is inevitable trend and more related scientific knowledge should be popularized. Besides, many commented that medical AI would bring an efficient and effective experience and reformation beyond imagination. A few respondents commented that the implementation of medical AI should cooperate with government for certain funding or companies for technical support. Several comments touched upon the importance of medical AI applications on telemedicine using augmented reality technology.
Discussion
AI, the core of the fourth revolution of science and technology, provides an opportunity to achieve personalized medicine and precision public health (17). Even though an increasing number of colleges students became aware of the development trend and involved in lectures, courses or research fields on AI in medicine, the curriculum system of medical AI subject has not yet been established, nor an independent subject been set up. In this study, an on-line survey was designed for both the medical related and non-medical field professions, involving diverse academic qualifications, job positions, and majors. The main part of the questionnaire contains 5 matrix scales with progressive investigations from respondents on their perception on the issue, the willingness to participate, the understanding of the role, their advice for the course curriculum and the relationship between their career planning and medical AI. Taken together, this survey aims to provide an integrated analysis on the attitudes towards medical AI talent cultivation.
An overwhelming majority of respondents acknowledged the potential roles of medical AI teaching as was demonstrated in Table 2, nevertheless, their perception level (Figure 1) and willingness to participate (Figure 2) medical AI events varies especially considering profession, education background and gender. More than half the respondents were shown equipped with basic knowledge of AI applicational scenarios and specialties in medicine, meanwhile were willing to participate in related general science activities (conference and lectures). The results emphasized on the feasibility and necessity for medical AI educational propagation. Further, although most respondent showed interest for medical AI in professional perspectives (courses, research or teaching), far less of them had the experience or trained with the specialized concepts/skills. Respondents from medical healthcare related fields, with high academic qualifications of male ones demonstrated better understanding (http://cdn.amegroups.cn/static/application/804b63ed0646e190ee5d2a438323fd48/atm.2019.12.149-2.pdf) and stronger willingness for medical AI area (http://cdn.amegroups.cn/static/application/804b63ed0646e190ee5d2a438323fd48/atm.2019.12.149-2.pdf). This trend calls for the establishment of academic curriculum and research platform in colleges and universities; medical college might be an ideal scene for pilot regarding the highly relevant theoretical backgrounds and applicational demand in the future.
There is no doubt that, noteworthy changes with era of AI are coming to the practice of medicine and healthcare system in general (18). Topics arose at various medical fields, especially where AI pioneered to achieve rapid development, that doctors and healthcare related staff might be substituted and lose their jobs in the foreseeable future (19,20). Despite previous reports confirmed the anxiety from medical students and physicians on the subjects including radiology and pathology (15,21), there has not been a systematic survey amongst public on the attitude alteration of career planning regarding development of medical AI. In this survey, respondents from medical related fields chose surgeon, ophthalmologist, physicians and researchers with high considerations in top tier, regardless of the boosting development of AI (Figure 5). Interestingly, the respondents tend more to choose clinical service guiding, radiology or clinical laboratory subjects. They possibly look to further increase of challenges and opportunities brought by tight combination with AI and multidisciplinary fusion.
The limitations of our research should also be considered. The participants are mainly Chinese, and the data from other countries are limited; therefore, the perceptions and demands of other countries require additional study to ensure accuracy. Moreover, the online survey is only accessible by computer and mobile phones with internet access, and thus some people fail to participate because of insufficient access. Further studies are warranted to investigate the factors that influence the public perceptions and the variation of demands with the medical AI education.
How to teach students to practice successfully in a health care environment transformed by AI applications should be a central focus of educational reform today. As the mainstream agreed on the trends, AI cannot substitute doctors as a whole, but doctors with no concept of AI will lose their stage in the near future (22). New skills and expertise will be required as we move to an age of AI, including better use of the findings of cognitive psychology, closer alignment of humans and machines in education, and enhanced use of simulations focused on the integration of machines in care delivery and on patients as active collaborators in their career.
Acknowledgments
Funding: This study was supported by the National Key Research and Development Program (2018YFC0116500), the Key Research Plan for the National Natural Science Foundation of China in Cultivation Project (91846109), the Science Foundation of China for Excellent Young Scientists (81822010), the National Natural Science Foundation of China (81770967, 81873675, 81800810), the Science and Technology Planning Projects of Guangdong Province (2019B030316012, 2018B010109008, 2017B030314025), Guangdong Science and Technology Innovation Leading Talents (2017TX04R031), the Natural Science Foundation of Guangdong Province (2018A030310104). The sponsor or funding organization had no role in the design or conduct of this research.
Footnote
Provenance and Peer Review: This article was commissioned by the Guest Editors (Haotian Lin and Limin Yu) for the series “Medical Artificial Intelligent Research” published in Annals of Translational Medicine. The article was sent for external peer review organized by the Guest Editors and the editorial office.
Conflicts of Interest: All authors have completed the ICMJE uniform disclosure form (available at http://dx.doi.org/10.21037/atm.2019.12.149). The series “Medical Artificial Intelligent Research” was commissioned by the editorial office without any funding or sponsorship. HL served as the unpaid Guest Editor of the series. The other authors have no other conflicts of interest to declare.
Ethical Statement: The authors are accountable for all aspects of the work in ensuring that questions related to the accuracy or integrity of any part of the work are appropriately investigated and resolved.
Open Access Statement: This is an Open Access article distributed in accordance with the Creative Commons Attribution-NonCommercial-NoDerivs 4.0 International License (CC BY-NC-ND 4.0), which permits the non-commercial replication and distribution of the article with the strict proviso that no changes or edits are made and the original work is properly cited (including links to both the formal publication through the relevant DOI and the license). See: https://creativecommons.org/licenses/by-nc-nd/4.0/.
References
- De Fauw J, Ledsam JR, Romera-Paredes B, et al. Clinically applicable deep learning for diagnosis and referral in retinal disease. Nat Med 2018;24:1342-50. [Crossref] [PubMed]
- Ravizza S, Huschto T, Adamov A, et al. Predicting the early risk of chronic kidney disease in patients with diabetes using real-world data. Nat Med 2019;25:57-9. [Crossref] [PubMed]
- Jiang F, Jiang Y, Zhi H, et al. Artificial intelligence in healthcare: past, present and future. Stroke Vasc Neurol 2017;2:230-43. [Crossref] [PubMed]
- Wu X, Huang Y, Liu Z, et al. Universal artificial intelligence platform for collaborative management of cataracts. Br J Ophthalmol 2019;103:1553-60. [Crossref] [PubMed]
- Trivedi H, Mesterhazy J, Laguna B, et al. Automatic Determination of the Need for Intravenous Contrast in Musculoskeletal MRI Examinations Using IBM Watson's Natural Language Processing Algorithm. J Digit Imaging 2018;31:245-51. [Crossref] [PubMed]
- Korfiatis P, Kline TL, Lachance DH, et al. Residual Deep Convolutional Neural Network Predicts MGMT Methylation Status. J Digit Imaging 2017;30:622-8. [Crossref] [PubMed]
- Rios Velazquez E, Parmar C, Liu Y, et al. Somatic Mutations Drive Distinct Imaging Phenotypes in Lung Cancer. Cancer Res 2017;77:3922-30. [Crossref] [PubMed]
- Lee H, Tajmir S, Lee J, et al. Fully Automated Deep Learning System for Bone Age Assessment. J Digit Imaging 2017;30:427-41. [Crossref] [PubMed]
- Nemati S, Holder A, Razmi F, et al. An Interpretable Machine Learning Model for Accurate Prediction of Sepsis in the ICU. Crit Care Med 2018;46:547-53. [Crossref] [PubMed]
- Kolachalama VB, Garg PS. Machine learning and medical education. NPJ Digit Med 2018;1:54. [Crossref] [PubMed]
- Wartman SA, Combs CD. Medical Education Must Move From the Information Age to the Age of Artificial Intelligence. Academic Medicine 2018;93:1107-9. [Crossref] [PubMed]
- Chen JH, Asch SM. Machine Learning and Prediction in Medicine - Beyond the Peak of Inflated Expectations. N Engl J Med 2017;376:2507-9. [Crossref] [PubMed]
- AI diagnostics need attention. Nature 2018;555:285. [Crossref]
- Boulet JR, Durning SJ. What we measure .. and what we should measure in medical education. Med Educ 2019;53:86-94. [Crossref] [PubMed]
- Pinto Dos Santos D, Giese D, Brodehl S, et al. Medical students' attitude towards artificial intelligence: a multicentre survey. Eur Radiol 2019;29:1640-6. [Crossref] [PubMed]
- Oh S, Kim JH, Choi SW, et al. Physician Confidence in Artificial Intelligence: An Online Mobile Survey. J Med Internet Res 2019;21:e12422. [Crossref] [PubMed]
- Buch VH, Ahmed I, Maruthappu M. Artificial intelligence in medicine: current trends and future possibilities. Br J Gen Pract 2018;68:143-4. [Crossref] [PubMed]
- Miller DD, Brown EW. Artificial Intelligence in Medical Practice: The Question to the Answer? Am J Med 2018;131:129-33. [Crossref] [PubMed]
- Chockley K, Emanuel E. The End of Radiology? Three Threats to the Future Practice of Radiology. J Am Coll Radiol 2016;13:1415-20. [Crossref] [PubMed]
- Tajmir SH, Alkasab TK. Toward Augmented Radiologists: Changes in Radiology Education in the Era of Machine Learning and Artificial Intelligence. Acad Radiol 2018;25:747-50. [Crossref] [PubMed]
- Jha S, Topol EJ. Adapting to Artificial Intelligence: Radiologists and Pathologists as Information Specialists. JAMA 2016;316:2353-4. [Crossref] [PubMed]
- Winkler-Schwartz A, Bissonnette V, Mirchi N, et al. Artificial Intelligence in Medical Education: Best Practices Using Machine Learning to Assess Surgical Expertise in Virtual Reality Simulation. J Surg Educ 2019;76:1681-90. [Crossref] [PubMed]