Differentiation combined hepatocellular and cholangiocarcinoma from intrahepatic cholangiocarcinoma based on radiomics machine learning
Introduction
Combined hepatocellular and cholangiocarcinoma (CHC) account for 0.4–14.2% of primary hepatic malignancies (1,2), originate from hepatic progenitor cells (3) and show both hepatocytic and cholangiocytic differentiation (4,5). In contrast, intrahepatic cholangiocarcinoma (ICC) is the second most common hepatic malignancy after hepatocellular carcinoma (6) and histologically derived from the biliary epithelial cell.
Although the 7th and 8th editions of the American Joint Committee on Cancer Staging and the International Union for Cancer Control tumor-node-metastasis staging group CHC and ICC into one classification, the distinctions between CHC and ICC clinical characteristics remain unclear. Some studies proved clinical features, and prognosis of CHC is markedly different from those of ICC, while others reported CHC and ICC show similar genetic (7) and clinical characteristics (8). Lee et al. reported that the patients with CHC had 18 months’ median overall survival which was shorter than patients with ICC (9). A recent study proved that the rate of recurrence-free survival at 5 years was significantly different between CHC and ICC, with 0% and 37.7%, respectively (10). Surgical resection is the sole therapy choice that offers a chance of long-term survival for patients with ICC, while patients with CHC experience more frequently recurrence than patients with ICC after curative resection (9). However, drug-targeted therapy may bring desirable results to patients with CHC. Futsukaichi et al. reported a patient with CHC successfully treated with sorafenib (11). Liver transplantation is also one of choice for patients with ICC or CHC (12). Nonetheless, ICC patient surgical results following liver transplantation are quite poor due to higher recurrence and reduced survival (13). Liver transplantation may improve surgical outcomes of patients with CHC than ICC patients (14). Therefore, it has significant clinical implications to differentiate CHC from ICC preoperatively accurately.
In clinical practice, using needle biopsy to differentiate CHC from ICC may increase the possibility of tumors metastasis, so its clinical application is limited. Additionally, radiographic imaging plays a vital role in the diagnosis and differential diagnosis of liver tumors. However, CHC can share some similar radiographic characteristics with ICC (15,16), so it is challenging to differentiate diagnosis preoperatively through those methods (17). For example, there have been some studies regarding CHC’s radiographic characteristics (18-25), but these studies’ results are contradictory. It poses a big challenging in clinical practice.
Radiomics is a method for imaging analysis based on data-mining and statistical analysis of high-throughput imaging features (26). In recent years, it successfully showed favorable abilities in clinical management (27). Xu et al. reported that integrating clinical-radiologic and radiomic features can predict microvascular invasion and outcome in patients with hepatocellular carcinoma (26). Ji et al. showed that the radiomics-based model could predict lymph node metastasis and survival outcomes in patients with biliary tract cancer (28). However, to our knowledge, there is still a lack of radiomics-based methods or tools for differentiation between CHC and ICC. Our research aimed to establish and validate a radiomics-based model for ICC preoperative differentiation of CHC.
Methods
Patients
The institutional review board approved this study in our hospital. All procedures were performed following the 1975 Helsinki declaration and its later amendments.
The medical records were reviewed from January 2014 to December 2018 to identify all patients who underwent resection for CHC and ICC in our hospital. The inclusion criteria were as follows: (I) postoperatively pathological confirmation for CHC and ICC; (II) CHC is a classical type according to WHO classification criteria; (III) cholangiocarcinoma-dominant in CHC; (IV) preoperative CT imaging, including arterial phase (AP) and portal vein phase (PVP), were performed in our radiology unit; (V) without anti-tumor therapy before CT examination; (VI), routine preoperative laboratory examinations, including total bilirubin (TB), serum alanine aminotransferase (ALT), aspartate aminotransferase (AST), γ-glutamyl transpeptidase (GGT), serum a-fetoprotein (AFP), carcinoembryonic antigen (CEA), cancer antigen 199 (CA-199), and hepatitis B surface antigen. The exclusion criteria were as follows: (I) biopsy confirmation for CHC and ICC without operation; (II) treated with other diseases before CT examination; (III) imaging with artifacts or incomplete; (IV), deficient of clinical data. Finally, a total of 189 patients (ICC =123; CHC =66) were enrolled during the study period. The flowchart of patients’ enrollment was shown in Figure 1.
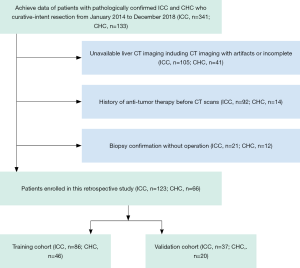
Imaging acquisition
All patients’ scans were performed using multi-slice CT (Philips Brilliance64, Philips Medical Systems, Best, The Netherlands; Siemens SOMATOM Definition Flash, Siemens Healthcare, Erlangen, Germany) in our institution. The scanning parameters were as follows: voltage, 120 kV; current, 200–250 mAs; slice thickness, 5 mm. After plain scanning was completed, a nonionic iodine contrast agent (Omnipaque 300, GE Healthcare, Ireland) was injected into the antecubital vein with a dose of 1.2 mL per kilogram of body weight at a rate of 3.0 mL/s, followed by a saline flush of 20 mL. AP and PVP scanning started at 25 and 60 s after the contrast medium was injected.
Imaging analysis
All CT images were reviewed in picture archiving and communication system (Syngo-Imaging, version VB36A; Siemens Medical Solutions, PACS) and were retrospectively interpreted by two radiologists (Yi Wei, six years of liver imaging experience, and Zixing Huang, fourteen years of liver imaging experience), both of whom were blinded to pathological outcomes and clinical factors. Before the study, they followed a complete day lecture-based and firsthand instruction session that explained in detail a structural liver imaging score (LI-score) system. During the course, 100 cases randomly selected from the institutional PACS database (not included in this study cohort) were reviewed individually, scored, and then reviewed as a group. The two radiologists independently reviewed all radiologic imaging individually first and then reviewed them together. Any discrepancies between two readers were discussed until a final consensus was generated. Individual scores were used for the calculation of inter-observer agreement, and consensus scores were used for classifying performance.
The LI-score, most referring to Xu et al. (26), were obtained depending on characteristics of tumor imaging: (I) lobes with lesions involvement (0, one lobe; 1, more than one lobes); (II) numbers of lesions (0, single; 1, multiple); (III) maximum diameter of the lesions (0, ≤5; 1, >5 cm); (IV) lesions margin (0, will-defined; 1, ill-defined); (V) hypodense halos (0, absent; 1, present); (VI) enhancement patterns (1, wash-in and wash-out; 2, rim-like enhancement with progressive centripetal filling; 3, weak or invisible enhancement with delay enhancement); (VII) peritumoral bile duct dilatation (0, absent; 1, resent); (VIII) intratumor necrosis (0, absent; 1, present); (IX) intratumor stones (0, absent; 1, present); (X) intratumor vascularity (0, absent; 1,present); (XI) peritumoral vascular invasion: defined as vessel stenosis, occlusion or unclear boundary with lesion (0, absent; 1, present); (XII) intravascular tumor thrombus: defined as intravascular low density (0, absent; 1, present); (XIII) capsular retraction (0, absent; 1, present); (XIV) hepatic lobe atrophy (0, absent; 1, present). The largest lesion was analyzed when there were multiple lesions.
Histopathologic analysis
Another researcher (Zhen Zhang, two years of liver imaging experience) who did not take part in imaging evaluation independently searched the pathological database. The histologic parameters ordinarily included tumor differentiation, satellite lesions, lymph node metastasis, adjacent organ invasion, and cirrhosis. The lowest differentiation was recorded when there were different differentiation components in the tumor.
Tumors segmentation
As lymphatic invasion and tumor-infiltration might spatial heterogeneously distributed, three regions were considered for tumor segmentation to involve more infiltration features and better reflect tumor heterogeneity (29). Here, the tumor, peritumoral area (a 2-mm wide band of outer tissue adjacent to the outer tumor margin), and the tumor plus peritumoral area were delineated, respectively. Each area was segmented from the original image (5 mm AP and 5 mm PVP) and preprocessed image. For obtaining tumor regions of interest (ROItumor), tumors were manually segmented in three layers for each lesion, along the tumor contour at the largest section, up and down from this section 5 mm distance respectively, by using an open-source imaging platform (ITK-SNAP, version 3.6.0; www.itksnap.org). The peritumoral regions of interest (ROIperitumoral) were automatically reconstructed according to the ROItumor contours with an erosion and dilation algorithm and the process was performed by AK software (Artificial Intelligent Kit, V3.2.0, GE Healthcare). Additionally, the tumor plus peritumoral regions of interest (ROItumor+peritumoral) was computed based on the ROItumor and ROIperitumoral. Radiomics features were extracted from each ROI, and the imaging preprocessing methods using AK software include the resampling with 1×1×1 pixels and noise reduction using classic Gaussian filter.
The ROIs segmentation was performed by two radiologists to assess the intra-observer reliability (Jun Zhang, reader 1, fifteen years of liver imaging experience; Likun Cao, reader 2, three years of liver imaging experience) in a blinded fashion; both did not participate in imaging analysis and were blinded to pathological outcomes. The reader 1 and reader 2 performed segmentation of lesions in one week, respectively, the readout sessions were held within one month. The reliability was calculated by using the intraclass correlation coefficient, and the values greater than 0.8 were selected for later investigation.
Radiomics signature
A total of 396 radiomic features for each CT imaging modality were calculated for each ROI (Table S1). The extracted radiomic features were standardized using the zero-mean normalization method, and the equation is
| [1] |
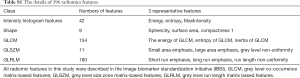
Full table
where χn is the value of feature N, ì is the average of all feature N, and σ is the standard deviation.
The potential combination of the radiomic features with ICC and CHC was first assessed in training cohort by using a Mann-Whitney U test; and the least absolute shrinkage and selection operator (LASSO) method, which is suitable for regression of high-dimensional data, was used to select the most useful predictive features. A logistic regression model was developed based on radiomic features selected, and a radiomic score (Radscore) was calculated for each patient via the Radiomics model (R model).
Development and validation of models
For clinical factors and liver imaging data, the univariable analysis with a generalized linear model (GLM) was performed to predictor selection, respectively, and the clinical score (C-score) and LI-score were computed using the same method mentioned above. The multivariable analysis, association with Radscore, C-score, and LI-score, was then conducted to select the significant predictors using a backward step-down choice process with the Akaike information criterion (AIC). The final prediction model was selected from the combined model, which had the highest predictive ability and clinical benefit.
The development of the nomogram was based on proportionally converting regression coefficients of each predictor in the final model to a 0- to a 100-point scale. The effect of the variable with the highest ā coefficient (absolute value) is assigned 100 points. The points are added across independent variables to derive total points, which are converted to predicted probabilities (Pi). The workflow of construction and validation of the models was shown in Figure 2.
Statistical analysis
Continuous variables were expressed as mean ± standard deviation or median ± Interquartile Range(IQR), compared by the t-test or Mann-Whitney U test as proper. Categorical variables were expressed as numbers and percentage, compared by the chi-square test or Fisher exact test. The inter-observer agreement was applied to assess the reliability of imaging analysis using the Kappa test; 0–0.2 represents slight, 0.21–0.40: fair, 0.41–0.60: moderate, 0.61–0.80: substantial, 0.81–1: excellent.
The discrimination performance of each prediction model was quantified by the receiver operating characteristic curve (ROC) and the area under the curve (AUC). The Delong’s test was used to compare the statistical differences between any two AUCs. The decision curve analysis (DCA) was applied to evaluate the clinical usefulness and the net benefits of the models. The DCA calculates the net benefits of a model as the difference between the true-positive and false-positive rates, weighted by the odds of the selected threshold probability of risk. In addition, for nomograms, the calibration plots were generated to evaluate the calibration of the nomogram via bootstrapping resamples using the Hosmer-Lemeshow goodness-of-fit test.
Statistical analysis was performed using the R software (version 3.5.1, R Project for Statistical Computing, http://www.r-project.org). Two-sided P<0.05 was considered statistically significant.
Results
Clinicopathologic characteristics
The 189 patients (ICC =123; CHC =66) were grouped into training cohort (n=132, ICC =86, CHC =46) and validation cohort (n=57, ICC =37, CHC =20) randomly. There was no significant difference in clinicopathologic characteristics between the training and validation cohorts (all P>0.05) (Table 1). Gender, age, AFP, cirrhosis, and hepatitis B were a significant difference between ICC and CHC based on univariable analysis (all P<0.05).
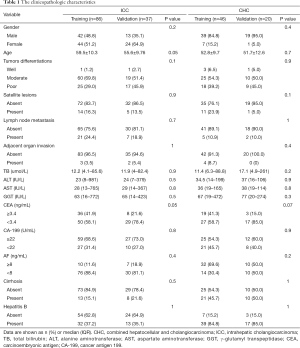
Full table
Imaging analysis
Kappa test showed substantial performance for enhancement patterns of tumors and excellent for remain imaging features. No significant imaging features can be found between the training cohort and validation cohort (all P>0.05) (Table 2). However, intratumor necrosis and intravascular tumor thrombus were a significant difference between ICC and CHC based on univariable analysis (all P<0.05).
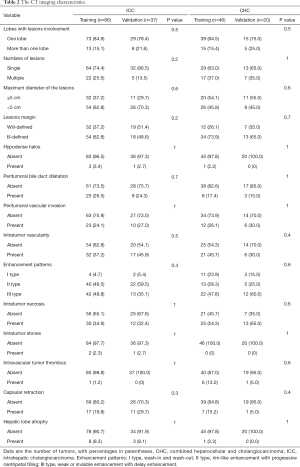
Full table
Development and validation of clinical model and liver imaging model
The clinical model (C model) contained gender [odds ratio (OR): 0.24; 95% confidence interval (95% CI): 0.07–0.8], AFP (OR: 0.06, 95% CI: 0.02–0.14), cirrhosis (OR: 0.44; 95% CI: 0.15–1.28) and hepatitis B (OR: 0.23; 95% CI: 0.07–0.72) in training cohort by multivariable analysis (all P<0.05); furtherly be verified in the validation cohort. The liver imaging model (L model) contained intratumor necrosis (OR: 0.5; 95% CI: 0.24–1.06) and intravascular tumor thrombus (OR: 0.09; 95% CI: 0.01–0.81) in training cohort by multivariable analysis (all P<0.05); also be verified in the validation cohort.
The AUC of the C model was 0.893 (95% CI: 0.831–0.954) and 0.858 (95% CI: 0.762–0.954), and the L model was 0.623 (95% CI: 0.532–0.715) and 0.671 (95% CI: 0.538–0.804) in training cohort and validation cohorts, respectively. The accuracy, sensitivity, and specificity of each model were shown in Table 3. The formulas for each model are shown in the Supplementary file.
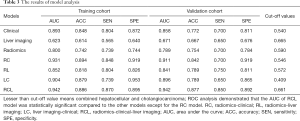
Full table
Radiomics analysis
The AUC of each R model is close to or higher than 0.7 both in the training cohort and validation cohort (Table S2), indicating the radiomics features exacted from 12 ROIs all had high discrimination ability and accuracy (ACC). However, the ROC analysis demonstrated that AUCs were not significantly different among 12 R models (P>0.05) (Figure S1A,B), suggesting imaging preprocessing did not exert influence on results of the radiomics analysis. The model constructed from ROItumor in AP with preprocessing was selected as the final R model due to the best performance. The formula for the R model is shown in the Supplementary file.
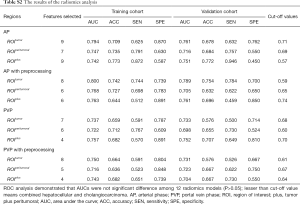
Full table
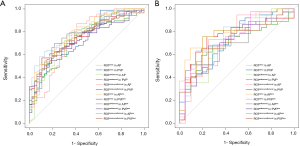
Performance of predictive models
The Radscore, C-score, and LI-score were identified as essential predictors by multivariate regression analysis (all P<0.05), and the single-factor models and combined models were established respectively. The AUC of the R model was slightly inferior to that of the C model, while the AUC of the L model was the lowest among single-factor models. The best performance of the model was the Radiomic-Clinical-Liver image model (RCL model) (Table 3), which reached the higher AUC [raining cohort, 0.942 (95% CI, 0.901–0.983); validation cohort, 0.942 (95% CI, 0.886–0.998)]. The Delong’s test illustrated that there was a significant difference between the RCL model and other single-factor models (all P<0.05), while no significant difference can be found between RCL and Radiomics-clinical models (RC model) (P=0.2) (Figure 3A,B).
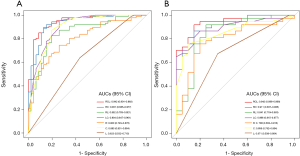
Moreover, the DCA was used to estimate the net benefit for each combined model, respectively. The results showed that adding the LI-scores did not improve the net benefit of the RCL model compared with the RC model (P=0.7) (Figure 4), while the AUC of the RCL model was the highest (Table 3). The final predictive model was named as the RCL model. The formula for RCL model was described as:
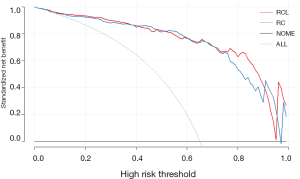
Y=3.71 + 0.951× Radscore −1.159 × Sex − 2.813× AFP − 1.97 × Hepatitis B − 1.523× intratumor necrosis.
The radiomics nomogram for visualization of the final predictive model is shown in Figure 5A. The calibration curve of the model showed excellent agreement between prediction and observation in both the training (C-index 0.935) and the validation cohort (C-index 0.931) (Figure 5B). The final predictive model was right in both data sets (P=0.2 and 0.9).
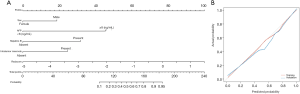
Discussion
In our study, we developed and confirmed a radiomics-based model for differentiation CHC from ICC. The proposed model proved excellent discrimination ability and reliability both in the training cohort and the validation cohort. It may play an essential role in clinical practice.
In the present study, we found that hepatitis B was an independent risk factor for CHC, inconsistent with the western study, which reported CHC is less related to hepatitis B (19). A reason for this discrepancy might be the different population characteristics between two studies, showing higher rates of hepatitis B virus infection in Chinese populations than Western counterparts. Moreover, we found that AFP was an independent predictor for CHC, which agrees with a study conducted by Tian et al. (30). However, CA-199 had no significant difference in our study. Our results were partly not consistent with previously published results, which found simultaneous elevation of both CA199 and AFP highly indicate CHC (31). The reason for this inconsistency may be low sensitivity for diagnosis CHC using the simultaneous elevation of both AFP and CA199 (32). Furthermore, we found that CHC occurs in males more frequent; our results are comparable with earlier studies (32,33).
Radiomics plays a vital role in oncology (27,34,35), so we tried to find CHC and ICC using radiomics features. Our results showed the radiomics features had a favorable ability for differentiating CHC from ICC in the training cohort and was verified in the validation cohort, suggesting the difference of intratumor heterogeneity between CHC and ICC. However, the AUC of the R model is slightly inferior to that of the C model, suggesting radiomics were weaker compare to evidence-based clinical factors in the differential diagnosis of CHC and ICC. Our results were like studies conducted by Banerjee et al. (36) and Renzulli et al. (37). We speculated the reason for this might be the same existence component, which is biliary epithelial cell carcinoma, both in CHC and ICC, so that the radiomics features may be overlapping to a certain extent. It also may be a reason for hardly using radiographic imaging to differentiate CHC from ICC.
Besides, we also found radiomics features from ROIperitumoral and ROItumor+peritumoral had favorable AUC and ACC in differentiation CHC from ICC, although no significant difference can be observed compared with radiomics features from ROItumor. The plausible reason may appear to tumor-infiltrating lymphocytes and tumor-associated macrophages around tumors (38); however, the density of lymphocytes and macrophages maybe not exactly similar between CHC and ICC, resulting in radiomics features difference.
We found that intratumor necrosis was an independent predictor for CHC, not consistent with the published papers (14-21), conclusions of which were also inconsistent. We suggest two explanations of why radiographic imaging of CHC varies among researches. First, it may be population characteristics included in studies ranging from region to region. Second, it may be inconsistence with equipment and evaluation methods used for research. Moreover, the DCA demonstrated the net benefit of the RCL model did not be improved than the RC model, which also showing radiographic imaging plays a negligible effect on diagnosis CHC from another perspective.
Interestingly, we proved that imaging preprocessing did not influence on results of radiomics analysis. In general, the imaging in AP is superior to that in PVP as radiomics features extraction for differentiation CHC from ICC, although there is no significant difference (P>0.05). We guessed some reasons for these phenomena. First, imaging preprocessing may play a weak effect on imaging with thicker layers. Second, the reason for the imaging in AP slightly better than PVP may be linked to the different arterial blood flow between CHC and ICC. Further investigation is needed.
Several limitations must be considered in interpreting our results. First, a large number of patients who did not undergo surgery were excluded due to the retrospective nature of the study so that potential selection bias may exist. Second, our study was drawn from a single-center, and expanding results to other centers will need to confirm its reproducibility. Third, we only extracted two-dimensional radiomics features with a 5 mm layer from different equipment due to retrospective analysis, but it may be generalized. Fourth, we tried to extend 2 mm outside from tumor as peritumoral area, but it needs more researches considering the actual size. Fifth, delayed scanning did not use tracer technology, so the collected data may be biased. Finally, our participant sample was modest in size due to the rarity of CHC.
Conclusions
In this study, we proposed a radiomics-based model to help preoperative differentiation of CHC from ICC. By fully considering and combining the specific radiomics and clinical manifestations, the model may help clinicians to be more confident in inpatient management.
Supplementary
The formulas for clinical model, liver imaging model, and radiomics model
The formula for clinical model was described as:
Y=3.81-1.413 × gender (0, male; 1, female) − 2.772 × AFP (0, <8; 1, ≥8) − 0.817 × cirrhosis (0, absent; 1, present) − 1.483 × hepatitis B (0, absent; 1, present).
The formula for liver imaging model was described as:
Y=1.052 − 0.695 × intratumor necrosis (0, absent; 1, present) − 2.384 × intravascular tumor thrombus (0, absent; 1, present).
The formula for radiomics model was described as:
Y = 2.335 + 0.651 × skewness − 0.169 × stdDeviation + 0.844 × ClusterProminence_angle0_offset1 − 0.249 × ClusterShade_angle0_offset3 − 0.244 × GLCMEntropy_AllDirection_offset1_SD + 7.273 × GLCMEntropy_AllDirection_offset3_SD + 0.748 × HaralickCorrelation_AllDirection_offset1_SD-0.61 × Inertia_angle90_offset1.
Acknowledgments
Funding: This research was supported by the Science and Technology Support Program of Sichuan Province, China (Grant Number 2017SZ0003) and the 1.3.5 Project for Disciplines of Excellence, West China Hospital, Sichuan University, China (Grant Number ZYJC18008).
Footnote
Conflicts of Interest: The authors have no conflicts of interest to declare.
Ethical Statement: The authors are accountable for all aspects of the work in ensuring that questions related to the accuracy or integrity of any part of the work are appropriately investigated and resolved. Institutional Review Board approval (ID: 2016-297) was obtained before this retrospective study.
References
- Jung DH, Hwang S, Hong SM, et al. Post-resection Prognosis of Combined Hepatocellular Carcinoma-Cholangiocarcinoma According to the 2010 WHO Classification. World J Surg 2017;41:1347-57. [Crossref] [PubMed]
- Vienot A, Neuzillet C. Cholangiocarcinoma: the quest for a second-line systemic treatment. Transl Cancer Res 2019;8:S275-88. [Crossref]
- Ogasawara S, Akiba J, Nakayama M, et al. Epithelial cell adhesion molecule-positive human hepatic neoplastic cells: development of combined hepatocellular-cholangiocarcinoma in mice. J Gastroenterol Hepatol 2015;30:413-20. [Crossref] [PubMed]
- Hang H, Jeong S, Sha M, et al. Cholangiocarcinoma: anatomical location-dependent clinical, prognostic, and genetic disparities. Ann Transl Med 2019;7:744. [Crossref] [PubMed]
- Connell LC, Harding JJ, Shia J, et al. Combined intrahepatic cholangiocarcinoma and hepatocellular carcinoma. Chin Clin Oncol 2016;5:66-74. [Crossref] [PubMed]
- Petrick JL, Braunlin M, Laversanne M, et al. International trends in liver cancer incidence, overall and by histologic subtype, 1978-2007. Int J Cancer 2016;139:1534-45. [Crossref] [PubMed]
- Cazals-Hatem D, Rebouissou S, Bioulac-Sage P, et al. Clinical and molecular analysis of combined hepatocellular-cholangiocarcinomas. J Hepatol 2004;41:292-8. [Crossref] [PubMed]
- Jarnagin WR, Weber S, Tickoo SK, et al. Combined hepatocellular and cholangiocarcinoma: demographic, clinical, and prognostic factors. Cancer 2002;94:2040-6. [Crossref] [PubMed]
- Lee JH, Chung GE, Yu SJ, et al. Long-term Prognosis of Combined Hepatocellular and Cholangiocarcinoma After Curative Resection Comparison With Hepatocellular Carcinoma and Cholangiocarcinoma. J Clin Gastroenterol 2011;45:69-75. [PubMed]
- Song P, Midorikawa Y, Nakayama H, et al. Patients' prognosis of intrahepatic cholangiocarcinoma and combined hepatocellular-cholangiocarcinoma after resection. Cancer Med 2019;8:5862-71. [Crossref] [PubMed]
- Futsukaichi Y, Tajiri K, Kobayashi S, et al. Combined hepatocellular-cholangiocarcinoma successfully treated with sorafenib: case report and review of the literature. Clin J Gastroenterol 2019;12:128-34. [Crossref] [PubMed]
- Sapisochin G, Facciuto M, Rubbia-Brandt L, et al. Liver transplantation for "very early" intrahepatic cholangiocarcinoma: International retrospective study supporting a prospective assessment. Hepatology 2016;64:1178-88. [Crossref] [PubMed]
- Lee DD, Croome KP, Musto KR, et al. Liver transplantation for intrahepatic cholangiocarcinoma. Liver Transpl 2018;24:634-44. [Crossref] [PubMed]
- Machairas N, Stamopoulos P, Kostakis ID, et al. Mixed Hepatocellular Cholangiocarcinoma: A Review of Long-Term Outcomes Following Liver Transplantation. Transplant Proc 2019;51:437-9. [Crossref] [PubMed]
- Saboo SS, Krajewski KM, Jagannathan JP, et al. Rapid progression of combined hepatocellular carcinoma and cholangiocarcinoma. Cancer Imaging 2011;11:37-41. [Crossref] [PubMed]
- Sagrini E, Iavarone M, Stefanini F, et al. Imaging of combined hepatocellular-cholangiocarcinoma in cirrhosis and risk of false diagnosis of hepatocellular carcinoma. United European Gastroenterol J 2019;7:69-77. [Crossref] [PubMed]
- Gera S, Ettel M, Acosta-Gonzalez G, et al. Clinical features, histology, and histogenesis of combined hepatocellular-cholangiocarcinoma. World J Hepatol 2017;9:300-9. [Crossref] [PubMed]
- Wang Y, Yang Q, Li S, et al. Imaging features of combined hepatocellular and cholangiocarcinoma compared with those of hepatocellular carcinoma and intrahepatic cholangiocellular carcinoma in a Chinese population. Clin Radiol 2019;74:407.e1-e10. [Crossref] [PubMed]
- Fowler KJ, Sheybani A, Parker RA 3rd, et al. Combined hepatocellular and cholangiocarcinoma (biphenotypic) tumors: imaging features and diagnostic accuracy of contrast-enhanced CT and MRI. AJR Am J Roentgenol 2013;201:332-9. [Crossref] [PubMed]
- Ye J, Xie X, Lin Y, et al. Imaging features of combined hepatocellular-cholangiocarcinoma on contrast-enhanced ultrasound: correlation with clinicopathological findings. Clin Radiol 2018;73:237-43. [Crossref] [PubMed]
- Shetty AS, Fowler KJ, Brunt EM, et al. Combined hepatocellular-cholangiocarcinoma: what the radiologist needs to know about biphenotypic liver carcinoma. Abdom Imaging 2014;39:310-22. [Crossref] [PubMed]
- Li F, Han J, Han F, et al. Combined Hepatocellular Cholangiocarcinoma (Biphenotypic) Tumors: Potential Role of Contrast-Enhanced Ultrasound in Diagnosis. AJR Am J Roentgenol 2017;209:767-74. [Crossref] [PubMed]
- Potretzke TA, Tan BR, Doyle MB, et al. Imaging Features of Biphenotypic Primary Liver Carcinoma (Hepatocholangiocarcinoma) and the Potential to Mimic Hepatocellular Carcinoma: LI-RADS Analysis of CT and MRI Features in 61 Cases. AJR Am J Roentgenol 2016;207:25-31. [Crossref] [PubMed]
- Wells ML, Venkatesh SK, Chandan VS, et al. Biphenotypic hepatic tumors: imaging findings and review of literature. Abdom Imaging 2015;40:2293-305. [Crossref] [PubMed]
- Sammon J, Fischer S, Menezes R, et al. MRI features of combined hepatocellular-cholangiocarcinoma versus mass forming intrahepatic cholangiocarcinoma. Cancer Imaging 2018;18:8-16. [Crossref] [PubMed]
- Xu X, Zhang HL, Liu QP, et al. Radiomic analysis of contrast-enhanced CT predicts microvascular invasion and outcome in hepatocellular carcinoma. J Hepatol 2019;70:1133-44. [Crossref] [PubMed]
- Huang Y, Liu Z, He L, et al. Radiomics signature: A Potential Biomarker for the Prediction of Disease-Free Survival in Early-Stage (I or II) Non-Small Cell Lung Cancer. Radiology 2016;281:947-57. [Crossref] [PubMed]
- Ji GW, Zhang YD, Zhang H, et al. Biliary Tract Cancer at CT: A Radiomics-based Model to Predict Lymph Node Metastasis and Survival Outcomes. Radiology 2019;290:90-8. [Crossref] [PubMed]
- Aishima S, Nishihara Y, Iguchi T, et al. Lymphatic spread is related to VEGF-C expression and D2-40-positive myofibroblasts in intrahepatic cholangiocarcinoma. Mod Pathol 2008;21:256-64. [Crossref] [PubMed]
- Tian MX, He WJ, Liu WR, et al. A Novel Risk prediction Model for Patients with Combined Hepatocellular-Cholangiocarcinoma. J Cancer 2018;9:1025-32. [Crossref] [PubMed]
- Tang D, Nagano H, Nakamura M, et al. Clinical and pathological features of Allen's type C classification of resected combined hepatocellular and cholangiocarcinoma: a comparative study with hepatocellular carcinoma and cholangiocellular carcinoma. J Gastrointest Surg 2006;10:987-98. [Crossref] [PubMed]
- Li R, Yang D, Tang CL, et al. Combined hepatocellular carcinoma and cholangiocarcinoma (biphenotypic) tumors: clinical characteristics, imaging features of contrast-enhanced ultrasound and computed tomography. BMC Cancer 2016;16:158-68. [Crossref] [PubMed]
- Kim SH, Park YN, Lim JH, et al. Characteristics of combined hepatocelluar-cholangiocarcinoma and comparison with intrahepatic cholangiocarcinoma. Eur J Surg Oncol 2014;40:976-81. [Crossref] [PubMed]
- Kickingereder P, Gotz M, Muschelli J, et al. Large-scale Radiomic Profiling of Recurrent Glioblastoma Identifies an Imaging Predictor for Stratifying Anti-Angiogenic Treatment Response. Clin Cancer Res 2016;22:5765-71. [Crossref] [PubMed]
- Aerts HJ, Velazquez ER, Leijenaar RT, et al. Decoding tumour phenotype by noninvasive imaging using a quantitative radiomics approach. Nat Commun 2014;5:4006-14. [Crossref] [PubMed]
- Banerjee S, Wang DS, Kim HJ, et al. A computed tomography radiogenomic biomarker predicts microvascular invasion and clinical outcomes in hepatocellular carcinoma. Hepatology 2015;62:792-800. [Crossref] [PubMed]
- Renzulli M, Brocchi S, Cucchetti A, et al. Can current preoperative imaging be used to detect microvascular invasion of hepatocellular carcinoma? Radiology 2016;279:432-42. [Crossref] [PubMed]
- Beig N, Khorrami M, Alilou M, et al. Perinodular and Intranodular Radiomic Features on Lung CT Images Distinguish Adenocarcinomas from Granulomas. Radiology 2019;290:783-92. [Crossref] [PubMed]