This article has an erratum available at: http://dx.doi.org/10.21037/atm-2022-17 the article has been update on 2022-06-10 at here.
Development and validation of an individualized gene expression-based signature to predict overall survival in metastatic colorectal cancer
Introduction
Metastatic colorectal cancer (mCRC) ranks the third most common cause of death from cancer worldwide (1), and the incidence of mCRC is growing, especially among younger patients (2). mCRC is a highly heterogeneous disease, which can show a wide range of clinical behavior, from curable oligometastatic disease to rapidly developing lethal disease (3).
Biomarkers which can reliably evaluate disease progression and survival outcome would have great value in guiding the treatment of mCRC. For instance, only a small proportion of mCRC patients are responsive to EGF receptor (EGFR)-targeted or VEGF receptor (VEGFR)-targeted therapies (4-6), while no appropriate targeted therapies have been shown efficacy to the remaining patients. Thus, identification of new biomarkers to optimize patient selection at highest risk for death and to provide a novel insight into target therapy is warranted.
Current prognostic models use histoclinical parameters for prognostication of patients have limitation in capturing molecular heterogeneity of this disease. Previous studies have provided some prognostic mRNAs for mCRC patients (7-10). However, none has been applicated into clinical use due to issues such as lack of consideration of other gene expressions and adequate validation. The availability of public, large-scale gene expression data profiling provides the opportunity to define reliable mCRC markers. Multiple gene expression data sets were combined to develop and validate an individualized gene-expressed signature for the survival of mCRC. The aim of this study is to identify the potential prognostic gene expression-based biomarkers of metastatic tumors.
Methods
Patients
Gene expression profiles of frozen colorectal cancer tumor tissue samples were from 4 public cohorts, including 73 stage IV CRC patients from TCGA CRC (11) as discovery cohort and 3 microarray data sets (GSE39582, GSE39084, and GSE17536) (12-14) obtained from Gene Expression Omnibus (GEO) database that were merged into a meta-validation cohort. We retrospectively analyzed these profiles. TCGA CRC cohort was downloaded from Broad GDAC Firehose (http://gdac.broadinstitute.org/) and transcripts per million (TPM) of level 3 RNA-Seq data in log2 scale were used. Other data sets were obtained directly in its processed format from GEO database through Bioconductor package ‘GEOquery’. Overall, 192 mCRC patients with valid survival information were included in this study. The batch effects were corrected using ‘combat’ algorithm implemented in R package ‘sva’ and z-scores for each gene were used for the following analyses. Data were collected from Dec 03 to Feb 04, 2018. We carefully reviewed both paper charts and electronic medical records when necessary. The present study obtained ethics approval from Sun Yat-sen University, Sixth Affiliated Hospital.
Construction and validation of mCRC prognostic gene signature
In order to construct a mCRC Prognostic Gene Signature (PGS), we first identified a list of candidate genes with relatively large variation [(median absolute deviation (MAD) >0.5]. Furthermore, to increase the robustness of the identification for the limited sample size, prognostic signature genes were further selected using the log-rank test with 100 randomizations (80% portion of samples each time) to assess the correlation between each candidate gene and patients’ overall survival (OS) in the discovery cohort. The genes showed significance repeatedly were selected as the candidates of the mCRC prognostic signature. For minimize over-fitting risk, we applied a Cox proportional hazards regression model on all advanced stage samples (stage III/IV) in combination of the least absolute shrinkage and selection operator (LASSO) (glmnet, version 2.0-16). The penalty parameter was calculated by 10-fold cross-validation in the training data set at the minimum partial likelihood deviance.
In order to stratify patients into low-risk or high-risk subgroups, the optimal cutoff value was determined by a time-dependent receiver operating characteristic (ROC) curve (survival ROC, version 1.0.3) at 3 years in the training dataset. The ROC curve was estimated by the Kaplan-Meier estimation method. We used the shortest distance between point representing the 100% true positive rate and 0% false-positive rate and the ROC curve as the cutoff value.
The prognostic value of the PGS was assessed in mCRC patients in the training and independent validation cohorts in univariable analyses respectively. Then we compared the risk scores derived from the gene signature with available clinicopathologic parameters in multivariable analyses.
Functional annotation and analysis
To investigate the biological characteristic of the gene signature, we conducted enrichment analysis for interested biological pathways, Bioconductor package ‘HTSanalyzeR’ was used to perform by Gene Set Enrichment Analysis (GSEA) (15).
Statistical analysis
R software (version 3.5.1; http://www.Rproject.org) was used to conduct statistical analysis. Statistical description was analyzed for all variables. These included frequencies for categorical parameters, and means and standard deviations (SD) or medians and interquartile ranges (IQR) for continuous parameters. Continuous values were compared using Student-t tests between different groups. Univariable analysis of the correlation of PGS and other clinicopathologic features with OS was estimated using log-rank test. For features significantly correlated with OS in univariable analyses, the Cox proportional hazards regression model was used to perform multivariable analysis. P value less than 0.05 was defined as statistical significance in all tests.
Results
Construction and definition of the gene expression signature
A total of 192 CRC patients were included in the analysis. The baseline clinical characteristics of training and validation data sets were shown in Table 1. From TCGA dataset, 18,113 genes were measured by all platforms and 4,172 genes were filtered by the conditions with MAD more than 0.5 and expression level more than median level. Using Cox regression to resample the discovery cohort, the association of 4,172 genes with OS was analyzed, resulting in 197 robust prognostic genes (>75 times showed significant during resampling). Then a prognostic gene signature (PGS) consisting of 15 genes was constructed with the use of LASSO Cox proportional hazards regression on the training cohort (Figure 1 and Table S1). The optimal cutoff from ROC curve analysis for the PGS to classify patients into the high or low risk group was 0.971 for 3-year OS (Figure 2).
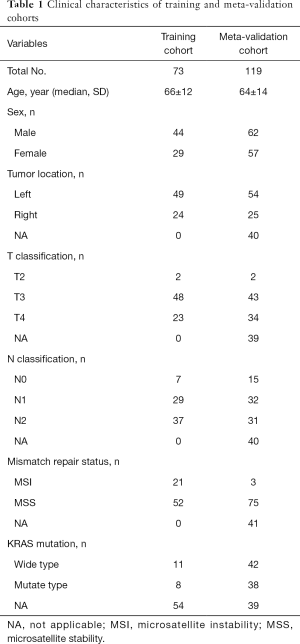
Full table
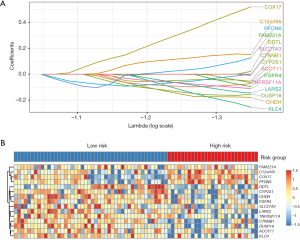
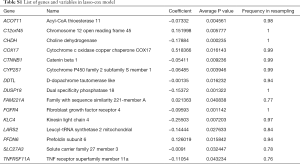
Full table
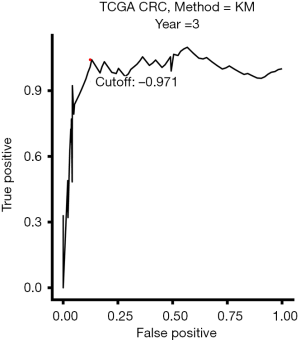
Validation of the gene expression signature
Univariate analysis showed that the PGS classified patients into low-risk and high-risk groups in terms of OS in mCRC patients from TCGA dataset (Figure 3A) and meta-validation (Figure 3B). After adjusting for clinical features such as age, gender, tumor location and molecular types, PGS still resulted as an independent prognostic factor in multivariate analyses (11.54; 95% CI: 4.44–29.99; P<0.001). A higher GPI was associated with significantly poorer prognosis in the independent meta-validation cohorts (1.57; 95% CI: 1.16–2.11; P=0.003). Overall, the PGS may estimate OS independently of clinical parameters in mCRC (Table 2).
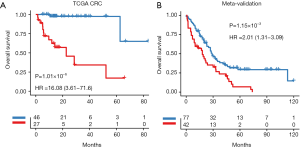
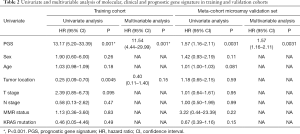
Full table
Functional annotation of the prognostic genes
We further investigate the potential functional mechanisms between the high risk and low risk divided by PGS. Gene Set Enrichment Analysis (GSEA) was performed between predicted high-risk vs. low-risk groups for cancer hallmark pathways, and identified several cancer-related biological processes gene sets including angiogenesis (P<0.001), epithelial mesenchymal transit (P<0.001), inflammatory response (P=0.001), TNF-α-NF-κB (P<0.001), IL6-JAK-STAT3 (P=0.002) and interferon-α response (P<0.001) signal pathways (Figure 4). These findings suggested that an altered expression of PGS may be related with colorectal cancer biology via the disruption of known critical biological pathways involved in cancer progression.
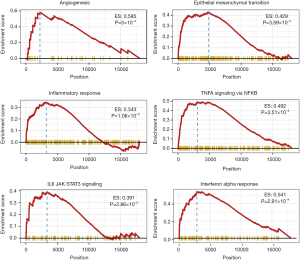
Discussion
Patients with mCRC are at substantial risk for death. The treatment for mCRC is complicated and the outcome is not promising. Therefore, reliable prognostic markers are in great need to select patients at the highest risk for death and require more intensive treatment. Here a prognostic gene expression signature was developed for mCRC and was further validated in independent multiple datasets. This prognostic gene signature stratifies stage IV CRC patients into subsets with different survival outcomes.
Recently several independent studies have proposed prognostic subtypes based on distinct global gene expression profiles to improve the stratification and treatment of CRC patients (16-19). However, none has investigated the role of gene expression in mCRC that harbor tumor heterogeneity. Thus, an individualized approach to stratify patients and guide treatment of mCRC is in great need. To provide a more accurate calculation of OS, we integrated clinicopathological characteristics and gene expression-based signatures from multiple public data sets and applied methods which are exclusively designed across different platforms with RNA-Seq or microarray technologies. Moreover, our prognostic gene signature was validated by multiple independent data sets, which may provide opportunity to translate into clinical routine practice.
Prognostic or predictive biomarkers which was associated with tumor microenvironment may hold great promise to identify new molecular targets and improve patient individualized management. Our analysis showed that gene expressions from angiogenesis signal pathway were associated with survival outcome of mCRC, which was consistent with the previous findings. The only antiangiogenic agent—bevacizumab, is approved by the US Food and Drug Administration for the first-line treatment of mCRC and shows improvement in response rate (RR) and progression-free survival (PFS) (20-22). Furthermore, some genes contained in signature played an important role in the pathways of epithelial mesenchymal transition (EMT), which is responsible for the development and aggressiveness of mCRC. Similarly, Calon et al. identified stromal gene expressions for EMT which defined the poor-prognosis subtype in CRC (23). Our proposed gene signatures also implied the crucial role of inflammatory response in mCRC. As many studies showed, an increased inflammatory microenvironment has been demonstrated to be an important element of neoplastic process and tumor progression (24-27). Our GSEA identified TNF-α-NF-κB and IL6-JAK-STAT3 pathways, which were well-known to play a crucial role in the progression and proliferation of mCRC in numerous studies (28-30). Above all, our proposed gene signatures included the molecules from various crucial biological processes.
Limitations of the present study include its retrospective nature, although we validated the signatures in independent data sets. Furthermore, gene expression-based signatures that are subject to the samples from primary tumor or metastatic disease may have inconsistent genetic heterogeneity. Although we investigated as many genes as possible, future studies are need to explore different biological processes that could provide a more comprehensive molecular landscape of mCRC.
Conclusions
In summary, the proposed gene expression-based signature is a promising prognostic tool to predict survival in mCRC. Further prospective studies are in need to validate its feasibility of analysis for assessing prognoses and to exam its clinical utility in personized treatment of mCRC.
Acknowledgments
Funding: This work was supported by National Natural Science Foundation of China (Grant No. 81703060, 81802441), Natural Science Foundation of Guangdong Province (Grant No. 2017A030310644), China Postdoctoral Science Foundation funded project (Grant No. 2018T110911, 2019B020229002), the National Key Research and Development Program of China (N0.2017YFC1308800), Science and Technology Planning Project of Guangdong Province (No. 20160916, 2014SC111, 201902020009), and National Key Clinical Discipline.
Footnote
Conflicts of Interest: The authors have no conflicts of interest to declare.
Ethical Statement: The authors are accountable for all aspects of the work in ensuring that questions related to the accuracy or integrity of any part of the work are appropriately investigated and resolved. Data can be obtained from Gene Expression Omnibus (GEO) database and Broad GDAC Firehose (http://gdac.broadinstitute.org/), therefore the ethical review is exempted.
References
- Siegel RL, Miller KD, Jemal A. Cancer Statistics, 2017. CA Cancer J Clin 2017;67:7-30. [Crossref] [PubMed]
- Siegel RL, Fedewa SA, Anderson WF, et al. Colorectal Cancer Incidence Patterns in the United States, 1974-2013. J Natl Cancer Inst 2017. [Crossref] [PubMed]
- Yaeger R, Chatila WK, Lipsyc MD, et al. Clinical Sequencing Defines the Genomic Landscape of Metastatic Colorectal Cancer. Cancer Cell 2018;33:125-36. [Crossref] [PubMed]
- Thomsen CB, Andersen RF, Lindebjerg J, et al. Plasma Dynamics of RAS/RAF Mutations in Patients With Metastatic Colorectal Cancer Receiving Chemotherapy and Anti-EGFR Treatment. Clin Colorectal Cancer 2019;18:28-33.e3. [Crossref] [PubMed]
- Garcia-Foncillas J, Tabernero J, Elez E, et al. Prospective multicenter real-world RAS mutation comparison between OncoBEAM-based liquid biopsy and tissue analysis in metastatic colorectal cancer. Br J Cancer 2018;119:1464-70. [Crossref] [PubMed]
- Mooi JK, Wirapati P, Asher R, et al. The prognostic impact of consensus molecular subtypes (CMS) and its predictive effects for bevacizumab benefit in metastatic colorectal cancer: molecular analysis of the AGITG MAX clinical trial. Ann Oncol 2018;29:2240-6. [Crossref] [PubMed]
- Martinez-Useros J, Rodriguez-Remirez M, Borrero-Palacios A, et al. DEK is a potential marker for aggressive phenotype and irinotecan-based therapy response in metastatic colorectal cancer. BMC Cancer 2014;14:965. [Crossref] [PubMed]
- Ning Y, Hanna DL, Zhang W, et al. Cytokeratin-20 and Survivin-Expressing Circulating Tumor Cells Predict Survival in Metastatic Colorectal Cancer Patients by a Combined Immunomagnetic qRT-PCR Approach. Mol Cancer Ther 2015;14:2401-8. [Crossref] [PubMed]
- Barbazan J, Dunkel Y, Li H, et al. Prognostic Impact of Modulators of G proteins in Circulating Tumor Cells from Patients with Metastatic Colorectal Cancer. Sci Rep 2016;6:22112. [Crossref] [PubMed]
- Maddalena F, Simeon V, Vita G, et al. TRAP1 protein signature predicts outcome in human metastatic colorectal carcinoma. Oncotarget 2017;8:21229-40. [Crossref] [PubMed]
- Comprehensive molecular characterization of human colon and rectal cancer. Nature 2012;487:330-7. [Crossref] [PubMed]
- Marisa L, de Reynies A, Duval A, et al. Gene expression classification of colon cancer into molecular subtypes: characterization, validation, and prognostic value. PLoS Med 2013;10:e1001453. [Crossref] [PubMed]
- Kirzin S, Marisa L, Guimbaud R, et al. Sporadic early-onset colorectal cancer is a specific sub-type of cancer: a morphological, molecular and genetics study. PLoS One 2014;9:e103159. [Crossref] [PubMed]
- Smith JJ, Deane NG, Wu F, et al. Experimentally derived metastasis gene expression profile predicts recurrence and death in patients with colon cancer. Gastroenterology 2010;138:958-68. [Crossref] [PubMed]
- Wang X, Terfve C, Rose JC, et al. HTSanalyzeR: an R/Bioconductor package for integrated network analysis of high-throughput screens. Bioinformatics 2011;27:879-80. [Crossref] [PubMed]
- Nannini M, Pantaleo MA, Maleddu A, et al. Gene expression profiling in colorectal cancer using microarray technologies: results and perspectives. Cancer Treat Rev 2009;35:201-9. [Crossref] [PubMed]
- Dienstmann R, Vermeulen L, Guinney J, et al. Consensus molecular subtypes and the evolution of precision medicine in colorectal cancer. Nat Rev Cancer 2017;17:268. [Crossref] [PubMed]
- Sinicrope FA, Okamoto K, Kasi PM, et al. Molecular Biomarkers in the Personalized Treatment of Colorectal Cancer. Clin Gastroenterol Hepatol 2016;14:651-8. [Crossref] [PubMed]
- Guinney J, Dienstmann R, Wang X, et al. The consensus molecular subtypes of colorectal cancer. Nat Med 2015;21:1350-6. [Crossref] [PubMed]
- Fakih MG. Metastatic colorectal cancer: current state and future directions. J Clin Oncol 2015;33:1809-24. [Crossref] [PubMed]
- Kabbinavar FF, Hurwitz HI, Yi J, et al. Addition of bevacizumab to fluorouracil-based first-line treatment of metastatic colorectal cancer: pooled analysis of cohorts of older patients from two randomized clinical trials. J Clin Oncol 2009;27:199-205. [Crossref] [PubMed]
- Cunningham D, Lang I, Marcuello E, et al. Bevacizumab plus capecitabine versus capecitabine alone in elderly patients with previously untreated metastatic colorectal cancer (AVEX): an open-label, randomised phase 3 trial. Lancet Oncol 2013;14:1077-85. [Crossref] [PubMed]
- Calon A, Lonardo E, Berenguer-Llergo A, et al. Stromal gene expression defines poor-prognosis subtypes in colorectal cancer. Nat Genet 2015;47:320-9. [Crossref] [PubMed]
- Di Caro G, Carvello M, Pesce S, et al. Correction: Circulating Inflammatory Mediators as Potential Prognostic Markers of Human Colorectal Cancer. PLoS One 2016;11:e156669. [PubMed]
- Mima K, Nishihara R, Yang J, et al. MicroRNA MIR21 (miR-21) and PTGS2 Expression in Colorectal Cancer and Patient Survival. Clin Cancer Res 2016;22:3841-8. [Crossref] [PubMed]
- Galdiero MR, Bianchi P, Grizzi F, et al. Occurrence and significance of tumor-associated neutrophils in patients with colorectal cancer. Int J Cancer 2016;139:446-56. [Crossref] [PubMed]
- Becht E, de Reynies A, Giraldo NA, et al. Immune and Stromal Classification of Colorectal Cancer Is Associated with Molecular Subtypes and Relevant for Precision Immunotherapy. Clin Cancer Res 2016;22:4057-66. [Crossref] [PubMed]
- De Simone V, Franze E, Ronchetti G, et al. Th17-type cytokines, IL-6 and TNF-alpha synergistically activate STAT3 and NF-kB to promote colorectal cancer cell growth. Oncogene 2015;34:3493-503. [Crossref] [PubMed]
- Cooks T, Pateras IS, Tarcic O, et al. Mutant p53 prolongs NF-kappaB activation and promotes chronic inflammation and inflammation-associated colorectal cancer. Cancer Cell 2013;23:634-46. [Crossref] [PubMed]
- Lu YX, Ju HQ, Wang F, et al. Inhibition of the NF-kappaB pathway by nafamostat mesilate suppresses colorectal cancer growth and metastasis. Cancer Lett 2016;380:87-97. [Crossref] [PubMed]