Altered expression of long noncoding RNAs in peripheral blood mononuclear cells in patients with impaired leptomeningeal collaterals after acute anterior large vessel occlusions
Introduction
Acute ischemic stroke (AIS) due to anterior large vessel occlusion (aLVO) is a devastating event with poor functional outcomes and high mortality rates in spite of recent advances in treatment (1-3). Indeed, in the event of aLVO, leptomeningeal collaterals (LMCs) are believed to provide substantial protection to the ischemic brain tissue, which made the LMCs status a key factor that defines the severity and functional prognosis of this disease and an important parameter in identifying patients who may benefit from recanalization therapy (4-9). However, there is robust evidence from imaging studies to show that the extent of LMCs at baseline exhibits substantial variability among patients with aLVO (1,2,4-9). Thus, it is critical to address the potential causes for this inter-individual variability for developing more efficient and accurate diagnostic method to predict LMCs status and personalizing the therapy for individual patients of aLVO.
Previous findings indicated that LMCs status is genetic determined (7,8). In the past few years, long non-coding RNAs (lncRNAs) which have a long transcription sequence between 200 nucleotides and 100 kb have been found to be involved in specific physiological and pathological processes of a wide range of human diseases and disorders, including AIS and revascularization after AIS (10-14). LncRNA expression profiles in human peripheral blood were found to be altered after AIS and several lncRNAs in peripheral blood mononuclear cells (PBMCs) were identified as novel potential diagnostic biomarkers of AIS (11,12). However, whether there are specific lncRNAs associated with LMC status and serve as biomarkers for predicting LMCs status in patients with aLVO has not yet been investigated.
Therefore, to facilitate the molecular diagnosis of LMCs status and to expand the understanding of pathogenesis of LMCs formation (a process termed ‘arteriogenesis’) following AIS, we performed microarray analysis to identify the expression profiles of lncRNAs in aLVO patients with different LMCs status and control subjects. Twenty-three differentially expressed lncRNAs from the microarray were identified in patients with poor LMCs status. The sample size was expanded for further independent validations and then, a significant downregulated lncRNA, ENST00000422956, designated as lncLMC1, was initially identified to be related with poor LMCs status. Our results indicated that lncLMC1 may be a candidate in predicting poor LMCs status in AIS and our finding might be the foundation for understand the potential roles of lncRNAs in arteriogenesis after ischemic stroke.
Methods
Study subjects
This is a prospective cohort study at the First Affiliated Hospital of Chongqing Medical University. A total of 207 subjects including 134 AIS patients with aLVO and 73 healthy controls (HCs) were enrolled from January 2018 to April 2019. Patients were recruited in the study if they were ≥18 years old, first found abnormal (FFA) within 24 h and baseline computed tomographic angiography (CTA) demonstrated M1 middle cerebral artery (MCA) ± internal carotid artery (ICA) occlusions. The exclusive criteria consisted of intracranial hemorrhage on non-contrast CT (NCCT), transient ischemic stroke, malignant tumors and other severe systemic diseases, autoimmune disease and drug or alcohol abuse. During the same period, a group of HCs who had no history of previous ischemic or other neurological disorders were recruited from the physical examination center in our hospital. Healthy subjects were matched for age, sex, and number of major stroke risk factors (hypertension, diabetes mellitus, hypercholesterolemia, smoking, obesity and coronary heart disease).
The protocol was approved by the institutional review board at the participating hospital (File No. 2018-066-2) and was registered in Chinese Clinical Trial Registry (File No. ChiCTR1800018569). Written informed consent was provided by the participants or their proxy in accordance with Declaration of Helsinki of 1975.
Clinical variables and neuroimaging
All participants had standard assessments of demographic characteristics, classical risk factors, blood pressure (BP) and blood glucose level obtained at admission. For patients, baseline National Institutes of Health Stroke Scale (NIHSS) score, stroke etiology according to the Trial of ORG 10172 in Acute Stroke Treatment (TOAST) criteria (15), and modified Rankin scale (mRS) score pre-stroke and 90 days post-stroke were noted. Use of antiaggregant/anticoagulant was determined when the participant used within 3 months due to non-stroke reasons. The treatment protocol was applied according to the international stroke management guide and was decided at the treating neurologist’s discretion. The primary outcome was the stroke severity assessed by baseline NIHSS score; mild stroke was defined as NIHSS ≤5 and moderate-severe stroke was defined as NIHSS ≥6 (12). Secondary outcomes included mRS score at 3 months (a favorable functional outcome was defined as mRS ≤2) and rate of symptomatic intracranial hemorrhage (SICH) by applying the definition from the second European-Australasian acute stroke study (ECASS II) criteria (5).
All patients underwent a standard non-helical NCCT at admission performed on a multi-slice scanner (GE Discovery CT750HD, GE Healthcare, WI, USA) using 100 kV, 120 mAs with 5 mm slice thickness. NCCT was followed by CTA of head and neck, which was performed by scanning from the cerebral vertex to the aortic arch with 5 mm section thickness slices. Nonionic contrast media (70–80 mL) was administered into the antecubital vein at 3–5 mL/s, and the CTA source images were postprocessed and reformatted to create axial, coronal and sagittal multiplanar images using 20-mm-thick slabs. Follow-up (24 h–7 d) magnetic resonance imaging (MRI) (GE 3.0T, GE Healthcare), unless there were contraindications to MRI, in which case repeat NCCT was performed. Baseline and follow-up imaging was analyzed were interpreted separately by one neuroradiologist and one stroke neurologist, and final decisions on the findings were reached by a consensus. LMCs were assessed on baseline CTA by consensus using the regional LMC (rLMC) score (16). AIS patients were classified into 2 groups based on baseline rLMC score: good LMCs (rLMC score >10) and poor LMCs (rLMC score ≤10) (4).
Blood sampling and processing
Peripheral venous blood samples (4–5 mL) were obtained in a sodium citrate tube from the enrolled patients on arrival at the Emergency Department, whereas the blood samples from the controls were taken before their breakfast. PBMCs were isolated within 2 h of blood draw by using Ficoll (TBD science, Tianjin, China) gradient centrifugation. PBMCs were then transferred into 1 mL TRIzol Reagent in 1.5 mL centrifuge tubes and stored at –80 °C until RNA extraction.
RNA extraction and lncRNA microarray analysis
Total RNA was extracted from PBMCs by using TRIzol Reagent (Invitrogen, CA, USA) according to the manufacturer’s protocol and quantified with the NanoDrop ND-1000 (Thermo Scientifc, USA). Then, RNA samples obtained from 3 patients with good LMCs status, 3 patients with poor LMCs status and 3 HCs (discovery set) were used for lncRNA microarray analysis using Shbio Human lncRNA Microarray (4×180 K, platform: GPL21047, Agilent Technologies, Santa Clara, CA, USA), provided by Shanghai Biotechnology Corporation (Shanghai, China). A total of 77,103 lncRNAs and 18,853 coding transcripts were detected. Slides were scanned by Agilent microarray scanner (Agilent Technologies) with default settings, dye channel: green, scan resolution =3 µm, photoelectric multiplication tube (PMT) 100%, 20 bit. Data were extracted with Feature Extraction software 12.0 (Agilent Technologies). Raw data were normalized by Quantile algorithm, limma packages in R. Significant differential expressed transcripts were screened by fold change (FC) ≥2 or ≤–2 and P value <0.05.
Real-time polymerase chain reaction (RT-PCR) validations
After reverse transcription using a PrimeScript RT reagent Kit (Takara, Dalian, China), lncRNA expressions were detected by quantitative reverse-transcription RT-PCR. The first stage RT-PCR validation was performed in 10 patients with good LMCs status, 10 patients with poor LMCs status and 10 HCs (training set). The expression of screened lncRNAs was further confirmed in another independent set of 62 patients with poor LMCs status, 46 with good LMCs status and 60 HCs (test set). LncRNA Primers were provided by Invitrogen (CA, USA) (Table S1).
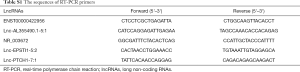
Full table
Quantitative RT-PCR was performed using a T100TM Thermal Cycler (Bio-Rad, CA, USA). All experiments were repeated in triplicate. Briefly, 10 µL reaction mixture containing 5 µL of SYBR Master mix, 1 µL of cDNA template, 0.50 µL of each primer and 3 µL of ddH2O was amplified by the following thermal parameters: an initial incubation at 95 °C for 3 min, followed by 41 cycles of denaturation at 95 °C for 30 s, annealing at 56.2 °C for 30 s, and extension at 72 °C for 30 s. The cycle threshold (Ct) value of lncRNA was normalized to the housekeeping gene [glyceraldehyde 3-phosphate dehydrogenase (GAPDH)]. The data analysis was carried out using the Bio-Rad CFX Manager software based on the comparative Ct method. The relative expression levels were determined by the 2–△△Ct method.
Bioinformatic analysis
LncRNA target prediction and functional group analysis: we identified the target neighboring genes of differentially expressed lncRNAs via cis-or trans-regulatory effects. Gene ontology (GO) functional annotation and Kyoto Encyclopedia of Genes and Genomes (KEGG) pathway analysis were performed according to the latest GO (http://geneontology.org/) or KEGG database (http://www.genome.jp/kegg/) to determine the biological roles of cis-/trans-target genes.
Co-expression network construction: we performed the lncRNAs-mRNAs co-expression analysis based on calculating the Pearson’s correlation coefficient (PCC) between the expression levels of mRNA and lncRNA in the SBC human lncRNA array analysis. The value of parameter PCC ≥0.70 and P value <0.05 was recommended for further analysis. The open-source bioinformatics software Cytoscape (version 3.4.0) was used to draw the network. Degree centrality (the number of links between nodes) of lncRNA-mRNA within the network determined the relative importance of the nodes.
Statistical analysis
All statistical analyses were performed with GraphPad Prism 5.0 (CA, USA) and SPSS 23.0 (SPSS, Chicago, IL, USA). Continuous variables are reported as mean ± standard deviation (SD) or as medians [interquartile range (IQR)]. Categorical variables are expressed as numbers (percentages). Comparison between groups were assessed by using Student’s t-test or one-way analysis of variance (ANOVA) for parametric data, Mann-Whitney U test or Kruskal-Wallis test for nonparametric data, and Pearson chi-square test for categorical data. Correlation analysis was performed using Spearman’s test. Diagnostic performance of candidate biomarkers was analyzed by receiver-operating characteristic (ROC) curves and the area under the ROC curve (AUC). Independent factors for poor LMCs status were evaluated using multivariate logistic regression analysis. Variables from univariate analyses at P<0.1 were considered to represent explanatory variables and were included in the multivariable model. A value of P<0.05 (two-sided) was considered statistically significant.
Results
Characteristics of the study participants
From January 2018 to April 2019, a total of 207 subjects including 134 patients with aLVO (39.6% females, mean age 67.9±11.8 years) and 73 HCs (54.8% females, mean age 63.9±10.2 years) were included in the analysis. According to the demonstration on admission CTA, 75 (56.0%) patients were graded as having poor LMCs and 59 (44.0%) patients were graded as having good LMCs (Figure 1). Then, all the subjects were divided into three independent sets: the discovery set included 3 patients with poor LMCs status, 3 patients with good LMCs status and 3 HCs (44.4% females, mean age 64.4±4.4 years). An independent training set of 10 poor LMCs patients, 10 good LMCs patients and 10 HCs (43.3% females, mean age 62.8±14.2 years) was investigated to verify the results of the discovery set. Then, we further validated the expression of candidate lncRNAs in another independent test set of 62 patients with poor LMCs, 46 patients with good LMCs and 60 HCs (45.2% females, mean age 67.3±11.0 years). As demonstrated in Tables 1-3, no significant differences were observed among the three subgroups with respect to age, sex and medical history.
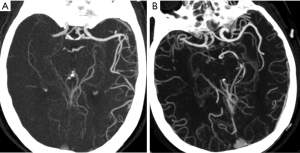
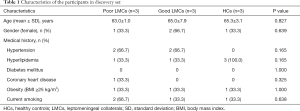
Full table
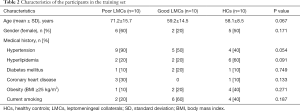
Full table
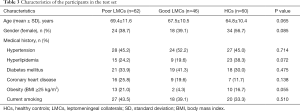
Full table
In the totality of patients of aLVO, 109 (81.3%) patients suffered from moderate-severe stroke. The overall median 90 days mRS was 4 [2–6]. Only 41 subjects (31.1%) (2 patients’ follow-up data were not available) achieved a good functional outcome (90-day mRS ≤2). Notably, the rate of moderate-severe stroke in patients with poor LMCs status was much higher than that in patients with good LMCs status (89.3% vs. 71.2%, P=0.007). Moreover, even though poor LMCs subgroup and good LMCs subgroup did not differ regarding the rates of receiving revascularization therapy (48.0% vs. 59.3%; P=0.192) and time-to-initiation of intravenous thrombolysis (IVT) (50.7±20.0 vs. 50.1±25.9, P=0.912) or endovascular thrombectomy (ET) (164.1±74.7 vs. 229.2±128.8, P=0.067), subjects with poor LMCs status had poorer clinical outcomes. Favorable functional outcomes were seen in only 10 of 74 patients with poor LMCs status and in 31 of 58 patients with good LMCs status (13.5% vs. 53.4%, P<0.001). Moreover, patients with poor LMCs status had higher rate of SICH (24.0% vs. 10.2%, P=0.038) and 3-month mortality (41.9% vs. 10.3%, P<0.001).
Discovery set: microarray profiling of lncRNAs
To determine whether there were specific lncRNAs associated with poor LMCs status in aLVO patients, we profiled lncRNA expression in PBMCs from 3 patients with good LMCs (G1, G2, G3), 3 patients with poor LMCs (P1, P2, P3) and 3 HCs (H1, H2, H3) using the Human LncRNA Array (4×180 K, Agilent Technologies). By using the criteria of absolute FC >2, P<0.05, 179 lncRNAs were found to be differentially expressed between poor LMCs subgroup and good LMCs subgroup with 93 up-regulated and 86 were down-regulated; 336 lncRNAs were found to be differentially expressed between good LMCs subgroup and HCs with 114 up-regulated and 222 down-regulated; 721 lncRNAs were found to be differentially expressed between poor LMCs subgroup and HCs with 207 up-regulated and 514 down-regulated. The lncRNA expression variations between each two sample subgroups were illustrated in the heat map (Figure 2A) and scatter plots (Figure 2B). A forward analysis of distinguished lncRNAs based on their categorization was performed. All differentially expressed lncRNAs were classified into five groups: bidirectional, exonic-antisense, exonic-sense, intergenic, and other. The pie charts show the components of the dysregulated lncRNAs in each category (Figure 2C).
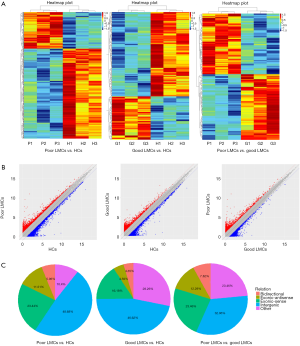
To identify specific lncRNAs associated with poor LMCs status, we selected potential biomarkers using the following strategy: gene that differentially expressed in poor LMCs subgroup when compared with good LMCs as well as HCs subgroups. After the selection procedure, 23 lncRNAs met these criteria (Table 4). Figure 3 presents the bar plots which were visualized to assess lncRNA expression variation. Then, to confirm the stability of the microarray data, we randomly selected 5 lncRNAs (ENST00000422956, lnc-AL355490.1-5:1, lnc-EPSTI1-5:2, lnc-PTCH1-7:1, NR_003672) among the 23 differentially expressed lncRNAs to detect their expression levels using RT-PCR. RT-PCR of these 5 lncRNAs showed that their expression signatures in the discovery set were consistent with the microarray profiling data (Figure 4).
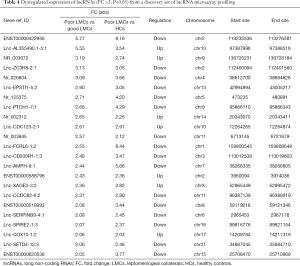
Full table
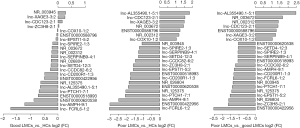
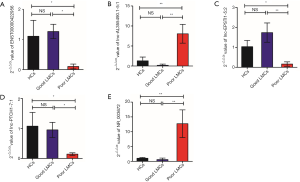
Training set: first stage RT-PCR validation and functional characteristic of the selected lncRNAs
At the first stage of validation, we selected the top 3 lncRNAs that were mostly differentially expressed in the two subgroups of patients (Figure 3C) (ENST00000422956, lnc-AL355490.1-5:1, NR_003672) and performed RT-PCR validation of their expression in the training set. The results revealed that 2 lncRNAs were differentially expressed in the poor LMCs patients. Compared to good LMCs patients and HCs, ENST00000422956 was significantly down-regulated in poor LMCs patients (P<0.05) (Figure 5A) and NR_003672 was significantly up-regulated (P<0.05) (Figure 5B). However, there was no difference of lnc-AL355490.1-5:1 expression in poor LMCs subgroup (Figure 5C).
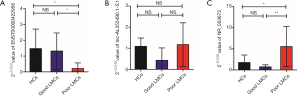
Meanwhile, to investigate whether the dysregulated lncRNAs are involved in the regulation of genes and potential signaling pathways related to poor LMCs, we used cis- or trans-regulatory predicted patterns to find target genes for the these 3 lncRNAs. As the result, the target gene of ENST00000422956 is paired box 8 (Pax8). Lnc-AL355490.1-5:1 is an antisense transcript of ribosomal RNA processing 12 homolog (PRP 12). Additionally, family with sequence similarity 69 member B (FAM69B) and lipocalin 10 (LCN10) are the target genes of NR_003672. GO analysis indicated that several functional pathways were enriched, including DNA binding, nucleic acid binding and cellular response to stimulus, etc. Besides, KEGG pathway analysis showed that these lncRNAs were mapped in thyroid cancer pathway, pathways in cancer and base excision repair.
Then, we constructed lncRNAs-mRNA co-expression network analysis containing these top 3 mostly differentially expressed lncRNAs (2 up-regulated and 1 down-regulated) and 145 associated mRNAs (8 up-regulated and 137 down-regulated). Figure 6 showed that ENST00000422956 correlated with the largest numbers of associations and has a particularly high degree, suggesting that it might be the hub for this lncRNAs module.
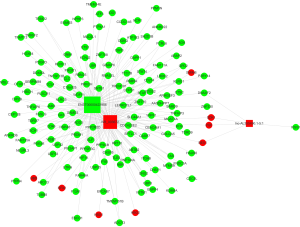
Test set: independent validation of lncRNA-ENST00000422956 and its diagnostic values for poor LMCs status
Considering lncRNA-ENST00000422956 might be the most important among the three lncRNAs and involved in the process of LMCs formation, we investigated this lncRNA in another independent set of 62 poor LMCs patients, 46 good LMCs patients and 60 HCs. By using RT-PCR, ENST00000422956 exhibited significantly decreased expression in patients with poor LMCs status compared to patients with good LMCs status and HCs (both P value <0.05) (Figure 7A). Then, to assess the potential usefulness of ENST00000422956 for prediction of poor LMCs status in aLVO patients, we performed a ROC curve analysis, which revealed an AUC of 0.749 [95% confidence interval (CI), 0.657–0.841; P<0.001] with sensitivity of 71.7% and specificity of 66.1% at the cutoff value of 1.136-fold (Figure 7B).
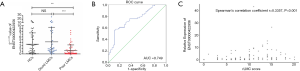
To further determine the predictors for poor LMCs status, two subgroups of patients according to LMCs status were compared in this group. In univariate analyses, there were a statistically significant difference in ENST00000422956 PBMCs level (P<0.001), baseline NIHSS score (P<0.001), admission diastolic BP (P=0.040) and rate of M1 + ICA occlusion (P=0.008) between the two subgroups of patients (Table 5). In multivariate analysis, PBMCs ENST00000422956 expression level [OR, 0.792 (0.669–0.936); P=0.006] and baseline NIHSS score [OR, 1.130 (1.040–1.227); P=0.004] were identified as independent predictors for impaired LMCs (Table 6). Besides, a significantly positive correlation was observed between lncRNA-ENST00000422956 level and LMCs status, as assed by rLMC score among all patients in the test set (Spearman’s correlation coefficient r=0.3357, P<0.001) (Figure 7C). These results indicated that ENST00000422956 was associated with poor LMCs status in aLVO patients and may be a potential candidate biomarker to predict poor LMCs. Thus, ENST00000422956 was renamed lncLMC1 in the present study.
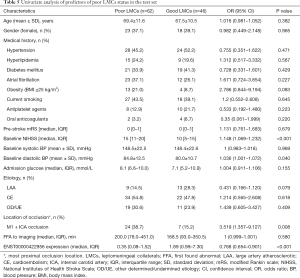
Full table
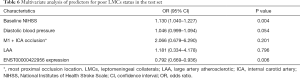
Full table
LncLMC1 was associated with stroke severity of aLVO patients in the test set
Given that lncRNAs are involved in AIS with ischemia/reperfusion injury (10-14,17,18) and LMCs status is a key factor that defines the severity of aLVO, we hypothesized that the identified PBMCs lncLMC1 may be associated with neurological deficit severity in aLVO patients. To test this hypothesis, we analyzed their expression levels in the test set of AIS patients classified by the NIHSS scores. The results showed that PBMCs LncLMC1 level in moderate-severe stroke were significantly lower than those in mild stroke [0.99 (0.20–2.64) vs. 1.89 (0.72–7.15); P=0.035].
Discussion
This is the first study reporting the altered expression of lncRNAs in blood of humans with impaired LMCs after acute aLVOs. A key finding of the present study was that decreased lncLMC1 expression in PBMCs was associated with impairment of LMCs in patients with aLVO, suggesting that measurement of circulatory lncRNAs might now be included as possible biomarkers to be explored further for evaluation of LMCs status in AIS and its causes.
As demonstrated in our results, in the event of aLVO, LMCs status is a key factor to predict the severity and functional prognosis of this devastating disease. Previous work aimed at estimating LMCs status mainly focused on digital subtraction angiography (DSA) or on CTA evaluations (16,19). However, collateral imaging techniques are limited by offering only a snapshot assessment of LMCs viability, and providing no visualization of the small inter-arteriolar connections directly (20). Meanwhile, it takes a certain time and the patient’s cooperation to complete the imaging examination. Therefore, this is an imprecise and challenging process to assess LMCs status based on information extracted only from radiologic examinations and there is an urgent need for new methods to aid in the evaluation of LMCs status in aLVO.
Previous studies have indicated that epigenetic biomarkers may facilitate clinical assessment and accurate prediction of various diseases (12). LncRNAs belong to a novel class of non-coding RNAs and constitute a significant portion of the mammalian genome (10,13). They are often cell and tissue specific and can be found between gene coding regions (long intergenic noncoding RNAs), overlapping coding genes in either sense or antisense directions, as pseudogenes that have sequence similarity to coding genes or as mRNA-like lncRNAs (17). LncRNAs are stable in the plasma and other body fluids and have therefore served as biomarkers for cardiovascular disease (21) and cancers (22-24). In ischemic stroke, Dykstra-Aiello et al. (17) and Deng et al. (12) provided evidence that lncRNAs were aberrantly expressed in peripheral blood of patients with and suggested that lncRNAs have potential for stroke biomarker development. Therefore, our current findings that lncRNA ENST00000422956 (referred as ‘lncLMC1’) significantly differentially expressed in patients with poor LMCs status could be considered in development of future biomarker panels to differentiate poor LMCs status in aLVO patients.
Furthermore, growing evidences suggest that although lncRNAs have no protein-coding capability, they can execute functions by regulating the expression of the neighboring or overlapping coding genes and are involved in specific physiological and pathological processes of a wide range of human diseases (10-14,21,25). In ischemic stroke, lncRNAs were reported to be involved in not only the pathophysiology of stroke but also angiogenesis after AIS (11-14,17). Downregulation of the lncRNA Meg3 resulted in a proangiogenic effect evidenced by increased endothelial cell migration, proliferation, sprouting, and tube formation after ischemic brain injury in rats (13). Also, Zhang et al. (26) identified oxygen-glucose deprivation (OGD)-responsive brain endothelial lncRNAs suggesting potential pathological roles for these lncRNAs in mediating endothelial responses to ischemic stimuli. However, whether lncRNAs are involved in the regulation of arteriogenesis after ischemic stroke remains poorly defined. To address this issue, we analyzed our microarray datasets and identified a novel human lncRNA, lncLMC1 which correlated with poor LMCs status as well as stroke severity in patients with aLVO, indicating that lncLMC1 might be involved in the process arteriogenesis following AIS. Via cis-regulatory prediction analysis, Pax8 was identified as the target gene for lncLMC1. As a cell lineage-restricted transcription factor, Pax8 is known to control the development of the central nervous system, eye, kidney, thyroid gland, organs deriving from the mesonephric duct and those related to the Müllerian duct, and it is constantly expressed in these normal organs or tissues (27). Current studies of Pax8 were mainly focused on tumors derived from these organs, especially thyroid (28), kidney (29) and ovarian carcinomas (30). Our KEGG analysis showed that Pax8 was involved in several pathways in cancers, which was consistent with the previous demonstrations. Moreover, a recent study of Pax8 in thyroid tumors demonstrated that neuropilin-2 (NRP2) was its potential transcriptional target (31). By directly binding to a region of the NRP2 promoter, Pax8 negatively regulated the expression of NRP2, which contributed to reduction of tumor cell proliferation, migration ability, and invasion activity (31,32). Interestingly, as multifunctional single-spanning trans-membrane glycoprotein, NRP2 also plays a central role in neuronal and blood vessel development as a co-receptor for vascular endothelial growth factor A (VEGF-A) and its homologues (32-34). Recent animal studies have reported that VEGF-A was critical for promoting collateral growth and de novo arteriogenesis and thus impacted LMCs status in development and ischemic disease (7,35-37). Indeed, arteriogenesis is intrinsically related to endothelial function and VEGF-A exerts the majority of its actions by binding to VEGF receptor 2 (VEGFR2), a master regulator of endothelial cell function (2,8,35,38). Activation of VEGFR2 will initiate multiple signaling pathways that regulate endothelial proliferation, migration, adhesion and lumenization (39). Thus, we speculated that a network regulated by lncLMC1 through Pax8/NRP2 pathway might be involved in arteriogenesis in AIS. Subsequent work should be performed in vitro and vivo to further understand the role and underlying mechanism of LMCs formation.
Certain limitations of our study need to be acknowledged. First, this was a single-center study with a relatively small sample size. Thus, the possibility of a type II error must be admitted and a selection bias is likely. Multicenter trials and larger sample size will have more stringency. Furthermore, the determination of peripheral lncRNAs levels was based on the measurement of a single sample obtained upon admission and, therefore, we could not evaluate possible changes of lncRNAs according to the stages of AIS.
In conclusion, this is the first study reporting the altered expression of long noncoding RNAs in humans with impaired LMCs after acute aLVOs. We have shown that circulating lncLMC1 was differentially expressed in PBMCs of patients with poor LMCs status, suggesting that measurement of circulatory lncRNAs might now be included as possible biomarkers to be explored further for evaluation of LMCs status in AIS. More importantly, it may help us to have a better understanding of the pathogenesis of arteriogenesis in AIS that lncRNAs might be involved in this process. This conclusion prompts the necessity for further studies to the function of lncRNAs in modulating LMCs formation following ischemic stroke.
Acknowledgments
Funding: This work was supported by the National Natural Science Foundation of China (grant numbers 81771275).
Footnote
Conflicts of Interest: The authors have no conflicts of interest to declare.
Ethical Statement: The authors are responsible for all aspects of the work in ensuring that questions related to the accuracy or integrity of any part of the work are appropriately investigated and resolved. And the study was approved by the institutional review boards at the participating hospital (File No. 2018-066-2) and was registered in Chinese Clinical Trial Registry (File No. ChiCTR1800018569). Written informed consent was provided by the participants or their proxy in accordance with Declaration of Helsinki of 1975.
References
- Lima FO, Furie KL, Silva GS, et al. The pattern of leptomeningeal collaterals on CT angiography is a strong predictor of long-term functional outcome in stroke patients with large vessel intracranial occlusion. Stroke 2010;41:2316-22. [Crossref] [PubMed]
- Yukami T, Yagita Y, Sugiyama Y, et al. Chronic elevation of tumor necrosis factor-α mediates the impairment of leptomeningeal arteriogenesis in db/db mice. Stroke 2015;46:1657-63. [Crossref] [PubMed]
- Shuaib A, Butcher K, Mohammad AA, et al. Collateral blood vessels in acute ischaemic stroke: a potential therapeutic target. Lancet Neurol 2011;10:909-21. [Crossref] [PubMed]
- Menon BK, Smith EE, Coutts SB, et al. Leptomeningeal collaterals are associated with modifiable metabolic risk factors. Ann Neurol 2013;74:241-8. [PubMed]
- Hacke W, Kaste M, Fieschi C, et al. Randomised double-blind placebo-controlled trial of thrombolytic therapy with intravenous alteplase in acute ischaemic stroke (ECASS II). Second European-Australasian acute stroke study investigators. Lancet 1998;352:1245-51. [Crossref] [PubMed]
- Chalothorn D, Faber JE. Formation and maturation of the native cerebral collateral circulation. J Mol Cell Cardiol 2010;49:251-9. [Crossref] [PubMed]
- Zhang H, Prabhakar P, Sealock R, et al. Wide genetic variation in the native pial collateral circulation is a major determinant of variation in severity of stroke. J Cereb Blood Flow Metab 2010;30:923-34. [Crossref] [PubMed]
- Rizzi A, Benagiano V, Ribatti D. Angiogenesis versus arteriogenesis. Rom J Morphol Embryol 2017;58:15-9. [PubMed]
- Park JS, Kwak HS, Chung GH, et al. The prognostic value of CT-angiographic parameters after reperfusion therapy in acute ischemic stroke patients with internal carotid artery terminus occlusion: leptomeningeal collateral status and clot burden score. J Stroke Cerebrovasc Dis 2018;27:2797-803. [Crossref] [PubMed]
- Ponting CP, Oliver PL, Reik W. Evolution and functions of long noncoding RNAs. Cell 2009;136:629-41. [Crossref] [PubMed]
- Guo X, Yang J, Liang B, et al. Identification of novel lncRNA biomarkers and construction of lncRNA-related networks in Han Chinese patients with ischemic stroke. Cell Physiol Biochem 2018;50:2157-75. [Crossref] [PubMed]
- Deng QW, Li S, Wang H, et al. Differential long noncoding RNA expressions in peripheral blood mononuclear cells for detection of acute ischemic stroke. Clin Sci (Lond) 2018;132:1597-614. [Crossref] [PubMed]
- Liu J, Li Q, Zhang KS, et al. Downregulation of the long non-coding RNA Meg3 promotes angiogenesis after ischemic brain injury by activating notch signaling. Mol Neurobiol 2017;54:8179-90. [Crossref] [PubMed]
- Dharap A, Pokrzywa C, Vemuganti R. Increased binding of stroke-induced long non-coding RNAs to the transcriptional corepressors Sin3A and coREST. ASN Neuro 2013;5:283-9. [Crossref] [PubMed]
- Adams HP Jr, Bendixen BH, Kappelle LJ, et al. Classification of subtype of acute ischemic stroke. Definitions for use in a multicenter clinical trial. TOAST. Trial of Org 10172 in acute stroke treatment. Stroke 1993;24:35-41. [Crossref] [PubMed]
- Menon BK, Smith EE, Modi J, et al. Regional leptomeningeal score on CT angiography predicts clinical and imaging outcomes in patients with acute anterior circulation occlusions. AJNR Am J Neuroradiol 2011;32:1640-5. [Crossref] [PubMed]
- Dykstra-Aiello C, Jickling GC, Ander BP, et al. Altered expression of long noncoding RNAs in blood after ischemic stroke and proximity to putative stroke risk loci. Stroke 2016;47:2896-903. [Crossref] [PubMed]
- Saugstad JA. Non-coding RNAs in stroke and neuroprotection. Front Neurol 2015;6:50. [Crossref] [PubMed]
- Christoforidis GA, Mohammad Y, Kehagias D, et al. Angiographic assessment of pial collaterals as a prognostic indicator following intra-arterial thrombolysis for acute ischemic stroke. AJNR Am J Neuroradiol 2005;26:1789-97. [PubMed]
- Miteff F, Levi CR, Bateman GA, et al. The independent predictive utility of computed tomography angiographic collateral status in acute ischaemic stroke. Brain 2009;132:2231-8. [Crossref] [PubMed]
- Yang Y, Cai Y, Wu G, et al. Plasma long non-coding RNA, CoroMarker, a novel biomarker for diagnosis of coronary artery disease. Clin Sci (Lond) 2015;129:675-85. [Crossref] [PubMed]
- de Kok JB, Verhaegh GW, Roelofs RW, et al. DD3(PCA3), a very sensitive and specific marker to detect prostate tumors. Cancer Res 2002;62:2695-8. [PubMed]
- Arita T, Ichikawa D, Konishi H, et al. Circulating long non-coding RNAs in plasma of patients with gastric cancer. Anticancer Res 2013;33:3185-93. [PubMed]
- Xie H, Ma H, Zhou D. Plasma HULC as a promising novel biomarker for the detection of hepatocellular carcinoma. Biomed Res Int 2013;2013:136106. [Crossref] [PubMed]
- Chen ZH, Wang WT, Huang W, et al. The lncRNA HOTAIRM1 regulates the degradation of PML-RARA oncoprotein and myeloid cell differentiation by enhancing the autophagy pathway. Cell Death Differ 2017;24:212-24. [Crossref] [PubMed]
- Zhang J, Yuan L, Zhang X, et al. Altered long non-coding RNA transcriptomic profiles in brain microvascular endothelium after cerebral ischemia. Exp Neurol 2016;277:162-70. [Crossref] [PubMed]
- Ozcan A, Shen SS, Hamilton C, et al. PAX 8 expression in non-neoplastic tissues, primary tumors, and metastatic tumors: a comprehensive immunohistochemical study. Mod Pathol 2011;24:751-64. [Crossref] [PubMed]
- Dupain C, Ali HM, Mouhoub TA, et al. Induction of TTF-1 or PAX-8 expression on proliferation and tumorigenicity in thyroid carcinomas. Int J Oncol 2016;49:1248-58. [Crossref] [PubMed]
- Peat TJ, Edmondson EF, Miller MA, et al. Pax8, napsin A, and CD10 as immunohistochemical markers of canine renal cell carcinoma. Vet Pathol 2017;54:588-94. [Crossref] [PubMed]
- Ghannam-Shahbari D, Jacob E, Kakun RR, et al. PAX8 activates a p53-p21-dependent pro-proliferative effect in high grade serous ovarian carcinoma. Oncogene 2018;37:2213-24. [Crossref] [PubMed]
- Lucci V, Di Palma T, Zannini M. Neuropilin-2 is a newly identified target of PAX8 in thyroid cells. PLoS One 2015;10:e0128315. [Crossref] [PubMed]
- Jubb AM, Sa SM, Ratti N, et al. Neuropilin-2 expression in cancer. Histopathology 2012;61:340-9. [Crossref] [PubMed]
- Klagsbrun M, Eichmann A. A role for axon guidance receptors and ligands in blood vessel development and tumor angiogenesis. Cytokine Growth Factor Rev 2005;16:535-48. [Crossref] [PubMed]
- Eichmann A, Makinen T, Alitalo K. Neural guidance molecules regulate vascular remodeling and vessel navigation. Genes Dev 2005;19:1013-21. [Crossref] [PubMed]
- Lautz T, Lasch M, Borgolte J, et al. Midkine controls arteriogenesis by regulating the bioavailability of vascular endothelial growth factor a and the expression of nitric oxide synthase 1 and 3. EBioMedicine 2018;27:237-46. [Crossref] [PubMed]
- Chalothorn D, Zhang H, Smith JE, et al. Chloride intracellular channel-4 is a determinant of native collateral formation in skeletal muscle and brain. Circ Res 2009;105:89-98. [Crossref] [PubMed]
- Cristofaro B, Shi Y, Faria M, et al. Dll4-Notch signaling determines the formation of native arterial collateral networks and arterial function in mouse ischemia models. Development 2013;140:1720-9. [Crossref] [PubMed]
- Castro PR, Barbosa AS, Pereira JM, et al. Cellular and molecular heterogeneity associated with vessel formation processes. Biomed Res Int 2018;2018:6740408. [Crossref] [PubMed]
- Koch S, Tugues S, Li X, et al. Signal transduction by vascular endothelial growth factor receptors. Biochem J 2011;437:169-83. [Crossref] [PubMed]