Clinical significance of the immune cell landscape in hepatocellular carcinoma patients with different degrees of fibrosis
Introduction
Liver cirrhosis is the 14th leading cause of death in the world and the 10th leading cause in developed countries (1). Being the 2nd most common cancer death in the world, most of the hepatocellular carcinoma (HCC) patients have liver cirrhosis (2).
In hepatitis B virus (HBV)-related cirrhosis, the 5-year cumulative HCC risk is 10% in developed countries. Meanwhile, in hepatitis C virus (HCV)-related cirrhosis, the same risk is 30% in Japan and 17% in developed countries (3,4). Although the anticancer drugs for advanced HCC have progressed quickly, the severity of liver cirrhosis can prevent effective treatment (5-7). Most early-stage HCC patients with cirrhosis are asymptomatic, hard to diagnose, and subsequently progress to advanced-stage with poor survival (8). Cirrhosis is the final stage of chronic liver disease and is associated with the deposition of extracellular matrix proteins, including collagen, in higher-order structures within hepatic parenchyma (9), with hepatic stellate cells and fibroblasts representing major producers of collagen (10). Resolution of liver fibrosis or cirrhosis has been observed in animal models and patients with chronic liver disease of diverse etiologies (11,12). Importantly, regression of fibrosis has also been reported to correlate with improved clinical outcomes (13,14).
Previous studies have been concerned with innate and adaptive immune dysfunction, which play import roles in the pathogenesis of cirrhosis progress in both acute and chronic liver diseases (15). Most research has focused on the mechanism of monocyte and macrophage cells in hepatic fibrogenesis and fibrosis resolution. Macrophage cells can produce proinflammatory and profibrotic mediators, such as the archetypal profibrogenic cytokine TGFβ, which impacts myofibroblasts for activation and synthesizing extracellular matrix (ECM) (16,17). Meanwhile, macrophage cells can also produce other soluble mediators, for example, TNF-α and IL-1β, both of which play key roles in inducing the activity of NF-κB, increasing liver fibrosis and promoting survival through activating hepatic stellate cell-myofibroblast (17).
In adaptive immune response, various subtypes of T cells have distinct functions. TH2 (T helper cells subtype 2) regulates IL-4/5/13/21 and promotes fibrogenesis. In contrast, TH1 is associated with the expression level of IFN-γ and IL-12 and promotes anti-fibrosis (18). Regulatory T cells are also a specific subtype that have been reported as an antifibrotic factor and have been verified in liver disease and animal models (19,20). Previous studies also declared that gamma delta T cells could inhibit hepatic fibrosis by inducing the apoptosis of hepatic stellate cell-myofibroblast (21). All these results suggest that various subtypes of T cells influence fibrosis progression with a complex molecular mechanism.
Other immune cells also have been reported in hepatic fibrosis. For example, natural killer (NK) cells, as an antifibrotic factor, can kill and clear the hepatic stellate cell-myofibroblast and dendritic cells (DCs) involved in ECM degradation during liver fibrosis resolution (22-24). These studies taken together suggest that detecting the dysfunction of innate and adaptive immunity in the occurrence and development of HCC (from cirrhosis, dysplastic nodules, and early HCC to advanced HCC) is necessary for uncovering the potential molecular mechanism.
Newman et al. developed the tool CIBERSORT, which can in silico quantify 22 immune cell types by 547 gene expression profiles from various tissues (25). It is easier and more convenient for identification of immune cell-based prognostic and therapeutic markers after stratification into molecular subtypes. This method has been successfully validated and used in several malignant tumors located in areas like the colon, lung, and breast (26-28). In our studies, CIBERSORT was used for estimating the proportions of different immune cells in normal, cirrhotic, and HCC liver tissues. The significant different adaptive and innate immune cell types were found.
Meanwhile, CIBERSORT was also used in HCC samples with different liver fibrosis degrees in The Cancer Genome Atlas (TCGA). Comparative studies and survival analysis were carried out, and special immune cells significantly related to patient outcome were selected. Finally, potential genes involved in immune cells and associated with patient outcome were identified. These genes may be used as novel checkpoints or targets for immunotherapy and antifibrotic therapy of HCC patients.
Methods
Gene expression profiles
Gene expression was profiled by Affymetrix human genome U133 Plus 2.0 Array [GEO: GPL570] and could be downloaded into the Gene Expression Omnibus (GEO) with the following accession number: GEO:GSE6764 (29,30). These datasets had 10 healthy livers (control), 13 cirrhotic tissues, 17 dysplastic nodules, and 35 HCCs (18 early and 17 advanced). The details are shown in Table S1. The TCGA dataset was downloaded from the UCSC Xena platform (31), and 212 samples with fibrosis Ishak score were selected, including 74 no fibrosis, 31 portal fibrosis, 28 fibrous septa, 9 nodular formation and incomplete cirrhosis, and 70 established cirrhosis samples, in which 209 samples had the information of overall survival (OS) and 182 samples had relapse-free survival (RFS) information (Table S2).
Immune cell profiles by CIBERSORT
CIBERSORT is an analytical tool which accurately quantifies the relative levels of distinct immune cell types within a complex gene expression mixture (25). To characterize and quantify each immune cell subtype, CIBERSORT uses gene expression signatures consisting of 547 genes (LM22 files). In our studies, immune cell profiles were calculated for each sample from GEO and TCGA, and mean values for each tissue type were calculated. Kruskal-Wallis test was applied to analyze the differences between various tissues.
Total macrophage fraction was calculated as a sum of M0, M1, and M2 macrophage fractions. Total T cells were calculated as a sum of CD8+ T cells, CD4+ naïve T cells, CD4+ memory resting T cells, CD4+ memory-activated T cells, follicular helper T cells, regulatory T cells (Tregs), and T cell gamma delta fractions. Total B cells were calculated as a sum of B cell naïve T cells and B memory cells.
Differentially expressed genes
Differentially expressed genes (DEGs) in GSE6764 were identified between different liver tissues with the threshold of absolute log2-fold-change >1 and an adjusted P value <0.05 with R package “limma”.
Survival analysis
In survival analysis, Cox proportional hazards (COXPH) model is the most commonly used. In our studies, COXPH was carried out to assess whether proportions of immune cells from CIBERSORT and related gene expression levels were associated with patient outcome. We assumed that there were n samples (i=1, 2, …, n) in the expression profiles, and the ith sample’s survival time was ti; at the same time, the sample had a set of candidate genes Xi1, Xi2, Xi3, and Xip. The model was as follows:
h(t, X) represents the risk function related to hazard function at time t. h0(t) represents the baseline hazard function. p represents the counts of influencing factors. X represents the state vector of the influencing factors. β represents the coefficients’ vector. β1ξ1+β2ξ2+…+βπξπ represents the prognostic index. The COXPH analysis was performed using the “Survival” R package with the “coxph” function.
Proportions of immune cells from CIBERSORT and expression values of genes consistently identified were discretized into high and low categories based on median values. Kaplan-Meier survival analysis and log-rank test were used. All statistics were calculated by R language (Version 3.5.2).
Results
Patient characteristics
Gene expression profiles of 75 samples (10 normal tissues, 13 cirrhotic tissues, 17 dysplastic nodules, 17 early HCCs, and 18 advanced HCCs) were downloaded from GEO (GEO Accession: GSE6764) and analyzed in this study (30). Additionally, gene expression profiles of 212 HCC samples staged with the Ishak fibrosis score (ranging from 0= no fibrosis to 6= cirrhosis) were selected from TCGA for further analysis, among which 209 (or 182) samples had clinical outcome defined as OS (or RFS). Detailed patient characteristics are listed in Tables S1,S2. The dataset selection scheme and workflow of studies are shown in Figure 1.
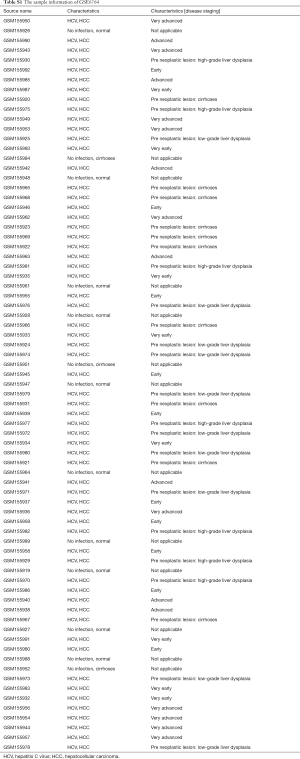
Full table
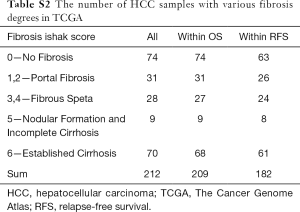
Full table
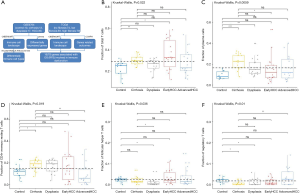
Different proportions of adaptive and innate immune cells types in normal, cirrhotic, and tumor tissues
Firstly, the proportions of different immune cells of 75 samples in GSE6764 were estimated based on the LM22 signature files by CIBERSORT and the results were shown in Figure 2. Then the comparative studies were carried out for revealing the differences between various liver tissues (Tables S3,S4).
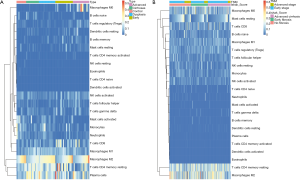
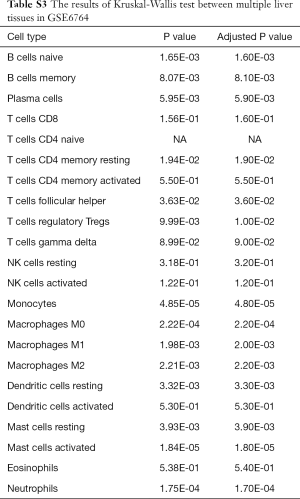
Full table
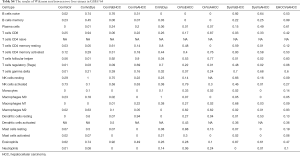
Full table
For adaptive immune cells, the total T cells (P=2.17E-2, Figure 1B) and plasma cells (P=5.95E-3, Figure 1C) were significantly different, and the total B cells were not significantly (P=2.56E-1, Figure 2A) altered between various liver tissues. There were 5 main T cell subpopulations with higher fractions, in which 3 subgroups (CD4 resting memory (Figure 1D), follicular helper (Figure 1E), and regulatory Tregs (Figure 1F) were significantly altered, while the other 2 subgroups (CD8 and gamma delta) were not significantly altered between the different live tissues (Figure S1A,S1B). The fraction of CD4 resting memory and gamma delta T cells were increased in cirrhosis and dysplasia tissues and then decreased in HCC (especially in advanced HCC). In contrast, the Tregs and follicular helper T cells were down-regulated in cirrhosis and dysplasia tissues and then up-regulated in HCC (especially in advanced HCC). The correlation test suggested that follicular helper was significantly positively associated with CD8 (R=0.68) and gamma delta (R=0.53), and negatively associated with CD4 resting memory (R=−0.55).
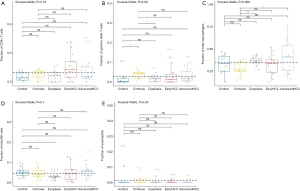
The plasma cells were increased in cirrhosis (22.28%±7.56%), dysplasia (17.31%±8.58%), and HCC (15.65%±11.98%) when compared to healthy liver (9.41%±3.83%). Although the total of B cells was not significantly altered between different liver tissues (Figure 3A), the fraction of B cell naïve (or B cell memory) in cirrhosis tissues was significantly higher (or lower) than dysplasia, and early and advanced HCC (Figure 3B,C).
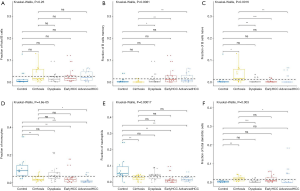
For innate immune cells, the results of Kruskal-Wallis tests suggested that monocytes (P=4.85E-5, Figure 3D), neutrophils (P=1.75E-4, Figure 3E), dendritic cells (P=2.96E-3, Figure 3F) and the total mast cells (P=1.18E-3, Figure 4A) were significantly altered. Meanwhile, macrophages, the total NK cells, and eosinophils were not significantly altered (Figure S1C,S1D,S1E). The fractions of monocytes and neutrophils were lower in cirrhosis and HCC than in the healthy liver, and the correlation test suggested that they were highly related to each other (R=0.82). For mast cells, activated subtypes were higher in healthy liver, whereas resting subtypes were higher in advanced HCC (Figure 4B). For dendritic cells, the results suggested that resting subtypes were significantly increased in cirrhosis when compared to healthy liver tissues and then decreased in advanced HCC (Figure 4C). The main polarized macrophage subtypes, named M1 and M2, significantly changed between different liver tissues. Furthermore, M1 was decreased, and M2 was increased in cirrhosis in comparison to the normal liver tissues (Figure 4D,E).
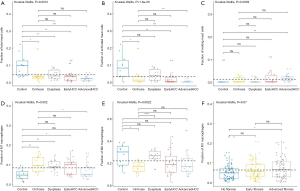
Different proportions of immune cells types in HCCs with different fibrosis degrees
To further study whether the degree of liver fibrosis could have an impact on the fraction of immune cell type, the gene profiles of 212 samples with a fibrosis Ishak score (ranging from 0 to 6) were downloaded from the UCSC Xena platform (31). Similarly, the samples were divided into 3 groups with different fibrosis Ishak scores (no fibrosis: 0, early fibrosis: 1–4, advanced fibrosis: 5–6) and the proportions of different immune cells were estimated (Figure 2B) and comparative studies were carried out (Tables S5,S6).
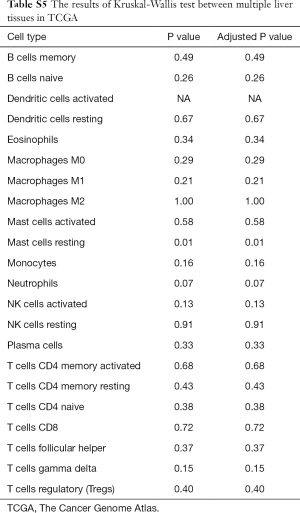
Full table
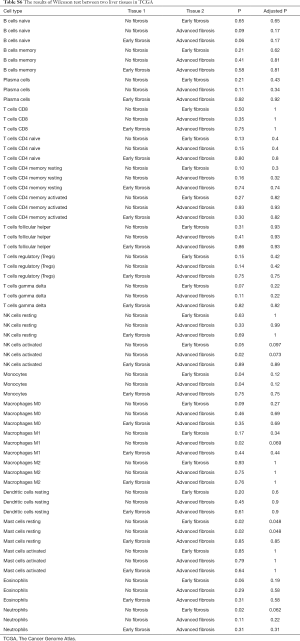
Full table
For adaptive immune cells, the subtypes of T cells, B cells, and plasma cells were not significantly different in each group of HCC samples. For innate immune cells, comparative studies illustrated that the polarized macrophage M1 subtype increased with an increase of fibrosis degree (no fibrosis vs. advanced fibrosis, P=2.32E-2, Figure 4F). In contrast, the fractions of some immune cell subtypes were significantly decreased with an increase of fibrosis degree, such as resting mast cells (P=2.30E-2 Figure 5A), monocytes (P=5.80E-2, Figure 5B), neutrophils (P=5.00E-2, Figure 5C), and activated NK cells (P=4.6E-2, Figure 5D). Resting mast cell number in HCC patients without fibrosis was significantly higher than that in other HCC patients with early fibrosis (P=2.13E-2) and advanced fibrosis (P=1.60E-2). Meanwhile, monocytes in HCC patients without fibrosis were significantly higher than the other HCC patients with early fibrosis (P=4.36E-2) and advanced fibrosis (P=4.02E-2). Neutrophils in HCC patients without fibrosis were significantly higher than early fibrosis HCC patients (P=2.06E-2). The results of activated NK cells were also similar, and HCC without fibrosis had a higher number than early fibrosis HCC patients (P=2.44E-2) and advanced fibrosis HCC patients (P=4.87E-2).
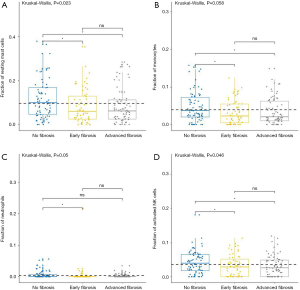
Association between immune genes and OS (RFS) by TCGA datasets
As previously mentioned, the analysis of comparative studies in microarray datasets and TCGA both revealed that significant differences in immune cell composition existed not only in healthy livers vs. cirrhosis and cirrhosis vs. HCC, but also in types of HCC with different degrees of fibrosis. Previous studies have reported that immune cell migration and/or retention in tumors can impact patients’ overall survival and/or recurrence-free survival. Therefore, our studies hypothesized that genes involved in those immune cells could be significantly associated with OS and RFS.
For revealing the molecular mechanism of the different fraction of immune cells and identifying candidate genes, we first re-analyzed the microarray datasets. Differentially expressed genes (DEGs) were identified between different liver tissues with the threshold of absolute log2-fold-change >1 and adjusted P value <0.05 (Table 1). In different DEG groups (control vs. cirrhosis and cirrhosis vs. HCC), the enrichment results of top 20 Gene Ontology BP (biological process) were shown in Figure 6, respectively. With the development of the disease, there were apparent significant dysfunction of immune system in both DEG groups, including immune response and inflammatory response. The enrichment results of KEGG pathways were shown in Figure S2, the abnormal pathways related immune and cirrhosis were also found, such as ECM-receptor interaction, Toll-like receptor signaling pathway and TGF-beta signaling pathway. These pathway analysis results were consistent with the Wurmbach et al. who published the GSE6764 (30). They also suggested dysregulation of the Notch and Toll-like receptor pathway in early carcinogenesis.
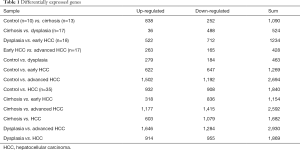
Full table
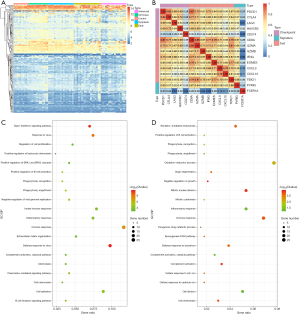
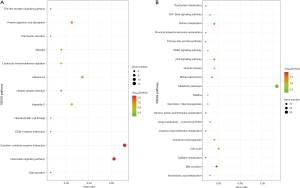
There were 189 DEGs found in the different DEG groups which were also included in the CIBERSORT gene signatures (http://fp.amegroups.cn/cms/8c16a193b0b926dc420fa7bd4e8a85c6/atm.2019.09.122-1.pdf) and the heatmap was shown in Figure 6A. Secondly, COXPH analysis was carried out on the fractions of immune cell types. Thirdly, COXPH analysis was carried out on the TCGA datasets to detect whether those DEGs were significantly associated (P<0.05) with OS and/or RFS. There were 16 (or 79) DEGs significantly associated with OS (or RFS) (Tables S7,S8).
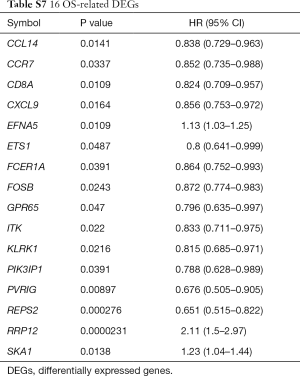
Full table

Full table
For example, the fraction of resting NK cells were found not only to be significantly related with OS (P=3.23E-2, HR >1) but also significantly related with RFS (P=3.93E-2, HR >1). Finally, the gene PVRIG (PVR related immunoglobulin domain-containing, also named CD112R) involving NK cell testing was found (32). COXPH analysis found that PVRIG was significantly associated with OS [P=8.97E-3, HR =0.676 (0.505–0.905)] and RFS [P=5.50E-3, HR=0.694 (0.505–0.898)]. As shown in in Figure 7A,B, Kaplan-Meier analyses were performed, and log-rank test suggested PVRIG was significantly related by positive association with OS (log-rank P=3.90E-2) and RFS (log-rank P=1.70E-2). Many cancers failed to respond due to their resistance to NK cell-triggered antibody-dependent cellular cytotoxicity (ADCC). PVRIG is known to be important for lymphocyte functions, and PVR-like receptors have been found to be expressed in NK cells (32). Trastuzumab is the first-line drug in treating breast cancer with high Her2 expression, and previous studies have proven that blockade of TIGIT or PVRIG, separately or together, could enhance trastuzumab-triggered antitumor response by human NK cells for improving trastuzumab therapy for breast cancer (32). Furthermore, the values of Pearson correlation coefficient between PVRIG and other checkpoint molecules (PDCD1, CTLA4, LAG3, HAVCR2, CD274) and Teff (effector T-cell) gene signatures (33) were calculated. In Figure 6B, the results suggested that PVRIG is significantly positively related to other immune genes (correlation coefficients: 0.35–0.67).
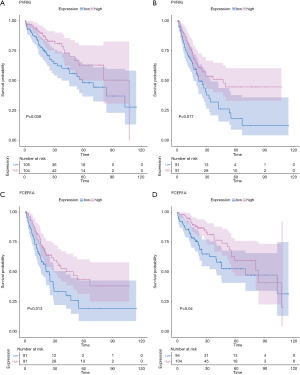
In innate immune cells, the monocytes, neutrophils, total mast cells, and dendritic cells are significantly altered. Mast cells play key roles in immune regulation, and the mechanism behind mast cell inactivation in HCC is still unknown. Mast cell activator IgE has been observed in HBV-associated HCC (34). Our results also found the fraction of active mast cells to be higher in healthy liver, whereas the resting subtype was higher in advanced HCC. COXPH analysis also found that the fraction of resting mast cells was significantly associated with patients’ outcomes. FCER1A (Fc fragment of IgE receptor Ia), involving the activated and resting mast cells, was significantly associated with HCC patients’ overall survival [P=4.01E-2, HR =0.864 (0.752–0.993)] and relapse-free survival [P=1.28E-2, HR =0.864 (0.752–0.993)]. The results of the log-rank test and Kaplan-Meier analyses are shown in Figure 7C,D, respectively. Mast cells are among the fastest immune cell responders, and are especially abundant at barrier sites. They rapidly release preformed mediators, such as vasoactive amines, proteoglycans, proteases, and cytokines from intracellular stores upon the crosslinking of FCER1-bound IgE. In Figure 6B, the results suggested that FCER1A is less significantly positively related to other immune genes than PVRIG. The function of mast cells and FCER1A in aberrant liver is still unclear, and more studies are needed.
Discussion and conclusions
The major causes of morbidity and mortality of patients with chronic liver disease are liver fibrosis and cirrhosis. With chronic inflammation and intra-hepatic immunosuppressive microenvironment, liver fibrosis contributes to hepatocellular carcinoma. Previous studies have found immune gene profiles are consistently down-regulated during HCC progression, which leads to tumor immunity with a lower level, especially in advanced HCC. Okrah et al. suggested that the fibrotic liver state makes a barrier by collagens and ECM proteins and then prevents CD8+ intra-tumor infiltration, which favors tumor progression (35). Further, Okrah et al. found that administration of α-TGF-β appeared to improve the fibrotic environment of STAM™ model of murine HCC and enhance distribution of CD8+ T cells (35). All of this research has suggested that uncovering the different immune cell fractions of aberrant liver, revealing the potential molecular mechanism, and identifying key biomarkers are necessary, as they may lead to finding possible novel ways to improve immunotherapy efficacy by disrupting the function of collagen and ECM proteins and promoting immune infiltration.
In this manuscript, CIBERSORT was applied to assess differential immune cell fractions in various kinds of liver tissues (from healthy, cirrhotic, dysplastic nodules to HCC). In adaptive immune cells, the total of T cells plays a central role and is significantly altered. Among subtypes of T cells, the fraction of CD4 memory resting and gamma delta T cells were increased in cirrhosis and dysplasia tissues and then decreased in HCC. In contrast, Tregs and follicular helper T cells were down-regulated in cirrhosis and dysplasia tissues and then up-regulated in HCC. Although there was no significant difference found between HCC samples with different degrees of fibrosis, T cell activation was involved in all stages of the T cell response. Our workflow suggested PRIVG was significantly associated with survival. Previous studies have reported PRIVG as a novel checkpoint for T cells. PRIVG was preferentially expressed in T cells and inhibited T cell receptor-mediated signals. It is known to compete with CD226 to bind to CD112, disrupting the PRIVG-CD112 interaction that enhances T cell response (36). Another 2 studies declared that PVRIG is an inducible checkpoint receptor and that targeting PVRIG-PVRL2 interactions results in increased CD8+ T-cell function and reduced tumor growth (37).
Alternative treatments, including immunotherapies, are necessary and urgent for HCC. Preliminary clinical trials with immune checkpoint inhibitors show great potential in HCC as first and second-line treatment (38). Although checkpoint inhibitors that block CTLA-4 and PD-1 have improved cancer immunotherapies, targeting additional checkpoint receptors may be required to broaden patient response to immunotherapy. Meanwhile, the antifibrotic agents to liver fibrosis have advanced in development. Several novel agents focusing on special molecular targets involved in fibrosis progression have entered the clinical stage (39). Combination chemotherapy has been evaluated in several pre-clinical settings and a handful of clinical trials (40). The combination of immunotherapy with antifibrotic therapy may treat HCC patients, especially with advanced fibrosis and cirrhosis.
Our research attempted to study the variant immune cell fractions in cirrhosis and HCC patients with variant fibrosis degrees and to identify the key biomarkers, but naturally, there were some limitations. Firstly, in the microarray datasets, the patient sample size, especially the cirrhosis samples were not big, and the fibrosis degree of the HCC samples was not clear. Secondly, the TCGA datasets only had the tumor samples, and the paired adjacent tissues were lacking. Thirdly, more method and immune-related genes could be used for estimating the fraction and activation of variant types of immune cells. All these shortcomings disturb the study’s robustness and could be improved in future studies.
Acknowledgments
Funding: This work was supported by the Zhejiang Natural Science Foundation (grant number LY16H160021), the Public Welfare Technology and Social Development Project of Zhejiang Provincial Bureau of Science and Technology (grant number 2017C33069), the Public Welfare Technology of Zhejiang Provincial Bureau of Science and Technology (grant number LGF19H030017).
Footnote
Conflicts of Interest: The authors have no conflicts of interest to declare.
Ethical Statement: The authors are accountable for all aspects of the work in ensuring that questions related to the accuracy or integrity of any part of the work are appropriately investigated and resolved.
References
- Tsochatzis EA, Bosch J, Burroughs AK. Liver cirrhosis. Lancet 2014;383:1749-61. [Crossref] [PubMed]
- Takayama T, Makuuchi M. Segmental liver resections, present and future-caudate lobe resection for liver tumors. Hepatogastroenterology 1998;45:20-3. [PubMed]
- Kanda T, Takahashi K, Nakamura M, et al. Androgen Receptor Could Be a Potential Therapeutic Target in Patients with Advanced Hepatocellular Carcinoma. Cancers (Basel) 2017;9. [Crossref] [PubMed]
- Kanda T, Goto T, Hirotsu Y, et al. Molecular Mechanisms Driving Progression of Liver Cirrhosis towards Hepatocellular Carcinoma in Chronic Hepatitis B and C Infections: A Review. Int J Mol Sci 2019.20. [PubMed]
- Bruix J, Qin S, Merle P, et al. Regorafenib for patients with hepatocellular carcinoma who progressed on sorafenib treatment (RESORCE): a randomised, double-blind, placebo-controlled, phase 3 trial. Lancet 2017;389:56-66. [Crossref] [PubMed]
- Schachter J, Ribas A, Long GV, et al. Pembrolizumab versus ipilimumab for advanced melanoma: final overall survival results of a multicentre, randomised, open-label phase 3 study (KEYNOTE-006). Lancet 2017;390:1853-62. [Crossref] [PubMed]
- Kudo M, Finn RS, Qin S, et al. Lenvatinib versus sorafenib in first-line treatment of patients with unresectable hepatocellular carcinoma: a randomised phase 3 non-inferiority trial. Lancet 2018;391:1163-73. [Crossref] [PubMed]
- Bruix J, Reig M, Sherman M. Evidence-Based Diagnosis, Staging, and Treatment of Patients With Hepatocellular Carcinoma. Gastroenterology 2016;150:835-53. [Crossref] [PubMed]
- Bataller R, Brenner DA. Liver fibrosis. J Clin Invest 2005;115:209-18. [Crossref] [PubMed]
- Xu L, Hui AY, Albanis E, et al. Human hepatic stellate cell lines, LX-1 and LX-2: new tools for analysis of hepatic fibrosis. Gut 2005;54:142-51. [Crossref] [PubMed]
- Issa R, Williams E, Trim N, et al. Apoptosis of hepatic stellate cells: involvement in resolution of biliary fibrosis and regulation by soluble growth factors. Gut 2001;48:548-57. [Crossref] [PubMed]
- Ellis EL, Mann DA. Clinical evidence for the regression of liver fibrosis. J Hepatol 2012;56:1171-80. [Crossref] [PubMed]
- Marcellin P, Gane E, Buti M, et al. Regression of cirrhosis during treatment with tenofovir disoproxil fumarate for chronic hepatitis B: a 5-year open-label follow-up study. Lancet 2013;381:468-75. [Crossref] [PubMed]
- Shiffman ML, Sterling RK, Contos M, et al. Long term changes in liver histology following treatment of chronic hepatitis C virus. Ann Hepatol 2014;13:340-9. [Crossref] [PubMed]
- Irvine KM, Ratnasekera I, Powell EE, et al. Causes and Consequences of Innate Immune Dysfunction in Cirrhosis. Front Immunol 2019;10:293. [Crossref] [PubMed]
- Karlmark KR, Weiskirchen R, Zimmermann HW, et al. Hepatic recruitment of the inflammatory Gr1+ monocyte subset upon liver injury promotes hepatic fibrosis. Hepatology 2009;50:261-74. [Crossref] [PubMed]
- Wynn TA, Barron L. Macrophages: master regulators of inflammation and fibrosis. Semin Liver Dis 2010;30:245-57. [Crossref] [PubMed]
- Wynn TA. Cellular and molecular mechanisms of fibrosis. J Pathol 2008;214:199-210. [Crossref] [PubMed]
- Katz SC, Ryan K, Ahmed N, et al. Obstructive jaundice expands intrahepatic regulatory T cells, which impair liver T lymphocyte function but modulate liver cholestasis and fibrosis. J Immunol 2011;187:1150-6. [Crossref] [PubMed]
- Claassen MA, de Knegt RJ, Tilanus HW, et al. Abundant numbers of regulatory T cells localize to the liver of chronic hepatitis C infected patients and limit the extent of fibrosis. J Hepatol 2010;52:315-21. [Crossref] [PubMed]
- Hammerich L, Bangen JM, Govaere O, et al. Chemokine receptor CCR6-dependent accumulation of gammadelta T cells in injured liver restricts hepatic inflammation and fibrosis. Hepatology 2014;59:630-42. [Crossref] [PubMed]
- Radaeva S, Sun R, Jaruga B, et al. Natural killer cells ameliorate liver fibrosis by killing activated stellate cells in NKG2D-dependent and tumor necrosis factor-related apoptosis-inducing ligand-dependent manners. Gastroenterology 2006;130:435-52. [Crossref] [PubMed]
- Glassner A, Eisenhardt M, Kramer B, et al. NK cells from HCV-infected patients effectively induce apoptosis of activated primary human hepatic stellate cells in a TRAIL-, FasL- and NKG2D-dependent manner. Lab Invest 2012;92:967-77. [Crossref] [PubMed]
- Jiao J, Sastre D, Fiel MI, et al. Dendritic cell regulation of carbon tetrachloride-induced murine liver fibrosis regression. Hepatology 2012;55:244-55. [Crossref] [PubMed]
- Newman AM, Liu CL, Green MR, et al. Robust enumeration of cell subsets from tissue expression profiles. Nat Methods 2015;12:453-7. [Crossref] [PubMed]
- Zhou R, Zhang J, Zeng D, et al. Immune cell infiltration as a biomarker for the diagnosis and prognosis of stage I-III colon cancer. Cancer Immunol Immunother 2019;68:433-42. [Crossref] [PubMed]
- Ali HR, Chlon L, Pharoah PD, et al. Patterns of Immune Infiltration in Breast Cancer and Their Clinical Implications: A Gene-Expression-Based Retrospective Study. PLoS Med 2016;13:e1002194. [Crossref] [PubMed]
- Liu YZ, Saito S, Morris GF, et al. Proportions of resting memory T cells and monocytes in blood have prognostic significance in idiopathic pulmonary fibrosis. Genomics 2018. [Epub ahead of print]. [Crossref] [PubMed]
- Edgar R, Domrachev M, Lash AE. Gene Expression Omnibus: NCBI gene expression and hybridization array data repository. Nucleic Acids Res 2002;30:207-10. [Crossref] [PubMed]
- Wurmbach E, Chen YB, Khitrov G, et al. Genome-wide molecular profiles of HCV-induced dysplasia and hepatocellular carcinoma. Hepatology 2007;45:938-47. [Crossref] [PubMed]
- Goldman M, Craft B, Hastie M, et al. The UCSC Xena platform for public and private cancer genomics data visualization and interpretation. bioRxiv 2018.326470.
- Xu F, Sunderland A, Zhou Y, et al. Blockade of CD112R and TIGIT signaling sensitizes human natural killer cell functions. Cancer Immunol Immunother 2017;66:1367-75. [Crossref] [PubMed]
- Socinski MA, Jotte RM, Cappuzzo F, et al. Atezolizumab for First-Line Treatment of Metastatic Nonsquamous NSCLC. N Engl J Med 2018;378:2288-301. [Crossref] [PubMed]
- Li N, Zhu Q, Li Z, et al. IL17A gene polymorphisms, serum IL-17A and IgE levels, and hepatocellular carcinoma risk in patients with chronic hepatitis B virus infection. Mol Carcinog 2014;53:447-57. [Crossref] [PubMed]
- Okrah K, Tarighat S, Liu B, et al. Transcriptomic analysis of hepatocellular carcinoma reveals molecular features of disease progression and tumor immune biology. NPJ Precis Oncol 2018;2:25. [Crossref] [PubMed]
- Zhu Y, Paniccia A, Schulick AC, et al. Identification of CD112R as a novel checkpoint for human T cells. J Exp Med 2016;213:167-76. [Crossref] [PubMed]
- Whelan S, Ophir E, Kotturi MF, et al. PVRIG and PVRL2 Are Induced in Cancer and Inhibit CD8(+) T-cell Function. Cancer Immunol Res 2019;7:257-68. [Crossref] [PubMed]
- Buonaguro L, Mauriello A, Cavalluzzo B, et al. Immunotherapy in hepatocellular carcinoma. Ann Hepatol 2019;18:291-7. [Crossref] [PubMed]
- Schuppan D, Ashfaq-Khan M, Yang AT, et al. Liver fibrosis: Direct antifibrotic agents and targeted therapies. Matrix Biol 2018;68-69:435-51. [Crossref] [PubMed]
- Zhu J, Yin T, Xu Y, et al. Therapeutics for advanced hepatocellular carcinoma: Recent advances, current dilemma, and future directions. J Cell Physiol 2019;234:12122-32. [Crossref] [PubMed]