Quantitative feature analysis of CT images of transbronchial dye markings mimicking true pulmonary ground-glass opacity lesions
Introduction
The use of low-dose computed tomography (CT) screening is increasing, thus resulting in an increase in the detection rate of small lung nodules; most of these lesions are indeterminate (1,2). Biopsy via the transbronchial or transthoracic approach has remained challenging, especially for lesions smaller than 2 cm with a predominately ground-glass opacity (GGO) component (3,4). Therefore, patients with screened lung nodules and suspicious malignancy are often suggested surgical excision for a definite diagnosis and curative treatment. To identify those small pulmonary nodules under a minimally invasive surgical approach, preoperative localization is crucial to guide adequate resection (5). Bronchoscopic dye marking had been developed as a standardized system for image-guided sublobar lung resections (6-9), and the post-marking CT confirmation of actual marking locations is essential for accurate surgical performance (10).
The typical appearance of sprayed dye on CT is ground-glass opacities, with or without bronchial dilation, which mimics the actual ground-glass lesion. Through the quantitative analysis, image textures of the ground-glass lesions can be extracted from the segmented region of interest (11). Our aim is to analyze the marking dyes in both multi-detector computed tomography (MDCT) and cone beam computed tomography (CBCT) using a radiomic approach, and provide the basic radiological information on the dye marking.
Methods
The institutional review board of National Taiwan University Hospital, Hsin-Chu Branch, approved the retrospective retrieval of data for this study (approval number 107-090-E). The patients who underwent preoperative bronchoscopy-guided dye localization before thoracoscopic pulmonary resection, were retrospectively analyzed. The images of thin-cut CT performed after bronchoscopic dye marking were obtained for quantitative feature extraction.
Image acquisition
To identify the bronchoscopic dye and ground glass nodule (GGN) lesions using MDCT scanners manufactured by GE and Philips, the following MDCT parameters were included: pixel spacing 0.4–0.6 mm; slice thickness: 0.625–1.25 mm; general parameters: 120 kVp, and 50–600 mA. Furthermore, for bronchoscopic dye analysis of images obtained using a CBCT scanner manufactured by Siemens, the following parameters were used: pixel spacing 0.248 mm; slice thickness: 0.469 mm; general parameters: 90–97 kVp and 153–277 mA.
Segmentation and intensity component extraction
Segmentation of bronchoscopic dyes and GGNs is a difficult task due to fuzzy boundaries and complex surrounding structures, including vessels, bronchi, and other tissues in the CT images. To overcome these challenges and extract features from targets, a segmentation algorithm was proposed to describe the boundary and separate complex adjacent tissues from the targets. The target edges were preliminarily delineated using the level set method (12). To achieve good separation of surrounding tissues from targets, we adopted the Frangi vesselness algorithm (13) to enhance the plate-like structures and tubular structures. The outcome excluded adjacent tissue components from targets. The final segmentation results were verified and modified to ensure correctness of the target boundaries by the thoracic specialists (SM Yang) (Figure 1).
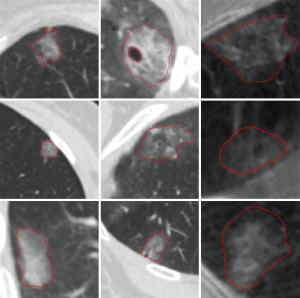
Feature extraction
In order to quantitatively analyze the differences between the bronchoscopic dyes and ground glass nodules, we utilized the gray-level co-occurrence matrix (GLCM) (14) to extract texture features from segmented targets in the CT images. The quantified GLCM features include auto correlation (illustrates fineness and roughness of the texture), contrast (calculates adjacent pixel intensity contrast, which represents gray level variations), correlation (calculates the direction of similarity in each column or row), energy (portrays homogeneity of the distribution in GLCM and texture thickness), entropy (reflects the state of chaos in gray level distribution), and homogeneity (measures compactness of distribution of each element). Statistical analyses of GLCM features were conducted to help identify the difference between bronchoscopic dye and GGN lesions on MDCT, and also compare bronchoscopic dye images from MDCT and CBCT image modalities. Levene’s test was applied prior to the Student’s t-test to evaluate the equality of variances. Once the Levene’s test represented negativity (P<0.05, homoscedasticity), the Students t-test with equal variance was adopted; conversely, when Levene’s test represented positivity (P<0.05, heteroscedasticity), the Student’s t-test with unequal variance was adopted. Statistical analyses were implemented using MATLAB version 2018a (Mathworks, Natick, Mass). A P value<0.05 indicated statistical significance.
Results
From March 2017 to September 2018, bronchoscopic dye markings (n=13) and ground glass nodules (GGNs, n=13) were used to distinguish the differences in the texture features between MDCT images. The CBCT images were chosen for the images with bronchoscopic dyes (n=15) to compare the consistency of texture features using MDCT.
We utilized the student’s t-test for GLCM feature recognition of images with bronchoscopic dye and GGN lesions using MDCT. The statistical results (Table 1) revealed that the features of auto correlation, contrast, energy, entropy, and homogeneity had significant differences. Figure 2 demonstrates the energy features and entropy features; interquartile ranges could be separated from bronchoscopic dye and GGN lesions in MDCT. In contrast with energy and entropy features, the correlation feature could not identify bronchoscopic dye and GGN lesions in MDCT due to their overlap in the interquartile ranges. As for the discrimination of GLCM features of bronchoscopic dye from MDCT and CBCT (Table 2), the contrast, correlation, energy, entropy, and homogeneity features were significantly different (Figure 3). Apart from the auto correlation features, interquartile ranges of most of the features could separate bronchoscopic dye from MDCT and CBCT.
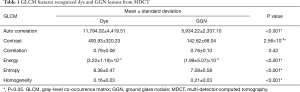
Full table
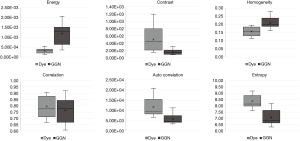
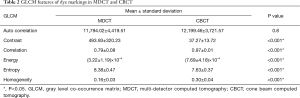
Full table
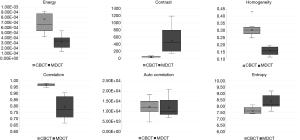
Discussion
Bronchoscopic dye marking has been getting popular for its usefulness in localization, with multiple markings for various procedures of pulmonary resection, with less complications of pneumothorax and intrapulmonary hemorrhage (15). To ensure proper results with localization contributing to navigational resection, post-marking CT was selectively performed to confirm the accurate locations of the dye markings. Additionally, discrepancies between the original design by the virtual bronchoscopic mapping system and actual markings were observed (10); therefore, post-mapping CT confirmation is recommended to provide precise spatial information. On identifying the viable dye marking on CT, GGO appearance was searched for in the anticipated bronchial territory, especially near the pleura, and the CT image before dye marking was viewed as reference to exclude the previously existing findings. However, identifying the dye markings on CT was sometimes difficult, and the focal atelectasis change due to gravitational effect and inadequate air injection also interfered with the interpretation of post-mapping CT. According to a previous study on lung mapping, up to 20% of the marking dye was “not identifiable” or “difficult to identify” (7), furthermore, over 40% of unidentifiable markings were still found intraoperatively (7), which suggest that the visual detection of dye marking on CT is sometimes unreliable and there is still room for improvement.
Although the most sensitive window setting of CT for detecting fluid in air-containing space, which represents the status of bronchial lavage and dye spray, was window level (WL): −1,000 HU and window width (WW): 350 HU, the dye markings were usually observed as a ground-glass pattern in the setting of lung window (WL: −500 HU, WW: 1,500 HU). Before the use of an optimal window setting for the detection of dye marking had been validated, the quantitative texture analysis contributed to the global evaluation of the image with dye markings, without the limitations of a window setting, and it also provides basic radiomic information on the bronchoscopically sprayed dye markings. The problem in differentiation between true GGN lesions and dye markings was usually solved by correlating with the locations of the GGN lesions on the previous CT images. Besides, our results demonstrated that quantitative analysis provides discriminative information between the CT images of true GGN lesions and dye markings (Figure 3). In addition, the quantitative image data can be further used to develop an automatic detection system for both lesion and dye in the selected lung field without the necessity to adjust the window level.
Image-guided interventions have been frequently performed under CBCT in the recent years. CBCT can provide real-time fluoroscopic visualization during bronchoscopic lung marking, and provides three-dimensional images for CT confirmation. Although there was an increased probability of noise and motion artifacts on CBCT, in our current experience, most of the marking dye was recognizable in CBCT images with a typical GGO pattern. Additionally, the quantitative features measured on CBCT images are partly reproducible in MDCT (16). Our results also demonstrated discrepancies in the feature values of dye images on MDCT and CBCT, which suggest that the algorithm of auto-detection for the dye image should be separately constructed for different CT image acquisition systems.
There are several limitations in this study. First, this is a single-centered, retrospective study with a small sample size. Second, the images of bronchoscopic dye marking included in quantitative analysis are all visually identifiable with a typical GGO pattern. Besides, the comparative group of true GGN lesions have a pure-GGO appearance on the MDCT image, which spare the diversity in the markings and lesions, especially in those that were difficult to identify. Third, the thoracoscopic finding of the dye markings was not included in this study, otherwise, the small group of radiologically unidentifiable markings that were actually visible under thoracoscopy could have been analyzed.
In conclusion, the initial results of quantitative analysis of dye marking images demonstrated the discriminative variance in several, commonly used texture features, compared to those of true GGN lesions, and the image textures of dye markings on MDCT and CBCT also presented with obvious discrepancies. Further investigations and more image data for validation are needed to clarify the applicability of this technique in clinical practice.
Acknowledgements
Funding: This study was funded by the Ministry of Science and Technology, Taiwan (107-2221-E-002-080-MY3).
Footnote
Conflicts of Interest: The authors have no conflicts of interest to declare.
Ethical Statement: The institutional review board of National Taiwan University Hospital, Hsin-Chu Branch, approved the retrospective retrieval of data for this study (approval number 107-090-E).
References
- Krochmal R, Arias S, Yarmus L, et al. Diagnosis and management of pulmonary nodules. Expert Rev Respir Med 2014;8:677-91. [Crossref] [PubMed]
- Raad RA, Suh J, Harari S, et al. Nodule characterization: subsolid nodules. Radiol Clin North Am 2014;52:47-67. [Crossref] [PubMed]
- Shimizu K, Ikeda N, Tsuboi M, et al. Percutaneous CT-guided fine needle aspiration for lung cancer smaller than 2 cm and revealed by ground-glass opacity at CT. Lung Cancer 2006;51:173-9. [Crossref] [PubMed]
- Yeow KM, Tsay PK, Cheung YC, et al. Factors affecting diagnostic accuracy of CT-guided coaxial cutting needle lung biopsy: retrospective analysis of 631 procedures. J Vasc Interv Radiol 2003;14:581-8. [Crossref] [PubMed]
- Yang SM, Hsu HH, Chen JS. Recent advances in surgical management of early lung cancer. J Formos Med Assoc 2017;116:917-23. [Crossref] [PubMed]
- Sato M, Omasa M, Chen F, et al. Use of virtual assisted lung mapping (VAL-MAP), a bronchoscopic multispot dye-marking technique using virtual images, for precise navigation of thoracoscopic sublobar lung resection. J Thorac Cardiovasc Surg 2014;147:1813-9. [Crossref] [PubMed]
- Sato M, Yamada T, Menju T, et al. Virtual-assisted lung map-ping: outcome of 100 consecutive cases in a single institute. Eur J Cardiothorac Surg 2015;47:131-9. [Crossref] [PubMed]
- Sato M, Murayama T, Nakajima J. Techniques of stapler-based navigational thoracoscopic segmentectomy using virtual assisted lung mapping (VAL-MAP). J Thorac Dis 2016;8:S716-30. [Crossref] [PubMed]
- Sato M, Kuwata T, Yamanashi K, et al. Safety and reproducibility of virtual-assisted lung mapping: a multicentre study in Japan. Eur J Cardiothorac Surg 2017;51:861-8. [PubMed]
- Sato M, Nagayama K, Kuwano H, et al. Role of post-mapping computed tomography in virtual-assisted lung mapping. Asian Cardiovasc Thorac Ann 2017;25:123-30. [Crossref] [PubMed]
- Bak SH, Lee HY, Kim JH, et al. Quantitative CT scanning analysis of pure ground-glass opacity nodules predicts further CT scanning change. Chest 2016;149:180-91. [Crossref] [PubMed]
- Sethian JA. A fast marching level set method for monotonically advancing fronts. Proc Natl Acad Sci U S A 1996;93:1591-5. [Crossref] [PubMed]
- Frangi AF, Niessen WJ, Vincken KL, et al. Multiscale vessel enhancement filtering. In: International Conference on Medical Image Computing and Computer-Assisted Intervention. 1998, October. Berlin: Springer, Heidelberg; p. 130-7.
- Haralick RM, Shanmugam K. Textural features for image classification. IEEE Trans Syst Man Cybern 1973;6:610-21. [Crossref]
- Sato M. Virtual assisted lung mapping: navigational thoracoscopic lung resection. Cancer Res Front 2016;2:85-104. [Crossref]
- Fave X, MacKin D, Yang J, et al. Can radiomics features be reproducibly measured from CBCT images for patients with non-small cell lung cancer? Med Phys 2015;42:6784-97. [Crossref] [PubMed]