Targets without tolerances: improper evaluation of medical personnel
Introduction
Efforts to assess the performance of medical professionals relative to various local/regional/national standards are an integral part of mandated assessment of medical practice in the United States. Assessment is usually accomplished by comparing some measurement statistic [hospital mortality rate, patient length of stay (LOS), etc.] based on provider data with a performance target defined by the adjusted mean of historical data from an internal or external database. If the measurement is in the direction viewed as favorable relative to the target mean (i.e., lower is better, or higher is better) then provider performance is acceptable, otherwise it is unacceptable
This method of performance assessment has numerous shortcomings, which are well known to quality control practitioners in industry (1). In this paper, we identify these shortcomings and use our in-house program’s analysis of patient hospital LOS data to illustrate the incorrectness of current practice. We describe correct methods of performance assessment, which use means and variance measures to define targets and tolerances, and we demonstrate how the use of targets and their associated tolerances can be used to provide meaningful insight into medical practice, reduce costs, and identify real changes in provider performance. While the focus will be on assessment of medical personnel, the same methods apply to the assessment of performance at all levels of medical service.
The measured outcome of any effort at any level in any situation in any hospital is variable. This variation is due to a myriad of known and unknown factors. Because of variation, summaries of outcome measures are often expressed in terms of a single simple estimate of the central tendency of the distribution such as an average. An average is one of a series of moments (mean, standard deviation, skewness, kurtosis, etc.) needed to characterize the distribution of the measures with which they are associated.
When variation is present, no distribution of measurements can be uniquely summarized by a single moment. At the very minimum one must have an estimate of the central tendency and an estimate of the variation of the measurement distribution. Two moments that are frequently used for these measures are either the mean and the variance or the mean and the standard deviation; the square root of the variance.
Variation in an outcome is either expected or uncontrolled. In the literature, these variations are identified as ordinary and special (or assignable) cause (1,2). Ordinary variation is process variation when the process is functioning at its optimum whereas special cause variation is variation due to one or more process factors changing in a non-random manner.
Assessing provider performance relative to targets based on grand or covariate adjusted means, without incorporating into that assessment tolerances based on ordinary process variation, is standard practice in medicine. For example, the entire process for reimbursement for medical services is based on numeric estimates from the Relative Value Scale Update Committee (RUC) (3) or the Resource Based Relative Value Scale (RBRVS) (4). These values are the basis for predictive models built by various insurance institutions (Medicare/Medicaid, private insurer, HMO’s, etc.) to determine reimbursement. The terms in the predictive models vary from insurer to insurer (4) but, in the end, the predicted reimbursement for a rendered service is an adjusted mean (a target) without an associated estimate of ordinary variation (a tolerance).
Target only evaluations such as these assume they have correctly identified and adjusted for sources of special cause variation and that ordinary process variation does not exist.
This approach has three immediate and unacceptable consequences:
- Without tolerances, targets cease representing typical/acceptable and become a minimum/maximum bound for acceptable performance;
- Ignoring ordinary variation means every excursion away from target will be treated as though it is due to special cause variation. Thus, unfavorable excursions will be viewed as a call for corrective actions and favorable excursions will be viewed as an improvement in the process;
- If the excursion away from target is due to ordinary process variation efforts to implement the imagined improvement or correct the imagined problem will fail and will further degrade process performance because ordinary variation is variation in the absence of any special perturbation to the system. Under these circumstances unneeded adjustments are special cause variation and serve only to increase process variability and waste resources. In the statistics literature, this kind of process adjustment is called over control (5).
The final target value for a particular outcome will vary depending on the database, the covariates included in the analysis, and the statistical methods used to generate the target.
However, because they ignore ordinary variation and assume adequate adjustment for the effects of significant covariates has been achieved, the shortcomings of target only assessment methods are identical. Since our in-house program is a target only assessment we will use its output to highlight the problems with this approach.
Methods
Case study: LOS and provider Opportunity Days
At our institution, the target LOS statistic defines standards for individual patient LOS. It is generated by an in-house program based on the All Patient Refined Diagnosis-Related Group (APR-DRG) classification system and purchased covariate adjusted LOS target values augmented with in house patient data. Target LOS values are subtracted from actual LOS values and the remainder is called an Opportunity Day. Any positive Opportunity Day is bad and any negative Opportunity Day is good. Opportunity Days are reported in a myriad of different ways, however the interpretation is the same; negative values are “good”, positive values are “bad” and no attempt is made at any level of computation or assessment to account/adjust for process variation.
The data consists of admission/discharge patient data for the period between January and October 2010 for 12 physicians in the Cardiothoracic Surgery service line at the Cleveland Clinic. The usual population demographics are omitted from Table 1 because they are not the focus of Opportunity Days performance reports sent to providers. The severity of illness (SOI) summary is included because it, along with inherited time, will be used in the discussion about covariate adjustment for sources of special cause variation.
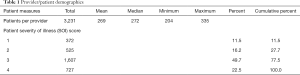
Full table
Current practice assigns all of the patient hospital time to the last physician of record. Thus, if a patient has spent 10 days in the hospital under the care of physician A and on day 11 is given to physician B and then discharged on day 12 under physician B’s instructions all 12 days will accrue to physician B. Thus, physician B will have inherited responsibility for 10 days of the patient stay over which he/she had no control. It is this segment of time outside of the control of a physician that we define as inherited time.
Inherited time is determined by differencing the date of admission and the provider service record date. Based on the experience of one of us (Johnston), a difference of 24 hours or less is a good indication the patient was under the care of the provider for the entire length of their stay whereas a larger difference indicates the patient was inherited. Provider time for a patient is computed by differencing the service record date and the date of discharge. If the LOS based on provider time only and the LOS based on provider time plus inherited time represent LOS from two different processes then a histogram of the combined LOS data will often exhibit two distinct peaks and is classed as bimodal (6). Figure 1 is a plot of the LOS attributed to one physician coded by inherited time. The difference between single physician time (7.98 days) and inherited time (16.1 days) means is clinically and statistically significant (P<0.0001). If LOS source is ignored, the overall mean patient LOS (12.1 days) attributed to the provider is significantly inflated when compared to single physician time (P=0.002) and does not represent actual provider LOS.
Under current assessment methods the analysis of the data for any particular period of time will result in reported average Opportunity Days for each of the 12 providers. A rank ordering of their averages will identify the numerically best and worst physician average (Table 2). This approach assumes all 66 pairwise differences in provider means are clinically meaningful.
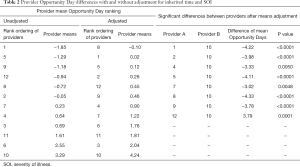
Full table
A better approach to provider assessment is a linear regression of Opportunity Days on provider ID employing Tukey-Kramer adjustments for multiple pairwise means comparisons to identify differences which are statistically significant. This method of comparing means is based on the residual variation of the data, and amounts to a performance assessment based on targets and tolerances. When this is done the correlation between provider and Opportunity Days exhibits overall significance (P<0.0001) and statistically significant (P<0.05) differences for 32 of the 66 possible comparisons.
This approach assumes the covariate-adjusted data on which it is based has adequately accounted for all other provider level sources of special cause variation save that due to providers. If true then regressing the outcome of Opportunity Days against provider and any other potential source of special cause variation should result in statistical significance for provider only. However if, as a test, we regress Opportunity Days against SOI (ordinal rating of 1–4) and inherited time (simply coded as 0= no inherited time, 1= inherited time), and provider ID all variables are statistically significant: inherited time (P<0.0001), SOI (P=0.022), and provider ID (P<0.0001). It should be emphasized that the point of this three variable analysis (provider, inherited time, and SOI) is to highlight the failure of the current practice of using covariate adjusted data to correctly account for all sources of special cause variation; not to suggest these variables alone adequately explain all the special cause variation present.
With just these two sources of special cause variation removed, the test for significant differences between provider means, using residual variation as an estimate of the process tolerance, reduces the count of significant differences to those between provider #10 and 8 others (columns 5–8 of Table 2). The Opportunity Days calculation does not adjust for inherited time, however, SOI is considered in the development of the externally provided LOS estimates. Therefore, if the database used by the in-house program had adequately adjusted for SOI at the provider level this variable should not have exhibited statistical significance when tested against Opportunity Days.
If the in house program had been based on a different or larger data set of covariate-adjusted data or if our institution had used any other covariate adjusted, target only, assessment program the problems outlined above would have remained. Alternate data sets and programs might not share the same weakness with respect to correctly adjusting for Inherited Time and SOI but they would fail with respect to adjusting for other important variables because hospitals and their service lines are not identical entities, the patient populations they serve are not uniform, and systemic factors which impact the mean and variation of a process outcome in one hospital setting may have greater, lesser or no impact on the same outcome in another and conversely (7,8).
At the hospital/service line level the best way to assess provider LOS differences and provide targets and tolerances is to use methods of regression analysis to identify and eliminate sources of special cause variation specific to a given service line/provider and provide target LOS values and their tolerances after these sources have been removed.
Using regression analysis to identify/control sources of special cause variation is the first step in process improvement whose result is often the physical removal of the variation source from the process and a concomitant overall improvement in process performance. However, in many hospital processes the actual removal of sources of special cause variation (i.e., patients with inherited time or given levels of severity) would be illegal, immoral, and/or unethical. In these instances statistical methods must be used to mathematically remove the variation in the data attributable to these special causes and provide adjusted data to use to evaluate provider performance (9-11). Once a regression equation is constructed and goodness-of-fit to the data has been confirmed, predicted values for each observed data point can be generated. The residuals of a regression equation are the differences between actual measures and their predicted values. Residuals therefore are data adjusted for the effects of known sources of special cause variation and as such provide an estimate of the ordinary variation of the process.
The best way to use residual data to understand process changes, identify ordinary and special cause variation, provide proper comparison between providers, and act as a starting point for further investigations of process performance and the regression equation is a control chart of the residuals (10).
There are many types of control charts (12,13)) (individual moving range, Xbar/R, p, c, and u charts, cumulative sum, etc.) and numerous articles (14-17) have been written illustrating their value in assessing performance relative to a target. Since provider LOS performance is assessed on a case-by-case basis the control chart of choice is an individual moving range (IMR) chart centered on the regression-adjusted provider mean.
An IMR plots residuals over time and the patterns of the residuals are assessed using standard control chart rules (1,18). The 99% control limits span the range of variation routinely (1) understood to be due to ordinary variation, which means these limits represent tolerances around the target mean. These limits also define the boundaries between ordinary and special cause variation (1).
We will use the control charts (Figures 2) of providers #1 and #10 to illustrate an immediate and a long-term benefit of this approach to performance assessment. The residuals in these charts are those resulting from the simple 3 parameter model discussed previously.
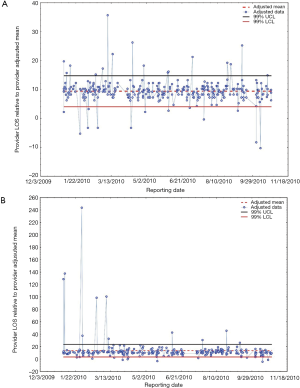
Regardless of the ranking criterion, provider #10 is the lowest ranked provider in Table 2. By the current rules concerning performance evaluation, he/she would be the one most likely to receive an unsatisfactory rating.
If the control charts for provider #1 (ranked either first or second) and #10 are compared visually four things are immediately apparent:
- The distribution of data around the target mean for provider #1 appears random whereas the majority of provider #10’s data is below the adjusted (target) mean;
- The majority of the adjusted LOS measures for both providers fall between their 99% limits;
- Both provider #1 and #10 have patients with adjusted responses outside the upper and lower 99% control limits;
- There is a marked difference in the distribution of patients with adjusted LOS means in excess of the upper 99% control limit between providers #1 and #10.
A visual inspection of the two control charts suggests the reason for the odd distribution of points inside #10’s control limits and the difference between #10’s average LOS and other providers is due to the five inherited patients with adjusted LOS values greater than 40 days. A re-examination of the data without the five extreme values confirms this. The immediate benefit to the service line is the recognition that, for this period of time, #10’s lower performance is due to chance and not to his/her performance.
If we assume the adjustments made for SOI and inherited time are adequate then the presence of patients outside the 99% control limits suggests the existence of other important process factors. Specifically LOS values above the upper tolerance limit suggest the possible existence of unknown factors detrimental to process performance and LOS values below the lower tolerance suggests the existence of process factors, which, if identified, could drive improvements in medical service.
All of the providers have data points outside the tolerance limits and it is this data that should be collected and subjected to additional analysis. The focus would be that of searching for process factors similar to and different from factors associated with patients whose outcomes are within tolerance. The long term benefit of testing for in and out of tolerance patient measure differences will be the identification of process factors, which, if controlled, will improve quality of care and outcome measures.
Results
The analysis of the shortcomings of the target only method of provider assessment highlights the importance of tolerances and identifies what needs to be done at the local, state and federal level to establish fair standards for assessing performance.
The analysis focused on the correct methods for internal assessment of provider performance; however, these issues also apply to the generation and use of target only state and national standards. Without tolerances it is impossible to use these standards fairly to judge provider or hospital performance. In spite of this grave shortcoming their use, for precisely this purpose, continues.
To be of value these standards must be provided with the sample size used, the sources of special causes controlled for in their development, and a meaningful estimate of their associated ordinary variation. How this variation is reported will depend on the kinds of measurements involved (19).
Used in conjunction with the methods described in this paper such standards would give everyone, patient, provider, and governing agency alike, a clear and correct understanding of the quality of the performed medical services. It would provide an accurate means of assessing cost and reimbursement for medical practice, permit fair inter-hospital/inter-provider assessment, and would clearly define ordinary and special cause variation at any level of medical practice with respect to any local, state, or national standard.
Conclusions
The focus of this paper has been on the correct assessment of hospital personnel performance with respect to LOS. However, the methods described apply to any effort in the hospital setting where the emphasis is on performance relative to any mandated internal or external target.
Pay for performance if done correctly makes complete sense. However, if it is based only on comparing differences between performance measures and targets it is incorrect, unfair, counterproductive (20), and central to declining physician satisfaction. If variation is not taken into account, ranking, rewarding, and punishing clinicians becomes a largely random event where blind chance, instead of physician effort, govern performance evaluation. Continuing use of current performance assessment methods guarantees unending, large-scale waste of time, money, and effort.
Acknowledgements
The authors would like to thank Stephanie Kocian for her time and work on the manuscript.
Footnote
Conflicts of Interest: The authors have no conflicts of interest to declare.
References
- Ash AS, Fienberg SE, Louis TA, et al. Statistical Issues in Assessing Hospital Performance: Commissioned by the Committee of Presidents of Statistical Societies. Washington, D.C.: CMS, 2011.
- Baltic S. Uncovering the Mysteries of RUC. Med Econ 2013;90:35-6, 38-40. [PubMed]
- Benneyan JC. Statistical Quality Control Methods in Infection Control and Hospital Epidemiology, Part II: Chart Use, Statistical Properties and Research Issues. Infect Control Hosp Epidemiol 1998;19:265-83. [Crossref] [PubMed]
- Bothe DR. Measuring Process Capability: A Reference Handbook for Quality and Manufacturing Engineers. Cedarburg, WI: Landmark Publishing Inc., 2001.
- Carmel S, Rowan K. Variation in intensive care unit outcomes: a search for the evidence on organizational factors. Curr Opin Crit Care 2001;7:284-96. [Crossref] [PubMed]
- Cooper R, Kramer TR. RBRVS costing: the inaccurate wolf in expensive sheep's clothing. J Health Care Finance 2008;34:6-18. [PubMed]
- Deming WE. Quality, Productivity, and Competitive Position. Cambridge, MA: Massachusetts Institute of Technology, 1982;125-7.
- Duncan AJ. Quality Control and Industrial Statistics. 4th edition. Homewood, IL: Richard D. Irwin Inc., 1974.
- Hartz AJ, Kuhn EM. Comparing hospitals that perform coronary artery bypass surgery: the effect of outcome measures and data sources. Am J Public Health 1994;84:1609-14. [Crossref] [PubMed]
- Hawkins DM. Regression Adjustment for Variables in Multivariate Quality Control. J Qual Technol 1993;25:170-82. [Crossref]
- Lee K, McGreevey C. Using control charts to assess performance measurement data. Jt Comm J Qual Improv 2002;28:90-101. [Crossref] [PubMed]
- Lilford R, Mohammed MA, Spiegelhalter D, et al. Use and misuse of process and outcome data in managing performance of acute medical care: avoiding institutional stigma. Lancet 2004;363:1147-54. [Crossref] [PubMed]
- Mandel BJ. The Regression Control Chart. J Qual Technol 1969;1:1-9. [Crossref]
- Ryan TP. Statisticl Methods for Quality Improvement. 3rd edition. New York, NY: John Wiley & Sons, 1989.
- Tague NR. The Quality Toolbox. 2nd edition. Milwaukee, WI: ASQ Quality Press, 2005:292-9.
- Tukey JW. Exploratory Data Analysis. Reading, MA: Addison-Wesley Publishing Company, 1977.
- Wheeler DJ. Understanding Statistical Process Control. In: Chambers C. editor. Understanding Statistical Process Control. 2nd edition. Knoxville, TN: SPC Press, 1992.
- Wheeler DJ. Understanding Variation. In: Understanding Variation. Knoxville, TN: SPC Press, 2000.
- Wheeler DJ. Advanced Topics in Statistical Process Control. 2nd edition. Knoxville, TN: SPC Press, 2004.
- Woodhall WH. The Use of Control Charts in Health-Care and Public Surveillance. J Qual Technol 2006;38:89-104. [Crossref]