Design, methods and baseline characteristics of the Beijing Hospital Atherosclerosis Study: a prospective dynamic cohort study
Introduction
Atherosclerotic coronary artery disease (CAD) is associated with an increased risk of mortality and is a primary cause of death worldwide (1). Atherosclerosis is the result of a complex metabolic disorder caused by the interaction of various environmental, lifestyle, and genetic factors. The identification and early intervention of atherosclerosis risk factors are the key measures for reducing the morbidity and mortality of CAD patients in both primary and secondary prevention measures. However, the performance of conventional risk factors (e.g., age, smoking status, blood pressure, and serum lipids) is only moderate (2). The current screening method has limitations in identifying patients who will develop clinical CAD. Significant numbers of recurrent cardiovascular events continue to occur even when aggressive treatment strategies are adopted. Such events are known as residual risks (3,4). The identification of novel risk factors may extend our knowledge of CAD physiopathology and enable us to identify patients at high risk in the context of primary and secondary cardiovascular prevention (4).
Metabolomics refers to a holistic approach for analyzing metabolites of low-molecular weight in biological samples. Unlike genetic profiles, metabolomics respond to environmental influences and represent the chemical basis of current physiologic states and clinical phenotypes (5). Due to its high sensitivity and specificity, liquid chromatography-tandem mass spectrometry (LC-MS/MS)-based metabolomics technologies have the potential to identify novel disease markers and provide insights into biological mechanisms (6). In previous studies, metabolomics analyses in this field have led to the discovery of novel molecular biomarkers associated with CAD, individually or as a group, including amino acids, such as branched-chain amino acids (BCAAs) (6-9), acylcarnitines, dicarboxylacylcarnitines (10-12), trimethylamine N-oxide (TMAO) (13,14), and several lipid classes (15-17). Untargeted metabolomics provide an unbiased approach to biomarker identification; however, the challenges of untargeted approach include characterizing unknown metabolites and processing extensive raw data. By quantitatively analyzing certain metabolites, the targeted metabolomics approach is more sensitive and specific than untargeted approach and is suitable for the validation of the metabolites. Thus, the usefulness of these metabolites in CAD risk assessment needs to be confirmed and validated in external independent populations with targeted metabolomics to allows for the simultaneous measurement of all the potential metabolites from different pathways.
Lifestyle choices, such as drinking, smoking, exercising and nutrition, are closely related to the development and recurrence of CAD (18). Healthy lifestyle choices include not smoking, regular physical activity, maintaining a normal body mass index (BMI), and moderate alcohol consumption. Adherence to a combination of a healthy diet and lifestyle behaviors may be the most important and effective way to intervene and prevent CAD. However, the relationship between lifestyle and disease has conventionally been investigated by questionnaires, which have biases and are likely to affect the observed associations between lifestyle risk factors and clinical outcomes. Conversely, nutritional and lifestyle biomarkers may offer a more objective and reliable method for CAD risk assessment and nutritional intervention (19-21).
Some single blood biomarkers, such as plasma vitamin C, and serum carotenoids, have been used as indicators of fruit and vegetable intake (22). The use of a combination of potential biomarkers may more accurately predict fruit and vegetable intake in intervention studies than the use of any single biomarker (23,24). In a recent study, Ottosson et al. discovered novel independent associations between plasma dimethylguanidino valerate (DMGV) and incident CAD and cardiovascular mortality (25). Additionally, strong associations of DMGV levels with sugar-sweetened beverages, vegetable intake, and physical activity suggest that lifestyle interventions have the potential effects on plasma DMGV levels (25). The identification of lifestyle-modifiable metabolic pathways related to CAD risk is essential for CAD prediction and prevention in susceptible individuals.
Age is the most profound risk factor for almost all non-communicable diseases, including CAD, and has played an important role in CAD risk assessment. However, the ageing rate, which is measured as the decline in functional capacity and stress resistance, differs in every individual (26). Biomarkers of human ageing, which measure biological age (BA), could be used to identify individuals at high risk of developing age-associated diseases or disabilities. BA has been evaluated or calculated by different strategies [e.g., telomere length (27), epigenetics (28), proteostasis, metabolomics, and laboratory tests (29,30)]. It remains unclear whether most biomarkers forecast chronological age or BA, but it is likely that the 2 are distinct. As many factors can influence single markers, an analysis that uses a sole biomarker to describe the complex process of ageing may be impossible. Thus, a set of biomarkers should be used to determine the BA, all of which should be based on mechanisms described by major theories of ageing. In the Framingham offspring study, Murabito et al. tested the associations of BA measures constructed from different types of biomarkers with mortality and disease in a community-based sample, and found that BA based on laboratory and clinical parameters provided the best prediction of mortality (31). BA, when substituted for chronological age, has the potential to improve the prediction of ageing and age-related diseases, such as CAD.
Based on these analyses, we planned to conduct a prospective, single-centre, observational cohort study in patients undergoing coronary angiography (CAG) in Beijing Hospital. Although there were some previous cohort studies involved in subjects undergoing CAG (32-35), studies systematically focusing on the prediction of metabolomics, lifestyle biomarkers and BA on CAD risk are rare. For example, the Guangdong Coronary Artery Disease Cohort (34) investigated the associations between diet and lifestyle and risks for cardiovascular disease. However, the information about diet and lifestyle were collected by questionnaires, which may have biases in dietary misreporting and likely to impact the observed associations between lifestyle and disease. Thus, the current cohort may offer a valid complementary information to self-reported surveys in analyzing the relationships between lifestyle and CAD risk in China. Another study included a cohort of 2,324 patients who underwent CAG for suspected CAD from 4 independent centers in Jiangsu, China (35). Untargeted metabolomic profiles were determined in plasma samples, and 89 differential metabolites were identified among different CAD subgroups. However, the study did not follow up on cardiovascular events, so the findings need to be confirmed in prospective analysis. Some other cohorts (32,33) focused new biomarkers for prediction of the presence of significant CAD and related events, but they do not investigate lifestyle biomarkers and BA. In our study, baseline analysis of traditional CAD risk factors, metabolic and lifestyle-related biomarkers, and BA derived from clinical tests and metabolomics will be performed. The relationship between these parameters and the presence of CAD will be analysed. New factors for the risk of future events will be verified.
Therefore, the ultimate goal of the present cohort study is to identify and validate the new biosignatures (especially the metabolomics, lifestyle biomarkers and biological age), and elucidate their predictive effect on CAD and subsequent cardiovascular events. In this report, we present the study design/protocol together with baseline data, including demographic characteristics, clinical profiles, and medical treatment, of the initial 2970 patients enrolled. We present the following article in accordance with the STROBE reporting checklist (available at https://atm.amegroups.com/article/view/10.21037/atm-22-2834/rc).
Methods
General design
Figure 1 shows the general design of this study. Patients who are suspected of having CAD or have a definite history of CAD and have been subjected to CAG have been enrolled in this study. These patients’ baseline demographic information has been recorded. Biochemical parameters, metabolomics and lifestyle biomarkers, and BA have been recorded and measured. The patients will be followed up annually. The associations between the above parameters and the occurrence of CAD and the recurrence of CAD events will be analyzed. Novel CAD risk factors will be screened and validated.
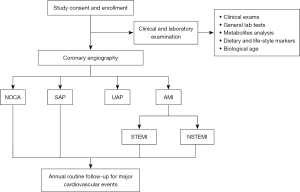
Patient enrolment and exclusion criteria
The subjects comprise all hospitalized patients (aged >18 years) who underwent CAG at Beijing Hospital from March 2017 to 2020. All potentially eligible patients are examined for any diseases or conditions that may exclude them from the study. Patients are excluded from the study if they meet any of the following exclusion criteria: (I) have undergone an aortic dissection, have a pulmonary embolism, malignant tumor, autoimmune disorder, severe infectious disease, or trauma, have undergone a recent surgical procedure, have had severe heart failure with a left ventricular ejection fraction <20%, have liver dysfunction [alanine amino transferase (ALT) level >135 U/L], severe renal dysfunction [creatinine (Cr) >4.0 mg/dL], or blood-borne infectious diseases, including human immune-deficiency virus/acquired immune-deficiency syndrome, hepatitis B, or hepatitis C; (II) have a significant malignancy or tumor disease that will require advanced medical or surgical therapy or both in the following year; (III) have other major systemic diseases that will require hospitalization or operation in the following year; (IV) are unable or unwilling to be followed up during the subsequent 1-year period; (V) have a life expectancy of <12 months; (VI) are unable to complete the baseline questionnaires; and/or (VII) are pregnant or nursing, suffering from alcoholism or drug abuse, or have a mental illness for which they are undergoing treatment. The confirmed eligible patients are included in this study after obtaining their personal consent. All the included patients complete a questionnaire with the help of a doctor. Each patient’s weight, height, and blood pressure are measured. The baseline demographics and medical histories associated with CAD, such as smoking, hypertension (HTN), diabetes mellitus (DM) and dyslipidemia are recorded by specially trained cardiologists. HTN, DM, and dyslipidemia patients include those who have already been diagnosed with these diseases at enrolment according to certain guidelines (36-38). Before the CAG procedure, venous blood samples are collected in common vacutainer tubes after overnight fasting, and the serum is isolated within 2 h, aliquoted into vials and stored at −80 ℃ awaiting analysis.
To guarantee data quality and reliable results, we formulated and revised the survey forms, designed the standardized investigation guidelines and trained the investigators. In the phase of the data collection, the contents of questionnaire survey are directly entered on the portable computer, and the electronic questionnaire software system is set up with logic error checking and quality control functions, so possible errors at the time of data input were minimized through real-time monitoring.
Ethics and dissemination
This study was reviewed and approved by the Ethics Committee of Beijing Hospital (No. 2016BJYYEC-121-02) and was conducted in accordance with the Declaration of Helsinki (as revised in 2013). All patients admitted to the Cardiology Department of Beijing Hospital for CAG signed the written consent forms, detailing the purpose of this study, its duration, follow-up time and period, sample collection procedure, and potential risks. On the final completion of this study, we plan to submit the findings for publication in relevant peer-reviewed journals. We also plan to present the findings of this study at national and international conferences and meetings.
CAG and definition of clinical types of CAD
Elective CAG is performed using standard techniques. All the targeted coronary lesions of enrolled patients are analyzed by the built-in QCA software of the AlluraXper FD20 Angiography System (Philips Healthcare, Netherlands). The measurements are performed in all coronary segments according to the 15-segment American Heart Association classification. The coronary artery segments are carefully selected by 2 cardiologists on the basis of smooth luminal borders and the absence of stenosis. CAD is defined as stenosis ≥50% in any 1 of the coronary arteries or major branches. Individuals with <50% stenosis and those with negative findings in computed tomography of the coronary arteries or stress myocardial perfusion imaging are allocated to the non-CAD group.
The diagnosis of the type of CAD is based on the clinical symptoms, laboratory tests, electrocardiogram (ECG), and coronary angiographic results by specially trained cardiologists. Patients with <50% stenosis in all coronary vessels are allocated to the non-obstructive coronary artery (NOCA) group. The stable angina pectoris (SAP) group includes patients with angina of stable frequency lasting up to 10 min or provocative-palliative factors. Patients with a history of angina (within 1 month), irregular angina at rest or upon minimal exertion, and no elevation in troponin are allocated to the unstable angina pectoris (UAP) group. Patients who have ischemic chest pain, increased values of cardiac enzymes, and dynamic ST-T change on ECG are allocated to the acute myocardial infarction (AMI) group, in which patients with and without an elevation of ST-segment are allocated to the ST-segment elevation myocardial infarction (STEMI) and the non-STEMI (NSTEMI) groups, respectively. The angiographic data are confirmed independently by 2 observers.
Clinical laboratory tests, metabolomics, and BA
Biochemical and immunological profiles, including blood glucose, lipids, liver and kidney function, N-terminal pro-B-type natriuretic peptide, immunoglobulin M, and complete blood count are measured at the clinical laboratory of Beijing Hospital before CAG. Biomarkers related to oxidative stress and ageing, such as superoxide dismutase, and dehydroepiandrosterone, are measured by the KingMed Diagnostics Group Co., Ltd. using reagents and instruments from Roche Diagnostics Ltd. These laboratories routinely participate in external quality assessment/proficiency testing programs organized by the National Center for Clinical Laboratory in China. To monitor inter-run variation, 2 serum samples are included as quality controls (QCs) and measured in each run to monitor variations between different assays.
Serum metabolites will be screened and identified based on a literature review and our previous studies. In our previous study, we used LC-MS/MS methods for amino acids, lysophosphatiydicholine (LPC), carnitines, choline, and metabolites (39-44), as well as lifestyle and nutritional biomarkers, such as cotinine, phosphatidyethanol (45), and polyphenols (46). In addition to the above-mentioned metabolites and lifestyle biomarkers, other substances, such as fatty acids, DMGV and vitamins, which have been consistently reported to be associated with CAD (22-25), will be integrated into our targeted metabolomics approach. Serum metabolites are extracted with organic solvents. After vortexing, the vials are centrifuged, and the supernatant is transferred to another vial and evaporated under a stream of nitrogen. The residue is reconstituted with the mobile phase and analyzed by LC-MS/MS. LC separation is performed using an Agilent 1260 Series High-performance liquid chromatography (HPLC) system (Santa Clara, CA, USA). Tandem MS/MS detection is carried out on an AB Sciex 5500 QTRAP system (Framingham, MA, USA) with both positive and negative electronic spray ionization in multiple-reaction monitor mode. Quantification is carried out by isotopically labelled internal standards and calibration curves. All patient samples will be analyzed, with 50–98 samples in each run. Additionally, 2 QC materials will be measured in each run to monitor inter-run variations.
The BA models are based on our previous analysis. We have analyzed the changes in over 100 laboratory indices with age and disease morbidity using routine clinical data from the Physical Examination Center of Beijing Hospital (n=200,000). We identified some of the indices that have strong relationships with age and established a mathematical model for BA (unpublished data) using the basic principle of Klemera-Doubal (31). A total of 14 indicators were used to build the BA model, including albumin, ALT, Cr, total cholesterol (TC), fasting blood glucose (FBG), inorganic phosphorus, and some indicators of complete blood count. The BA model will be further optimized and validated in the current study.
Clinical follow-up for adverse cardiovascular events
All the studied patients will attend an annual routine follow-up appointment for up to 10 years after the CAG procedure. The follow-up data will be recorded during the outpatient clinic visits as applicable. For those who do not visit the clinic, telephone follow-up interviews will be conducted by trained physicians. As selection bias can occur in a prospective cohort study due to differences in retention during the follow-up period after enrolment, we will do our best to reduce loss to follow-up by maintaining contact with participants at regular intervals and collecting baseline information to facilitate the tracking of subjects (e.g., the addresses, phone numbers, and email addresses of not only the subjects but also possible contacts, such as next of kin or close friends). Loss to follow-up will be recorded and analyzed to determine if there are differences in the likelihood of it being related to exposure status and outcome. Follow-up outcomes includes mortality, myocardial infarction, stroke, rehospitalization, coronary revascularization procedures, and medication use. The primary end points for the study are major adverse cardiovascular events (MACEs), which are defined as death from any cause, non-fatal myocardial infarction, and non-fatal stroke. After the follow-up period, associations between baseline parameters and cardiovascular events will be analyzed to evaluate the risk prediction efficiencies of these biomarkers.
Statistical analysis
In the cross-sectional design for the baseline data, the studied subjects will be divided into different groups according to clinical, anatomical or prognostic features. Missing data will be filled by subsequent measurements. Multiple imputation methods will account for additional variability in the parameter estimates for clinical variables with missing data. Continuous variables will be expressed as the mean and standard deviation (SD) if the distribution is normal or as the median and interquartile range (IQR) if the distribution is skewed. Categorical variables will be presented as both absolute frequencies (the number of patients) and relative frequencies (percentage). Comparisons of continuous variables between groups will be performed by an analysis of variance, while subgroup comparisons of categorical variables will be assessed by the χ2 or Fisher’s exact test.
Baseline clinical, biochemical, angiographic, and novel biomarkers that predict MACEs in the prospective study will be used to build a predictive model by regression analysis. The outcome measures of interest will be described as an overall percentage. These outcomes will also be expressed as the mean of proportions and their 95% confidence intervals (CIs). For the regression models, we will report the odds ratio (for the logistic regression) or hazard ratio (for the Cox proportional hazards regression), the corresponding standard error, the 95% CIs, and the P values. Net reclassification improvement and integrated discrimination improvement analyses will be performed to compare the identification of MACEs between different groups using traditional risk factors and novel biomarkers. In all the tests, we will use the 2-tailed alpha significance level of 0.05.
According to published data (47), the 3-year occurrence of MACEs for CAG patients is approximately 13%. Thus, we have conservatively estimated that a sample size of 2,800–3,000 subjects will provide sufficient sensitivity and specificity for the detection of the primary outcome using biomarkers if the follow-up rate is 80–90%.
Results
Baseline demographic characteristics of the enrolled 2,970 patients
The demographic, clinical, and biochemical data of the enrolled 2,970 patients have been collected and are presented in this article. The baseline demographic data of the patients are presented in Table 1. The baseline biochemical parameters are set out in Table 2. The results indicate that there were more males (62.5%) than females, and the patients tended to be old and overweight with an average age of 65.6 years and a BMI of 25.7. The percentages of diagnosed hypertension, diabetes, dyslipidemia, and current smokers were 67.3%, 35.2%, 44.2%, and 31.8%, respectively. A total of 8.5% of the patients had a family history of CAD, and 7.7% had previously suffered an ischemic stroke. However, the mean levels of TC, triglycerides (TG), high-density lipoprotein cholesterol (HDL-C), and low-density lipoprotein cholesterol (LDL-C) were within the normal ranges, which was probably due to the use of statins.
Table 1
Baseline demographic variable | Values |
---|---|
Age at enrolment, years | 65.6±10.9 |
Male | 1,855 (62.5) |
Body mass index, kg/m2 | 25.7±3.5 |
Systolic blood pressure, mmHg | 136.7±18.5 |
Diastolic blood pressure, mmHg | 78.3±11.3 |
Heart rate, bpm | 74.5±12.0 |
Hypertension | 2,000 (67.3) |
Diabetes mellitus | 1,046 (35.2) |
Dyslipidaemia | 1,312 (44.2) |
Smoking | 944 (31.8) |
Family history of CAD | 170 (8.5) |
Previous ischemic stroke | 229 (7.7) |
Values are presented as mean ± SD or n (%). CAD, coronary artery disease; SD, standard deviation.
Table 2
Baseline blood biochemical parameter | Mean ± SD | Median (IQR) |
---|---|---|
FBG, mmol/L | 6.7±2.2 | 5.9 (5.1–7.5) |
TC, mmol/L | 3.9±0.9 | 3.8 (3.2–4.5) |
LDL-C, mmol/L | 2.4±0.8 | 2.3 (1.8–2.8) |
HDL-C, mmol/L | 1.1±0.3 | 1.0 (0.9–1.2) |
TG, mmol/L | 1.4±0.7 | 1.3 (0.9–1.8) |
Non-HDL-C, mmol/L | 2.9±0.9 | 2.7 (2.2–3.4) |
Cr, μmol/L | 71.7±17.2 | 70.0 (60.0–81.0) |
eGFR, mL/min per 1.73 m2 | 96.8±24.0 | 95.3 (81.5–110.3) |
Uric acid, μmol/L | 327.0±88.1 | 319.0 (266.0–379.0) |
White blood cells, /CUMM | 6.4±1.8 | 6.1 (5.2–7.3) |
Hemoglobin, g/L | 131.5±16.4 | 132.0 (121.0–143.0) |
Platelet, /CUMM | 203.0±57.1 | 198.0 (164.0–235.8) |
SD, standard deviation; IQR, interquartile range; FBG, fasting blood glucose; TC, total cholesterol; LDL-C, low-density lipoprotein cholesterol; HDL-C, high-density lipoprotein cholesterol; TG, triglycerides; Cr, creatinine; eGFR, estimated glomerular filtration rate; CUMM, cubic millimetre.
Baseline clinical characteristics of the study population
The baseline clinical data of the patients are set out in Table 3. Based on clinical characteristics and laboratory examinations, CAD is divided into 4 different categories: NOCA, SAP, UAP, and AMI. The percentages of NOCA, SAP, UAP, and AMI were 17.9%, 20.8%, 50.1%, and 9.3% respectively. The mean Gensini score was 25.8±33.6, with a median of 12.0 (2.0–37.5). The percentage of patients who had received statin treatment was 34.4%, of whom, 25.4% had continued statin treatment for more than 1 year.
Table 3
Baseline clinical characteristic | Values |
---|---|
Clinical type of CAD*, n (%) | |
NOCA | 532 (17.9) |
SAP | 617 (20.8) |
UAP | 1,487 (50.1) |
AMI | 275 (9.3) |
STEMI | 81 (2.7) |
NSTEMI | 194 (6.5) |
Gensini score | |
Mean ± SD | 25.8±33.6 |
Median (IQR) | 12.0 (2.0–37.5) |
Statins#, n (%) | |
Not use | 1,651 (55.6) |
Less than 1 year | 267 (9.0) |
Over 1 year | 754 (25.4) |
*, 59 patients could not be classified into any category; #, statin use was unclear in 298 records. CAD, coronary artery disease; NOCA, non-obstructive coronary atherosclerosis; SAP, stable angina pectoris; UAP, unstable angina pectoris; AMI, acute myocardial infarction; STEMI, ST-segment elevation myocardial infarction; NSTEMI, non-ST-segment elevation myocardial infarction; SD, standard deviation; IQR, interquartile range.
Discussion
It is recognized that traditional cardiovascular risk factors (e.g., age, hypertension, hyperlipidemia, smoking, and diabetes) do not fully explain interindividual variations in cardiovascular risk. In the case of CAD, most individuals have only 1 or none of the classic cardiovascular risk factors (48). Thus, the identification of novel risk factors that could contribute information in addition to that already provided by traditional risk factors is of crucial importance. Our ongoing study aims to investigate the associations between traditional and novel biomarkers and the occurrence of CAD. It also aims to identify novel biomarkers either independently or concomitantly to predict the occurrence of adverse cardiovascular events or aid in CAD interventions. Our target population is hospitalized patients at Beijing Hospital undergoing CAG. The study subjects have relatively high CAD risks and high risks of coronary events in the follow-up periods. Compared to low-risk populations, these patients have been adequately risk stratified and definitively diagnosed, which allows us to conduct biomarker screening in relatively small populations with short follow-up periods.
Based on previously published studies and our own experience, this study focuses on the following 3 different aspects: metabolomics, lifestyle biomarkers, and BA. First, circulating metabolites are closely related to cellular function and clinical phenotype and have the advantage of providing immediate information about physiological conditions. Thus, metabolomics provides a potential tool for identifying biomarkers for better risk classification and for understanding the pathophysiological mechanisms of CAD (6,9). The current data are promising, but the potentially useful biomarkers need to be verified in independent populations, especially in longitudinal studies. Second, lifestyle biomarkers not only serve as markers of exposure but are also used as indicators for CAD interventions (18), which is very important in CAD management. Additionally, as ageing in humans is highly variable with wide differences in health at a given chronological age, BA will add complementary information beyond that provided by chronological age to predict disease risk and lifespan (25,27). Given their potential to identify novel predictive biomarkers of CAD, studies in these fields have generated considerable excitement. However, prospective cohort studies need to be carried out to establish the relationships between biomarkers and CAD, improve CAD risk assessment, and verify the predictive values of certain biomarkers.
In previous studies, we developed a series of targeted LC-MS/MS methods to determine CAD-related metabolites, including BCAAs, LPCs, and TMAO (39-41). The preliminary data indicated that serum BCAAs, glycine, some species of carnitines, and TMAO were significantly correlated with traditional CAD risk factors and CAD (32-44). We have also established LC-MS/MS methods to examine phosphatidyethanol, and polyphenol profiles, which not only reflect the frequency of drinking, and the dietary intake of fruits and vegetables but are also related to CAD risk factors (45,46). Further, we have investigated the correlations of all the conventional clinical parameters with age and generated a mathematical model for constructing BA, which has shown the ability to differentiate among individuals with different health conditions (data not provided). In this study, all the potentially useful biomarkers and lifestyle biomarkers will be integrated into reliable and specific targeted metabolomics methods. BA models based on clinical examinations, traditional laboratory tests, and metabolomics approach will be studied and constructed. These biomarkers will be evaluated either independently or concomitantly, predicting the occurrence of adverse cardiovascular events. Based on pre-existing studies, we chose to use death from any cause, non-fatal myocardial infarction, and non-fatal stroke as our MACEs.
Limitations
The main limitation of our work is that the Beijing Hospital Atherosclerosis Study (BHAS) is a single-center cohort study, and all of the subjects included in the study are undergoing cardiac catheterization because of suspected CAD and carry a high burden of cardiovascular risk. Thus, the representativeness of the study cohort may be an issue.
Conclusions
This BHAS, which is a prospective, single-center, observational cohort study, has been successfully started. Hospitalized patients undergoing CAG were enrolled from 2017 to 2020. The baseline demographic data, biochemical parameters, and clinical characteristics of the enrolled 2,970 patients were presented in this article. The associations between baseline parameters and the presence/severity of CAD will be analyzed. All the patients will be followed-up annually to assess the effects of the standard and novel biomarkers on future clinical events. In this study, novel metabolic biomarkers, lifestyle and nutritional biomarkers, and BA related to CAD will be investigated. Our primary goals are to assess the capability of these biomarkers to identify the presence of CAD and to explore their ability to predict subsequent cardiovascular events. Findings from this study will not only provide novel indicators of potential associations between secondary prevention measures and future adverse events but will also help to improve our understanding of effective therapeutic targets and strategies to retard or prevent the progression of CAD.
Acknowledgments
The authors would like to thank Yu Wang, Lijiao Zhang, Yufei Wang, Qinghui Meng, Helu Xiu, and Qichen Long from Peking University Health Science Center and Peking Union Medical College for collecting and preparing the blood samples.
Funding: This work was supported by the National High Level Hospital Clinical Research Funding (No. BJ-2022-113), the CAMS Innovation Fund for Medical Sciences (grant No. 2021-I2M-1-050), and the National Natural Science Foundation of China (grant No. 81672075).
Footnote
Reporting Checklist: The authors have completed the STROBE reporting checklist. Available at https://atm.amegroups.com/article/view/10.21037/atm-22-2834/rc
Data Sharing Statement: Available at https://atm.amegroups.com/article/view/10.21037/atm-22-2834/dss
Conflicts of Interest: All authors have completed the ICMJE uniform disclosure form (available at https://atm.amegroups.com/article/view/10.21037/atm-22-2834/coif). The authors have no conflicts of interest to declare.
Ethical Statement: The authors are accountable for all aspects of the work in ensuring that questions related to the accuracy or integrity of any part of the work are appropriately investigated and resolved. This study was reviewed and approved by the Ethics Committee of Beijing Hospital (No. 2016BJYYEC-121-02) and was conducted in accordance with the Declaration of Helsinki (as revised in 2013). All patients admitted to the Cardiology Department of Beijing Hospital for CAG signed the written consent forms, detailing the purpose of this study, its duration, follow-up time and period, sample collection procedure, and potential risks.
Open Access Statement: This is an Open Access article distributed in accordance with the Creative Commons Attribution-NonCommercial-NoDerivs 4.0 International License (CC BY-NC-ND 4.0), which permits the non-commercial replication and distribution of the article with the strict proviso that no changes or edits are made and the original work is properly cited (including links to both the formal publication through the relevant DOI and the license). See: https://creativecommons.org/licenses/by-nc-nd/4.0/.
References
- GBD 2013 Mortality and Causes of Death Collaborators. Global, regional, and national age-sex specific all-cause and cause-specific mortality for 240 causes of death, 1990-2013: a systematic analysis for the Global Burden of Disease Study 2013. Lancet 2015;385:117-71. [Crossref] [PubMed]
- Gilstrap LG, Wang TJ. Biomarkers and cardiovascular risk assessment for primary prevention: an update. Clin Chem 2012;58:72-82. [Crossref] [PubMed]
- Alagona P Jr, Ahmad TA. Cardiovascular disease risk assessment and prevention: current guidelines and limitations. Med Clin North Am 2015;99:711-31. [Crossref] [PubMed]
- Stephens JW, Ambler G, Vallance P, et al. Cardiovascular risk and diabetes. Are the methods of risk prediction satisfactory? Eur J Cardiovasc Prev Rehabil 2004;11:521-8. [Crossref] [PubMed]
- Omori K, Katakami N, Yamamoto Y, et al. Identification of Metabolites Associated with Onset of CAD in Diabetic Patients Using CE-MS Analysis: A Pilot Study. J Atheroscler Thromb 2019;26:233-45. [Crossref] [PubMed]
- Ussher JR, Elmariah S, Gerszten RE, et al. The Emerging Role of Metabolomics in the Diagnosis and Prognosis of Cardiovascular Disease. J Am Coll Cardiol 2016;68:2850-70. [Crossref] [PubMed]
- Newgard CB, An J, Bain JR, et al. A branched-chain amino acid-related metabolic signature that differentiates obese and lean humans and contributes to insulin resistance. Cell Metab 2009;9:311-26. [Crossref] [PubMed]
- Wang TJ, Larson MG, Vasan RS, et al. Metabolite profiles and the risk of developing diabetes. Nat Med 2011;17:448-53. [Crossref] [PubMed]
- Shah SH, Sun JL, Stevens RD, et al. Baseline metabolomic profiles predict cardiovascular events in patients at risk for coronary artery disease. Am Heart J 2012;163:844-850.e1. [Crossref] [PubMed]
- Khan A, Choi Y, Back JH, et al. High-resolution metabolomics study revealing l-homocysteine sulfinic acid, cysteic acid, and carnitine as novel biomarkers for high acute myocardial infarction risk. Metabolism 2020;104:154051. [Crossref] [PubMed]
- Gundogdu G, Senol O, Demirkaya Miloglu F, et al. Serum metabolite profiling of ST-segment elevation myocardial infarction using liquid chromatography quadrupole time-of-flight mass spectrometry. Biomed Chromatogr 2020;34:e4738. [Crossref] [PubMed]
- Guasch-Ferré M, Zheng Y, Ruiz-Canela M, et al. Plasma acylcarnitines and risk of cardiovascular disease: effect of Mediterranean diet interventions. Am J Clin Nutr 2016;103:1408-16. [Crossref] [PubMed]
- Thomas MS, Fernandez ML. Trimethylamine N-Oxide (TMAO), Diet and Cardiovascular Disease. Curr Atheroscler Rep 2021;23:12. [Crossref] [PubMed]
- Li XS, Obeid S, Klingenberg R, et al. Gut microbiota-dependent trimethylamine N-oxide in acute coronary syndromes: a prognostic marker for incident cardiovascular events beyond traditional risk factors. Eur Heart J 2017;38:814-24. [Crossref] [PubMed]
- Stegemann C, Pechlaner R, Willeit P, et al. Lipidomics profiling and risk of cardiovascular disease in the prospective population-based Bruneck study. Circulation 2014;129:1821-31. [Crossref] [PubMed]
- Alshehry ZH, Mundra PA, Barlow CK, et al. Plasma Lipidomic Profiles Improve on Traditional Risk Factors for the Prediction of Cardiovascular Events in Type 2 Diabetes Mellitus. Circulation 2016;134:1637-50. [Crossref] [PubMed]
- Ottosson F, Emami Khoonsari P, Gerl MJ, et al. A plasma lipid signature predicts incident coronary artery disease. Int J Cardiol 2021;331:249-54. [Crossref] [PubMed]
- Yu E, Rimm E, Qi L, et al. Diet, Lifestyle, Biomarkers, Genetic Factors, and Risk of Cardiovascular Disease in the Nurses' Health Studies. Am J Public Health 2016;106:1616-23. [Crossref] [PubMed]
- Mastrangelo A, Barbas C. Chronic Diseases and Lifestyle Biomarkers Identification by Metabolomics. Adv Exp Med Biol 2017;965:235-63. [Crossref] [PubMed]
- Beckmann M, Lloyd AJ, Haldar S, et al. Dietary exposure biomarker-lead discovery based on metabolomics analysis of urine samples. Proc Nutr Soc 2013;72:352-61. [Crossref] [PubMed]
- Garcia-Perez I, Posma JM, Gibson R, et al. Objective assessment of dietary patterns by use of metabolic phenotyping: a randomised, controlled, crossover trial. Lancet Diabetes Endocrinol 2017;5:184-95. [Crossref] [PubMed]
- Baldrick FR, Woodside JV, Elborn JS, et al. Biomarkers of fruit and vegetable intake in human intervention studies: a systematic review. Crit Rev Food Sci Nutr 2011;51:795-815. [Crossref] [PubMed]
- Woodside JV, Draper J, Lloyd A, et al. Use of biomarkers to assess fruit and vegetable intake. Proc Nutr Soc 2017;76:308-15. [Crossref] [PubMed]
- McGrath AJ, Hamill LL, Cardwell CR, et al. Combining vitamin C and carotenoid biomarkers better predicts fruit and vegetable intake than individual biomarkers in dietary intervention studies. Eur J Nutr 2016;55:1377-88. [Crossref] [PubMed]
- Ottosson F, Ericson U, Almgren P, et al. Dimethylguanidino Valerate: A Lifestyle-Related Metabolite Associated With Future Coronary Artery Disease and Cardiovascular Mortality. J Am Heart Assoc 2019;8:e012846. [Crossref] [PubMed]
- Kennedy BK, Berger SL, Brunet A, et al. Geroscience: linking aging to chronic disease. Cell 2014;159:709-13. [Crossref] [PubMed]
- Zhang WG, Zhu SY, Bai XJ, et al. Select aging biomarkers based on telomere length and chronological age to build a biological age equation. Age (Dordr) 2014;36:9639. [Crossref] [PubMed]
- Jones MJ, Goodman SJ, Kobor MS. DNA methylation and healthy human aging. Aging Cell 2015;14:924-32. [Crossref] [PubMed]
- Ferrucci L, Gonzalez-Freire M, Fabbri E, et al. Measuring biological aging in humans: A quest. Aging Cell 2020;19:e13080. [Crossref] [PubMed]
- Justice JN, Ferrucci L, Newman AB, et al. A framework for selection of blood-based biomarkers for geroscience-guided clinical trials: report from the TAME Biomarkers Workgroup. Geroscience 2018;40:419-36. [Crossref] [PubMed]
- Murabito JM, Zhao Q, Larson MG, et al. Measures of Biologic Age in a Community Sample Predict Mortality and Age-Related Disease: The Framingham Offspring Study. J Gerontol A Biol Sci Med Sci 2018;73:757-62. [Crossref] [PubMed]
- Ibrahim NE, Januzzi JL Jr, Magaret CA, et al. A Clinical and Biomarker Scoring System to Predict the Presence of Obstructive Coronary Artery Disease. J Am Coll Cardiol 2017;69:1147-56. [Crossref] [PubMed]
- Leu HB, Yin WH, Tseng WK, et al. Identification of new biosignatures for clinical outcomes in stable coronary artery disease - The study protocol and initial observations of a prospective follow-up study in Taiwan. BMC Cardiovasc Disord 2017;17:42. [Crossref] [PubMed]
- Ding D, Qiu J, Li X, et al. Hyperglycemia and mortality among patients with coronary artery disease. Diabetes Care 2014;37:546-54. [Crossref] [PubMed]
- Fan Y, Li Y, Chen Y, et al. Comprehensive Metabolomic Characterization of Coronary Artery Diseases. J Am Coll Cardiol 2016;68:1281-93. [Crossref] [PubMed]
- Mancia G, Fagard R, Narkiewicz K, et al. 2013 ESH/ESC guidelines for the management of arterial hypertension: the Task Force for the Management of Arterial Hypertension of the European Society of Hypertension (ESH) and of the European Society of Cardiology (ESC). Eur Heart J 2013;34:2159-219. [Crossref] [PubMed]
- Classification and Diagnosis of Diabetes. Diabetes Care 2016;39:S13-22. [Crossref] [PubMed]
- Joint Committee for the Prevention and Treatment of Dyslipidemia in Chinese Adults. Guidelines for the prevention and treatment of dyslipidemia in Chinese adults (2016 Revised Edition). Chin J Cardiol 2016;44:833-50.
- Yang R, Dong J, Guo H, et al. Rapid and precise measurement of serum branched-chain and aromatic amino acids by isotope dilution liquid chromatography tandem mass spectrometry. PLoS One 2013;8:e81144. [Crossref] [PubMed]
- Wang M, Yang R, Dong J, et al. Simultaneous quantification of cardiovascular disease related metabolic risk factors using liquid chromatography tandem mass spectrometry in human serum. J Chromatogr B Analyt Technol Biomed Life Sci 2016;1009-1010:144-51. [Crossref] [PubMed]
- Wang M, Yang R, Mu H, et al. A simple and precise method for measurement of serum free carnitine and acylcarnitines by isotope dilution HILIC-ESI-MS/MS. Int J Mass Spectrom 2019;446:116208. [Crossref]
- Yang RY, Wang SM, Sun L, et al. Association of branched-chain amino acids with coronary artery disease: A matched-pair case-control study. Nutr Metab Cardiovasc Dis 2015;25:937-42. [Crossref] [PubMed]
- Li X, Sun L, Zhang W, et al. Association of serum glycine levels with metabolic syndrome in an elderly Chinese population. Nutr Metab (Lond) 2018;15:89. [Crossref] [PubMed]
- Wang S, Yu X, Zhang W, et al. Association of serum metabolites with impaired fasting glucose/diabetes and traditional risk factors for metabolic disease in Chinese adults. Clin Chim Acta 2018;487:60-65. [Crossref] [PubMed]
- Wang S, Yang R, Ji F, et al. Sensitive and precise monitoring of phosphatidylethanol in human blood as a biomarker for alcohol intake by ultrasound-assisted dispersive liquid-liquid microextraction combined with liquid chromatography tandem mass spectrometry. Talanta 2017;166:315-20. [Crossref] [PubMed]
- Yang R, Xiu H, Zhou Q, et al. Application of Urinary Polyphenol Biomarkers Measured by Liquid Chromatography Tandem Mass Spectrometry to Assess Polyphenol Intake and Their Association with Overweight and Obesity in Free-Living Healthy Subjects. Oxid Med Cell Longev 2019;2019:4809836. [Crossref]
- Tang WH, Wang Z, Levison BS, et al. Intestinal microbial metabolism of phosphatidylcholine and cardiovascular risk. N Engl J Med 2013;368:1575-84. [Crossref] [PubMed]
- Khot UN, Khot MB, Bajzer CT, et al. Prevalence of conventional risk factors in patients with coronary heart disease. JAMA 2003;290:898-904. [Crossref] [PubMed]
(English Language Editor: L. Huleatt)