Urinary proteomic analysis during pregnancy and its potential application in early prediction of gestational diabetes mellitus and spontaneous abortion
Introduction
Maternal physiological adaptation during gestation is a complex process characterized by metabolic, anatomical, and immune changes (1), which have a significant impact on diverse systems in the body. The hematological, cardiovascular, respiratory, renal, and endocrine systems undergo changes to meet the demands of fetal growth and development, and prepare the mother for parturition (2). Pregnancy has been reported to affect protein expression in maternal serum and urine (3), and thus, a full description of protein expression may aid in understanding the physiological changes during pregnancy and the factors affecting maternal health and pregnancy outcomes. With the rapid development of mass spectrometry (MS) technology in recent years, proteomics has become an important tool for assessing protein expression profiles and can provide an understanding of physiological changes and disease pathogenesis.
Biological specimens, including maternal plasma (1,4), umbilical cord plasma (5), amniotic fluid (6), and urine (3), have been used to characterize protein and peptide changes in normal pregnancy. Unlike plasma and other biofluids in vivo, urine is not responsible for maintaining the homeostatic microenvironment, which makes it superior as a source of biomarkers (7). Urine has also been shown to have great potential in reflecting systemic physiological characteristics, which are influenced by factors such as sex, age, diet, hormone status, lifestyle, and extreme environments (8). To date, only one urinary proteomic study on the process of normal pregnancy has been published (3). In urine samples collected before and after vaginal delivery, 16 differential urinary phosphoproteins were found (3). However, whether the urinary proteome reflects the physiological changes of normal pregnancy remains largely unknown.
Urinary proteome analysis has so far been employed to understand and characterize prenatal complications such as hypertension (9), preeclampsia (10-12), and gestational diabetes mellitus (GDM) (13,14). GDM is considered the most common metabolic disorder of pregnancy, and it has severe implications (15). Clinical diagnostic guidelines for GDM recommend that a 75-g oral glucose tolerance test (OGTT) be performed at 24 to 28 weeks (16). However, as the diagnosis is established during the second trimester of pregnancy, drug therapy and dietary intervention are started relatively late. Consequently, inevitable and sometimes irreversible adverse effects on the mother and fetus can occur during the first trimester; early diagnosis and intervention are therefore extremely important. Previous urinary proteome research studied differential proteins during the second or third trimester (13,14), and none of them focused on early biomarkers of GDM.
Spontaneous abortion (SA)—defined as pregnancy loss without intervention before 20 gestational weeks—is a complication that affects approximately 20% of recognized pregnancies (17). Around half of SAs are attributable to genetic abnormalities, such as chromosomal abnormalities (18). There are also multiple other risk factors, including maternal infections, medications, toxins, uterine abnormalities, and chronic maternal diseases (17). It is vital that predictive biomarkers for women who are at increased risk of SA are developed. To the best of our knowledge, no urinary proteomic study has been published on the early diagnosis of SA.
This prospective cohort study aimed to collect urine samples from pregnant women during the first, second, and third trimesters (6–8, 22–24, and 32–34 weeks, respectively). The aim of the study was to perform serial urinary proteome analyses using a data-independent acquisition (DIA) approach to compare physiological and pathophysiological changes during gestation in normal pregnancy, pregnancy affected by GDM, and SA. The differential proteins in the GDM group and the SA group were then verified using a parallel reaction monitoring (PRM) approach (Figure 1). This study showed that the urinary proteome has the potential to reflect the changes of pregnancy progression and might facilitate early diagnosis of pregnancy complications. We present the following article in accordance with the STROBE reporting checklist (available at https://atm.amegroups.com/article/view/10.21037/atm-21-3497/rc).
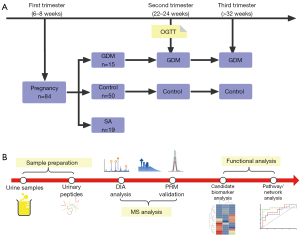
Methods
Ethical statement
The research protocol of this study was approved by the Ethics Committee of Peking Union Medical College Hospital (No. ZS-976). The study was conducted under the guidance of the Major New Drugs Innovation and Development Program (Clinical Trial No. NCT03246295). Between October 2015 and May 2016, all pregnant women treated at the Peking Union Medical College Hospital were recruited. All study participants were given a verbal explanation of the study before enrollment, and each participant signed an informed consent form. The study was conducted in accordance with the Declaration of Helsinki (as revised in 2013).
Patient groups
A total of 84 pregnant women were recruited, and urine samples were collected at 6 to 8 weeks of gestation. GDM was diagnosed at 24 to 28 gestational weeks using an OGTT test. For a diagnosis to be made, at least one of the following criteria needed to be present: fasting blood glucose ≥5.1 mmol/L, 1-hour blood glucose ≥10.0 mmol/L, or 2-h blood glucose ≥8.5 mmol/L. Fifteen pregnant women developed GDM at 24 to 28 weeks, and 19 women were diagnosed as having had an SA before 12 weeks. Women diagnosed with GDM implemented lifestyle (diet and exercise) interventions to control blood glucose.
The participants were divided into four groups. (I) The GDM group: urine samples were collected at 6–8 weeks, 22–24 weeks, and >32 weeks from the 15 women who developed GDM; (II) the Control-1 group: urine samples were collected during each trimester from 15 healthy pregnant women, who were matched to GDM group based on clinical characteristics; (III) the SA group: urine samples were collected at 6–8 weeks from the 19 women who were diagnosed as having had an SA before 12 weeks; and (IV) the Control-2 group: urine samples were collected during the first trimester from who were matched to SA group based on clinical characteristics. The clinical characteristics of patients in the GDM, SA, and control groups are shown in Tables 1,2. Second-morning midstream urine samples were collected from all the participants, centrifuged, and stored at −80 ℃ for analysis.
Table 1
Characteristics | GDM group | Control-1 group | P value |
---|---|---|---|
Sample size (N) | 15 | 15 | |
Maternal age (years) | 33.8±3.61 | 32±5.01 | 0.31 |
Prepregnancy BMI (kg/m2) | 24.2±2.88 | 23.8±2.33 | 0.34 |
24–28 weeks fasting glucose (mmol/L) | 4.98±0.42 | 4.4±0.21 | 0.01 |
24–28 weeks OGTT 1-h glucose (mmol/L) | 10.28±1.45 | 7.2±2.08 | 0.02 |
24–28 weeks OGTT 2-h glucose (mmol/L) | 8.49±1.06 | 6.4±1.06 | 0.02 |
Gravity | 1.7±0.9 | 2±1.31 | 0.21 |
Deliveries | 1.3±0.49 | 1.2±0.41 | 0.21 |
GDM, gestational diabetes mellitus; OGTT, oral glucose tolerance test.
Table 2
Characteristics | SA group | Control-2 group | P value |
---|---|---|---|
Sample size (N) | 19 | 19 | |
Maternal age (years) | 34.71±4.57 | 34.11±3.95 | 0.34 |
Gravity | 2.6±1.45 | 2.21±1.2 | 0.21 |
Deliveries | 0.6±0.49 | 1.32±0.46 | 0.00 |
Systolic blood pressure (mmHg) | 107.19±10.27 | 106.17±7.57 | 0.38 |
Diastolic blood pressure (mmHg) | 68.44±9.08 | 69±7.8 | 0.43 |
Gestation age at delivery | 275.17±8.44 |
SA, spontaneous abortion.
Sample preparation
Urinary proteins were extracted from 10 mL of urine from each sample via acetone precipitation, and then digested using a filter-aided sample preparation method (19). To generate a protein library and for quality control, a pooled sample with equal amounts of protein from all participants was digested simultaneously. Briefly, proteins were denatured through incubation with 50 mM dithiothreitol at 56 ℃ for 1 h and alkylated in the dark for 45 min in 55 mM iodoacetamide at room temperature. The proteins were then treated with trypsin (1:50) and incubated at 37 ℃ for 8 h. The tryptic peptides were desalted using Oasis HLB cartridges (Waters, Milford, MA, USA).
High-pH reversed-phase liquid chromatography separation
To generate a library for DIA analysis, urinary peptides from the pooled sample were loaded on offline high-pH reversed-phase liquid chromatography (RPLC) columns (4.6 mm × 250 mm, C18, 3 µm; Waters, Milford, MA, USA) in buffer A1 (H2O, pH 10). The peptides were eluted for 60 minutes with 5−30% buffer B1 (90% acetonitrile, pH 10; flow rate, 0.7 mL/min) and collected at one fraction per minute. In total, 30 fractions were lyophilized, resuspended, and concatenated into 10 fractions by combining fractions 1, 11, 21 and so on.
Liquid chromatography–MS analysis
The individual samples were analyzed using DIA mode. According to the previously described DIA-MS workflow (20,21), firstly, a pooled sample was obtained by mixing the same amount of digested peptide from each individual in the GDM, SA, and Control-1 and Control-2 groups. Then, an extensive data-dependent acquired (DDA) spectral library of the pooled samples was generated using two-dimensional liquid chromatography with tandem MS (LC-MS/MS) in DDA mode. Finally, the individual samples were analyzed using the DIA mode.
The Orbitrap Fusion Lumos MS (Thermo Scientific, Dreieich, Germany) coupled with an EASY-nLC 1000 liquid chromatography system was used for MS analysis. The digested peptides were dissolved in 0.1% formic acid and separated on an RP C18 self-packing capillary LC column (75 µm × 100 mm, 3 µm). The eluted gradient was 5–30% buffer B2 (0.1% formic acid, 99.9% ACN; flow rate, 0.3 µL/min) for 60 min.
To generate the spectral library, the peptide fractions separated by RPLC were analyzed in DDA mode. The parameters were set as follows: the full scan was acquired at a resolution of 60,000, and tandem MS scans were performed at a resolution of 15,000 with an isolation window of 1.6 Da and higher-energy collisional dissociation (HCD) energy of 32%.
The DIA data were acquired with the maximum injection time set to 50 ms for full and DIA scans, and the cycle time set to 1.55 s. The full scan was set at a resolution of 120,000 over an m/z range of 400 to 900, and was followed by DIA scans with a resolution of 30,000 and HCD collision energy of 32%.
Data analysis
To generate the spectral library, the DDA data acquired were processed using the Proteome Discoverer (Thermo Scientific, Dreieich, Germany) and searched against the human UniProtfasta database (Homo sapiens, 71,592 sequences, 2017_09 version) appended with the iRT fusion protein sequence. The search allowed two missed cleavage sites in the trypsin digestion; cysteine carbamidomethylation was set as a fixed modification, parent ion mass tolerances were set to 10 ppm, and fragment ion mass tolerances were set to 0.02 Da. The applied false discovery rate (FDR) cutoff was 0.01 at the protein level. The spectral library was then generated by importing the results to the Spectronaut™ Pulsar software (Biognosys, Schlieren, Switzerland).
The DIA-MS raw files were analyzed using Spectronaut Pulsar 14.10 (Biognosys, Schlieren, Switzerland). Briefly, the DIA raw data were loaded to Spectronaut™ to calculate peptide retention time based on iRT data. The Spectronaut™ software identified and quantitated proteins by matching the retention time and m/z to the peptide library. The proteins were filtered with at least two unique peptides, at an FDR threshold of 1% at the protein level. Then, those proteins that were identified in less than 70% of the samples were removed for further analysis in the GDM and SA groups. Missing values were imputed with the median value of each group.
Principal component analysis (PCA) and orthogonal partial least squares discriminant analysis (OPLS-DA) were conducted using SIMCA 13.0.2 (Umetrics, Umeå, Sweden) statistical software.
The area under the receiver operating characteristic (ROC) curve (AUC) was calculated using MedCalc 15.8 (www.medcalc.org). A GDM diagnostic panel was constructed using a logistic regression algorithm available from MedCalc.
Bioinformatics analysis
For ingenuity pathway analysis (IPA), the UniProt accession numbers were uploaded to IPA software (QIAGEN, Germanland, MD, USA). The proteins were mapped to disease and function categories and canonical pathways available in Ingenuity and other databases, and were ranked according to the P value. For each function and network, the enrichment significance was calculated using a one-sided Fisher’s exact test.
Targeted proteomic analysis
Data derived from the spectral library using HCD collision were imported into Skyline (v.3.6) (22). The most intense peptide transitions were selected. Up to five transitions per peptide were traced on a TripleTOF 5600 mass spectrometer (AB Sciex, Framingham, MA, USA). Between one and three peptides from each protein were selected for quantification. For ionization, a spray voltage of 2.20 kV and a capillary temperature of 150 ℃ were used. The peptides were monitored by using the PRM acquisition mode to perform tandem MS scans of the precursor ions for the allpeptide markers along the complete chromatographic run. The normalized collision energy was fixed to 35%, and the accumulated time was 100 ms. The resulting tandem MS data were processed using Skyline for further visualization, transition detection, and quantity calculation.
Statistical analysis
PCA and orthogonal partial least squares discriminant analysis (OPLS-DA) were conducted using SIMCA 13.0.2 (Umetrics, Umeå, Sweden) statistical software. One hundred permutation tests was performed to estimate there was no over-fitting in these models.
A comparison of the proteins between multiple trimesters were analyzed using one-way analysis of variance (ANOVA). A P value of less than 0.05 was considered to be serially changed proteins. A comparison of the differential proteins between disease and control groups was analyzed using unpaired student t-test in DIA and PRM analysis. Proteins with fold change >1.5 and P<0.05 was considered to be significant differential proteins.
The area under the receiver operating characteristic (ROC) curve (AUC) was calculated using MedCalc 15.8 (www.medcalc.org). A GDM diagnostic panel was constructed using a logistic regression algorithm available from MedCalc.
Results
Demographic characteristics of the study participants
A cohort of 84 pregnant women was initially recruited for this prospective study. Among the initial cohort, 19 women were diagnosed as having had an SA before 12 weeks of gestation, and 15 women were diagnosed with GDM through an OGTT test performed at 24 to 28 weeks of gestation. Fifteen healthy pregnant women were also included for the purpose of studying the longitudinal dynamic urinary proteomic changes in different trimesters during pregnancy. These healthy women (Control-1 group) were matched to the 15 patients with GDM by age, body mass index, and clinical laboratory data. Furthermore, another 19 healthy pregnant women (Control-2 group) were matched to the 19 women with SAs based on above clinical characteristics. Tables 1,2 shows the general clinical characteristics of women who had a normal pregnancy and those diagnosed with GDM or who had an SA.
Library generation, and protein identification and quantification
To generate the urinary proteome spectral library, the pooled urinary peptides from all samples were separated into 30 fractions using offline high-pH RPLC and analyzed using DDA LC/MS/MS analysis. The raw data were searched and filtered with Proteome Discoverer software. Finally, a spectral library containing 21,190 precursors, 10,173 peptides, and 2,015 proteins was generated.
Urinary peptides from each sample were analyzed using a DIA approach, and the data were loaded to the Spectronaut Pulsar software for qualitative and quantitative analysis. A total of 1,783 proteins which had at least two unique peptides and met an FDR threshold of 1% at the protein level were identified. For each sample, the identification protein number ranged from 1,112 to 1,620 (Figure S1A and available online: https://cdn.amegroups.cn/static/public/atm-21-3497-1.xlsx). A total of 1,328 proteins remained for further analysis after missing values had been filtered.
The urinary peptides from all pooled samples were also assessed as a quality control measure, whereby every eighth sample was assessed through a standard DIA analysis. Fifteen replicates were then generated to evaluate the technical performance. For the 15 technical replicates, the average number of proteins identified was 1,465, and 1,277 proteins were identified in all 15 runs. The median and 90% quantile Coefficient of variation (CV) of the quantity of all the identified proteins were 0.14 and 0.34, respectively. The Spearman’s correlation coefficients between replicates were calculated, and the median R-squared value was 0.97 for all 15 replicates (Figure S1B). The above results evidenced that the integrated analysis system was stable and the technical reproducibility was acceptable.
Longitudinal urinary proteomic study of normal pregnancy
The urinary proteomes of patients with normal pregnancies were characterized during each trimester (at 6–8, 22–24, and 32–36 weeks) using high-throughput LC-MS/MS methods. The DIA data were subjected to multivariate statistical analysis using SIMCA 13.0.2 software. The PCA score plot showed that there is a considerable intergroup distinction of the proteins altered in 1st, 2nd and 3rd trimester of gestation of healthy controls (Figure 2A). The first trimester differed significantly from the second and third trimesters. The OPLS-DA score plots showed greater significant differences between the three trimesters (Figure 2B). One hundred permutation tests showed that there was no overfitting (Figure S2A). The above data indicated that the urinary proteome exhibited differences across the trimesters of normal pregnancies.
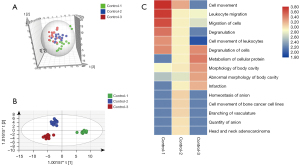
To illustrate the variability in the urinary proteome with the progression of pregnancy, the differential proteins across the three trimesters were analyzed using one-way analysis of variance (ANOVA). In total, 249 urinary proteins (available online: https://cdn.amegroups.cn/static/public/atm-21-3497-2.xlsx) were identified as serially changed proteins (P<0.05). Figure 2C depicts the functional annotation analysis (IPA) of the differentially expressed proteins during the three trimesters. The proteins that were highly expressed during the first trimester were mostly involved in the cell movement/migration and leukocyte migration. Most of the differential proteins during the second trimester were associated with vasculature branching, anion quantity, and homeostasis. For the third trimester, cellular protein metabolism, body cavity, and morphology were enriched. The functional and pathway analyses of the three trimesters demonstrated that the urinary proteome differed in terms of protein composition and function during gestation, suggesting that it may reflect the physiological changes of pregnancy.
A cross-sectional study of predictive markers in GDM pregnancy
As with normal pregnancies, it was possible to separate the first, second, and third trimesters of pregnancies affected by GDM using PCA and F-DA analysis (Figure 3A, Figure S2). In the GDM group, 216 urinary proteins were identified as serially changed proteins (ANOVA) (available online: https://cdn.amegroups.cn/static/public/atm-21-3497-2.xlsx). The functional annotation analysis (Figure S2) of urinary proteins in the GDM group showed that during the first trimester, proteins were enriched in cell movement and migration, which was similar to what was observed in normal pregnancies. However, during the second trimester, proteins were functionally annotated in receptor-mediated endocytosis and the classical complement pathway. During the third trimester, proteins participated in cellular invasion and organismal injury and abnormalities. The longitudinal urinary proteomic results of pregnancies affected by GDM differed from those of normal pregnancies, especially during the second and third trimesters.
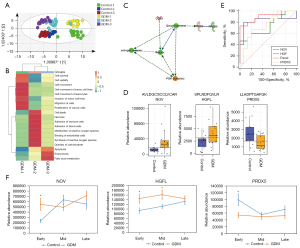
Comparisons of normal pregnancies and pregnancies affected by GDM across the different trimesters through multivariate analysis using OPLS-DA showed a marked difference between the two groups in all three trimesters (Figure 3A, Figure S3). In particular, women with GDM and the normal controls exhibited different urinary proteome patterns, even during the first trimester. To probe the underlying difference, a cross-sectional differential proteome analysis was performed. A total of 48, 90, and 82 urinary proteins were found to be differentially expressed between the two groups during the first, second, and third trimesters, respectively (available online: https://cdn.amegroups.cn/static/public/atm-21-3497-3.xlsx). The functional annotations of the differential proteins during the three trimesters are shown in Figure 3B. In the first trimester, the differential proteins were associated with intracellular activities, such as cell movement, migration, and viability. The differential proteins during the second trimester were functionally related to necrosis, immune response, and reactive oxygen species (ROS). The third-trimester differential proteins participated in fatty acid metabolism, apoptosis, and endocytosis. The cross-sectional urine proteome analysis of GDM indicated that the differential expressed proteins in the three trimesters are functionally distinct.
As the early diagnosis of GDM is highly important but has not been implemented in clinical practice, the 21 altered urinary proteins during the first trimester were validated using targeted LC-PRM-MS. To evaluate the stability of the platform, a pooled urine sample was randomly analyzed for quality control. The median CV of all replicates was 0.19, which indicated the stability of the MS signal throughout the analysis. Significant abundance changes (P<0.05 using a two-sided t-test) were observed for the 28 peptides corresponding to 21 proteins (available online: https://cdn.amegroups.cn/static/public/atm-21-3497-4.xlsx); the tendency of the 21 proteins was consistent with the DIA results.
The differentially expressed proteins were then imported into IPA to generate the molecular network. As shown in Figure 3C, insulin served as the core component of the network, and was directly and positively connected to growth hormone secretion. Insulin secretion is modulated by upstream negative elements like CCN3 and PI3K, while the elevation of growth hormone has a negative effect on another molecule, PRDX3.
Marked differences were observed in three urinary proteins, NOV, PRDX5, and HGFL (HGFL/MSP-induced motility in cells is known to be mediated by PI3K pathways (23), that also play central parts in the network of insulin effect. The predictive performance of these three proteins was further evaluated using the ROC curve (Tables 2,3). The AUC values of the three proteins for discriminating GDM and healthy pregnancies ranged from 0.72 to 0.83 (Figure 3D). According to the above functional annotation and ROC analyses, a panel of NOV, PRDX5, and HGFL could achieve an AUC value of 0.91 (95% confidence interval: 0.76–1), with sensitivity and specificity of 73.3% and 100%, respectively (Figure 3E). These three proteins are functionally associated with the insulin secretion network and might serve as valuable predictive biomarkers for the early diagnosis of GDM.
Table 3
Peptides | ID | Protein | PRM | DIA | Related diseases | |||||
---|---|---|---|---|---|---|---|---|---|---|
ROC | Disease/CON | P value | ROC | Disease/CON | P value | |||||
AVLDGCSCCLVCAR | P48745 | NOV_HUMAN | 0.83 | 2.84 | 0.02 | 0.78 | 2.14 | 0.02 | GDM | |
LLADPTGAFGK | P30044 | PRDX5_HUMAN | 0.74 | 0.57 | 0.02 | 0.76 | 0.55 | 0.03 | GDM | |
SPLNDFQVLR | P26927 | HGFL_HUMAN | 0.72 | 1.77 | 0.02 | 0.71 | 1.47 | 0.04 | GDM | |
VADPAYLPTQQDVLR | P50148 | GNAQ_HUMAN | 0.78 | 0.44 | 0.01 | 0.83 | 0.55 | 0.01 | SA | |
LFVSGACDASAK | P62873 | GBB1_HUMAN | 0.80 | 0.55 | 0.00 | 0.74 | 0.65 | 0.03 | SA | |
TDTGVSLQTYDDLLAK | Q99988 | GDF15_HUMAN | 0.81 | 0.41 | 0.02 | 0.85 | 0.28 | 0.00 | SA | |
MFDVGGQR | P08754 | GNAI3_HUMAN | 0.75 | 0.63 | 0.03 | 0.81 | 0.61 | 0.01 | SA |
PRM, parallel reaction monitoring; DIA, data-dependent acquisition; ROC, receiver operating characteristic curve; CON, control; GDM, gestational diabetes mellitus.
To understand the changing trends of these three proteins during pregnancy, their relative abundance during the first, second, and third trimesters was analyzed in the control and GDM groups. Using normal pregnancies for comparison, NOV was upregulated during the first trimester in women with GDM pregnancies, but recovered to a normal level during the second and third trimesters. HGF was upregulated during the first and second trimesters in the GDM group, but decreased to a normal level during the third trimester. PRDX5 was downregulated during the first trimester in the GDM group, before recovering to a normal level during the second and third trimesters (Figure 3F).
A cross-sectional study of predictive markers in SA
In this study, 19 urine samples from women who experienced SAs and 19 from Control-2 group, collected at 6 to 8 gestational weeks, were used to study potential predictive biomarkers of SA. The PCA and OPLS-DA of the urinary proteome showed a significant difference between the two groups (Figure 4A,4B). The differential proteins were defined by P<0.05 and fold change >1.5. A total of 86 differentially expressed proteins (56 upregulated and 30 downregulated) were found. IPA functional analysis of these proteins revealed a series of pathways and functions to be involved in the pathogenesis of SA. The top canonical pathways (Figure 4C) included the synaptogenesis signaling pathway, nNOS signaling, and superoxide radical degradation. An upstream analysis of differential proteins indicated that estrogen receptors play important roles in their regulation. Nine proteins (RAB2A, EVPL, ECM1, SPRR1A, GNA/B, EFNA, AHNAK, GNAQ, and GDF) were found to be modulated by the estrogen hormone upstream pathway (Figure 4D).
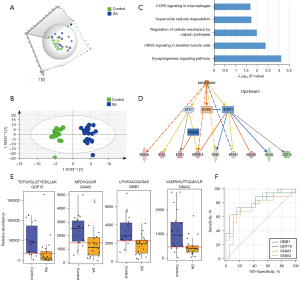
Next, 42 proteins (available online: https://cdn.amegroups.cn/static/public/atm-21-3497-4.xlsx) were validated using the PRM method. The protein change tendency of these 42 proteins in PRM validation was consistent with the DIA results. The predictive performance of these proteins was further evaluated using ROC curve analysis. Four proteins (GDF-15, GNAQ, GBB1, and GNAI3) included in the upstream estrogen metabolism pathway had higher AUC values, ranging from 0.75 to 0.81, than the other proteins (Figure 4E,4F, Tables 3,4). The above data showed that the cause of SA was multifactorial and that the profile of the urinary proteome might provide some clues for early diagnosis.
Table 4
Protein name | Disease | AUC | Sensitivity | Specificity | Accuracy | MCC |
---|---|---|---|---|---|---|
NOV | GDM | 0.742 | 0.667 | 0.8 | 0.733 | 0.471 |
HGF | GDM | 0.827 | 0.8 | 0.933 | 0.867 | 0.74 |
PRDX5 | GDM | 0.724 | 0.6 | 0.8 | 0.7 | 0.408 |
combine | GDM | 0.902 | 0.733 | 1 | 0.867 | 0.761 |
GDF15 | SA | 0.812 | 0.632 | 0.947 | 0.79 | 0.61 |
GNAQ | SA | 0.778 | 0.632 | 0.895 | 0.763 | 0.546 |
GBB1 | SA | 0.801 | 0.737 | 0.842 | 0.789 | 0.582 |
GNAI3 | SA | 0.751 | 0.684 | 0.842 | 0.763 | 0.533 |
AUC, area under the curve; MCC, Matthews correlation coefficient; GDM, gestational diabetes mellitus; SA, spontaneous abortion.
Discussion
In this study, comprehensive urinary proteomic profiling of pregnant women during each trimester was undertaken using the DIA LC-MS/MS approach. During the three trimesters, the urinary proteome showed different patterns and suggested various important biofunctions, which indicated that the longitudinal urinary proteome may reflect the physiological changes during normal pregnancy. Urinary proteomic analysis applied to women with GDM pregnancy and SA further revealed proteomic differences in both protein composition, and canonical pathways and networks, indicating that the urinary proteome may reflect changes related to pregnancy disorders. In summary, the urinary proteome might be used to monitor pregnancies and study pregnancy complications.
The urinary proteome could reflect the changes in normal pregnancy
The urinary proteomic analysis showed that cell movement and leukocyte/cell migration were significantly elevated during the first trimester of normal pregnancy. During this time, cell movement and migration increase to meet the needs of placental development, implantation, and pregnancy. A previous study reported that endometrial epithelial cell-derived interleukin-8 stimulated the migration and survival of first-trimester villous cytotrophoblast cells (24). In terms of the immune responses during the early stage of pregnancy, the elevated leukocyte activity may be attributable to possible changes in progesterone levels, which affects innate immune cells, pathways, and humoral immunity (25).
In the second trimester, the functions of anion homeostasis and branching of vasculature, which might serve in maintaining acid or base balance and optimal solute transport rates, were more significant. Fetal growth accelerates toward the end of the second trimester. In order to meet these alterations in the fetal growth rate, the capacity of the placenta to exchange solute and water also has to increase over the gestation period (26), which may be the cause of the anion homeostasis elevation.
During the third trimester, cellular aggregation, cellular protein metabolism, and the humoral immune response were significantly changed. Cellular aggregation, especially platelet aggregation, may be related to complications in pregnancy or childbirth. Platelet reactivity has been reported to drop during the first trimester of pregnancy but is significantly elevated during the third trimester (27). In the current study, the increased cellular aggregation changes in the late phase might provide some useful clues about platelet reactivity changes throughout pregnancy. Regarding protein metabolism, metabolites such as fatty acid and glucose are well known to be elevated during late pregnancy; however, protein metabolism is also an important component of fetal growth. Some proteins that play a role in metabolic processes, like apolipoprotein C-II, are reported to increase significantly in plasma during the third trimester (1). Based on the above, urinary proteomes in different stages of pregnancy are closely related to known physiological processes and may also provide some new findings.
The urinary proteome might be used for early diagnosis of GDM
When compared with healthy pregnancies, significant differences in physiology were observed for pregnancies affected by GDM depending on the pregnancy stage. In the first trimester, compared to the controls, the GDM group showed upregulation of intracellular activities such as cell survival, viability, movement, and migration. As mentioned previously, in a normal pregnancy, cell movement and migration are significantly elevated during the first trimester to support morphogenesis and body shape establishment. In GDM pregnancies, these changes in basic physiological activities are even more obvious. Previous reports show that in the early stage, the damage to insulin sensitivity is unknown (28). In the current study, PRM was used to validate differential urinary proteins during this early phase of the insulin-related network, which indicated that possible islet damage may occur during the early trimester, even when blood glucose is normal.
During the second trimester, it is evident that increased blood glucose, which results in elevated ROS production (29), may eventually cause pancreatic β-cell damage and cell apoptosis (30). Our results are comparable with the previously reported finding that β-cell levels are significantly lower in women with GDM during the second and third trimesters (31). Functional analysis of the urinary proteome in the GDM group showed more differences in cell death or necrosis and immune response (immune cell adhesion, metabolism, and ROS synthesis), which is consistent with the above reports.
Our urinary proteomic analysis indicated that the fatty acid metabolism pathway varies significantly during the third trimester. As reported previously (1), fatty acid metabolism is closely related to insulin resistance and progress in late pregnancy. A previous study also reported that fatty acid metabolism in women with GDM was different to that of controls (32). Also, cell apoptosis in the third trimester was as significant as that during the second trimester, indicating that the body is recovering during this time.
The above data showed that the urinary proteome can reflect the disease progression of GDM. During the first trimester, the network of differential proteins targeted at insulin-related functions and the glucose level remains normal, which may be the result of tissue changes in the compensatory stage. With the increase of stress stimulation in the second trimester, insulin secretion is impaired, ROS production and blood glucose are increased, and cell apoptosis occurs; this may reflect a decompensation stage. During the third trimester, with lifestyle intervention, blood glucose returns to normal and cell damage is reduced, but fatty acid metabolism remains abnormal, indicating that the body is recovering.
We also attempted to identify urinary biomarkers for the early diagnosis of GDM. Through functional analysis, three proteins related to the insulin network were defined: NOV, PRDX5, and HGFL. The circulating NOV level is reported to be positively correlated with HbA1c, body mass index (BMI) and fat mass (33). NOV, which is highly expressed in smooth muscle cells of the arterial vessel wall (34), inhibits β-cell proliferation via different mechanisms to impair β-cell insulin secretion (35). Therefore, the upregulation of NOV in the early trimester might be related to β cells. However, NOV has also been reported to be a proangiogenically secreted molecule involved in placental development, and is increasingly expressed in the placenta and serum during normal pregnancy. NOV recovers to normal levels during normal pregnancy (36). PRDX5, a member of the family of antioxidant enzyme, participates in eliminating hydrogen peroxide and neutralizing other ROS (37). PRDX5 has been found to significantly prevent high glucose-induced apoptotic cell death, and it has been reported as an early sign of retinal pathology in diabetic patients (38). In the first trimester of pregnancy, when maternal–fetal blood circulation has only just been established, the oxygen concentration increases rapidly in the placenta, leading to an increase in oxidative stress and the subsequent activation of the antioxidant enzymes to protect against oxidative stress. The generation of ROS causes a decrease in endogenous antioxidants. Therefore, the early downregulation of PRDX5in patients with GDM might be related to its overconsumption in the process of protecting against oxidative stress. During the second and third trimesters of GDM pregnancy, the consumption of PRDX5 of GDM and normal pregnancy was similar and showed the similar expression level (39-41). HGF, a vital component of insulin resistance pathophysiology, plays roles in β-cell homeostasis, inflammatory response mediation, and the glucose metabolic flux in diverse insulin-sensitive cell types (42). Increased levels of HGF are associated with the occurrence of insulin resistance, and HGF has been regarded as a serum biomarker of macroangiopathy in DM (43). The decrease of HGF during the third trimester of GDM pregnancy might reflect a clinical treatment effect. The above three urinary proteins have not been reported in previous urinary proteomic studies of GDM, but all of them have been reported to play roles in the pathogenesis of insulin resistance or the inflammatory response. Therefore, changes in the protein panel might be used for the early diagnosis of GDM.
The urinary proteome could potentially be used for early diagnosis of SA
According to previous reports, the causes of SA are multifactorial. In our study, analysis of differential urinary proteins showed that the synaptogenesis signaling, nNOS signaling, and superoxide radical degradation pathways were the top canonical pathways involved (Figure 4C). According to IPA annotations, synaptogenesis signaling and nNOS signaling play important roles in the brain and skeletal muscle, while superoxide radical degradation signaling is related to cell death and survival. Estrogen receptor alpha (ESR1) and estrogen receptor beta (ESR2), two major isoforms of estrogen receptors, act directly or indirectly on these differentially expressed molecules. Estrogen modulates multiple reproductive functions, including progesterone production and uteroplacental blood flow (44), and is thus important for fertilization and gestation. Some polymorphisms in the ESR1 gene, such as PvuII and XbaI, have been linked to increased abortion risk (45). However, the relationships between gene polymorphisms in ESR2 and abortion still need further research.
Four proteins (GDF-15, GNAQ, GBB1, and GNAI3) that were validated by PRM in the current study with high confidence are modulated by ESR1 and ESR2 (Figure 4B). GDF-15, which modulates food intake, energy consumption, and body weight when metabolic and toxin-induced stress increases (46), was elevated in all of the SA samples this study. This suggests that the metabolic and stress pathway may play important roles in SA. Oxidative stress-induced placental dysfunction has been put forward as a common cause of multifactorial and polygenic etiologies of abortion (47). GNAQ, GNAI3, and GBB1 are subunits of guanine nucleotide-binding protein G (G proteins) which are involved as modulating or transduction proteins in various transmembrane signaling cascades (48). Also, G-protein coupled receptors, in response to hormones, neurotransmitters, and environmental stimulants, may serve as potential therapeutic targets for a broad spectrum of patients (49). The changes of growth/differentiation factor and G proteins in urine of SA patients may provide some clues for the causes such as metabolic/toxin-induced stresses, GTPase activity and G protein-effector interaction. However, these proteins need to be validated in future studies, and the causes of the SAs in participants in this study remain unknown.
Conclusions
In this study, a longitudinal and cross-sectional cohort urinary proteomic study of 84 pregnant women was conducted. Analysis of the urinary proteome in each trimester of normal pregnancy reflected the progression of pregnancy and can be utilized to understand the physiological changes that take place during pregnancy. The application of urinary proteome analysis in GDM and SA provide candidate biomarkers for the early diagnosis of GDM during the first trimester and clues as to the pathogenesis of SA. Therefore, the urinary proteome has the potential to reflect the changes with the progression of normal pregnancies, and our candidate biomarker panel may facilitate early diagnosis of pregnancy complications. With the advancement of proteomics technology, in-depth and high-throughput analysis will be provided more comprehensive data in the future. Multiomics studies with large sample sizes and multicenter clinical trials may provide more reliable results in the future.
Acknowledgments
Funding: This work was supported by the 13th Five-Year National Science and Technology Major Project for New Drugs (No. 2019ZX09734001), the National Natural Science Foundation of China (No. 82170524,31901039), the Beijing Science Program for the Top Young (No. 2015000021223TD04), the Peking Union Medical College Hospital (No. 2016-2.27), the Beijing Hospital (No. BJ-2019-136), the CAMS Innovation Fund for Medical Sciences (No. 2021-1-I2M-016), Beijing Medical Research (No.2018-7), and the Biologic Medicine Information Center of China, National Scientific Data Sharing Platform for the Population and Health.
Footnote
Reporting Checklist: The authors have completed the STROBE reporting checklist. Available at https://atm.amegroups.com/article/view/10.21037/atm-21-3497/rc
Peer Review File: Available at https://atm.amegroups.com/article/view/10.21037/atm-21-3497/prf
Data Sharing Statement: Available at https://atm.amegroups.com/article/view/10.21037/atm-21-3497/dss
Conflicts of Interest: All authors have completed the ICMJE uniform disclosure form (available at https://atm.amegroups.com/article/view/10.21037/atm-21-3497/coif). The authors have no conflicts of interest to declare.
Ethical Statement: The authors are accountable for all aspects of the work in ensuring that questions related to the accuracy or integrity of any part of the work are appropriately investigated and resolved. The study was conducted in accordance with the Declaration of Helsinki (as revised in 2013). The study was approved by the Ethics Committee of Peking Union Medical College Hospital (No. ZS-976), and informed consent was obtained from all participants.
Open Access Statement: This is an Open Access article distributed in accordance with the Creative Commons Attribution-NonCommercial-NoDerivs 4.0 International License (CC BY-NC-ND 4.0), which permits the non-commercial replication and distribution of the article with the strict proviso that no changes or edits are made and the original work is properly cited (including links to both the formal publication through the relevant DOI and the license). See: https://creativecommons.org/licenses/by-nc-nd/4.0/.
References
- Flood-Nichols SK, Tinnemore D, Wingerd MA, et al. Longitudinal analysis of maternal plasma apolipoproteins in pregnancy: a targeted proteomics approach. Mol Cell Proteomics 2013;12:55-64. [Crossref] [PubMed]
- Carlin A, Alfirevic Z. Physiological changes of pregnancy and monitoring. Best Pract Res Clin Obstet Gynaecol 2008;22:801-23. [Crossref] [PubMed]
- Zheng J, Liu L, Wang J, et al. Urinary proteomic and non-prefractionation quantitative phosphoproteomic analysis during pregnancy and non-pregnancy. BMC Genomics 2013;14:777. [Crossref] [PubMed]
- Romero R, Erez O, Maymon E, et al. The maternal plasma proteome changes as a function of gestational age in normal pregnancy: a longitudinal study. Am J Obstet Gynecol 2017;217:67.e1-67.e21. [Crossref] [PubMed]
- Bruschi M, Santucci L, Ravera S, et al. Metabolic Signature of Microvesicles from Umbilical Cord Mesenchymal Stem Cells of Preterm and Term Infants. Proteomics Clin Appl 2018;12:e1700082. [Crossref] [PubMed]
- Liu X, Song Y, Guo Z, et al. A comprehensive profile and inter-individual variations analysis of the human normal amniotic fluid proteome. J Proteomics 2019;192:1-9. [Crossref] [PubMed]
- Gao Y. Urine-an untapped goldmine for biomarker discovery? Sci China Life Sci 2013;56:1145-6. [Crossref] [PubMed]
- Wu J, Gao Y. Physiological conditions can be reflected in human urine proteome and metabolome. Expert Rev Proteomics 2015;12:623-36. [Crossref] [PubMed]
- Chen G, Zhang Y, Jin X, et al. Urinary proteomics analysis for renal injury in hypertensive disorders of pregnancy with iTRAQ labeling and LC-MS/MS. Proteomics Clin Appl 2011;5:300-10. [Crossref] [PubMed]
- Guo HX, Zhu YB, Wu CP, et al. Potential urine biomarkers for gestational hypertension and preeclampsia. Mol Med Rep 2019;19:2463-70. [Crossref] [PubMed]
- Kononikhin AS, Starodubtseva NL, Bugrova AE, et al. An untargeted approach for the analysis of the urine peptidome of women with preeclampsia. J Proteomics 2016;149:38-43. [Crossref] [PubMed]
- Carty DM, Siwy J, Brennand JE, et al. Urinary proteomics for prediction of preeclampsia. Hypertension 2011;57:561-9. [Crossref] [PubMed]
- Ramachandrarao SP, Hamlin AA, Awdishu L, et al. Proteomic analyses of Urine Exosomes reveal New Biomarkers of Diabetes in Pregnancy. Madridge J Diabetes 2016;1:11-22. [Crossref] [PubMed]
- Guo Y, Han Z, Guo L, et al. Identification of urinary biomarkers for the prediction of gestational diabetes mellitus in early second trimester of young gravidae based on iTRAQ quantitative proteomics. Endocr J 2018;65:727-35. [Crossref] [PubMed]
- Coustan DR. Gestational diabetes mellitus. Clin Chem 2013;59:1310-21. [Crossref] [PubMed]
- American Diabetes Association. Executive summary: standards of medical care in diabetes--2011. Diabetes Care 2011;34:S4-10. [Crossref] [PubMed]
- Griebel CP, Halvorsen J, Golemon TB, et al. Management of spontaneous abortion. Am Fam Physician 2005;72:1243-50. [PubMed]
- Morales C, Sánchez A, Bruguera J, et al. Cytogenetic study of spontaneous abortions using semi-direct analysis of chorionic villi samples detects the broadest spectrum of chromosome abnormalities. Am J Med Genet A 2008;146A:66-70. [Crossref] [PubMed]
- Wiśniewski JR, Zougman A, Nagaraj N, et al. Universal sample preparation method for proteome analysis. Nat Methods 2009;6:359-62. [Crossref] [PubMed]
- Liu Y, Buil A, Collins BC, et al. Quantitative variability of 342 plasma proteins in a human twin population. Mol Syst Biol 2015;11:786. [Crossref] [PubMed]
- Zhao M, Liu X, Sun H, et al. Evaluation of Urinary Proteome Library Generation Methods on Data-Independent Acquisition MS Analysis and its Application in Normal Urinary Proteome Analysis. Proteomics Clin Appl 2019;13:e1800152. [Crossref] [PubMed]
- MacLean B, Tomazela DM, Shulman N, et al. Skyline: an open source document editor for creating and analyzing targeted proteomics experiments. Bioinformatics 2010;26:966-8. [Crossref] [PubMed]
- Jiang WG, Ye L, Ablin RJ, et al. The prostate transglutaminase, TGase-4, coordinates with the HGFL/MSP-RON system in stimulating the migration of prostate cancer cells. Int J Oncol 2010;37:413-8. [Crossref] [PubMed]
- Hirota Y, Osuga Y, Hasegawa A, et al. Interleukin (IL)-1beta stimulates migration and survival of first-trimester villous cytotrophoblast cells through endometrial epithelial cell-derived IL-8. Endocrinology 2009;150:350-6. [Crossref] [PubMed]
- Ghedin E, Schultz-Cherry S. Host response: Pregnancy impairs flu defences. Nat Microbiol 2017;2:17077. [Crossref] [PubMed]
- Lacey HA, Nolan T, Greenwood SL, et al. Gestational profile of Na+/H+ exchanger and Cl-/HCO3- anion exchanger mRNA expression in placenta using real-time QPCR. Placenta 2005;26:93-8. [Crossref] [PubMed]
- Burke N, Flood K, Murray A, et al. Platelet reactivity changes significantly throughout all trimesters of pregnancy compared with the nonpregnant state: a prospective study. BJOG 2013;120:1599-604. [Crossref] [PubMed]
- Catalano PM, Huston L, Amini SB, et al. Longitudinal changes in glucose metabolism during pregnancy in obese women with normal glucose tolerance and gestational diabetes mellitus. Am J Obstet Gynecol 1999;180:903-16. [Crossref] [PubMed]
- Yu T, Sheu SS, Robotham JL, et al. Mitochondrial fission mediates high glucose-induced cell death through elevated production of reactive oxygen species. Cardiovasc Res 2008;79:341-51. [Crossref] [PubMed]
- Liu K, Paterson AJ, Chin E, et al. Glucose stimulates protein modification by O-linked GlcNAc in pancreatic beta cells: linkage of O-linked GlcNAc to beta cell death. Proc Natl Acad Sci U S A 2000;97:2820-5. [Crossref] [PubMed]
- Kenna LA, Olsen JA, Spelios MG, et al. β-Cell death is decreased in women with gestational diabetes mellitus. Diabetol Metab Syndr 2016;8:60. [Crossref] [PubMed]
- Visiedo F, Bugatto F, Quintero-Prado R, et al. Glucose and Fatty Acid Metabolism in Placental Explants From Pregnancies Complicated With Gestational Diabetes Mellitus. Reprod Sci 2015;22:798-801. [Crossref] [PubMed]
- Pakradouni J, Le Goff W, Calmel C, et al. Plasma NOV/CCN3 levels are closely associated with obesity in patients with metabolic disorders. PLoS One 2013;8:e66788. [Crossref] [PubMed]
- Lin CG, Leu SJ, Chen N, et al. CCN3 (NOV) is a novel angiogenic regulator of the CCN protein family. J Biol Chem 2003;278:24200-8. [Crossref] [PubMed]
- Martinerie C, Garcia M, Do TT, et al. NOV/CCN3: A New Adipocytokine Involved in Obesity-Associated Insulin Resistance. Diabetes 2016;65:2502-15. [Crossref] [PubMed]
- Gellhaus A, Schmidt M, Dunk C, et al. The circulating proangiogenic factors CYR61 (CCN1) and NOV (CCN3) are significantly decreased in placentae and sera of preeclamptic patients. Reprod Sci 2007;14:46-52. [Crossref] [PubMed]
- Kropotov A, Usmanova N, Serikov V, et al. Mitochondrial targeting of human peroxiredoxin V protein and regulation of PRDX5 gene expression by nuclear transcription factors controlling biogenesis of mitochondria. FEBS J 2007;274:5804-14. [Crossref] [PubMed]
- Kubo E, Singh DP, Fatma N, et al. TAT-mediated peroxiredoxin 5 and 6 protein transduction protects against high-glucose-induced cytotoxicity in retinal pericytes. Life Sci 2009;84:857-64. [Crossref] [PubMed]
- Jauniaux E, Watson AL, Hempstock J, et al. Onset of maternal arterial blood flow and placental oxidative stress. A possible factor in human early pregnancy failure. Am J Pathol 2000;157:2111-22. [Crossref] [PubMed]
- Myatt L, Cui X. Oxidative stress in the placenta. Histochem Cell Biol 2004;122:369-82. [Crossref] [PubMed]
- Houstis N, Rosen ED, Lander ES. Reactive oxygen species have a causal role in multiple forms of insulin resistance. Nature 2006;440:944-8. [Crossref] [PubMed]
- Oliveira AG, Araújo TG, Carvalho BM, et al. The Role of Hepatocyte Growth Factor (HGF) in Insulin Resistance and Diabetes. Front Endocrinol (Lausanne) 2018;9:503. [Crossref] [PubMed]
- Konya H, Miuchi M, Satani K, et al. Hepatocyte growth factor, a biomarker of macroangiopathy in diabetes mellitus. World J Diabetes 2014;5:678-88. [Crossref] [PubMed]
- Pepe GJ, Albrecht ED. Actions of placental and fetal adrenal steroid hormones in primate pregnancy. Endocr Rev 1995;16:608-48. [PubMed]
- Pineda B, Hermenegildo C, Tarín JJ, et al. Alleles and haplotypes of the estrogen receptor alpha gene are associated with an increased risk of spontaneous abortion. Fertil Steril 2010;93:1809-15. [Crossref] [PubMed]
- Hsu JY, Crawley S, Chen M, et al. Non-homeostatic body weight regulation through a brainstem-restricted receptor for GDF15. Nature 2017;550:255-9. [Crossref] [PubMed]
- Gupta S, Agarwal A, Banerjee J, et al. The role of oxidative stress in spontaneous abortion and recurrent pregnancy loss: a systematic review. Obstet Gynecol Surv 2007;62:335-47; quiz 353-4. [Crossref] [PubMed]
- Neves SR, Ram PT, Iyengar R. G protein pathways. Science 2002;296:1636-9. [Crossref] [PubMed]
- Rosenbaum DM, Rasmussen SG, Kobilka BK. The structure and function of G-protein-coupled receptors. Nature 2009;459:356-63. [Crossref] [PubMed]