Surface-based morphological patterns associated with neuropsychological performance, symptom severity, and treatment response in Parkinson’s disease
Introduction
Parkinson’s disease (PD) is a chronic disabling neurodegenerative disorder clinically characterized by tremor, rigidity, akinesia, and postural instability. PD is caused mainly by dopaminergic neuronal degeneration in the substantia nigra (1,2). Aside from the motor-related symptoms, decreased emotional and cognitive social processes are also commonly observed in PD and are often manifest as behavioral disorders (3). Further, depression, anosmia, constipation, excessive daytime sleepiness, rapid eye movement sleep behavior disorder, visual changes, and cognitive changes may also be present during the prodromal stages of PD (4,5). Levodopa and other dopamine agonists are used as dopamine-replacement therapies resulting in effective relief of motor symptoms in the early stages of the disease. However, this treatment is eventually impeded by the increasing occurrence of motor complications, hyperkinesia, and sudden on-off phenomena associated with the feeling that levodopa is “wearing off” earlier and earlier each dose (6). In these patients, deep brain stimulation (DBS) provides a promising means of relief from these symptoms. The effectiveness of DBS depends on patient selection based on multidisciplinary presurgical evaluation, in addition to stereotactic implantation and postoperative stimulator programming.
Although the neurobiological mechanisms of PD remain inconclusive, the advent of high-resolution magnetic resonance imaging (MRI) in recent years has prompted the undertaking of neuroimaging studies that have provided evidence for PD being associated with morphological changes in the brain, particularly in gray matter volume (GMV) (7). Pereira et al. reported that in comparison to controls, patients with PD displayed cortical thinning in the right lateral occipital, parietal, and left temporal, frontal, and premotor regions (8). A voxel-wise meta-analysis of studies using voxel-based morphometry (VBM) was conducted to explore consistent GMV changes in PD and found significant reductions in GMV in the basal ganglia, and networks associated with theory of mind, vocalization, and vision (7). These studies have associated specific neuroimaging alterations with a diagnosis of PD. Further, studies have used machine learning models to predict the effect of DBS on PD symptoms with satisfactory results (9). However, VBM only assesses the GMV, while the shape of the cortical surface provides useful information reflecting cortical organization that can be used to better understand the neuroanatomical changes occurring in patients with PD.
The human cerebral cortex is highly convoluted with gyri and sulci. The integrity of the surface is dependent on the neurobiological processes occurring within and represents the development and degeneration of the brain. A series of structural indicators are used to investigate changes in the shape of the surface in patients with PD. PD has been associated with significantly reduced overall gyrification, most notably in bilateral inferior parietal, precentral, postcentral, superior frontal, and supramarginal areas. This effect may be accelerated within the early stages (<1 year) of the disease (10). However, the joint effects of PD on surface-based cortical morphology remain unsubstantiated as a single feature cannot be used to reflect a more complete cortical aberrance. Previous work has used regional GM changes and age to predict an individual’s treatment response (11), while the predictive accuracy and the binary outcomes design could be further improved.
Therefore, the aim of the present study was to (I) perform a comprehensive analysis of alterations in cortical surface-based morphology associated with PD, (II) explore the associations between the neuroimaging pattern of PD and clinical assessment (neuropsychological changes and motor symptoms), and (III) construct a machine learning model to predict the treatment response to medication and DBS based on changes in the neuroimaging pattern. The findings from this study will be valuable in guiding clinical strategy, for example, in demonstrating that cortical surface-based morphological measures are conducive to increasing our understanding of the neural mechanisms of PD. Further, this can be used to explore the relationships between neuroimaging patterns and clinical assessment measures. These measures can then further be considered in the identification of diagnosis biomarkers, potential treatment targets, and presurgical prognosis to improve the surgical candidacy criteria. We present the following article in accordance with the STROBE reporting checklist (available at https://atm.amegroups.com/article/view/10.21037/atm-22-630/rc).
Methods
Participants
The study was conducted in accordance with the Declaration of Helsinki (as revised in 2013). This study was approved by the Ethics Committee at the Beijing Tiantan Hospital (KYSQ2018-172-01). All patients and control participants provided informed consent for access to their medical records.
In this case-control study, consecutive patients with PD and healthy control participants were retrospectively collected from the medical database of Beijing Tiantan Hospital between January 2018 and December 2020. Participants’ clinical information, including their age at PD onset, duration of PD, Hoehn and Yahr (H-Y) scale scores, and levodopa equivalent daily dosage (LEDD) were also collected from their medical records.
All patients underwent a conventional presurgical examination including emotional assessment using the Hamilton anxiety scale (HAMA-14) (12) and Hamilton rating scale for depression (HRSD-24) (13), assessment of cognitive impairment using the mini-mental state examination (MMSE) (14), and the Montreal cognitive assessment (MoCA) (15). For participants not receiving either medication or DBS (medication-off, DBS-off status), motor-related symptoms were assessed using the Movement Disorder Society-sponsored revision of the unified Parkinson’s disease rating scale (MDS-UPDRS) (16), including the UPDRS-III total score, Tremor score (the average score of items 3.15–3.17), Akinetic/rigidity score (the average score of items 3.10–3.12), and the postural instability and gait disorders (PIGD) score (the average score of items 3.3–3.9 and item 3.14) in the medication-off, DBS-off status. Patients underwent surgical DBS implantation and started to receive stimulation one month after their surgery. DBS patients were then re-evaluated three months after surgery (medication-off, DBS-on status). All healthy control participants were free of any significant neurological history.
MRI acquisition and image processing
Magnetic resonance images were acquired on a 3.0-T Siemens Verio scanner using a 3-dimensional T1-weighted magnetization-prepared rapid acquisition with gradient echo (T1w MPRAGE) sequence, as follows: repetition time (TR) =2,300 ms, echo time (TE) =2.53 ms, flip angle =12°, slice thickness =1 mm, no slice gap, voxel size =1.0 mm × 1.0 mm × 1.0 mm (17,18).
The surface-based analysis was performed using the Computational Anatomy Toolbox (CAT12) (http://dbm.neuro.uni-jena.de/cat/) that provides a fully automated method of estimating cortical thickness and the central surface of each hemisphere based on the projection-based thickness method (19). The processing pipeline included automated brain segmentation into gray matter (GM), white matter (WM), and cerebrospinal fluid (CSF), affine registration to MNI-template space, and subsequent nonlinear deformation. In CAT12, a new fully automated method allows the central surface to be reconstructed in one step. This projection-based thickness method also includes partial volume correction, sulcal blurring, and handles sulcal asymmetries without sulcus reconstruction. Newly created images were smoothed using a Gaussian smoothing kernel of 15 mm full-width half-maximum before the total intracranial volume was calculated. Surface extractions and topological defects were visually verified.
Computation of surface-based morphometry
- Cortical thickness: the thickness of the cortex reflects various cellular-level features including size, density, arrangement of neurons, neuroglia, and nerve fibers (20). Cortical thickness was measured using tissue segmentation to estimate the WM distance and project the local maxima to other GM voxels (thereby providing the cortical thickness) using a nearest-neighbor algorithm based on the WM distance (21).
- Sulcal depth: this accounts for both the depth and wideness of the cortical folding (22). The square root-transformed sulcal depth was extracted based on the Euclidean distance between the central surface and its convex hull and then transformed using the square root function.
- Gyrification index: the gyrification index reflects the folding geometry of the cortical surface. Lower values indicate sulcal widening induced by brain atrophy (23). Gyrification is calculated by estimating the ‘‘smoothed absolute mean curvature’’ of the cortical surface based on averaging curvature values from each vertex of the spherical surface mesh within a distance of 3 mm and then calculating the absolute value within this region (24).
- Fractal dimension: the fractal dimension is a quantification of the complexity of cortical folding at the vertex level. Surface complexity information was extracted using spherical harmonic (SPH) reconstructions (25).
Evaluation of the surface-based neuroimaging pattern
Univariate analysis was used to assess differences in sulcal depth, gyrification index, and fractal dimension between patients with PD and the healthy control group using a 2-tailed Student’s t-test at each vertex. As these features are all related to cortical morphological changes and may be affected by variations in cortical thickness, this metric was statistically adjusted at each vertex by the corresponding thickness measure (26).
The multivariate analysis evaluates the correlation/covariance of significance across brain regions, rather than proceeding on a voxel-by-voxel basis, making the interpretation of a signature of neural networks more straightforward (27). We thus used a general linear model with multivariate Hotelling’s t-test to determine the joint effect of surface-based shape differences between the two cohorts. In this explorative study, the correction for multiple comparison was not performed, but the significance threshold was set at a two-tailed threshold of P<0.01.
Regression association with neuroimaging pattern and clinical assessment
Multivariate linear regression was performed (28) using multivariate surface-based shape abnormalities (significant vertices in the calculated neuroimaging pattern) as a predictor for neurophysiological performance measures (HAMA, HRSD, MMSE, and MoCA) and clinical measures of motor symptoms (UPDRS-III total score, Tremor score, Akinetic/rigidity score, and PIGD score).
Prediction of medication and DBS response based on neuroimaging pattern
We evaluated the group-level results to predict the response to medication and DBS using a machine learning approach with a leave-one-out cross-validation (LOOCV) (29,30) method. The search space was confined to regions displaying group-level differences between PD patients and healthy controls. We then extracted mean sulcal depth, gyrification index, and fractal dimension scores from the significant cluster, and fed them into a support vector machine (SVM) classifier that evaluated the response to medication and DBS. We constructed the classification model to predict a good prognosis (improvement >50%) or lesser improvement, as well as the regression model of the actual score.
Statistical analysis
For univariate and multivariate analysis, the age and sex of patients and controls were included as covariates. For the multivariate linear regression model and prediction model, the age at PD onset, duration, H-Y scale, and LEDD were included as covariates. Moreover, to assess the model accuracy, the area under the receiver operating characteristic curve (AUC) was reported with 95% confidence intervals (CIs) to evaluate the performance of the classification model and the correlation coefficient to assess the regression model. Accuracy is a measure of the correct detection of the class labels (31), as follows:
A Kolmogorov-Smirnov test was used to test for normality of the continuous variables. Where data were normally-distributed, group differences were assessed using the two-sample t-test, whereas asymmetrically distributed variables were assessed using the Mann-Whitney U test. A Pearson chi-square test was used to assess group differences in categorical variables. Significant was considered at a two-tailed threshold of P<0.05. Demographic and clinical data were analyzed using IBM SPSS version 23.0 (SPSS, Chicago, IL, USA).
Results
Demographic information
The study recruited 78 patients with PD and 55 healthy controls who were comparable across groups for sex (female: patients =47.4%, healthy controls =52.7%; χ² =0.36, P=0.60) and age [mean ± standard deviation (SD): patients =60.4±9.7 years; healthy controls =58.7±5.6 years; t=1.36, P=0.18]. The mean age of PD onset was 51.6±10.5 years, mean PD duration 8.8±3.8 years, and mean LEDD 300.0±216.0 in PD patients. The median H-Y score was 3.5 (25th percentile =3.1, 75th percentile =4.0) (Table 1).
Table 1
Variables | Parkinson’s disease patients (n=78) | Healthy controls (n=55) | Statistic |
---|---|---|---|
Sex (female, %) | 37/78 (47.4%) | 29/55 (52.7%) | χ²=0.36, P=0.60 |
Age (yrs) | 60.4±9.7 | 58.7±5.6 | t=1.36, P=0.18 |
Age of onset (yrs) | 51.6±10.5 | – | |
Duration (yrs) | 8.8±3.8 | – | |
H-Y scale | 3.5 (3.1, 4.0) | – | |
LEDD | 300.0±216.0 | – |
Continuous data were represented using mean ± standard deviation (SD) values. H-Y scores were represented using median (25th percentile, 75th percentile). H-Y scale, Hoehn and Yahr scale; LEDD, levodopa equivalent daily dosage.
Results of the standard clinical assessment are given in Table 2, including emotion (HAMA =18.5±9.0 and HRSD =18.3±8.8), cognition (MMSE =25.5±4.5 and MoCA =21.4±6.0), and motor symptoms (UPDRS-III total score =48.9±19.0, tremor score =0.8±0.8, akinetic/rigidity score =1.7±0.7 and PIGD score =2.0±1.1) during their medication-off period. Of these, 30 received DBS treatment and displayed an improvement in motor symptoms at the revaluation three months after surgery (UPDRS-III total score =26.0±15.1, tremor score =0.4±0.5, akinetic/rigidity score =0.9±0.5 and PIGD score =1.0±0.9) (Table 2).
Table 2
Variables | Medication-off, DBS-off (n=78) | Medication-off, DBS-on (n=33) |
---|---|---|
HAMA | 18.5±9.0 | – |
HRSD | 18.3±8.8 | – |
MMSE | 25.5±4.5 | – |
MoCA | 21.4±6.0 | – |
UPDRS-III total score | 48.9±19.0 | 26.0±15.1 |
Tremor score | 0.8±0.8 | 0.4±0.5 |
Akinetic/rigidity score | 1.7±0.7 | 0.9±0.5 |
PIGD score | 2.0±1.1 | 1.0±0.9 |
Data are presented as mean ± standard deviation. DBS, deep brain stimulation; PD, Parkinson’s disease; HAMA, Hamilton anxiety scale; HRSD, Hamilton rating scale for depression; MMSE, mini-mental state examination; MoCA, Montreal cognitive assessment; UPDRS, unified Parkinson’s disease rating scale; PIGD, postural instability and gait disorders.
Evaluation of the surface-based neuroimaging pattern
Compared to the healthy control group, the PD patient group showed widespread decreased cortical surface folding and cortical complexity (P<0.01). The neuroimaging pattern across 3 surface-based features (sulcal depth, gyrification index, and fractal dimension) was similar, mainly presenting decreased abnormalities in the precentral gyrus, supplementary motor area (SMA), temporal neocortex, and occipital lobe. Scattered increased clusters were found in the frontal and insular lobes (Figure 1A). The multivariate analysis showed significant abnormalities (P<0.01) in bilateral precentral gyrus (left: F=4.32; right: F=4.13), insular lobe (left: F=4.87; right: F=4.53), paracentral lobe (left: F=4.01; right: F=4.26), left posterior cingulate cortex (PCC) (F=4.48), and left occipital lobe (F=4.27) (Figure 1B).
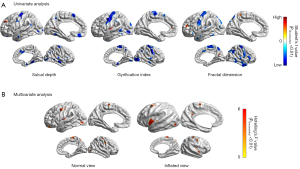
Association between neuroimaging pattern and clinical assessment
The results of the multivariate linear regression model showed that the surface-based features of the right frontal lobe were able to significantly predict the clinical cognitive assessment measure (MMSE, F=4.10). However, there were no other significant findings from the regression model involving any other neurophysiological performance measures (HAMA, HRSD, or MoCA) at the level of P<0.01 (Figure 2A and Figure S1A).
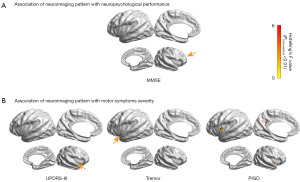
The neuroimaging pattern also showed a significant correlation with the severity of motor symptoms, with differences in the right insular being associated with the UPDRS-III total score (F=4.33), left insular differences being associated with the Tremor score (F=4.37), and differences in the bilateral insular (left: F=4.27; right: F=4.84) and left PCC (F=6.62) being associated with the PIGD score. There were no significant associations with the akinetic/rigidity score at the level of P<0.01 (Figure 2B and Figure S1B).
Prediction of response to medication and DBS based on neuroimaging patterns
To predict the response to medication, the classification model based on the neuroimaging pattern did not accurately assess the 4 different motor symptoms (41.0% for the UPDRS-III total score, 55.1% for the Tremor score, 48.7% for the Akinetic/Rigidity score, and 53.9% for the PIGD score). The regression model was, however, able to predict tremor and PIGD scores (R=−0.34, P<0.01; R=0.24, P=0.04, respectively) (Figure 3).
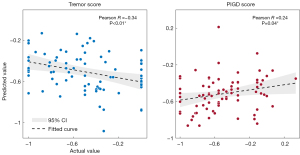
To predict improvement following DBS, the classification model was able to accurately predict an improvement in the tremor score in 60.6% of patients and an improvement in the akinetic/rigidity score in 69.7% of patients, but not to satisfactorily predict changes in the UPDURS-III score (51.5%) or the PIGD score (45.5%). The regression model failed to predict all 4 symptom measures: UPDRS-III total score (R=−0.14, P=0.21), Tremor score (R=0.18, P=0.35), Akinetic/Rigidity score (R=0.20, P=0.25), and PIGD score (R=−0.18, P=0.30) (Table 3).
Table 3
Motor symptoms severity | Drug response | DBS response | |||
---|---|---|---|---|---|
Classification model | Regression model | Classification model | Regression model | ||
UPDRS-III total score | 32/78 (41.0%) | R=0.04, P=0.70 | 17/33 (51.5%) | R=−0.14, P=0.21 | |
Tremor score | 43/78 (55.1%) | R=−0.34, P<0.01* | 20/33 (60.6%) | R=0.18, P=0.35 | |
Akinetic/rigidity score | 38/78 (48.7%) | R=−0.04, P=0.75 | 23/33 (69.7%) | R=0.20, P=0.25 | |
PIGD score | 42/78 (53.9%) | R=0.24, P=0.04* | 15/33 (45.5%) | R=−0.18, P=0.30 |
*: statistical significance. DBS, deep brain stimulation; UPDRS, unified Parkinson’s disease rating scale; PIGD, postural instability and gait disorders.
Discussion
Highlights
In the present study, we identified the surface-based neuroimaging pattern of PD, comprising decreased morphological metrics in the bilateral precentral gyrus, insular lobe, paracentral lobe, posterior cingulate cortex, and occipital lobe. We then found this neuroimaging pattern to be significantly related to clinical measures of cognitive performance and motor symptoms. Machine learning predictions based on morphological metrics were shown to have potential value in predicting the response to medication in the Tremor score and the PIGD score.
Evaluation of the surface-based neuroimaging pattern
Compared to healthy controls, PD patients showed widespread lower values of surface-based morphological metrics (sulcal depth, gyrification index, and fractal dimension) including in bilateral precentral gyrus, insular lobe, paracentral lobe, posterior cingulate cortex, and occipital lobe. These findings are in line with a previous voxel-wise meta-analysis of structural changes in PD (7), and also in agreement with Braak’s hypothesis of PD progression which states that the temporal lobe is the first cortical region to be affected by Lewy bodies, followed by the insula, prefrontal, parietal, and occipital lobes, and finally the primary sensory and motor areas (32). Our results showed brain network alterations based on surface-morphometry were similar to those based on voxel-based calculations. We then performed a multivariate analysis based on the joint distribution of 3 surface-based features to further identify the neuroimaging pattern of PD.
GMV decreases in the precentral gyrus have been reported previously, with a wider range compared to hypometabolism (33). Guimarães et al. reported cortical thinning in the precentral gyrus only in severe PD (H-Y scale 3–5) (34), which is in agreement with our findings as our cohort had a median H-Y scale of 3.5. Cortical changes associated with PD were not found to be confined to the nigrostriatal dopaminergic pathway but propagated along the cortico-basal ganglia-thalamocortical network. The primary motor cortex (M1) is a crucial node in this functional circuit as it generates neural impulses that regulate movement (35,36). The involvement of the insular lobe in PD was related to non-motor symptoms and the effect of medication (37). The insular lobe is a central hub involved in integrating diverse information for behavioral processes, and its dysfunction has been related to both cognitive and affective symptoms (38-40). The posterior cingulate cortex had been reportedly engaged in apathy and visual hallucinations (41,42), as well as dysfunction of the default mode network in patients with PD (43). Reduced γ-aminobutyric acid (GABA) in the primary visual cortex has also been associated with visual hallucinations in PD (44).
Association between neuroimaging pattern and clinical measures
Our results show a significant correlation between structural changes in the right frontal lobe and the MMSE, but no findings related to other neurophysiological measures of performance (HAMA, HRSD, or MoCA). The MMSE score declined at a greater rate when associated with frontotemporal degeneration (FTD) (45). However, previous findings have conflicted with this, reporting that the MMSE was intended as of gross measure of cognitive status rather than a measure of frontal lobe function (46).
Our results also revealed significant associations between structural changes in the insula and UPDRS-III total score, Tremor score, and PIGD score, and changes in the PCC that were associated with the PIGD score. The insular cortex may play a key role in specific symptoms such as impaired working memory, postural instability, and autonomic dysfunction (47). However, as discussed above, the insular lobe and PCC are crucial in the modulation of non-motor symptoms (48). These results will need to be validated in future studies.
Prediction in drug response and DBS prognosis based on neuroimaging pattern
We constructed 4 machine learning models based on the neuroimaging pattern (2 classification models and 2 regression models) to predict the response to treatment with medication and DBS. The response to medication in the Tremor score and PIGD score were successfully predicted. Most previous studies have focused on DBS programming optimization and surgical targeting (49). But few studies have explored the treatment effect based on the neuroimaging pattern. The present study has been able to address this gap. Shamir et al. developed a clinical decision support system based on clinical information to assist in treatment optimization, achieving an accuracy of 86% (12/14) (50). Another study used functional MRI to provide a brain response pattern which was able to accurately (88%) predict the optimal parameters for deep brain stimulation (51). Our results suggest that surface-based features have potential value in predicting a treatment effect in patients with PD.
Limitations
This study has some limitations that need to be mentioned. Firstly, we measured morphological indicators of cortical surface structure in patients with PD. However, we cannot truly determine any causal relationship between changes in these cortical characteristics and the symptoms of PD. Secondly, when making comparisons between participant groups, the significance of the results was not corrected for multiple comparisons such that we were able to detect and report all relevant findings.
Conclusions
In conclusion, this is the first comprehensive investigation of sulcal depth, gyrification index, and fractal dimension in PD patients. Compared to healthy controls, patients with PD had decreased surface-based morphological metrics in the bilateral precentral gyrus, insular lobe, paracentral lobe, posterior cingulate cortex, and occipital lobe. Cortical morphological indicators were found to be associated with clinical neuropsychological measures and motor symptoms. Finally, we have demonstrated the potential value of using these neuroimaging patterns to predict the treatment responses. These results may provide important insight into the neurophysiological mechanisms of PD as well as inform the assessment of surgical candidacy.
Acknowledgments
The authors would like to thank all the reviewers who participated in the review. The authors also would like to thank MJEditor (www.mjeditor.com) for its linguistic assistance during the preparation of this manuscript.
Funding: This work was sponsored by the National Key R&D Program of China (2021YFC2401201), Capital’s Funds for Health Improvement and Research (2022-1-1071, 2020-2-1076), and National Natural Science Foundation of China (82071457).
Footnote
Reporting Checklist: The authors have completed the STROBE reporting checklist. Available at https://atm.amegroups.com/article/view/10.21037/atm-22-630/rc
Data Sharing Statement: Available at https://atm.amegroups.com/article/view/10.21037/atm-22-630/dss
Conflicts of Interest: All authors have completed the ICMJE uniform disclosure form (available at https://atm.amegroups.com/article/view/10.21037/atm-22-630/coif). The authors have no conflicts of interest to declare.
Ethical Statement: The authors are accountable for all aspects of the work in ensuring that questions related to the accuracy or integrity of any part of the work are appropriately investigated and resolved. The study was conducted in accordance with the Declaration of Helsinki (as revised in 2013). The study was approved by the Ethics Committee at the Beijing Tiantan Hospital (KYSQ2018-172-01). All patients and control participants provided informed consent for access to their medical records.
Open Access Statement: This is an Open Access article distributed in accordance with the Creative Commons Attribution-NonCommercial-NoDerivs 4.0 International License (CC BY-NC-ND 4.0), which permits the non-commercial replication and distribution of the article with the strict proviso that no changes or edits are made and the original work is properly cited (including links to both the formal publication through the relevant DOI and the license). See: https://creativecommons.org/licenses/by-nc-nd/4.0/.
References
- Bloem BR, Okun MS, Klein C. Parkinson’s disease. Lancet 2021;397:2284-303. [Crossref] [PubMed]
- Groiss SJ, Wojtecki L, Südmeyer M, et al. Deep brain stimulation in Parkinson’s disease. Ther Adv Neurol Disord 2009;2:20-8. [Crossref] [PubMed]
- Narme P, Mouras H, Roussel M, et al. Emotional and cognitive social processes are impaired in Parkinson’s disease and are related to behavioral disorders. Neuropsychology 2013;27:182-92. [Crossref] [PubMed]
- Aarsland D, Batzu L, Halliday GM, et al. Parkinson disease-associated cognitive impairment. Nat Rev Dis Primers 2021;7:47. [Crossref] [PubMed]
- Getz SJ, Levin B. Cognitive and Neuropsychiatric Features of Early Parkinson’s Disease. Arch Clin Neuropsychol 2017;32:769-85. [Crossref] [PubMed]
- Goetz CG, Poewe W, Rascol O, et al. Evidence-based medical review update: pharmacological and surgical treatments of Parkinson’s disease: 2001 to 2004. Mov Disord 2005;20:523-39. [Crossref] [PubMed]
- Xu X, Han Q, Lin J, et al. Grey matter abnormalities in Parkinson’s disease: a voxel-wise meta-analysis. Eur J Neurol 2020;27:653-9. [Crossref] [PubMed]
- Pereira JB, Ibarretxe-Bilbao N, Marti MJ, et al. Assessment of cortical degeneration in patients with Parkinson’s disease by voxel-based morphometry, cortical folding, and cortical thickness. Hum Brain Mapp 2012;33:2521-34. [Crossref] [PubMed]
- Chen Y, Zhu G, Liu D, et al. Brain morphological changes in hypokinetic dysarthria of Parkinson’s disease and use of machine learning to predict severity. CNS Neurosci Ther 2020;26:711-9. [Crossref] [PubMed]
- Sterling NW, Wang M, Zhang L, et al. Stage-dependent loss of cortical gyrification as Parkinson disease "unfolds". Neurology 2016;86:1143-51. [Crossref] [PubMed]
- Ballarini T, Mueller K, Albrecht F, et al. Regional gray matter changes and age predict individual treatment response in Parkinson’s disease. Neuroimage Clin 2019;21:101636. [Crossref] [PubMed]
- HAMILTON M. The assessment of anxiety states by rating. Br J Med Psychol 1959;32:50-5. [Crossref] [PubMed]
- HAMILTON M.. A rating scale for depression. J Neurol Neurosurg Psychiatry 1960;23:56-62. [Crossref] [PubMed]
- Folstein MF, Folstein SE, McHugh PR. "Mini-mental state". A practical method for grading the cognitive state of patients for the clinician. J Psychiatr Res 1975;12:189-98. [Crossref] [PubMed]
- Nasreddine ZS, Phillips NA, Bédirian V, et al. The Montreal Cognitive Assessment, MoCA: a brief screening tool for mild cognitive impairment. J Am Geriatr Soc 2005;53:695-9. [Crossref] [PubMed]
- Goetz CG, Tilley BC, Shaftman SR, et al. Movement Disorder Society-sponsored revision of the Unified Parkinson’s Disease Rating Scale (MDS-UPDRS): scale presentation and clinimetric testing results. Mov Disord 2008;23:2129-70. [Crossref] [PubMed]
- Mo J, Zhang J, Hu W, et al. Whole-brain morphological alterations associated with trigeminal neuralgia. J Headache Pain 2021;22:95. [Crossref] [PubMed]
- Mo J, Zhao B, Adler S, et al. Quantitative assessment of structural and functional changes in temporal lobe epilepsy with hippocampal sclerosis. Quant Imaging Med Surg 2021;11:1782-95. [Crossref] [PubMed]
- Dahnke R, Yotter RA, Gaser C. Cortical thickness and central surface estimation. Neuroimage 2013;65:336-48. [Crossref] [PubMed]
- Sowell ER, Thompson PM, Leonard CM, et al. Longitudinal mapping of cortical thickness and brain growth in normal children. J Neurosci 2004;24:8223-31. [Crossref] [PubMed]
- Mo J, Wei W, Liu Z, et al. Neuroimaging Phenotyping and Assessment of Structural-Metabolic-Electrophysiological Alterations in the Temporal Neocortex of Focal Cortical Dysplasia IIIa. J Magn Reson Imaging 2021;54:925-35. [Crossref] [PubMed]
- Lyu I, Kang H, Woodward ND, et al. Sulcal Depth-based Cortical Shape Analysis in Normal Healthy Control and Schizophrenia Groups. Proc SPIE Int Soc Opt Eng 2018;10574:1057402.
- Choi M, Youn H, Kim D, et al. Comparison of neurodegenerative types using different brain MRI analysis metrics in older adults with normal cognition, mild cognitive impairment, and Alzheimer’s dementia. PLoS One 2019;14:e0220739. [Crossref] [PubMed]
- Luders E, Thompson PM, Narr KL, et al. A curvature-based approach to estimate local gyrification on the cortical surface. Neuroimage 2006;29:1224-30. [Crossref] [PubMed]
- Yotter RA, Nenadic I, Ziegler G, et al. Local cortical surface complexity maps from spherical harmonic reconstructions. Neuroimage 2011;56:961-73. [Crossref] [PubMed]
- Glasser MF, Van Essen DC. Mapping human cortical areas in vivo based on myelin content as revealed by T1- and T2-weighted MRI. J Neurosci 2011;31:11597-616. [Crossref] [PubMed]
- Habeck CG. Basics of multivariate analysis in neuroimaging data. J Vis Exp 2010;1988. [Crossref] [PubMed]
- Chung MK, Worsley KJ, Nacewicz BM, et al. General multivariate linear modeling of surface shapes using SurfStat. Neuroimage 2010;53:491-505. [Crossref] [PubMed]
- Mo J, Liu Z, Sun K, et al. Automated detection of hippocampal sclerosis using clinically empirical and radiomics features. Epilepsia 2019;60:2519-29. [Crossref] [PubMed]
- Mo JJ, Zhang JG, Li WL, et al. Clinical Value of Machine Learning in the Automated Detection of Focal Cortical Dysplasia Using Quantitative Multimodal Surface-Based Features. Front Neurosci 2019;12:1008. [Crossref] [PubMed]
- Zhang H, Mo J, Jiang H, et al. Deep Learning Model for the Automated Detection and Histopathological Prediction of Meningioma. Neuroinformatics 2021;19:393-402. [Crossref] [PubMed]
- Braak H, Del Tredici K, Rüb U, et al. Staging of brain pathology related to sporadic Parkinson’s disease. Neurobiol Aging 2003;24:197-211. [Crossref] [PubMed]
- González-Redondo R, García-García D, Clavero P, et al. Grey matter hypometabolism and atrophy in Parkinson’s disease with cognitive impairment: a two-step process. Brain 2014;137:2356-67. [Crossref] [PubMed]
- Guimarães RP, Arci Santos MC, Dagher A, et al. Pattern of Reduced Functional Connectivity and Structural Abnormalities in Parkinson’s Disease: An Exploratory Study. Front Neurol 2016;7:243. [PubMed]
- Burciu RG, Vaillancourt DE. Imaging of Motor Cortex Physiology in Parkinson’s Disease. Mov Disord 2018;33:1688-99. [Crossref] [PubMed]
- Underwood CF, Parr-Brownlie LC. Primary motor cortex in Parkinson’s disease: Functional changes and opportunities for neurostimulation. Neurobiol Dis 2021;147:105159. [Crossref] [PubMed]
- Criaud M, Christopher L, Boulinguez P, et al. Contribution of insula in Parkinson’s disease: A quantitative meta-analysis study. Hum Brain Mapp 2016;37:1375-92. [Crossref] [PubMed]
- Christopher L, Koshimori Y, Lang AE, et al. Uncovering the role of the insula in non-motor symptoms of Parkinson’s disease. Brain 2014;137:2143-54. [Crossref] [PubMed]
- Jonkman LE, Fathy YY, Berendse HW, et al. Structural network topology and microstructural alterations of the anterior insula associate with cognitive and affective impairment in Parkinson’s disease. Sci Rep 2021;11:16021. [Crossref] [PubMed]
- Zhao B, Seguin C, Ai L, et al. Aberrant Metabolic Patterns Networks in Insular Epilepsy. Front Neurol 2020;11:605256. [Crossref] [PubMed]
- Zhan ZW, Lin LZ, Yu EH, et al. Abnormal resting-state functional connectivity in posterior cingulate cortex of Parkinson’s disease with mild cognitive impairment and dementia. CNS Neurosci Ther 2018;24:897-905. [Crossref] [PubMed]
- Vogt BA. Cingulate cortex in Parkinson’s disease. Handb Clin Neurol 2019;166:253-66. [Crossref] [PubMed]
- van Eimeren T, Monchi O, Ballanger B, et al. Dysfunction of the default mode network in Parkinson disease: a functional magnetic resonance imaging study. Arch Neurol 2009;66:877-83. [Crossref] [PubMed]
- Firbank MJ, Parikh J, Murphy N, et al. Reduced occipital GABA in Parkinson disease with visual hallucinations. Neurology 2018;91:e675-85. [Crossref] [PubMed]
- Chow TW, Hynan LS, Lipton AM. MMSE scores decline at a greater rate in frontotemporal degeneration than in AD. Dement Geriatr Cogn Disord 2006;22:194-9. [Crossref] [PubMed]
- Axelrod BN, Goldman RS, Henry RR. Sensitivity of the Mini-Mental State Examination to frontal lobe dysfunction in normal aging. J Clin Psychol 1992;48:68-71. [Crossref] [PubMed]
- Kikuchi A, Takeda A, Kimpara T, et al. Hypoperfusion in the supplementary motor area, dorsolateral prefrontal cortex and insular cortex in Parkinson’s disease. J Neurol Sci 2001;193:29-36. [Crossref] [PubMed]
- Fathy YY, Hepp DH, de Jong FJ, et al. Anterior insular network disconnection and cognitive impairment in Parkinson’s disease. Neuroimage Clin 2020;28:102364. [Crossref] [PubMed]
- Watts J, Khojandi A, Shylo O, et al. Machine Learning’s Application in Deep Brain Stimulation for Parkinson’s Disease: A Review. Brain Sci 2020;10:809. [Crossref] [PubMed]
- Shamir RR, Dolber T, Noecker AM, et al. Machine Learning Approach to Optimizing Combined Stimulation and Medication Therapies for Parkinson’s Disease. Brain Stimul 2015;8:1025-32. [Crossref] [PubMed]
- Boutet A, Madhavan R, Elias GJB, et al. Predicting optimal deep brain stimulation parameters for Parkinson’s disease using functional MRI and machine learning. Nat Commun 2021;12:3043. [Crossref] [PubMed]