Single-cell transcriptomic analysis revealing changes in retinal cell subpopulation levels and the pathways involved in diabetic retinopathy
Introduction
Diabetes mellitus refers to a group of metabolic diseases involving chronic hyperglycemia, resulting from multiple causes, and its incidence has been increasing dramatically worldwide (1). Diabetic retinopathy (DR) is a common microvascular complication of diabetes that affects 1/3 of diabetic patients and is associated with an increased risk of life-threatening systemic vascular complications, such as stroke, coronary artery disease, and heart failure (2). DR involves specific neuroglial and microvascular abnormalities, such as microaneurysms, soft or hard exudates, intraretinal microhemorrhages, beaded veins, and intraretinal microvascular abnormalities (3,4), which have been shown to progressively disrupt retinal function (5). Traditional DR treatments include laser therapy, vitrectomy, and conventional medications to control hyperglycemia, hypertension, and hyperlipidemia. These treatments inhibit retinal neovascularization, reduce retinal macular edema, and prevent the stretching of fibrous tissue during the proliferation of new vessels in the retina (6). However, laser therapy is often accompanied by vitreous hemorrhage, while 23G minimally invasive vitrectomy can lead to retinal rupture and postoperative cataract formation (7,8). As these treatments can have a number of adverse effects, such as intraoperative retinal fissures and postoperative retinal detachment (9), a more in-depth exploration of the disease is needed to develop safer treatment plans.
The pathogenesis of DR involves multiple biochemical signaling pathways, such as protein glycosylation and the increased activity of protein kinase C, which lead to the formation of advanced glycosylation end products (AGEs). These can promote intercellular interactions involving the vascular endothelial growth factor, leading to neointima formation in the anterior and posterior segments of the eye, increased vascular permeability, resulting in leakage, and the collapse of the internal blood-retinal barrier (10). DR progression appears to involve AGE accumulation, an increase in glucose flux into the polyol and hexosamine pathways, and the excessive activation of the plasma kininase-releasing kinase pathway (11).
DR also involves inflammation (12,13), which damages and permeabilizes the retinal vascular system and disrupts neointima formation, 2 of the leading causes of visual impairment in diabetes (14,15). The dysfunction of retinal glial cells, such as astrocytes, Müller glia, and microglia, may contribute to early retinal inflammatory processes in DR (16). Cellular levels change during disease progression. Thus, this study sought to explore how abnormalities at the level of subpopulations of retinal tissue cells play a role in the development of DR.
Single-cell RNA sequencing (scRNA-seq) technology can reveal unique changes in each cell and enable precise classification of different cell types, as well as help us understand the changes in tumor cells during metastasis to target organs (17). It provides new and precise insights into our understanding of genetic heterogeneity during tumor evolution and tumor cell metastasis. In this study, we used single-cell transcriptome data from DR and healthy tissues to construct a single-cell atlas of DR and explore changes in the levels of different cell subpopulations in the disease. A functional enrichment analysis was also conducted to identify the signaling pathways that may be involved in the disease. In contrast to others’ studies, we will also explore intercellular interactions to further analyze their role in the development of DR. Our findings may help clarify the disease mechanism and inform the development of effective treatment strategies. We present the following article in accordance with the MDAR reporting checklist (available at https://atm.amegroups.com/article/view/10.21037/atm-22-1546/rc).
Methods
Data source
Single-cell transcriptomic data were obtained from the Gene Expression Omnibus database (18). The GSE178121 data set was based on the GPL24247 platform and included retinal tissue samples from healthy C57BL/6 mice (n=1) and C57BL/6 mice with streptozotocin-induced diabetes (n=1) (a total of 2 samples). In GSE178121, the mice kept high blood glucose concentration (>300 mg/dL) for 25 weeks before sacrificed to prepare retinal cell suspension for scRNA-Seq, all cells were coming from mice, thus there no cell lines.
Construction of the single-cell atlas of DR and cell-type annotation
Cell clustering was performed using the Seurat package in R with default parameters (19). The clustering results were uniformly downscaled and visualized using a principal component analysis and t-distributed stochastic neighbor embedding (20), and then projected in a 2-dimensional image defined as the single-cell atlas. The FindAllMarkers function of the Seurat package was also used to identify the marker genes that were highly expressed in each cell cluster. Cell-type annotation was performed based on known retinal cell markers (18) and cell markers of the Human Proteome Project (21).
Single-cell component analysis
A single-cell component analysis was performed based on the markers of different cell clusters to investigate differences in the numbers of retinal cell subpopulations between the DR and healthy samples and to identify cells dysregulated in DR.
Analysis of protein-protein interaction network and receiver operating characteristic (ROC) curve
Moreover, the differentially expressed genes with adjusted P<0.05 was conducted to constructed the protein-protein interaction (PPI) network based on STRING database (22). Then, the interaction between cells was plot with iTalk package (23).
To observe the diagnostic potential of differentially expressed genes (adjusted P<0.05, average log2 fold change >1), area under the receiver operating characteristic (ROC) curve was applied to the analysis using pROC package (24). While the closer the area under the curve (AUC) is to zero, the higher the diagnostic potential.
Cell subcluster analysis
The subcluster analysis of the identified cells was performed with the Seurat package, and the FindAllMarkers function was used to identify marker genes expressed in each cell subcluster. Cell subclusters were then classified based on which marker genes were expressed the most abundantly.
Functional enrichment analysis
A Kyoto Encyclopedia of Genes and Genomes (KEGG) pathway enrichment analysis was conducted using the clusterProfiler package (25) to identify the potential functions of molecular pathways occurring in each cell subpopulation. Pathways were considered significantly involved in marker genes when they had an adjusted P value <0.05. The adjusted P value was obtained using the Benjamin-Hochberg method (26), which can correct the P value and control the false/true positive ratio within a certain range.
Data and statistical analyses
All statistical analyses were performed using R (https://www.r-project.org/). We analyzed the expression levels of genes using unpaired t-tests. P<0.05 was consistent with statistical significance. And the analyses were performed using the online Platform Bioinforcloud platform (http://www.bioinforcloud.org.cn) and Biomarker Technologies Corporation (Beijing, China).
Results
Single-cell atlas of DR
A single-cell atlas comprising 55 cell clusters (Figure 1A) was constructed using a total of 21,588 cells, of which 13,336 came from the DR mice and 8,252 came from the healthy controls (Figure 1B). The obtained clusters were classified into the following 10 cell types: retinal pigment epithelial cells, pericytes, endothelial cells, astrocytes, microglia, anaplastic cells, cone photoreceptors, rod photoreceptors, bipolar cells, and Müller cells (Figure 1C). The following marker genes were the most strongly expressed in the various cell clusters: cone photoreceptors (Cone), Guca1; Müller cells, Rlbp1; bipolar cells (BCs), Trpm1; rod photoreceptors (Rod), Nrl; pericytes (Per), Sparcl1; endothelial cells (ECs), Pecam1; anaplastic cells (ACs), Pax6; retinal pigment epithelial cells (RPEs), Rpe65; microglia (Mic), Aldoc; astrocytes (Ast), and Gfap (Figure 1D). We explored the differences in the numbers of various cell types between the DR and healthy control samples (Figure 1E). The results indicated that Müller glial cells were significantly decreased in the DR samples. The analysis revealed a wide range of intercellular interactions, suggesting that DR development is closely related to intercellular interactions (Figure 1F). In the present study, Cirbp was found to have potential (AUC =0.795) as a diagnostic marker for DR (Figure 1G).
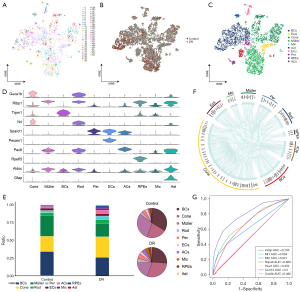
Müller glial cell function in DR
The Müller cells were divided into 78 subpopulations (Figure 2A), and a single-cell atlas was constructed according to the sample source (Figure 2B). The subpopulations Müller_Mt2 and Müller_Mir124-2hg were significantly larger in the DR tissues than the control tissues, while the other 5 subpopulations were significantly smaller (Figure 2C). The expression of the marker genes in each Müller cell subpopulation was determined by a cell subcluster analysis (Figure 2D). The KEGG pathway enrichment analysis showed that the subpopulation genes were involved in several inflammation-related pathways, such as synaptic vesicle cycling, interleukin 17 (IL-17) signaling, Toll-like receptor signaling, and the lysosomal pathways (Figure 2E).
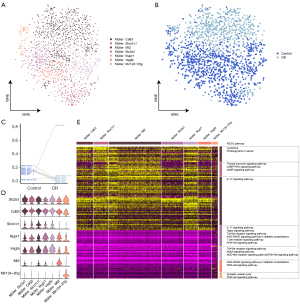
Endothelial cell function in DR
The endothelial cells were divided into 4 subpopulations (Figure 3A) and a single-cell atlas was constructed based on the sample source (Figure 3B). The levels of ECs_Mfsd2a were significantly lower in the DR group than in the healthy control group, while the other subpopulations were significantly increased (Figure 3C). The expression of the marker genes in each endothelial cell subpopulation was determined by a cell subcluster analysis (Figure 3D). The enrichment analysis showed that the subpopulation genes were involved in the cell adhesion-related signaling pathways, such as extracellular matrix-receptor interactions and gap junctions, and the chemokine signaling pathway (chemokine is known to play an important role in inflammation) (27) (Figure 3E).
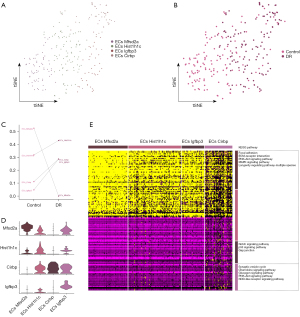
Microglial function in DR
The microglia were divided into 3 microglia subpopulations (Figure 4A) and were used to construct a single-cell atlas depending on the sample source (Figure 4B). The abundance of Mic_Fcer1g and Mic_Slco2b1 was lower in the DR group than the healthy control group, while that of the Mic_Bex2 was significantly higher in the DR group than the healthy control group (Figure 4C). The expression of the marker genes in each microglia subpopulation was determined by a cell subcluster analysis (Figure 4D). Consistent with previous findings (28), the subpopulation genes were found to be involved in several inflammation-related pathways, such as synaptic vesicle cycling, IL17 signaling, and Toll-like receptor signaling (Figure 4E).
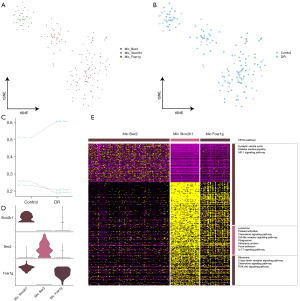
Bipolar cell function in DR
The bipolar cells were divided into 6 subpopulations (Figure 5A) and a single-cell atlas was constructed based on the sample source (Figure 5B). Our results showed that only 2 of the 6 subpopulations were larger in the DR tissues than the control tissues (Figure 5C). The expression of the marker genes in each bipolar cell subpopulation was determined by a cell subcluster analysis (Figure 5D). The enrichment analysis showed that the subpopulation genes were involved in inflammation-related pathways, including chemokine signaling, advanced glycosylation end product (AGE)-receptor for advanced glycation end product (RAGE) signaling in diabetic complications, and calcium signaling (Figure 5E).
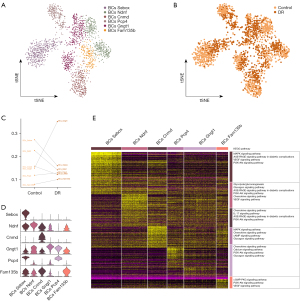
Discussion
In this study, we used DR-related single-cell transcriptome data to construct a single-cell atlas of the DR and healthy control samples. We also performed a cell subpopulation analysis to explore changes in the abundance of different cell subpopulations in DR and identify pathways involved in disease progression. Our results showed that DR was associated with significantly reduced numbers of bipolar cells, Müller glia, retinal pigment epithelial cells, and cone photoreceptors, but was also associated with significantly greater numbers of pericytes, rod photoreceptors, anaplastic cells, and microglia. The enrichment analysis suggests that the retinal cell subpopulations altered in DR are involved in pathways related to oxidative stress and inflammation.
Müller glial cells are the predominant cell type in the mammalian retina and play an important role in maintaining homeostasis and retinal integrity (29,30), as they can alter their morphology to respond to retinal damage and disease (31). However, retinal damage can reduce their abundance, thereby limiting the healing response and promoting the development of DR (32). Müller cells have also been found to produce high levels of pro-inflammatory molecules under hyperglycemic conditions (33). Our study showed that Müller cell subpopulation genes are involved in lysosomal pathways, and lysosomal membrane permeabilization, and the leakage of lysosomal contents are known to lead to cell death, which may explain their decreased abundance in DR. The genes are also involved in several inflammation-related pathways. These findings imply that restoring the response of Müller cells in DR lesions and lowering blood glucose levels may inhibit the secretion of pro-inflammatory factors and mitigate DR.
Endothelial cells are one of the main components of the retinal microvasculature, which is essential for retinal function (34). They play an important role in both inflammation and angiogenesis in DR (15) and are critical in attracting immune cells to sites of inflammation (35). Inflammatory cells produce angiogenic cytokines and growth factors, which in turn activate the microvascular endothelium, which expresses pro-inflammatory molecules (36-38). In our study, we showed that endothelial cell subpopulations are involved in similar inflammation-related pathways.
Microglia are a key component of the retina and are known to enhance neurodegeneration in ocular disease by producing pro-inflammatory neurotoxic cytokines and phagocytosing neurons (28). The activation of microglia may help initiate neuroinflammation in DR (14). Activated microglia produce pro-inflammatory mediators that promote neuronal cell death but that may also act as neuroprotective agents (39-41). In the present study, the abundance of microglia were increased, which might drive DR progression, and we found that microglia subpopulations in DR participate in inflammation-related pathways.
In the retina, bipolar cells receive input from rod and cone photoreceptors, ganglion cells receive input from bipolar cells, and amacrine cells regulate these interactions (42). Bipolar cells are a fundamental component of vision, as they serve as a link between the 2 synaptic layers of the retina (43,44). An earlier study using the GSE178121 data set showed that the bipolar cell population is not significantly affected in DR (18); however, we found that the abundance of bipolar cells was significantly lower in DR tissues than healthy tissues. We also found that the BCs_Gngt1 subpopulation participates in the calcium signaling pathway. It may be that the abnormal levels of bipolar cells in DR are the result of dysfunctional calcium homeostasis, as decreased calcium signaling inhibits neurotransmission (45), particularly in patients with diabetes (46-48).
Inflammation plays a key role in the development of DR (12,13,49). In this study, we found that Müller_Slc2a1, Müller_Nupr1, and Mic_Slco2b1 subpopulation genes are involved in the Toll-like receptor signaling pathway, which promotes the secretion of angiogenic growth factors in arterial endothelial cells and leads to choroidal neovascularization (50,51). We also found that the ECs_Cirbp subpopulation is involved in the chemokine and nucleotide-binding oligomerization domain (NOD)-like signaling pathways. Chemokines are a large class of chemotactic cytokines that act on G protein-coupled receptors and regulate various biological processes, and they can help drive diseases involving pathological inflammation (52,53). Conversely, NOD-like receptor (NLR) proteins are pattern recognition receptors that mediate the innate immune response to cellular injury and stress (54). NLRs can also form inflammatory vesicles, which mediate the activation of caspase-1 and the secretion of the pro-inflammatory cytokines IL-1β and IL-18 (55,56). Endothelial cells contain abundant danger signals, such as reactive oxygen species, which activate the NLRP3 inflammasome, exacerbating inflammatory signaling, and cellular damage (57). These results suggest that endothelial cell abnormalities in DR may be associated with the abnormal activation of these pathways.
The results of the enrichment analysis of the above subgroups revealed that the “AGE-RAGE signaling pathway in diabetic complications” is significantly enriched in the Müller_Slc2a1, Müller_Vegfa, BCs_Sebox, BCs_Ndnf, and BCs_Cnmd subpopulations. AGEs in diabetes are formed by the non-enzymatic reaction between glucose and long-lived proteins, such as collagen (58), and the AGE-RAGE axis is strongly associated with the progression of diabetes-related macrovascular complications, arterial damage, diabetic nephropathy, and retinopathy (59). AGEs have been found to induce inflammation and immunosuppression by binding to RAGE, leading to the production of pro-inflammatory cytokines, reactive oxygen species, and reactive nitrogen intermediates that alter innate and adaptive immune responses (60). These molecules also promote the pathogenesis of diabetic vascular complications mediated by oxidative stress (61-63), and activate nicotinamide adenine dinucleotide phosphate oxidase and the nuclear factor kappa light chain enhancer of activated B cells by binding to the specific RAGE on the cell surface (64), thus triggering a vicious cycle of oxidative stress and inflammation (62,65,66). Based on these results, we hypothesize that the AGE-RAGE signaling pathway in diabetic complications may be associated with abnormalities in bipolar cells and Müller glia. Further, we found that Müller glia, endothelial cell, microglia, and bipolar cell subpopulation genes are significantly involved in the phosphatidylinositol-3-kinase and protein kinase B signaling pathway, which accelerates the endothelial-mesenchymal transition in DR (67).
This study was done based on bioinformatics analysis and single-cell sequencing of cells, and the lack of clinical experimental validation is one of the drawbacks of this study. Since human single cell data on DR are scarce, this study used data from mice, whose samples are also small, and in future plans will expand the samples and collect data from humans as much as possible.
In summary, we showed that Müller glia, endothelial cells, microglia, and bipolar cells in DR tissues are involved in oxidative stress- and inflammation-related pathways, which may contribute to the progression of the disease and ultimately lead to visual impairment and blindness. If our findings can be confirmed, they suggest that the early detection of changes in specific retinal cell subpopulations may lead to earlier and more effective treatments.
Acknowledgments
Funding: This study was supported by the National Natural Science Foundation of China (No. 82074499).
Footnote
Reporting Checklist: The authors have completed the MDAR reporting checklist. Available at https://atm.amegroups.com/article/view/10.21037/atm-22-1546/rc
Conflicts of Interest: All authors have completed the ICMJE uniform disclosure form (available at https://atm.amegroups.com/article/view/10.21037/atm-22-1546/coif). The authors have no conflicts of interest to declare.
Ethical Statement: The authors are accountable for all aspects of the work, including ensuring that any questions related to the accuracy or integrity of any part of the work have been appropriately investigated and resolved.
Open Access Statement: This is an Open Access article distributed in accordance with the Creative Commons Attribution-NonCommercial-NoDerivs 4.0 International License (CC BY-NC-ND 4.0), which permits the non-commercial replication and distribution of the article with the strict proviso that no changes or edits are made and the original work is properly cited (including links to both the formal publication through the relevant DOI and the license). See: https://creativecommons.org/licenses/by-nc-nd/4.0/.
References
- American Diabetes Association. Diagnosis and classification of diabetes mellitus. Diabetes Care 2013;36:S67-74. [Crossref] [PubMed]
- Cheung N, Mitchell P, Wong TY. Diabetic retinopathy. Lancet 2010;376:124-36. [Crossref] [PubMed]
- Aliseda Perez de Madrid D, Berastegui I. Diabetic retinopathy. An Sist Sanit Navar 2008;31:23-34. [PubMed]
- Lois N, McCarter RV, O'Neill C, et al. Endothelial progenitor cells in diabetic retinopathy. Front Endocrinol (Lausanne) 2014;5:44. [Crossref] [PubMed]
- Lechner J, O'Leary OE, Stitt AW. The pathology associated with diabetic retinopathy. Vision Res 2017;139:7-14. [Crossref] [PubMed]
- Liu Y, Wu N. Progress of Nanotechnology in Diabetic Retinopathy Treatment. Int J Nanomedicine 2021;16:1391-403. [Crossref] [PubMed]
- Tuuminen R, Sahanne S, Haukka J, et al. Improved outcome after primary vitrectomy in diabetic patients treated with statins. Eur J Ophthalmol 2016;26:174-81. [Crossref] [PubMed]
- Yamada Y, Suzuma K, Ryu M, et al. Systemic factors influence the prognosis of diabetic macular edema after pars plana vitrectomy with internal limiting membrane peeling. Curr Eye Res 2013;38:1261-5. [Crossref] [PubMed]
- Hahn U, Krummenauer F, Ludwig K. 23G pars plana vitrectomy for vitreal floaters: prospective assessment of subjective self-reported visual impairment and surgery-related risks during the course of treatment. Graefes Arch Clin Exp Ophthalmol 2018;256:1089-99. [Crossref] [PubMed]
- Kollias AN, Ulbig MW. Diabetic retinopathy: Early diagnosis and effective treatment. Dtsch Arztebl Int 2010;107:75-83; quiz 84. [PubMed]
- Stitt AW, Curtis TM, Chen M, et al. The progress in understanding and treatment of diabetic retinopathy. Prog Retin Eye Res 2016;51:156-86. [Crossref] [PubMed]
- Adamis AP. Is diabetic retinopathy an inflammatory disease? Br J Ophthalmol 2002;86:363-5. [Crossref] [PubMed]
- Kern TS. Contributions of inflammatory processes to the development of the early stages of diabetic retinopathy. Exp Diabetes Res 2007;2007:95103. [Crossref] [PubMed]
- Gouliopoulos NS, Kalogeropoulos C, Lavaris A, et al. Association of serum inflammatory markers and diabetic retinopathy: a review of literature. Eur Rev Med Pharmacol Sci 2018;22:7113-28. [PubMed]
- Kaštelan S, Orešković I, Bišćan F, et al. Inflammatory and angiogenic biomarkers in diabetic retinopathy. Biochem Med (Zagreb) 2020;30:030502. [Crossref] [PubMed]
- Rübsam A, Parikh S, Fort PE. Role of Inflammation in Diabetic Retinopathy. Int J Mol Sci 2018;19:942. [Crossref] [PubMed]
- Chambers DC, Carew AM, Lukowski SW, et al. Transcriptomics and single-cell RNA-sequencing. Respirology 2019;24:29-36. [Crossref] [PubMed]
- Sun L, Wang R, Hu G, et al. Single cell RNA sequencing (scRNA-Seq) deciphering pathological alterations in streptozotocin-induced diabetic retinas. Exp Eye Res 2021;210:108718. [Crossref] [PubMed]
- Butler A, Hoffman P, Smibert P, et al. Integrating single-cell transcriptomic data across different conditions, technologies, and species. Nat Biotechnol 2018;36:411-20. [Crossref] [PubMed]
- Pezzotti N, Lelieveldt BPF, Van Der Maaten L, et al. Approximated and User Steerable tSNE for Progressive Visual Analytics. IEEE Trans Vis Comput Graph 2017;23:1739-52. [Crossref] [PubMed]
- Thul PJ, Lindskog C. The human protein atlas: A spatial map of the human proteome. Protein Sci 2018;27:233-44. [Crossref] [PubMed]
- Szklarczyk D, Gable AL, Nastou KC, et al. The STRING database in 2021: customizable protein-protein networks, and functional characterization of user-uploaded gene/measurement sets. Nucleic Acids Res 2021;49:D605-12. [Crossref] [PubMed]
- Wang Y, Wang R, Zhang S, et al. iTALK: an R Package to Characterize and Illustrate Intercellular Communication. 2019.
- Robin X, Turck N, Hainard A, et al. pROC: an open-source package for R and S+ to analyze and compare ROC curves. BMC Bioinformatics 2011;12:77. [Crossref] [PubMed]
- Yu G, Wang LG, Han Y, et al. clusterProfiler: an R package for comparing biological themes among gene clusters. OMICS 2012;16:284-7. [Crossref] [PubMed]
- Benjamini Y, Hochberg Y. Controlling the False Discovery Rate: A Practical and Powerful Approach to Multiple Testing. J R Stat Soc Series B Stat Methodol 1995;57:289-300.
- Charo IF, Ransohoff RM. The many roles of chemokines and chemokine receptors in inflammation. N Engl J Med 2006;354:610-21. [Crossref] [PubMed]
- Silverman SM, Wong WT. Microglia in the Retina: Roles in Development, Maturity, and Disease. Annu Rev Vis Sci 2018;4:45-77. [Crossref] [PubMed]
- Reichenbach A, Bringmann A. New functions of Muller cells. Glia 2013;61:651-78. [Crossref] [PubMed]
- Bringmann A, Pannicke T, Biedermann B, et al. Role of retinal glial cells in neurotransmitter uptake and metabolism. Neurochem Int 2009;54:143-60. [Crossref] [PubMed]
- Bringmann A, Iandiev I, Pannicke T, et al. Cellular signaling and factors involved in Muller cell gliosis: neuroprotective and detrimental effects. Prog Retin Eye Res 2009;28:423-51. [Crossref] [PubMed]
- Mizutani M, Gerhardinger C, Lorenzi M. Muller cell changes in human diabetic retinopathy. Diabetes 1998;47:445-9. [Crossref] [PubMed]
- Liu X, Ye F, Xiong H, et al. IL-1beta Upregulates IL-8 Production in Human Muller Cells Through Activation of the p38 MAPK and ERK1/2 Signaling Pathways. Inflammation 2014;37:1486-95. [Crossref] [PubMed]
- Huang H. Pericyte-Endothelial Interactions in the Retinal Microvasculature. Int J Mol Sci 2020;21:7413. [Crossref] [PubMed]
- Certo M, Elkafrawy H, Pucino V, et al. Endothelial cell and T-cell crosstalk: Targeting metabolism as a therapeutic approach in chronic inflammation. Br J Pharmacol 2021;178:2041-59. [Crossref] [PubMed]
- Capitão M, Soares R. Angiogenesis and Inflammation Crosstalk in Diabetic Retinopathy. J Cell Biochem 2016;117:2443-53. [Crossref] [PubMed]
- Joussen AM, Poulaki V, Qin W, et al. Retinal vascular endothelial growth factor induces intercellular adhesion molecule-1 and endothelial nitric oxide synthase expression and initiates early diabetic retinal leukocyte adhesion in vivo. Am J Pathol 2002;160:501-9. [Crossref] [PubMed]
- Qazi Y, Maddula S, Ambati BK. Mediators of ocular angiogenesis. J Genet 2009;88:495-515. [Crossref] [PubMed]
- Yang LP, Sun HL, Wu LM, et al. Baicalein reduces inflammatory process in a rodent model of diabetic retinopathy. Invest Ophthalmol Vis Sci 2009;50:2319-27. [Crossref] [PubMed]
- Zeng HY, Green WR, Tso MO. Microglial activation in human diabetic retinopathy. Arch Ophthalmol 2008;126:227-32. [Crossref] [PubMed]
- Grigsby JG, Cardona SM, Pouw CE, et al. The role of microglia in diabetic retinopathy. J Ophthalmol 2014;2014:705783. [Crossref] [PubMed]
- Eggers ED, Carreon TA. The effects of early diabetes on inner retinal neurons. Vis Neurosci 2020;37:E006. [Crossref] [PubMed]
- Euler T, Haverkamp S, Schubert T, et al. Retinal bipolar cells: elementary building blocks of vision. Nat Rev Neurosci 2014;15:507-19. [Crossref] [PubMed]
- Nawy S, von Gersdorff H. Bipolar cells in the vertebrate retina: from form to function. Introduction. Vis Neurosci 2011;28:1-2. [Crossref] [PubMed]
- Brini M, Calì T, Ottolini D, et al. Neuronal calcium signaling: function and dysfunction. Cell Mol Life Sci 2014;71:2787-814. [Crossref] [PubMed]
- Kruglikov I, Gryshchenko O, Shutov L, et al. Diabetes-induced abnormalities in ER calcium mobilization in primary and secondary nociceptive neurons. Pflugers Arch 2004;448:395-401. [Crossref] [PubMed]
- Satoh E, Takahashi A. Experimental diabetes enhances Ca2+ mobilization and glutamate exocytosis in cerebral synaptosomes from mice. Diabetes Res Clin Pract 2008;81:e14-7. [Crossref] [PubMed]
- Huang TJ, Sayers NM, Fernyhough P, et al. Diabetes-induced alterations in calcium homeostasis in sensory neurones of streptozotocin-diabetic rats are restricted to lumbar ganglia and are prevented by neurotrophin-3. Diabetologia 2002;45:560-70. [Crossref] [PubMed]
- Noda K, Nakao S, Ishida S, et al. Leukocyte adhesion molecules in diabetic retinopathy. J Ophthalmol 2012;2012:279037. [Crossref] [PubMed]
- van Beijnum JR, Buurman WA, Griffioen AW. Convergence and amplification of toll-like receptor (TLR) and receptor for advanced glycation end products (RAGE) signaling pathways via high mobility group B1 (HMGB1). Angiogenesis 2008;11:91-9. [Crossref] [PubMed]
- Feng L, Ju M, Lee KYV, et al. A Proinflammatory Function of Toll-Like Receptor 2 in the Retinal Pigment Epithelium as a Novel Target for Reducing Choroidal Neovascularization in Age-Related Macular Degeneration. Am J Pathol 2017;187:2208-21. [Crossref] [PubMed]
- Locati M, Murphy PM. Chemokines and chemokine receptors: biology and clinical relevance in inflammation and AIDS. Annu Rev Med 1999;50:425-40. [Crossref] [PubMed]
- Keane MP, Strieter RM. Chemokine signaling in inflammation. Crit Care Med 2000;28:N13-26.
- Platnich JM, Muruve DA. NOD-like receptors and inflammasomes: A review of their canonical and non-canonical signaling pathways. Arch Biochem Biophys 2019;670:4-14. [Crossref] [PubMed]
- Kelley N, Jeltema D, Duan Y, et al. The NLRP3 Inflammasome: An Overview of Mechanisms of Activation and Regulation. Int J Mol Sci 2019;20:3328. [Crossref] [PubMed]
- Yang Y, Wang H, Kouadir M, et al. Recent advances in the mechanisms of NLRP3 inflammasome activation and its inhibitors. Cell Death Dis 2019;10:128. [Crossref] [PubMed]
- Bai B, Yang Y, Wang Q, et al. NLRP3 inflammasome in endothelial dysfunction. Cell Death Dis 2020;11:776. [Crossref] [PubMed]
- Asif M, Egan J, Vasan S, et al. An advanced glycation endproduct cross-link breaker can reverse age-related increases in myocardial stiffness. Proc Natl Acad Sci U S A 2000;97:2809-13. [Crossref] [PubMed]
- Naka Y, Bucciarelli LG, Wendt T, et al. RAGE axis: Animal models and novel insights into the vascular complications of diabetes. Arterioscler Thromb Vasc Biol 2004;24:1342-9. [Crossref] [PubMed]
- Shen CY, Lu CH, Wu CH, et al. The Development of Maillard Reaction, and Advanced Glycation End Product (AGE)-Receptor for AGE (RAGE) Signaling Inhibitors as Novel Therapeutic Strategies for Patients with AGE-Related Diseases. Molecules 2020;25:5591. [Crossref] [PubMed]
- Brownlee M. The pathobiology of diabetic complications: a unifying mechanism. Diabetes 2005;54:1615-25. [Crossref] [PubMed]
- Stirban A, Gawlowski T, Roden M. Vascular effects of advanced glycation endproducts: Clinical effects and molecular mechanisms. Mol Metab 2013;3:94-108. [Crossref] [PubMed]
- Giacco F, Brownlee M. Oxidative stress and diabetic complications. Circ Res 2010;107:1058-70. [Crossref] [PubMed]
- Yan SF, Ramasamy R, Schmidt AM. The RAGE axis: a fundamental mechanism signaling danger to the vulnerable vasculature. Circ Res 2010;106:842-53. [Crossref] [PubMed]
- Wautier MP, Chappey O, Corda S, et al. Activation of NADPH oxidase by AGE links oxidant stress to altered gene expression via RAGE. Am J Physiol Endocrinol Metab 2001;280:E685-94. [Crossref] [PubMed]
- Bierhaus A, Chevion S, Chevion M, et al. Advanced glycation end product-induced activation of NF-kappaB is suppressed by alpha-lipoic acid in cultured endothelial cells. Diabetes 1997;46:1481-90. [Crossref] [PubMed]
- He Y, Dan Y, Gao X, et al. DNMT1-mediated lncRNA MEG3 methylation accelerates endothelial-mesenchymal transition in diabetic retinopathy through the PI3K/Akt/mTOR signaling pathway. Am J Physiol Endocrinol Metab 2021;320:E598-608. [Crossref] [PubMed]
(English Language Editor: L. Huleatt)