Integrative analysis of key microRNA-mRNA complexes and pathways in aortic aneurysm
Introduction
Aortic aneurysm (AA) is a permanent and fatal dilation of the aorta resulting from degeneration of the arterial wall. AAs are usually subdivided into thoracic aortic aneurysm (TAA) and abdominal aortic aneurysm (AAA) (1). It is well known that though TAA and AAA have different incidence rates, distribution, and inheritance, they share similar pathological and histological features, such as the breakdown of the extracellular matrix (ECM) and reductions in smooth muscle cells (SMCs) and inflammatory cell infiltration (2). Previous studies found that aberrant proteases, including metalloproteinase family, cathepsins, and granzymes, along with abnormal TGF-β signaling were associated with the initiation and development of TAA and AAA (3,4). AA can lead to irreversible progression and rupture, which are closely related to morbidity and mortality. Surgery can effectively prevent the development and rupture of AA (5). However, the features of the suddenness of AA and the aneurysmal diameters of most patients do not meet the surgical guidelines, resulting in no preventive treatment of AA (6). Therefore, it is vital to have an intensive understanding of the pathogenesis and molecular mechanisms of AA to improve early diagnosis, predict prognosis, and find new therapeutic targets.
MicroRNAs (miRNAs) are small non-coding RNA molecules which negatively regulate gene expression by binding to the 3' untranslated region of messenger RNAs (mRNAs) (7). It has been reported that miRNAs are important in the control of gene expression since up to 2/3 of human genes are modulated by miRNAs. MiRNAs play vital roles in the physiological and pathophysiological processes of cardiovascular diseases and heart dysfunction (8). However, the roles of miRNAs and the miRNA-mRNA complex in AA remain to be fully elucidated.
In the present study, we collected miRNA and mRNA datasets of AA tissue samples and AA blood samples from the Gene Expression Omnibus (GEO) database. We performed differential expression analyses and constructed miRNA-mRNA differential co-expression networks and a protein-protein interaction (PPI) network. Functional enrichment analysis was also performed, including Kyoto Encyclopedia of Genes and Genomes (KEGG) and Gene Ontology (GO) analyses and gene set enrichment analysis (GSEA). We also collected clinical tissue samples of TAA and normal aortas from heart transplantation recipients to further verify theco-differentially expressed miRNAs (theco-DE-miRNAs) by reverse transcription-quantitative polymerase chain reaction (RT-qPCR) and the tyramide signal amplification-in situ hybridization assay (TSA-ISH assay) (Figure 1). In this study, the differential regulatory 4 miRNA-mRNA networks were identified in AA blood and tissue samples revealed key genes and signaling pathways related to AA, and verified the upregulation of miR-4306 and the downregulation of miR-3198 in AA tissue samples. This study may provide novel clues for understanding the mechanism of the pathogenesis of AA and may lead to uncovering potential therapeutic targets of AA. We present the following article in accordance with the STREGA reporting checklist (available at https://atm.amegroups.com/article/view/10.21037/atm-22-514/rc).
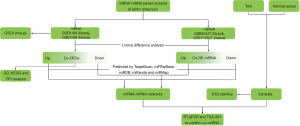
Methods
Microarray data
The microarray datasets were downloaded from the GEO database (https://www.ncbi.nlm.nih.gov/geo/). GSE9106 (https://www.ncbi.nlm.nih.gov/geo/query/acc.cgi?acc= GSE9106) is an mRNA dataset based on the ABI Human Genome Survey Microarray Version 2 platform, which contains 59 TAA blood samples and 34 control blood samples. GSE7084 (https://www.ncbi.nlm.nih.gov/geo/query/acc.cgi?acc= GSE7084) is an mRNA dataset based on the Sentrix Human-6 Expression BeadChip, which contains 6 AAA tissue samples and 7 control tissue samples. GSE92427 (https://www.ncbi.nlm.nih.gov/geo/query/acc.cgi?acc= GSE92427) is a miRNA dataset, which contains 8 AA blood samples and 8 healthy blood samples. GSE110527 (https://www.ncbi.nlm.nih.gov/geo/query/acc.cgi?acc= GSE110527) is a miRNA dataset based on the Agilent-070156 Human miRNA platform, which contains 19 TAA tissue samples and 19 control tissue samples.
Analyses of differentially expressed mRNAs and miRNAs
The expression matrices of mRNA datasets GSE9106 and GSE7084 were analyzed by limma (9) to identify the differentially expressed genes (DEGs) between the AA samples and the control samples. The differentially expressed mRNAs were defined by P value <0.05 and |fold change (FC)| >1.5 (|log2FC| >0.585), and then the intersection was compared to select DEGs.
The expression matrices of miRNA datasets GSE92427 and GSE110527 were analyzed by limma (9) to analyze the DE-miRNAs between the AA samples and the control samples. The significance threshold was P value <0.05 and FC >1.2 or FC <−1.2 (|log2FC| >0.263), and then the intersection was obtained to select the DE-miRNAs.
Prediction of miRNA-mRNA relationship and network construction
Based on the co-DEGs and co-DE-miRNAs, the miRNA-mRNA regulatory pairs were predicted by searching in TargetScan (10), miRTarBase (11), miRDB (12), miRanda (13), and miRMap (14). Cytoscape software (15) was further used to construct the miRNA-mRNA regulatory network.
Functional enrichment analysis
Based on the GO database (16) and the KEGG pathway database (17), the DEGs in blood samples and tissue samples were analyzed for functional enrichment. Based on Fisher’s exact test, P<0.05 was considered to be statistically significant.
PPI network construction
The Search Tool for the Retrieval of Interacting Genes/Proteins (STRING) (https://string-db.org/) is a public database that provides information about protein interactions (18). In our study, the STRING tool was used to generate PPIs among the DEGs in AA, with the threshold of combined score >0.4.
GSEA
GSEA was performed by the program downloaded from https://software.broadinstitute.org/gsea/index.jsp using MSigDB C2 CP: canonical pathways gene set collection. GSEA is a method of analyzing genome-wide expression profile chip data to compare genes with predefined gene sets. By analyzing the gene expression profile datasets, we can understand how they are expressed in a specific set of functional genes and whether there is statistical significance in this expression status. In this study, according to the functional enrichment analysis of DEGs, mRNA datasets of AAA tissue samples were selected for GSEA of the expression matrix. The nominal P value and normalized enrichment score (NES) were used to sort the pathways enriched in each group.
Sample collection and RT-qPCR assay
Eight TAA samples and 8 normal aortas of heart transplant recipients were collected from The First Affiliated Hospital of Zhejiang University (Hangzhou, China). Written informed consent for the use of the collected samples was obtained from all participants or their legal guardians. The study was conducted in accordance with the Declaration of Helsinki (as revised in 2013). The study was approved by the Ethics Committee of The First Affiliated Hospital of Zhejiang University (No. 2021IIT592). The basic information and the related medical records were collected in Table S1.
Total RNA was extracted from cells using TRIzol (Thermo Fisher Scientific, USA). Reverse transcription was performed using the RevertAid First Strand cDNA Synthesis Kit (Thermo Fisher, USA) and specific reverse transcription primers. FastStart Universal SYBR Green Master (Roche, Switzerland) was used for quantitative miRNA detection according to the manufacturer’s protocol. The miRNA PCR primers were purchased from Tsingke Biotechnology (Tsingke, China). The primer sequences can be found in Table S2. Cycle threshold (Ct) values were calculated, and the relative miRNA levels were analyzed using the 2−ΔΔCt method.
Verhoeff Van Gieson (EVG) staining assay
EVG staining was conducted with the EVG staining kit (Abcam, ab150667) according to the manufacturer’s instructions. The representative images were captured by a digital pathology camera (3DHISTECH, Hungary).
TSA-ISH assay
TSA-ISH was performed using the Paraffin-DIG-TSA-ISH Kit (Servicebio Technology Company, Wuhan, China) following the manufacturer’s instructions. Oligos 5'Digoxin-TCTCCATTCCCCAGGACTCCAC and 5'Digoxin-TACTGCCTTTCTCTCCA were used as ISH probes for miR-3198 and miR-4306, respectively. The representative images of ISH were captured under a fluorescence digital pathology camera (3DHISTECH, Hungary).
Statistical analysis
Statistical analyses were conducted with GraphPad Prism (v8.0). Data were presented as the means ± SEM. Student’s t-test was applied to compare the variance between two groups. P<0.05 was considered as statistically significant.
Results
Differential expression analyses
The overview of differentially expressed mRNAs and miRNAs, including the numbers of upregulated and downregulated genes and the numbers of co-differentially expressed mRNAs and miRNAs in the datasets, is shown in Table 1. A volcano plot showed the DEGs with log2FC scores and −log10 P values. The volcano plot of DEGs in the AA blood and tissue samples is shown in Figure 2A, in which the red dots represented the upregulated genes, and the blue dots represented the downregulated genes. The top 5 upregulated and downregulated genes were labeled genes. The heatmaps showed the DEGs clustered between AA and normal blood or tissue samples (Figure 2B).
Table 1
Dataset | Platform | Upregulated number | Downregulated number |
---|---|---|---|
Blood mRNA: GSE9106 | ABI Human Genome Survey Microarray Version 2 platform | 667 | 95 |
Tissue mRNA: GSE7084 | Sentrix Human-6 Expression BeadChip | 1,303 | 1,362 |
Co_diff_mRNA | – | 19 | 5 |
Blood miRNA: GSE92427 | Agilent-031181 Unrestricted Human miRNA V16.0 Microarray | 8 | 5 |
Tissue miRNA: GSE110527 | Agilent-070156 Human miRNA (miRNA version) platform | 394 | 294 |
Co_diff_miRNA | – | 1 | 1 |
MiRNA, microRNA; mRNA, messenger RNA.
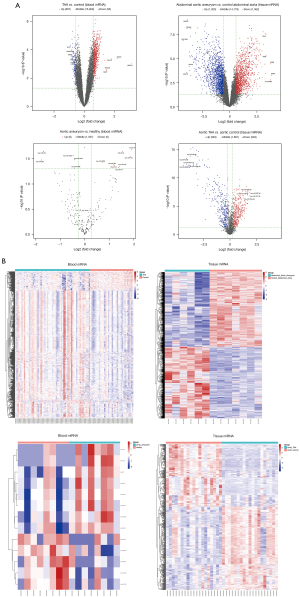
Prediction of miRNA-mRNA regulatory relationship and construction of miRNA-mRNA networks
Based on the co-DEGs and co-DE-miRNAs, the miRNA-mRNA regulatory pairs were predicted. By searching several databases, we found the pairs of the downregulated miR-3198 and the corresponding upregulated genes (SDS, NR2F1, and CLEC2D) and the pair of upregulated miR-4306 and the corresponding downregulated gene TPM2 (Figure 3).
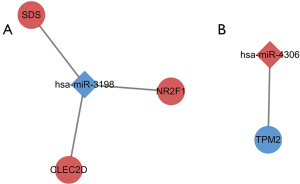
GO and KEGG enrichment analysis
GO functional enrichment analysis and KEGG pathway enrichment analysis were performed on DEGs in blood samples and tissue samples (Figure 4 and Figure S1). To investigate the biological roles of the DEGs in AA, we performed categorized GO annotation analyses including biological processes (BP), cellular components (CC), and molecular functions (MF). As shown in Table 2, multicellular organism development (P=1.81×10−9), G protein coupled receptor (GPCR) signaling pathway (P=1.82×10−8), and response to organic cyclic compound (P=4.35×10−7) were the most significantly enriched BPs in AA blood samples. Hsa04080 neuroactive ligand receptor interaction (P=1.60×10−4), hsa00260 glycine serine and threonine metabolism (P=1.92×10−3), and hsa04020 calcium signaling pathway (P=1.95×10−3) were the most significantly enriched pathways in the KEGG analysis of AA blood samples, as shown in Table 2. In AA tissue sample analysis as shown in Table 3, leukocyte activation (P=6.85×10−44), cell surface receptor signaling pathway (P=3.21×10−38), and myeloid leukocyte activation (P=8.99×10−34) were the most significantly enriched BPs in AA. Hsa04640 hematopoietic cell lineage (P=2.42×10−15), hsa04062 chemokine signaling pathway (P=1.05×10−12), and hsa04061 viral protein interaction with cytokine and cytokine receptor (P=2.49×10−12) were the most significantly enriched pathways in the KEGG analysis of AA.
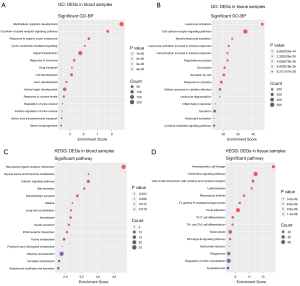
Table 2
Category | Description | Count | P value |
---|---|---|---|
BP | GO:0007275~multicellular_organism_development | 211 | 1.81×10−9 |
BP | GO:0007186~G_protein-coupled_receptor_signaling_pathway | 76 | 1.82×10−8 |
BP | GO:0014070~response_to_organic_cyclic_compound | 55 | 4.35×10−7 |
BP | GO:0019935~cyclic-nucleotide-mediated_signaling | 19 | 8.62×10−7 |
BP | GO:0007165~signal_transduction | 199 | 1.81×10−6 |
BP | GO:0009725~response_to_hormone | 52 | 5.03×10−6 |
BP | GO:0015893~drug_transport | 16 | 7.41×10−6 |
BP | GO:0048468~cell_development | 79 | 7.91×10−6 |
BP | GO:0061564~axon_development | 29 | 1.84×10−5 |
BP | GO:0048513~animal_organ_development | 131 | 2.47×10−5 |
KEGG | Hsa04080~neuroactive_ligand-receptor_interaction | 25 | 1.60×10−4 |
KEGG | Hsa00260~glycine_serine_and_threonine_metabolism | 6 | 1.92×10−3 |
KEGG | Hsa04020~calcium_signaling_pathway | 15 | 1.95×10−3 |
KEGG | Hsa04976~bile_secretion | 8 | 2.60×10−3 |
KEGG | Hsa04728~dopaminergic_synapse | 11 | 4.25×10−3 |
KEGG | Hsa05144~malaria | 6 | 6.03×10−3 |
KEGG | Hsa04720~long-term_potentiation | 7 | 6.69×10−3 |
KEGG | Hsa05146~amoebiasis | 9 | 6.82×10−3 |
KEGG | Hsa04911~insulin_secretion | 8 | 7.72×10−3 |
KEGG | Hsa04512~ECM-receptor_interaction | 8 | 8.84×10−3 |
GO, Gene Ontology; KEGG, Kyoto Encyclopedia of Genes and Genomes; BP, biological processes; ECM, extracellular matrix.
Table 3
Category | Description | Count | P value |
---|---|---|---|
BP | GO:0045321~leukocyte_activation | 282 | 6.85×10−44 |
BP | GO:0007166~cell_surface_receptor_signaling_pathway | 546 | 3.21×10−38 |
BP | GO:0002274~myeloid_leukocyte_activation | 191 | 8.99×10−34 |
BP | GO:0002366~leukocyte_activation_involved_in_immune_response | 197 | 1.24×10−32 |
BP | GO:0002263~cell_activation_involved_in_immune_response | 197 | 3.31×10−32 |
BP | GO:0045055~regulated_exocytosis | 212 | 3.32×10−32 |
BP | GO:0006887~exocytosis | 228 | 3.10×10−31 |
BP | GO:0032940~secretion_by_cell | 269 | 5.39×10−31 |
BP | GO:0034097~response_to_cytokine | 285 | 3.95×10−30 |
BP | GO:0071345~cellular_response_to_cytokine_stimulus | 267 | 1.18×10−29 |
KEGG | Hsa04640~hematopoietic_cell_lineage | 49 | 2.42×10−15 |
KEGG | Hsa04062~chemokine_signaling_pathway | 69 | 1.05×10−12 |
KEGG | Hsa04061~viral_protein_interaction_with_cytokine_and_cytokine_receptor | 45 | 2.49×10−12 |
KEGG | Hsa05140~leishmaniasis | 38 | 3.84×10−12 |
KEGG | Hsa05323~rheumatoid_arthritis | 41 | 5.28×10−11 |
KEGG | Hsa04666~Fc_gamma_R-mediated_phagocytosis | 40 | 2.29×10−10 |
KEGG | Hsa04510~focal_adhesion | 66 | 4.19×10−10 |
KEGG | Hsa04659~Th17_cell_differentiation | 42 | 2.46×10−9 |
KEGG | Hsa04658~Th1_and_Th2_cell_differentiation | 38 | 2.62×10−9 |
KEGG | Hsa05152~tuberculosis | 59 | 5.95×10−9 |
GO, Gene Ontology; KEGG, Kyoto Encyclopedia of Genes and Genomes; BP, biological processes.
PPI network construction
Co-DEGs were submitted to the STRING database to analyze the PPI relationship of the co-DEGs, and a total of 6 pairs were obtained, including ARRB2-MAPK8IP3, TK1-NT5E, NT5E-IL10, IL10-IL27RA, IL10-APOE, and APOE-PTK2B (Figure 5).
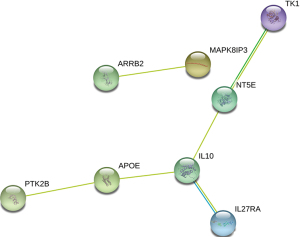
GSEA identifies AA-related signaling pathways
To identify key pathways that were differentially activated in AA, we conducted GSEA between patients with AA and healthy controls and selected the most significantly enriched signaling pathways (top 5) based on their NES (Figure 6, Table 4). The most significantly enriched pathways were related to natural killer (NK) cell mediated cytotoxicity, staphylococcus aureus infection, B cell receptor signaling pathway, toll-like receptor signaling pathway, and osteoclast differentiation.
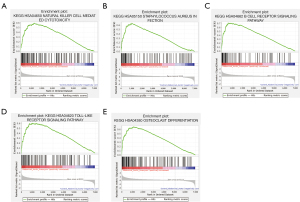
Table 4
Name | Size | NES | P value |
---|---|---|---|
KEGG_hsa04650_natural_killer_cell_mediated_cytotoxicity | 126 | 2.265 | <0.001 |
KEGG_hsa05150_staphylococcus_aureus_infection | 89 | 2.237 | <0.001 |
KEGG_hsa04662_B_cell_receptor_signaling_pathway | 79 | 2.187 | <0.001 |
KEGG_hsa04620_toll-like_receptor_signaling_pathway | 100 | 2.181 | 0.004 |
KEGG_hsa04380_osteoclast_differentiation | 126 | 2.178 | <0.001 |
GSEA, gene set enrichment analysis; KEGG, Kyoto Encyclopedia of Genes and Genomes; AAA, abdominal aortic aneurysm; NES, normalized enrichment score.
RT-qPCR and TSA-ISH staining predicts the expression of miR-3198 and miR-4306 in TAA patients
RT-qPCR assays showed that miR-3198 was downregulated (*P<0.05) and miR-4306 was upregulated (*P<0.05) in AA samples compared with the normal control tissues (Figure 7A).
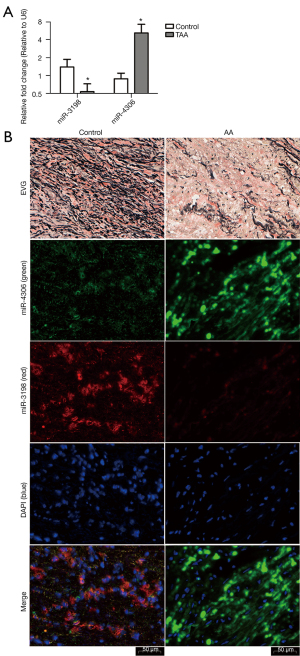
EVG staining showed disrupted elastic fibers in the aorta of AA patients. ISH was performed to investigate the role of miR-3198 and miR-4306 in clinical tissue samples. Compared with normal aorta samples from heart transplant recipients, the expression of miR-3198 (red) was significantly lower in AA samples, while miR-4306 (green) expression was significantly higher in AA samples (Figure 7B).
Discussion
AA is a focal dilation of the aorta, with high morbidity and mortality. Recently, several studies have reported that non-coding RNAs, such as long non-coding RNA (lncRNA), circular RNA (circRNA), and miRNA, play an important regulated role in the development of AA (19-21). LncRNAs/circRNAs act as competitive endogenous RNA (ceRNA)-miRNA-mRNA interaction played a key mechanism in the pathogenesis of AA and involved the occurrence and development of AA, including the proliferation, apoptosis and migration of vascular SMCs, and biomarkers (22,23). However, the molecular mechanisms of AA formation remain to be elucidated. It has been reported that miRNAs play important roles in various BP. Interestingly, miRNAs can be easily detected in peripheral blood and can serve as biomarkers for diseases, including AA. There is already a study aimed at targeting miRNAs and exploiting them as potential therapeutic targets for AA (24). This study identified candidate miRNAs, targeted genes, and BP closely associated with AA formation using bioinformatics methods.
Based on co-DEGs and co-DE-miRNAs in AA patients’ blood and tissue samples, 4 miRNA-mRNA regulatory pairs were predicted. It was reported that miR-3198 downregulates osteoprotegerin (OPG) expression in response to mechanical stress (25) and osteopontin (OPN) may be a driver of AAA formation (26). Therefore, miR-3198 might downregulate and upregulate its target genes in AA by inhibiting OPN signaling. One of the target genes of miR-3198, CLEC2D, directly binds to histones released upon necrotic cell death and thus contributes to inflammation and tissue damage (27). It is also uncovered that CLEC2D modulates the release of interferon-gamma and activates NK cells (28). NR2F1, a target gene of miR-3198, is a member of the steroid/thyroid hormone nuclear receptor superfamily, which participates in a wide range of BP, including cancer progression, cell differentiation, and neurogenesis (29). SDS, another target gene of miR-3198, encodes the enzymes that are involved in metabolizing serine and glycine, and is found predominantly in the liver (30). MiR-4306 was reported as a potential diagnostic biomarker for acute aortic dissection (31). In our study, we found that miR-4306 was upregulated and the corresponding target gene TPM2 was downregulated in AA blood and tissue samples. TPM2, a target gene of miR-4306, showed lower expression levels in an atherosclerosis model in a previous study (32), but was upregulated in aortic dissection (33). Considering their relationship with vascular disease, cell death, and inflammation, these 4 miRNA-mRNA regulatory pairs might play a role in AA formation.
In this study, the GO and KEGG analyses showed that the top 10 enriched GO and KEGG terms were related to development, inflammation, and immune response. The enriched signaling pathways were the GPCR signaling pathway, the calcium signaling pathway, ECM receptor interaction, cytokine induced signaling, and the chemokine signaling pathway. Studies revealed that GPCRs including angiotensin II (AngII) receptor type 1 (AT1) signaling and the regulator of G-protein signaling-1 (RGS1)-controlled signaling pathways in the vasculature control blood pressure homeostasis, vascular homeostasis, and injury (34,35). Active vascular calcification is known to be linked with high-risk atherosclerotic plaque and AAA formation (36). AA formation is characterized by ECM fragmentation and inflammation. The calcium signaling and cytokine/chemokine-induced signaling may be involved in the crosstalk between cells and the ECM (37-39).
According to the related pairs of the PPI network from the DEGs, interleukin-10 (IL-10) was the key factor. IL-10 is an immune-regulatory cytokine with a suppressive role in inflammatory processes. It was reported that increased systemic IL-10 levels could mitigate AAA progression (40). NT5E, also known as CD73, has cardioprotective effects during myocardial infarction and heart failure. NT5E deficiency leads to arterial calcifications (41). IL-27R was shown to suppress T cell activation in atherosclerosis, and IL27RA deficient mice developed significantly more atherosclerosis (42). A previous report also showed that IL-27R protects mice from AAA development (43). APOE knockout mice with an AngII releasing pump are used as an AAA formation model. PTK2B, also known as Pyk2, was related to contractile differentiation in arterial smooth muscle (44). However, the relationship between APOE and PTK2B remains unclear.
In this study, we found that signaling pathways including NK cell mediated cytotoxicity, staphylococcus aureus infection, B cell receptor signaling pathway, toll-like receptor signaling pathway, and osteoclast differentiation was related to AAA. Studies have also revealed that toll-like receptor 3 and 4 may be promising biomarkers in AA (45-47). RANKL, a mediator of osteoclast differentiation, is considered as one of the key factors in the pathogenesis of aortic dilatation (48). It has also been found that atherosclerosis was associated with an increase in NK cells in plaques (49). The staphylococcus aureus infection pathway and the immune/inflammatory responses regulated by B cells were linked to atherosclerotic plaque progression (50-52). However, this study is the first to report the association of AA formation with NK cells, staphylococcus aureus infection, and B cell receptor signaling, and further mechanisms need to be discovered. In future research, we will further analyze the lncRNA-miRNA-mRNA-ceRNA network in AA. Meanwhile, we further explore the functional studies of key miRNAs in AA.
Furthermore, AA tissue samples and normal aorta samples from heart transplant recipients were collected for miRNA RT-qPCR and TSA-ISH staining. The results showed that the expression levels of miR-3198 and miR-4306 in clinical samples matched those from the database analyses. Although the sample size was not large enough, this study was the first to explore miRNA-mRNA regulatory pairs in both blood and tissue samples from AA patients by integrated analysis.
Conclusions
In conclusion, integrated informatics analysis could identify regulatory networks and significant BP related to AA. Furthermore, the RT-qPCR and TSA-ISH assays determined that miR-3198 and miR-4306 may play significant roles in AA progression. These findings provide insights into the mechanisms of AA formation and progression, which may be used as potential diagnostic and therapeutic targets for AA.
Acknowledgments
Funding: This work was supported by Zhejiang Provincial Natural Science Foundation of China (No. LY22H290005), National Natural Science Foundation of China (Nos. 81802887, 81603340) and Natural Science Exploration Program of the Zhejiang Chinese Medical University (No. 2021JKZKTS039B).
Footnote
Reporting Checklist: The authors have completed the STREGA reporting checklist. Available at https://atm.amegroups.com/article/view/10.21037/atm-22-514/rc
Data Sharing Statement: Available at https://atm.amegroups.com/article/view/10.21037/atm-22-514/dss
Conflicts of Interest: All authors have completed the ICMJE uniform disclosure form (available at https://atm.amegroups.com/article/view/10.21037/atm-22-514/coif). The authors have no conflicts of interest to declare.
Ethical Statement: The authors are accountable for all aspects of the work in ensuring that questions related to the accuracy or integrity of any part of the work are appropriately investigated and resolved. The study was conducted in accordance with the Declaration of Helsinki (as revised in 2013). The study was approved by the Ethics Committee of The First Affiliated Hospital of Zhejiang University (No. 2021IIT592) and written informed consent for the use of the collected samples was obtained from all participants or their legal guardians.
Open Access Statement: This is an Open Access article distributed in accordance with the Creative Commons Attribution-NonCommercial-NoDerivs 4.0 International License (CC BY-NC-ND 4.0), which permits the non-commercial replication and distribution of the article with the strict proviso that no changes or edits are made and the original work is properly cited (including links to both the formal publication through the relevant DOI and the license). See: https://creativecommons.org/licenses/by-nc-nd/4.0/.
References
- Davis FM, Daugherty A, Lu HS. Updates of Recent Aortic Aneurysm Research. Arterioscler Thromb Vasc Biol 2019;39:e83-90. [Crossref] [PubMed]
- Li J, Pan C, Zhang S, et al. Decoding the Genomics of Abdominal Aortic Aneurysm. Cell 2018;174:1361-72.e10. [Crossref] [PubMed]
- Lindsay ME, Schepers D, Bolar NA, et al. Loss-of-function mutations in TGFB2 cause a syndromic presentation of thoracic aortic aneurysm. Nat Genet 2012;44:922-7. [Crossref] [PubMed]
- Doyle AJ, Doyle JJ, Bessling SL, et al. Mutations in the TGF-β repressor SKI cause Shprintzen-Goldberg syndrome with aortic aneurysm. Nat Genet 2012;44:1249-54. [Crossref] [PubMed]
- Lederle FA, Kyriakides TC, Stroupe KT, et al. Open versus Endovascular Repair of Abdominal Aortic Aneurysm. N Engl J Med 2019;380:2126-35. [Crossref] [PubMed]
- Pellenc Q, Castier Y, Steg PG. Open versus Endovascular Repair of Abdominal Aortic Aneurysm. N Engl J Med 2019;381:e24. [Crossref] [PubMed]
- Friedman RC, Farh KK, Burge CB, et al. Most mammalian mRNAs are conserved targets of microRNAs. Genome Res 2009;19:92-105. [Crossref] [PubMed]
- Quiat D, Olson EN. MicroRNAs in cardiovascular disease: from pathogenesis to prevention and treatment. J Clin Invest 2013;123:11-8. [Crossref] [PubMed]
- Ritchie ME, Phipson B, Wu D, et al. limma powers differential expression analyses for RNA-sequencing and microarray studies. Nucleic Acids Res 2015;43:e47. [Crossref] [PubMed]
- Agarwal V, Bell GW, Nam JW, et al. Predicting effective microRNA target sites in mammalian mRNAs. Elife 2015;4:e05005. [Crossref] [PubMed]
- Chou CH, Shrestha S, Yang CD, et al. miRTarBase update 2018: a resource for experimentally validated microRNA-target interactions. Nucleic Acids Res 2018;46:D296-302. [Crossref] [PubMed]
- Wong N, Wang X. miRDB: an online resource for microRNA target prediction and functional annotations. Nucleic Acids Res 2015;43:D146-52. [Crossref] [PubMed]
- Betel D, Wilson M, Gabow A, et al. The microRNA.org resource: targets and expression. Nucleic Acids Res 2008;36:D149-53. [Crossref] [PubMed]
- Vejnar CE, Zdobnov EM. MiRmap: comprehensive prediction of microRNA target repression strength. Nucleic Acids Res 2012;40:11673-83. [Crossref] [PubMed]
- Shannon P, Markiel A, Ozier O, et al. Cytoscape: a software environment for integrated models of biomolecular interaction networks. Genome Res 2003;13:2498-504. [Crossref] [PubMed]
- Ashburner M, Ball CA, Blake JA, et al. Gene ontology: tool for the unification of biology. The Gene Ontology Consortium. Nat Genet 2000;25:25-9. [Crossref] [PubMed]
- Kanehisa M, Goto S. KEGG: kyoto encyclopedia of genes and genomes. Nucleic Acids Res 2000;28:27-30. [Crossref] [PubMed]
- von Mering C, Huynen M, Jaeggi D, et al. STRING: a database of predicted functional associations between proteins. Nucleic Acids Res 2003;31:258-61. [Crossref] [PubMed]
- Zhang F, Zhang R, Zhang X, et al. Comprehensive analysis of circRNA expression pattern and circRNA-miRNA-mRNA network in the pathogenesis of atherosclerosis in rabbits. Aging (Albany NY) 2018;10:2266-83. [Crossref] [PubMed]
- Barwari T, Joshi A, Mayr M. MicroRNAs in Cardiovascular Disease. J Am Coll Cardiol 2016;68:2577-84. [Crossref] [PubMed]
- Han Y, Zhang H, Bian C, et al. Circular RNA Expression: Its Potential Regulation and Function in Abdominal Aortic Aneurysms. Oxid Med Cell Longev 2021;2021:9934951. [Crossref] [PubMed]
- Pierce JB, Feinberg MW. Long Noncoding RNAs in Atherosclerosis and Vascular Injury: Pathobiology, Biomarkers, and Targets for Therapy. Arterioscler Thromb Vasc Biol 2020;40:2002-17. [Crossref] [PubMed]
- Golledge J. Abdominal aortic aneurysm: update on pathogenesis and medical treatments. Nat Rev Cardiol 2019;16:225-42. [Crossref] [PubMed]
- Kumar S, Boon RA, Maegdefessel L, et al. Role of Noncoding RNAs in the Pathogenesis of Abdominal Aortic Aneurysm. Circ Res 2019;124:619-30. [Crossref] [PubMed]
- Kanzaki H, Wada S, Yamaguchi Y, et al. Compression and tension variably alter Osteoprotegerin expression via miR-3198 in periodontal ligament cells. BMC Mol Cell Biol 2019;20:6. [Crossref] [PubMed]
- Wang SK, Green LA, Gutwein AR, et al. Osteopontin may be a driver of abdominal aortic aneurysm formation. J Vasc Surg 2018;68:22S-9S. [Crossref] [PubMed]
- Lai JJ, Cruz FM, Rock KL. Immune Sensing of Cell Death through Recognition of Histone Sequences by C-Type Lectin-Receptor-2d Causes Inflammation and Tissue Injury. Immunity 2020;52:123-135.e6. [Crossref] [PubMed]
- Mathew PA, Chuang SS, Vaidya SV, et al. The LLT1 receptor induces IFN-gamma production by human natural killer cells. Mol Immunol 2004;40:1157-63. [Crossref] [PubMed]
- Manikandan M, Abuelreich S, Elsafadi M, et al. NR2F1 mediated down-regulation of osteoblast differentiation was rescued by bone morphogenetic protein-2 (BMP-2) in human MSC. Differentiation 2018;104:36-41. [Crossref] [PubMed]
- Xue HH, Sakaguchi T, Fujie M, et al. Flux of the L-serine metabolism in rabbit, human, and dog livers. Substantial contributions of both mitochondrial and peroxisomal serine:pyruvate/alanine:glyoxylate aminotransferase. J Biol Chem 1999;274:16028-33. [Crossref] [PubMed]
- Wang L, Zhang S, Xu Z, et al. The diagnostic value of microRNA-4787-5p and microRNA-4306 in patients with acute aortic dissection. Am J Transl Res 2017;9:5138-49. [PubMed]
- Meng LB, Shan MJ, Qiu Y, et al. TPM2 as a potential predictive biomarker for atherosclerosis. Aging (Albany NY) 2019;11:6960-82. [Crossref] [PubMed]
- Zhong XX, Wei X, Jiang DS, et al. Expression of tropomyosin 2 in aortic dissection tissue. Zhonghua Xin Xue Guan Bing Za Zhi 2020;48:777-81. [PubMed]
- Patel J, Chuaiphichai S, Douglas G, et al. Vascular wall regulator of G-protein signalling-1 (RGS-1) is required for angiotensin II-mediated blood pressure control. Vascul Pharmacol 2018;108:15-22. [Crossref] [PubMed]
- Fu Y, Huang Y, Yang Z, et al. Cartilage oligomeric matrix protein is an endogenous β-arrestin-2-selective allosteric modulator of AT1 receptor counteracting vascular injury. Cell Res 2021;31:773-90. [Crossref] [PubMed]
- Forsythe RO, Dweck MR, McBride OMB, et al. 18F-Sodium Fluoride Uptake in Abdominal Aortic Aneurysms: The SoFIA3 Study. J Am Coll Cardiol 2018;71:513-23. [Crossref] [PubMed]
- Lindberg S, Zarrouk M, Holst J, et al. Inflammatory markers associated with abdominal aortic aneurysm. Eur Cytokine Netw 2016;27:75-80. [Crossref] [PubMed]
- Satoh M, Nasu T, Osaki T, et al. Thrombospondin-1 contributes to slower aortic aneurysm growth by inhibiting maladaptive remodeling of extracellular matrix. Clin Sci (Lond) 2017;131:1283-5. [Crossref] [PubMed]
- Hadi T, Boytard L, Silvestro M, et al. Macrophage-derived netrin-1 promotes abdominal aortic aneurysm formation by activating MMP3 in vascular smooth muscle cells. Nat Commun 2018;9:5022. [Crossref] [PubMed]
- Adam M, Kooreman NG, Jagger A, et al. Systemic Upregulation of IL-10 (Interleukin-10) Using a Nonimmunogenic Vector Reduces Growth and Rate of Dissecting Abdominal Aortic Aneurysm. Arterioscler Thromb Vasc Biol 2018;38:1796-805. [Crossref] [PubMed]
- Minor M, Alcedo KP, Battaglia RA, et al. Cell type- and tissue-specific functions of ecto-5'-nucleotidase (CD73). Am J Physiol Cell Physiol 2019;317:C1079-92. [Crossref] [PubMed]
- Peshkova IO, Fatkhullina AR, Mikulski Z, et al. IL-27R signaling controls myeloid cells accumulation and antigen-presentation in atherosclerosis. Sci Rep 2017;7:2255. [Crossref] [PubMed]
- Peshkova IO, Aghayev T, Fatkhullina AR, et al. IL-27 receptor-regulated stress myelopoiesis drives abdominal aortic aneurysm development. Nat Commun 2019;10:5046. [Crossref] [PubMed]
- Grossi M, Bhattachariya A, Nordström I, et al. Pyk2 inhibition promotes contractile differentiation in arterial smooth muscle. J Cell Physiol 2017;232:3088-102. [Crossref] [PubMed]
- Jabłońska A, Neumayer C, Bolliger M, et al. Analysis of host Toll-like receptor 3 and RIG-I-like receptor gene expression in patients with abdominal aortic aneurysm. J Vasc Surg 2018;68:39S-46S. [Crossref] [PubMed]
- Li T, Jing JJ, Sun LP, et al. Serum Toll-like receptor 4: A novel and promising biomarker for identification of aortic aneurysmal diseases. Clin Chim Acta 2018;483:69-75. [Crossref] [PubMed]
- Li T, Jing J, Sun L, et al. TLR4 and MMP2 polymorphisms and their associations with cardiovascular risk factors in susceptibility to aortic aneurysmal diseases. Biosci Rep 2019;39:BSR20181591. [Crossref] [PubMed]
- Voronkina IV, Irtyuga OB, Smagina LV, et al. Expression of osteoprotegerin and soluble ligand of receptor of kappa-B transcription factor activator in the calcification of aortic valve. Biomed Khim 2019;65:57-62. [Crossref] [PubMed]
- Bonaccorsi I, Spinelli D, Cantoni C, et al. Symptomatic Carotid Atherosclerotic Plaques Are Associated With Increased Infiltration of Natural Killer (NK) Cells and Higher Serum Levels of NK Activating Receptor Ligands. Front Immunol 2019;10:1503. [Crossref] [PubMed]
- Yang R, Yao L, Du C, et al. Identification of key pathways and core genes involved in atherosclerotic plaque progression. Ann Transl Med 2021;9:267. [Crossref] [PubMed]
- Zhang S, Zhang S, Lin Z, et al. Deep sequencing reveals the skewed B-cell receptor repertoire in plaques and the association between pathogens and atherosclerosis. Cell Immunol 2021;360:104256. [Crossref] [PubMed]
- Yang W, Yin R, Zhu X, et al. Mesenchymal stem-cell-derived exosomal miR-145 inhibits atherosclerosis by targeting JAM-A. Mol Ther Nucleic Acids 2021;23:119-31. [Crossref] [PubMed]
(English Language Editor: C. Betlazar-Maseh)