Construction of survival-related co-expression modules and identification of potential prognostic biomarkers of osteosarcoma using WGCNA
Introduction
Osteosarcoma (OS) is a malignant tumor of the skeletal system that is invasive. Its condition deteriorates rapidly and has a poor prognosis. In children and adolescents, it is a fatal disease (1). Despite continued advancements in neoadjuvant and adjuvant chemotherapy and surgical resection technology in recent years (2), 5-year survival for OS has not improved much due to its high invasiveness, susceptibility to drug resistance, and limited treatment options following drug resistance (3). Additionally, the complexity of the OS genome combined with the tumor’s low incidence creates a barrier to conducting thorough investigations of OS genome biology (4). Therefore, using bioinformatics technology combined with the data of public databases to group patients according to the expression of immune-related genes and clinical prognosis will facilitate more in-depth analyses. This has important clinical application value for finding potential biomarkers to evaluate the clinical prognosis of OS and determining new therapeutic targets.
Weighted gene co-expression network analysis (WGCNA) groups genes with similar expression patterns into modules by calculating the expression correlation between genes, and then examines the relationship between the module and sample characteristics (5). At present, due to the heterogeneity of OS, biomarkers for OS prognosis based on differential expression analysis of small samples often have low sensitivity and specificity, implying that they lack clinical utility. The WGCNA method utilizes whole-genome data to summarize the phenotypic characteristics of the gene network, avoiding bias and subjective judgment (6). WGCNA identifies biomarkers with broader applicability by comparing the connectivity and genetic significance of modules (7). WGCNA has developed into a powerful bioinformatics tool for identifying disease-related genes due to its high reliability and throughput. Currently, many studies on various malignant tumors routinely employ WGCNA for data mining. By analyzing DNA microarray or RNA sequencing data in conjunction with clinical information, a series of modules related to tumor prognosis is obtained, and the module’s hub genes are further mined to identify genes involved in tumor occurrence and development (7,8).
Tumor immunotherapy has emerged as a new focus of cancer research in recent years. Numerous research has focused on immune cell infiltration into tumor tissues in order to better understand the link between the tumor microenvironment and clinical prognosis. For the first time in the history of osteosarcoma bioinformatics analysis, our study began with immune-related genes in order to examine predictive risk stratification of patients. For this study, we obtained the expression profiles and clinical data of OS patients from the therapeutically applicable research to generate effective treatments (TARGET) database. On the basis of the expression profiles of immune-related genes, we clustered the patients in the target database using the non-negative matrix factorization (NMF) cluster analysis method. Based on their prognosis, the patients were divided into 2 groups: high- and low-risk groups. This was independently verified in the Gene Expression Omnibus (GEO) data set. WGCNA was used to build a co-expression network of 2 groups of genes, identify modules related to prognosis, and obtain the important genes in the modules. Combining these important genes and prognostic information, random survival forest analysis was used to identify 3 genes that have a significant impact on the prognosis of OS patients: EPHB3, TEAD1, and KRR1P1. Furthermore, we performed multidimensional analysis and result visualization of these 3 genes from immune cell infiltration analysis, enrichment pathway analysis, gene pairing survival analysis, competitive endogenous RNA (ceRNA) network construction, and antitumor drug sensitivity analysis. These findings could greatly aid in the development of new therapeutic targets and could improve the clinical prognosis of OS patients. We present the following article in accordance with the REMARK reporting checklist (available at https://atm.amegroups.com/article/view/10.21037/atm-22-399/rc).
Methods
Data download
The TARGET database (https://ocg.cancer.gov/programs/target) employs comprehensive molecular characterization to identify genes and their mutations associated with the initiation and progression of pediatric cancer. Through data analysis, the TARGET database generates useful drug targets and prognostic markers for researchers, allowing for the development and application of new and more effective treatments. We downloaded the original OS mRNA expression data and clinical data, and collected data on 85 OS patients with a complete expression profile and survival information. The GEO database (https://www.ncbi.nlm.nih.gov/gds/) is a repository for data generated by chip, next-generation sequencing, and other high-throughput sequencing technologies. We obtained GSE21257 series matrix files from the GEO public database and used the GPL10295 annotation platform. A total of 53 OS patients were enrolled, each with a complete expression profile and survival data. The immune gene set used in this analysis was obtained from the ImmPort database (https://www.immport.org/home) and contained a total of 1,811 immune-related genes. The study was conducted in accordance with the Declaration of Helsinki (as revised in 2013).
Classification and validation of immune subtypes
The NMF package was used to perform unsupervised NMF clustering on the expression profiles of immune-related genes. In the subsequent step, Cox regression analysis was performed on all candidate genes using the R software package “survival”. The results of this analysis were compared to overall survival. Then, using the NMF package, the unsupervised NMF clustering method was applied to the GEO external verification set using the same candidate genes. The best clustering number was determined by the k value where the correlation coefficient began to decrease. Then, using the T-SNE method, we verified the subtype distribution using the mRNA expression data for the aforementioned immune genes.
Construction of the WGCNA co-expression network
The WGCNA standard procedure was used to construct the gene co-expression networks of high-risk and low-risk patients. The WGCNA R package (http://www.r-project.org/) was used to read and import transcriptome data and to eliminate genes that exhibited no variance between groups. The principle of soft threshold filtering is to make the constructed network more in line with the characteristics of scale-free network. The weighted adjacency matrix is transformed into topological overlap matrix (TOM) to estimate its connectivity in the network, and the hierarchical clustering method is used to construct the cluster tree structure of TOM. Different branches of the cluster tree represent distinct gene modules, and each module is represented by a distinct color. The expression patterns of genes were classified using their weighted correlation coefficients. Finally, the gene was partitioned into multiple modules based on its expression pattern. We compared the co-expression networks of high- and low-risk OS patients. We assessed module conservation using the Z-summary score, identified hub genes using the degree of gene linkage, and performed functional enrichment analysis.
Analysis of Gene Ontology (GO) and Kyoto Encyclopedia of Genes and Genomes (KEGG) functions
ClusterProfiler (R3.6) was used to annotate the functions of these genes in order to investigate their functional association. To evaluate related functional categories, GO and KEGG were used. Significant categories were defined as GO and KEGG enrichment pathways with P and q values less than 0.05.
Random survival forest
The randomForestSRC software package was used to select features. Additionally, we used the stochastic survival forest algorithm to rank the importance of prognosis-related genes (nrep =2,000, indicating 2,000 Monte Carlo simulation iterations; and nstep =5, indicating 5 forward steps). We chose genes with a relative importance greater than 0.1 as the final marker genes.
Analysis of correlations between gene expression and immune infiltration
The CIBERSORT algorithm was used to analyze RNA-seq data from patients in various subgroups in order to infer the relative proportion of immune infiltrating cells. The “corrplot” package was used to analyze the interaction between immune cells and the effect of that interaction. The relative immune cell content was plotted using the “vioplot” package. The effect of a gene on immune infiltration was determined, and Spearman correlation analysis of gene expression and immune cell content was performed. The expression of important genes was retrieved, and the Kruskal Test was used to investigate variations in expression between groups.
Gene set enrichment analysis (GSEA)
GSEA ranks genes based on their degree of differential expression between 2 types of samples and then determines whether the predefined gene sets are enriched at the top or bottom of the ranking table. In this study, we used GSEA to compare the signaling pathway differences between high and low expression groups in order to deduce the molecular mechanism underlying the difference between the 2 groups, with the number of replacements set to 1,000 and the type of replacement set to phenotype.
Construction of a regulatory network for ceRNA
In recent years, ceRNAs have garnered considerable attention in academic circles. They represent a novel model of gene expression regulation. In comparison to the miRNA regulatory network, the ceRNA regulatory network is more refined and complex, involving a greater number of RNA molecules, such as mRNAs, pseudogene encoding genes, long-chain non-coding RNAs, miRNAs, and lncRNAs. NPInter is a frequently used database for querying the relationships between lncRNAs and miRNAs. The NPInter database was used to predict lncRNA-miRNA interaction pairs in this study. Additionally, using FunRich software, the interactions between mRNAs and miRNAs were predicted inversely. The lncRNA-miRNA-mRNA network was then constructed by combining the lncRNA-miRNA and mRNA-miRNA interactions, and the network was visualized using Cytoscape software.
Drug sensitivity analysis
The CellMiner database is based on a list of 60 cancer cells compiled by the National Cancer Institute’s (NCI) Cancer Research Center. The NCI-60 cell line is the most frequently used cancer cell line for anticancer drug testing at present. In this study, we downloaded NCI-60 drug sensitivity and RNA-seq gene expression data and used correlation analysis to investigate the relationship between genes and common antineoplastic drug sensitivity, and P<0.05 indicated statistical significance.
Statistical analysis
The statistical analysis was carried out using the R programming language (version 3.6). All statistical tests were bilateral, and statistical significance was defined as P<0.05.
Results
We collected the expression profiles and clinical data of patients from TARGET and GSE21257, extracted immune-related genes from the immune gene set, and finally screened characteristic genes in TARGET patients with OS using the Cox univariate regression feature selection algorithm. Cox univariate regression analysis revealed that a total of 147 prognostic-related genes were screened (available online: https://cdn.amegroups.cn/static/public/atm-22-399-01.xlsx). We clustered the TARGET data set containing OS samples using the NMF consensus clustering method and determined the optimal k value based on the expression profiles of the above 147 candidate genes. After careful consideration, we concluded that k=2 was the optimal cluster number (Figure 1A). The GEO database’s dataset of OS samples was then independently validated using the previously mentioned k=2 classification, which revealed 2 distinct molecular subtypes. Significant differences in prognosis were observed in the TARGET data set, with the C1 subgroup exhibiting superior survival to the C2 subgroup (Figure 1B). Additionally, in the GSE21257 dataset, similar prognostic differences were observed between the 2 subgroups, and the overall survival time for the C2 subgroup was significantly shorter than that of the C1 subgroup (Figure 2).
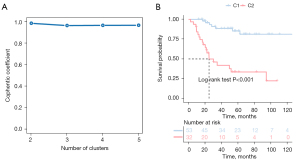
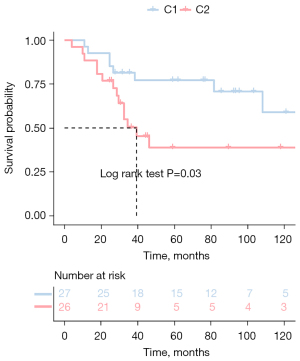
We classified OS patients into 2 subgroups using NMF clustering. Because C2 had a significantly lower survival rate than C1 in both data sets, we classified C2 as a high-risk subgroup and C1 as a low-risk subgroup. We started by preprocessing the TARGET data set, which contained 85 patients. We obtained the gene set required for subsequent analysis by removing genes with zero variance between groups. We then used the hclust function to check for outliers in the data set samples and then set the cutting height of the 2 cluster trees to 90 (Figure 3A,3B). Because the WGCNA algorithm is predicated on the assumption that the gene network exhibits a scale-free distribution, the appropriate soft threshold must be screened out in order to bring the network closer to the characteristics of a scale-free network. We set the soft threshold to 4 for the high-risk group and 3 for the low-risk group (Figure 3C,3D). R-square values of 0.89 and 0.9 were obtained by calculating the scale-free topology fitting index (Figure 3E,3F). These results confirmed and demonstrated the feasibility of WGCNA.
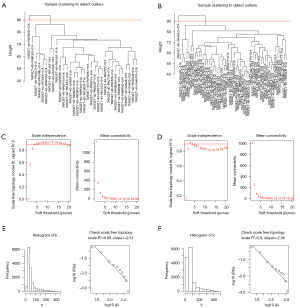
We created 2 co-expression networks of OS patients, one for high-risk and one for low-risk. Based on weighted correlation, hierarchical clustering analysis was carried out. The clustering results were segmented according to the set criteria, and different gene modules were obtained (Figure 4A,4B). Using the WGCNA algorithm for low-risk groups, we identified 29 modules of varying sizes and represented them with cluster tree branches and different colors. Then, the module with the high-risk group network was mapped to the module with the low-risk group network. This approach enabled us to identify modules that were not conservative. The non-conservative module can be thought of as a change in network characteristics between low-risk group networks. Additionally, these non-conservative modules may be associated with the survival and progression of tumors in patients with OS. We used module functions to calculate module conservatism in order to verify WGCNA’s stability. The median and Z-summary scores for conservatism for various colored modules are shown in Figure 4C. The turquoise module had the highest Z-summary score, indicating that it retained high-risk group network characteristics. The purple module with the lowest Z-summary score was more conservative, implying that it can be used as a module feature to differentiate between high- and low-risk patients (Figure 4D).
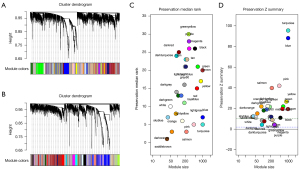
We chose the purple module for more detailed analysis because it was the least conservative, and based on the correlation analysis of the purple module’s first principal component, we obtained heat maps for 500 genes (Figure 5A). Following that, we performed enrichment analysis on these 500 genes and discovered that their biological processes were primarily enriched in the regulation of small GTPase mediated signal transduction, collagen-containing extracellular matrix, and Rho GTPase binding (Figure 5B). Additionally, we described in detail the relationship between significant cellular signaling pathways and critical genes (Figure 5C).
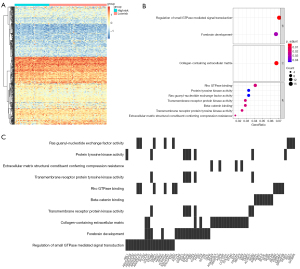
To further identify the core genes among the key genes affecting OS progression, we performed a random survival forest analysis on these 500 genes and chose the genes with a relative importance greater than 0.1 as the final marker. The order of importance of the 5 genes is depicted in Figure 6A. Finally, we analyzed the survival of these 5 critical genes, and the results indicated that EPHB3, KRR1P1, and TEAD1 were significant (Figure 6B-6D), and these 3 genes would serve as the core genes in subsequent research.
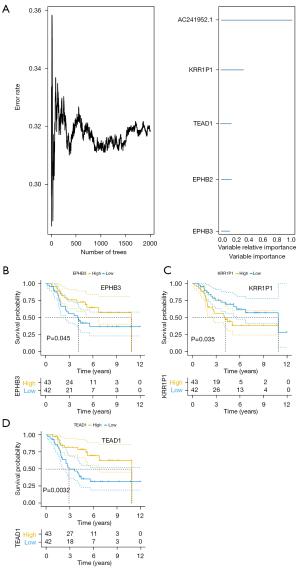
The immune microenvironment is primarily composed of immune cells, extracellular matrix, a variety of growth factors, inflammatory factors, and unique physical and chemical characteristics, all of which have a significant impact on the sensitivity of disease diagnosis and treatment. We further investigated the molecular mechanisms by which core genes affect the progression of OS by analyzing the relationship between core genes and immune infiltration in an OS data set. The analysis of the immune infiltration content demonstrated that M0 macrophages and M2 macrophages accounted for a significant proportion of the sample (Figure 7A). Figure 7B depicts the interaction between immune cells, with the highest correlation between regulatory T cells (Tregs) and CD8+ T cells (person correlation coefficient =0.59). Additionally, when compared to cluster 2, cluster 1 patients had significantly fewer resting dendritic cells, while activated memory CD4+ T cells and CD8+ T cells increased significantly (Figure 7C). The 3 genes were strongly correlated with the content of immune cells. Among them, the EPHB3 gene was significantly positively correlated with resting mast cells, and negatively correlated with M2 macrophages, M1 macrophages, mast cells activated, and dendritic cells resting. The KRR1P1 gene was significantly negatively correlated with B cells memory. The TEAD1 gene was significantly positively correlated with plasma cells and negatively correlated with dendritic cells resting and macrophages M2 (Figure 7D-7F). Three essential genes’ expression levels were compared in two subgroups. We discovered that cluster 1 had a higher level of EPHB3 and TED1 expression than cluster 2 (Figure 7G).
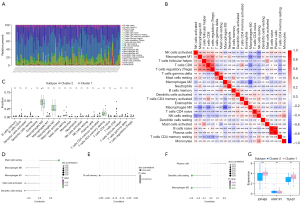
We then investigated the signaling pathways that were associated with the 3 core genes and the molecular mechanisms by which the core genes affect OS progression. The primary bile acid biosynthesis, circadian rhythm, and hedgehog signaling pathways were the primary enrichment pathways for EPHB3. Long-term depression, nicotine addiction, and nitrogen metabolism were the primary enrichment pathways for KRR1P1. Endometrial cancer, circadian rhythm, and type II diabetes mellitus were the primary enrichment pathways for TEAD1 (Figure 8).
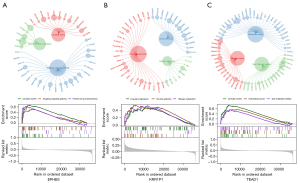
We conducted paired survival analyses on the 3 core genes, each paired with 2 other genes, and classified patients into 4 groups based on the median expression of the 2 genes. The results indicated that in the EPHB3 and KRR1P1 paired groups, group 1 versus 3 had a significant difference, and the survival of group 3 was significantly lower than that of other groups (Figure 9A). In the EPHB3 and TEAD1 paired groups, group 2 versus 3 and group 2 versus 4 had significant differences, and the survival of group 2 was significantly lower than that of other groups (Figure 9B). In the TEAD1 and KRR1P1 paired groups, group 1 versus 3 had a significant difference, with the survival of group 3 possibly being significantly lower than other groups (Figure 9C). Additionally, 14 miRNAs with a total of 14 mRNA-miRNA pairs were predicted using FunRich (version 3.1.3), and a total of 2,436 mRNA-miRNA-lncRNA pairs were predicted using NPInter (version 4.0), resulting in the successful construction of the core gene-related ceRNA network (Figure 10).
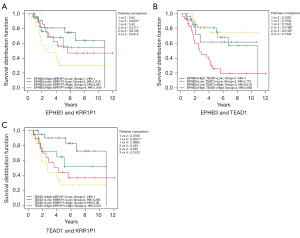
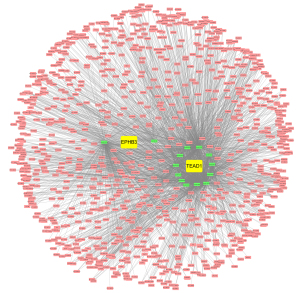
Early OS has a favorable prognosis, and the effect of surgery in combination with chemotherapy is well established. We used the CellMiner database to investigate the sensitivity of TEAD1, KRR1P1, and EPHB3 to commonly used anticancer drugs and calculated the correlation between gene expression and the drug IC50. TEAD1 and EPHB3 were found to be associated with resistance to a variety of anticancer drug types (Figure 11, available online: https://cdn.amegroups.cn/static/public/atm-22-399-02.xlsx). TEAD1 was positively correlated with irofulven, erlotinib, simvastatin, dasatinib, and staurosporine, but negatively correlated with imexon, cyclophosphamide, XK-469, hydroxyurea, and chelerythrine. EPHB3 was positively correlated with tyrothricin, benzimate, and okadaic acid, but negatively correlated with dacarbazine. Figure 11 depicts the most significant drug sensitivities associated with TEAD1 and EPHB3.
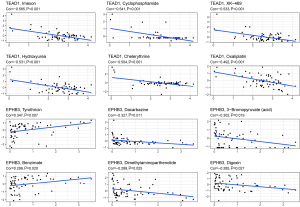
Discussion
OS is the most common primary bone malignant tumor in children. There is a significant correlation between the period of rapid bone growth and the development of the disease (9,10). OS has one of the lowest survival rates in children. In patients with local disease, the 5-year survival rate is 70%, but when there is metastasis, it is only 30% (11,12). The standard treatment is sandwich therapy combined with neoadjuvant chemotherapy, surgery, and adjuvant chemotherapy (13). However, because of its highly invasive characteristics, poor prognosis, and high mortality, there is an urgent need to explore molecular targets and treatments. Many key factors affecting the occurrence and development of OS have been identified through years of molecular research, especially with the progress of high-throughput genome technology, and it is possible to discover more potential molecular markers by bioinformatics.
In this study, OS patients with complete clinical data were obtained from the TARGET database. According to the follow-up time and survival status, patients were divided into 2 groups: high-risk group and low-risk group. In this study, WGCNA was used to construct survival-related co-expression modules in the 2 groups of patients for the first time. WGCNA has a lot of advantages over other methods. As a result, its results are more reliable and biologically important because it looks at how co-expression modules are linked to the clinical features of interest (14). We mapped the high-risk group network to the low-risk group network module. This approach helped us identify non-conservative modules. The lower the Z-summary score, the lower the conservatism of the module, indicating that it can be used as a modular feature to distinguish between high-risk and low-risk patients.
The saved Z-summary score of Figure 4C identified the purple module as the most conservative. Therefore, we focused on the purple module to investigate patient survival factors. We created a heat map of 500 key genes from the purple module. These genes influence the survival time and survival status of OS patients. In order to further identify the core genes that affect the progression of OS, we carried out random survival forest analysis of these 500 genes, and finally selected EPHB3, KRR1P1, and TEAD1 as core genes.
Ephrin-type B receptor 3 (EPHB3) is an EPH transmembrane tyrosine kinase receptor (TKR) which plays a key role in the progression or regression of many tumors. EPHB3 is a direct target motif for Wnt/β-catenin and Notch signal transduction (15,16), consistent with the key role of these pathways in tumorigenesis (17). In the early stage of tumorigenesis, the expression of EPHB3 increases dramatically, followed by a secondary downregulation in up to 30% of cancers (18). The histological expression and function of EPHB3 may explain its invasive and tumor inhibition abilities in colorectal cancer. Repulsive interactions between cells expressing the EPHB3 receptor and EphrinB ligands, respectively, compartmentalize tumors and thereby impede detachment of cells from the primary tumor, so as to reduce the distant spread of tumor (19,20). In addition, EPHB/EphrinB signaling affects the distribution and function of the intracellular adhesion molecule E-cadherin, which helps to stabilize the phenotype of non-invasive epithelial cells. In our study, we found that the low expression of EPHB3 is associated with the poor prognosis of OS. Similarly, Zhang et al. demonstrated that EPHB3 is also a negative regulator of cell proliferation in colon cancer cell lines (21).
TEA domain (TEAD) transcription factor, also known as transcription enhancer factor, is a key component of Hippo-YAP1 signal transduction. In mammals, 4 members (TEAD1–4) have highly conserved domains. By binding to coactivators, TEAD plays a key role in tumorigenesis, including liver cancer (22), ovarian cancer (23), breast cancer (24) and prostate cancer (25), and is overexpressed in these tumors. Some studies have found that the activation of the Hippo/TEAD1-Twist1 pathway can inhibit the growth and metastasis of renal clear cell carcinoma (26). Furthermore, some previous studies have suggested that TEAD1 silencing can inhibit the malignant phenotype of OS cells, including cell proliferation, apoptosis resistance, and invasive potential. However, in our study, we found that OS patients with high expression of TEAD1 had a better prognosis. In both single gene analysis of TEAD1 and the pairwise survival analysis of core genes, high expression of TEAD1 showed a better prognosis. Therefore, high expression of TEAD1 may play a beneficial role in the prognosis of OS through the activation of other pathways, which is a direction that needs to be explored in depth.
Interestingly, in our study, we found that high expression of KRR1P1 was associated with the poor prognosis of patients with OS. KRR1P1 is a pseudogene. In general, more attention is paid to the functional groups that can express proteins, with little research on pseudogenes. However, in the past decade, the completion of a large number of large-scale sequencing projects has provided a wealth of functional genomics data, revealing the importance of pseudogenes as active participants in genomic biology. Some pseudogenes can regulate the expression of protein-coding genes through their RNA products. Pseudogenes, previously thought to be “dead” genomic components, have been shown to be transcribed in a variety of tissues and diseases, including cancer. Pseudogene transcripts have been shown to interfere with the expression of protein-coding genes by acting as antisense transcripts (27), competing endogenous RNAs (28,29), endogenous siRNAs (30), and even forming chimeric RNAs with target protein-coding genes (31). KRR1P1 is a pseudogene of KRR1. However, there is not much data on the function of KRR1 in tumors. Only KRR1 has been reported to be associated with the metastasis of malignant fibrous histiocytoma (32). Through bioinformatics analysis in this study, we obtained the pseudogene KRR1P1 which was closely related to prognosis, opening up a new approach to explore the prognosis-related targets of OS.
In this study, we also analyzed immune infiltration in patients with OS. Zhang et al discovered that the number of resting dendritic cells in high immune score tissues was significantly increased in OS patients (P<0.05), implying a poor prognosis for patients (33). In our study, we showed that resting dendritic cell infiltration was higher in patients with high-risk OS. One study showed that basal-like breast cancer rich in activated memory CD4+ T cells has a better prognosis (34). We found that activated memory CD4+ T cells were significantly increased in low-risk patients. Previous studies on bladder, prostate, renal, and colorectal cancer have reported that the level of CD8+ T cell infiltration is positively correlated with tumor prognosis and immunotherapy responsiveness (35-37), which is consistent with our finding that CD8+ T cells were significantly increased in low-risk patients. According to literature reports, the TEAD family includes TEAD1–4, which is a key regulator of Hippo pathway. The imbalance of Hippo pathway and YAP/TAZ-TEAD activity is related to a variety of diseases, especially cancer. The changes of Hippo protein pathway in cancer cells can affect the interaction between cancer cells and host immune system (38). For example, in a mouse model of liver cancer, high YAP activity in cancer initiating cells promotes the recruitment of immunosuppressive type II macrophages to inhibit the host immune response and may help protect cancer cells from immune surveillance, which recruits macrophages through the expression of YAP-TEAD dependent cytokines (39). This suggests that TEAD1 may play a potential role in immunotherapy. One of the initial processes of establishing immune response is to activate immune cells. There is evidence that Eph receptor and ephin ligand may mediate the activation of immune cells (40). However, given the paucity of studies in the literature, it is still unknown how the signals conveyed by the Eph-eparin junction effect activation and how their expression on distinct immune cell subsets in cis and in trans affects this process. However, it is clear that EPHB3 receptors are expressed on immune cells (41), which indicates that they are also expected to become therapeutic targets in the future, but scientists still need to further explore. At the end of this study, we also screened some antineoplastic drugs based on EPHB3 and TEAD1 genes, in order to provide more options for the medical treatment of OS patients.
Although this study provides potential clinical targets, there are some limitations in this study, and further experiments need to be conducted to verify our analysis results.
Conclusions
In this study, WGCNA was used to construct a co-expression module associated with survival in patients with OS. We identified unpreserved modules and key genes associated with poor prognosis in patients with OS. EPHB3, TEAD1, and KRR1P1 were screened by random survival forest and their effects on the prognosis of patients with OS were verified. This study also explored the roles of EPHB3, TEAD1, and KRR1P1 in the immune microenvironment of OS. In addition, this study also screened out targeted gene-related antitumor drugs, providing new molecular targets and intervention strategies for improving the prognosis of patients with OS.
Acknowledgments
Funding: None.
Footnote
Reporting Checklist: The authors have completed the REMARK reporting checklist. Available at https://atm.amegroups.com/article/view/10.21037/atm-22-399/rc
Conflicts of Interest: All authors have completed the ICMJE uniform disclosure form (available at https://atm.amegroups.com/article/view/10.21037/atm-22-399/coif). Jintao Huang is from OrigiMed Co., Ltd. The other authors have no conflicts of interest to declare.
Ethical Statement: The authors are accountable for all aspects of the work in ensuring that questions related to the accuracy or integrity of any part of the work are appropriately investigated and resolved. The study was conducted in accordance with the Declaration of Helsinki (as revised in 2013).
Open Access Statement: This is an Open Access article distributed in accordance with the Creative Commons Attribution-NonCommercial-NoDerivs 4.0 International License (CC BY-NC-ND 4.0), which permits the non-commercial replication and distribution of the article with the strict proviso that no changes or edits are made and the original work is properly cited (including links to both the formal publication through the relevant DOI and the license). See: https://creativecommons.org/licenses/by-nc-nd/4.0/.
References
- Ren C, Liu J, Zheng B, et al. The circular RNA circ-ITCH acts as a tumour suppressor in osteosarcoma via regulating miR-22. Artif Cells Nanomed Biotechnol 2019;47:3359-67. [Crossref] [PubMed]
- Huang C, Wang Q, Ma S, et al. A four serum-miRNA panel serves as a potential diagnostic biomarker of osteosarcoma. Int J Clin Oncol 2019;24:976-82. [Crossref] [PubMed]
- Papakonstantinou E, Stamatopoulos A, I, Athanasiadis D, et al. Limb-salvage surgery offers better five-year survival rate than amputation in patients with limb osteosarcoma treated with neoadjuvant chemotherapy. A systematic review and meta-analysis. J Bone Oncol 2020;25:100319. [Crossref] [PubMed]
- Chen X, Bahrami A, Pappo A, et al. Recurrent somatic structural variations contribute to tumorigenesis in pediatric osteosarcoma. Cell Rep 2014;7:104-12. [Crossref] [PubMed]
- Langfelder P, Horvath S. WGCNA: an R package for weighted correlation network analysis. BMC Bioinformatics 2008;9:559. [Crossref] [PubMed]
- Wan Q, Tang J, Han Y, et al. Co-expression modules construction by WGCNA and identify potential prognostic markers of uveal melanoma. Exp Eye Res 2018;166:13-20. [Crossref] [PubMed]
- Tian Z, He W, Tang J, et al. Identification of Important Modules and Biomarkers in Breast Cancer Based on WGCNA. Onco Targets Ther 2020;13:6805-17. [Crossref] [PubMed]
- Chen P, Wang F, Feng J, et al. Co-expression network analysis identified six hub genes in association with metastasis risk and prognosis in hepatocellular carcinoma. Oncotarget 2017;8:48948-58. [Crossref] [PubMed]
- Cotterill SJ, Wright CM, Pearce MS, et al. Stature of young people with malignant bone tumors. Pediatr Blood Cancer 2004;42:59-63. [Crossref] [PubMed]
- Longhi A, Errani C, De Paolis M, et al. Primary bone osteosarcoma in the pediatric age: state of the art. Cancer Treat Rev 2006;32:423-36. [Crossref] [PubMed]
- Chou AJ, Kleinerman ES, Krailo MD, et al. Addition of muramyl tripeptide to chemotherapy for patients with newly diagnosed metastatic osteosarcoma: a report from the Children's Oncology Group. Cancer 2009;115:5339-48. [Crossref] [PubMed]
- Meyers PA, Schwartz CL, Krailo MD, et al. Osteosarcoma: the addition of muramyl tripeptide to chemotherapy improves overall survival--a report from the Children's Oncology Group. J Clin Oncol 2008;26:633-8. [Crossref] [PubMed]
- Lamplot JD, Denduluri S, Qin J, et al. The Current and Future Therapies for Human Osteosarcoma. Curr Cancer Ther Rev 2013;9:55-77. [Crossref] [PubMed]
- Chou WC, Cheng AL, Brotto M, et al. Visual gene-network analysis reveals the cancer gene co-expression in human endometrial cancer. BMC Genomics 2014;15:300. [Crossref] [PubMed]
- Jägle S, Rönsch K, Timme S, et al. Silencing of the EPHB3 tumor-suppressor gene in human colorectal cancer through decommissioning of a transcriptional enhancer. Proc Natl Acad Sci U S A 2014;111:4886-91. [Crossref] [PubMed]
- Rodilla V, Villanueva A, Obrador-Hevia A, et al. Jagged1 is the pathological link between Wnt and Notch pathways in colorectal cancer. Proc Natl Acad Sci U S A 2009;106:6315-20. [Crossref] [PubMed]
- Fre S, Pallavi SK, Huyghe M, et al. Notch and Wnt signals cooperatively control cell proliferation and tumorigenesis in the intestine. Proc Natl Acad Sci U S A 2009;106:6309-14. [Crossref] [PubMed]
- Rönsch K, Jägle S, Rose K, et al. SNAIL1 combines competitive displacement of ASCL2 and epigenetic mechanisms to rapidly silence the EPHB3 tumor suppressor in colorectal cancer. Mol Oncol 2015;9:335-54. [Crossref] [PubMed]
- Batlle E, Bacani J, Begthel H, et al. EphB receptor activity suppresses colorectal cancer progression. Nature 2005;435:1126-30. [Crossref] [PubMed]
- Cortina C, Palomo-Ponce S, Iglesias M, et al. EphB-ephrin-B interactions suppress colorectal cancer progression by compartmentalizing tumor cells. Nat Genet 2007;39:1376-83. [Crossref] [PubMed]
- Zhang G, Liu X, Li Y, et al. EphB3-targeted regulation of miR-149 in the migration and invasion of human colonic carcinoma HCT116 and SW620 cells. Cancer Sci 2017;108:408-18. Retraction in: Cancer Sci 2018;109:483. [Crossref] [PubMed]
- Bai N, Zhang C, Liang N, et al. Yes-associated protein (YAP) increases chemosensitivity of hepatocellular carcinoma cells by modulation of p53. Cancer Biol Ther 2013;14:511-20. [Crossref] [PubMed]
- Xia Y, Zhang YL, Yu C, et al. YAP/TEAD co-activator regulated pluripotency and chemoresistance in ovarian cancer initiated cells. PLoS One 2014;9:e109575. [Crossref] [PubMed]
- Wang C, Nie Z, Zhou Z, et al. The interplay between TEAD4 and KLF5 promotes breast cancer partially through inhibiting the transcription of p27Kip1. Oncotarget 2015;6:17685-97. [Crossref] [PubMed]
- Knight JF, Shepherd CJ, Rizzo S, et al. TEAD1 and c-Cbl are novel prostate basal cell markers that correlate with poor clinical outcome in prostate cancer. Br J Cancer 2008;99:1849-58. [Crossref] [PubMed]
- Yin L, Li W, Xu A, et al. SH3BGRL2 inhibits growth and metastasis in clear cell renal cell carcinoma via activating hippo/TEAD1-Twist1 pathway. EBioMedicine 2020;51:102596. [Crossref] [PubMed]
- Jasra N, Sanyal SN, Khera S. Effect of thiabendazole and fenbendazole on glucose uptake and carbohydrate metabolism in Trichuris globulosa. Vet Parasitol 1990;35:201-9. [Crossref] [PubMed]
- An Y, Furber KL, Ji S. Pseudogenes regulate parental gene expression via ceRNA network. J Cell Mol Med 2017;21:185-92. [Crossref] [PubMed]
- Poliseno L, Salmena L, Zhang J, et al. A coding-independent function of gene and pseudogene mRNAs regulates tumour biology. Nature 2010;465:1033-8. [Crossref] [PubMed]
- Chan WL, Yuo CY, Yang WK, et al. Transcribed pseudogene ψPPM1K generates endogenous siRNA to suppress oncogenic cell growth in hepatocellular carcinoma. Nucleic Acids Res 2013;41:3734-47. [Crossref] [PubMed]
- Chakravarthi BV, Dedigama-Arachchige P, Carskadon S, et al. Pseudogene Associated Recurrent Gene Fusion in Prostate Cancer. Neoplasia 2019;21:989-1002. [Crossref] [PubMed]
- Kyyamova RG, Filonenko VV. Tumor-associated antigens and development of immunotherapeutics strategies. Biopolym Cell 2005;21:220-9. [Crossref]
- Zhang C, Zheng J H, Lin Z H, et al. Profiles of immune cell infiltration and immune-related genes in the tumor microenvironment of osteosarcoma. Aging 2020;12:3486. [Crossref] [PubMed]
- Liu D, Vadgama J, Wu Y. Basal-like breast cancer with low TGFβ and high TNFα pathway activity is rich in activated memory CD4 T cells and has a good prognosis. Int J Biol Sci 2021;17:670-82. [Crossref] [PubMed]
- Ali HR, Chlon L, Pharoah PD, et al. Patterns of Immune Infiltration in Breast Cancer and Their Clinical Implications: A Gene-Expression-Based Retrospective Study. PLoS Med 2016;13:e1002194. [Crossref] [PubMed]
- Jansen CS, Prokhnevska N, Master VA, et al. An intra-tumoral niche maintains and differentiates stem-like CD8 T cells. Nature 2019;576:465-70. [Crossref] [PubMed]
- de Andrea CE, Schalper KA, Sanmamed MF, et al. Immunodivergence in Metastatic Colorectal Cancer. Cancer Cell 2018;34:876-8. [Crossref] [PubMed]
- Dey A, Varelas X, Guan KL. Targeting the Hippo pathway in cancer, fibrosis, wound healing and regenerative medicine. Nat Rev Drug Discov 2020;19:480-94. [Crossref] [PubMed]
- Guo X, Zhao Y, Yan H, et al. Single tumor-initiating cells evade immune clearance by recruiting type II macrophages. Genes Dev 2017;31:247-59. [Crossref] [PubMed]
- Darling TK, Lamb TJ. Emerging Roles for Eph Receptors and Ephrin Ligands in Immunity. Front Immunol 2019;10:1473. [Crossref] [PubMed]
- Yu G, Luo H, Wu Y, et al. Mouse ephrinB3 augments T-cell signaling and responses to T-cell receptor ligation. J Biol Chem 2003;278:47209-16. [Crossref] [PubMed]
(English Language Editor: C. Betlazar-Maseh)