Establishment and validation of a novel immune-related prognostic signature in malignant pleural mesothelioma
Introduction
Malignant pleural mesothelioma (MPM) is a rare malignant thoracic cavity cancer closely related to asbestos exposure and has an exceedingly poor prognosis with a median survival of 9.5 months (1). Cisplatin combined with pemetrexed systemic chemotherapy is used as the first-line treatment for the treatment of advanced MPM, and although this extends the median survival of patients who cannot undergo surgical resection (2,3), the overall 5-year survival rate is lower than 5% (4). While the gold standard for diagnosis of MPM is thoracoscopic biopsy, it is not suitable for some patients due to weakness, comorbidities, or personal choice, and there are currently few methods to evaluate and predict the prognosis of the disease. Therefore, there is considerable value in establishing novel and predictive signatures that can reliably estimate clinical outcomes in monitoring personalized prognosis and guiding clinical management of MPM patients.
As a promising antitumor strategy, immunotherapy has provided new methods for treating solid tumors in the past few years and has obtained long-lasting responses and long-term survival benefits for countless cancer patients (5). Immune checkpoints have shown promise as therapeutic targets for various types of tumors, and related studies have shown that genetic changes in MPM, including point mutations, minute deletions, and copy number changes, may lead to new antigens. In view of the crosstalk between tumor cells and the immune microenvironment is indeed closely related to pathogenesis of MPM (6,7), a better understanding of this immune microenvironment with targeted molecular vulnerabilities is particularly associated with the improvement of treatment approaches and patient outcomes. Due to the related factors and the clonal expansion of tumor-infiltrating T lymphocytes support the concept of MPM as an immunogenic disease (8-10), and related studies highlighted the prognostic role of lymphocytes and the occurrence of immunosuppression in the disease (11-13). Therefore, an immune-related prognostic model for MPM is worth exploring. Although there is a similar study has reported a signature for predicting overall survival (OS) of MPM (14), are there other mRNAs that affect the prognosis of patients? Compared with traditional features (such as stage, T-, N-, M-stage), how accurate is the prognosis prediction signature based on these mRNAs? These are the questions that our research needs to explore.
In our study, based on the immune-related genes (IRGs) of MPM in The Cancer Genome Atlas (TCGA) database, we identified and validated an immune-related 5-gene signature and verified the stability of the model through the external validator GSE2549. In addition, we analyzed the correlation between genes in the model and some clinical features and immune cell infiltration. We used comprehensive analysis to develop a new marker based on cancer immune genomics characteristics to predict MPM prognosis and provide targets for its diagnosis and prognosis. We present the following article in accordance with the TRIPOD reporting checklist (available at https://atm.amegroups.com/article/view/10.21037/atm-22-527/rc).
Methods
Data collection
We obtained the sequencing data and corresponding clinical information of MPM in TCGA database (https://cancergenome.nih.gov/) and acquired the microarray dataset GSE2549 (15), GSE51024 (16) from the Gene Expression Omnibus (GEO) database (https://www.ncbi.nlm.nih.gov/geo/). As all data are publicly available, ethics committee approval was not required. After converting the ID in TCGA into gene symbols according to the GENCODE file, a list of IRGs was obtained from the Immunology Database and Analysis Portal (ImmPort) (17). The study was conducted in accordance with the Declaration of Helsinki (as revised in 2013).
Data analyzing and identification of IRGs
Using the “limma” (18) package in R to obtain differential expression genes (DEGs) from GSE51024, the cut-off criteria for screening DEGs were |log2(fold change)| ≥1 and P<0.05. Differential expression IRGs (DEIRGs) were obtained through the intersection of IRGs and DEGs from GSE51204.
Establishment of DEIRGs prognostic signature
We established the DEIRGs signature for predicting the prognosis of MPM patients. Firstly, the “survival” R package was used to perform univariate Cox analysis to screen out genes that were significantly relevant to the OS (P<0.05), and multivariate Cox regression analysis was then performed to obtain the coefficient. According to the multivariate Cox regression analysis results, a prognostic signature was established, and the risk score calculated based as follows = regression coefficients1 × expression level of mRNA1 + regression coefficients2 × expression level of mRNA2 +… + regression coefficientsn × expression level of mRNAn.
Validation of the prognostic signature
To verify the prediction accuracy of the signature, the risk score of MPM patients was obtained from the prognostic signature, and patients were divided into high-risk groups and low-risk groups according to the median. The receiver operating characteristic (ROC) curve and area under the ROC curve (AUC value) were performed to evaluate the predictive value of the prognostic signature, and the external validation dataset GSE2549 was used to validate it.
Validation of the independence of the prognostic signature and nomogram analysis
To detect whether the risk score of patients evaluated by the prognostic signature of IRGs was independent of the clinical characteristics as a prognostic factor of MPM, the risk score and clinical characteristics (age, gender, T stage, N stage, and clinical stage) were combined. The “pheatmap” R package was used to analyze the relationship between the molecular characteristics of MPM patients and other clinical variables in the high-risk and low-risk groups, and the “rms” (19) R package based on the multivariate Cox regression analysis was used to construct the nomogram based on independent prognostic factors, including risk score and M stage. The performance of the nomogram was evaluated by using the ROC curve, and the predictive accuracy of the signature we constructed was evaluated by comparing it with the signature built by previous research (20,21).
Analysis of signature and clinicopathological characteristics
To further explore the correlation between the different groups and clinicopathological characteristics, patients were grouped according to their clinical information and the survival status of the high-risk and low-risk patients was compared.
Immune cells infiltration analysis
To explore the relationship between genes and immune cell infiltration levels in the constructed signature, we used the single-sample gene set enrichment analysis (ssGSEA) algorithm in the “GSVA” package (22) to quantify the relative abundance of 10 immune cell types (23) infiltration in MPM. Pearson correlation analysis was used to detect the correlation between the gene expression level and the infiltration of each immune cell.
GSEA enrichment analysis
Pathway differences between the high-risk and low-risk groups were investigated by GSEA enrichment analysis (24,25). mRNA expression microarray in the high- and low-risk groups in MPM patients was taken as the expression dataset, and c2.cp.kegg.v7.4.symbols.gmt was selected as the enrichment analysis gene set to run GSEA software. Displacement test times were set as 1,000, and a gene set with false discovery rate (FDR) <0.25 was considered significant enrichment.
Statistical analyses
All statistical analyses and related visualization were conducted to determine independent prognostic factors using the R package (R software version 3.6.3) and Perl (5.30.1) software.
Results
Identification of IRGs
MPM data in TCGA has only tumor patients and lacks normal tissues for comparison. Therefore, GSE51024 was analyzed by using the “limma” package in R, and by setting the criterion of |log2(fold change)| >1 and P<0.05 and comparing the tumor tissue with normal tissue, a total of 1,212 DEGs were obtained. We then intersected the 1,811 IRGs downloaded from the Immport database with the 1,212 DEGs to obtain 101 differentially expressed IRGs (DEIRGs) (Figure S1, Table 1). Relevant information of patients is shown in Table 2.
Table 1
Differential expression immune-related genes |
EDN1, IGF1, CXCL3, FGR, CXCL13, A2M, TEK, BIRC5, C3, SORT1, KL, THRB, EDNRA, PPBP, OXTR, FGFR3, IL6R, ADRB1, CTSB, SEMA3G, PDGFD, SPP1, SFTPD, PTGS2, AGER, IL20RA, IL18, BMP2, TNFRSF19, FGFR4, AREG, CXCL17, NR3C2, CRABP2, IL17RD, SCG2, CXCL2, WFDC2, FABP6, TGFBR3, CAT, LYZ, MARCO, CLEC11A, S100A12, BMP5, AGTR2, PROCR, PTGER3, CXCL14, PLAU, S100P, CLDN4, PLA2G2A, PLTP, HBEGF, TNFSF10, SLC40A1, IL1RL1, SDC4, S100A14, CSF3, EDNRB, CCL20, ARRB1, SCGB3A1, ADRB2, MUC4, S100A8, FGF14, ANGPT1, DUOX1, PDGFRL, RORA, TNFSF4, INHBA, LIFR, DMBT1, S1PR1, FGF9, SDC1, CDH1, TNFSF13B, AZGP1, CX3CL1, ICAM1, CALCRL, FGF18, LTF, CXCL1, DKK1, IL7R, VCAM1, ROBO2, IL33, PGC, CXCL5, FABP4, KITLG, VIPR1, SEMA3C |
DEGs, differential expression genes.
Table 2
Variables | TCGA | GSE2549 |
---|---|---|
Number of patients | 84 | 39 |
Age (years), median [range] | 64 [28–82] | NA |
Gender | ||
Female | 15 | NA |
Male | 69 | NA |
Status | ||
Alive | 12 | 8 |
Dead | 72 | 31 |
Clinical stage | ||
1 | 10 | NA |
2 | 15 | NA |
3 | 43 | NA |
4 | 16 | NA |
T stage | ||
T1 | 14 | NA |
T2 | 24 | NA |
T3 | 31 | NA |
T4 | 13 | NA |
Unknow | 2 | NA |
N stage | ||
N0 | 43 | NA |
N1 | 10 | NA |
N2 | 25 | NA |
N3 | 3 | NA |
Unknown | 3 | NA |
M stage | ||
M0 | 77 | NA |
M1 | 7 | NA |
MPM, malignant pleural mesothelioma; TCGA, The Cancer Genome Atlas; NA, not available.
Development and validation of DEIRGs signature
The prognostic value of DEIRGs in MPM was discussed according to their prominent role in the tumor microenvironment. We performed univariable Cox regression to analyze the 101 DEIRGs and survival data from 82 patients, and based on the univariable Cox regression, obtained 21 prognosis-related DEIRGs. Subsequently, based on multivariate Cox regression analysis, we finally obtained five DEIRGs; BIRC5, SORT1, CAT, INHBA, and TNFSF13B, to construct a prognostic signature. Based on the regression coefficients, risk score = (0.0889 × expression of BIRC5) + (0.0523 × expression of SORT1) + (−0.0358 × expression of CAT) + (0.0446 × expression of INHBA) + (−0.0222 × expression of TNFSF13B). According to the median value of the risk score, all patients were grouped into the high-risk and low-risk groups. By drawing the survival curve, the OS of the high-risk group was significantly lower than the low-risk group (Figure 1A). According to Liu’s research (26), we found that in multiple clinical evaluation indicators such as relapse-free survival (RFS), progression-free survival (PFS), and disease-free interval (DFI), patients with high-risk groups had shorter survival time compared to patients with low-risk groups (Figure 1B-1D). The ROC curve used to evaluate the predictive value of the signature showed good accuracy with 1 year (AUC =0.853), 2 years (AUC =0.881), and 3 years (AUC =0.914) (Figure 1E). The heatmap shows and compares the expression characteristics of the five genes signature between high-risk groups and low-risk groups (Figure 1F), and the death sample survival time decreased with an increase in the risk score are illustrated in Figure 1G,1H. The results show the constructed signature in the independent validation set GSE2549 and demonstrate patients in the high-risk group had shorter OS compared with low-risk groups (Figure 1I). The GSE2549 dataset was also used to evaluate the performance of the DEIRGs signature (Figure 1J-1L), and each patient’s risk score was calculated with a signature.
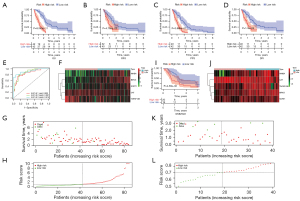
Independent prognostic factor analysis and construction of nomogram
To evaluate the role of the DEIRGs signature in some common clinicopathological features such as age, sex, T stage, N stage, M stage, and tumor stage, univariate and multivariate Cox regression analyses were performed, and the results confirm that the risk score was an independent prognostic indicator of the TCGA cohort (Figure 2A,2B). Taking into account the significance of the clinical utility we constructed, we drew the decision curve analysis (DCA) curve, and as shown in Figure 2C, the signature combined with the risk was more beneficial than other factors (age, gender, stage). Further, based on multivariate Cox regression analysis results, we established a novel nomogram incorporating two independent factors to predict the 1-, 2-, and 3-year survival rates of MPM patients (Figure 2D), and the AUC values for OS were 0.852, 0.875, and 0.878, respectively (Figure 2E-2G). In comparing the prediction performance of multiple signatures, these results show the prediction performance of our signature is superior to those previously published (Figure 3).
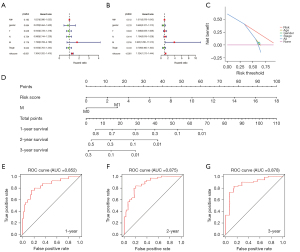
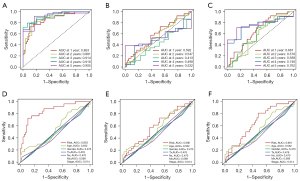
Subgroup analysis of DEIRGs signature for survival prediction
Subgroup analysis was performed to assess the prognostic value of the DEIRGs signature within the same clinicopathological risk factors by assigning patients into different subgroups, including a younger group (age ≤65 years), an elder group (age >65 years), a male group, an advanced-stage group (stage III & IV), a group with patients of T1 & T2, a group with patients of T3 & T4, and a group with patients of M0. Subgroup analysis demonstrated the DEIRGs signature could still distinguish patients into different survival groups within all subgroups with statistically significant prognostic ability (Figure 4).
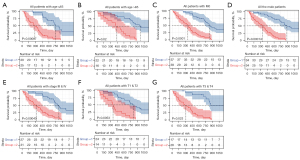
Signature associated with clinical characteristics
The heatmap revealed the levels of genes in the high-risk group and low-risk groups in the TCGA MPM cohort were different. However, the association between the high-risk and low-risk groups and T, N, M, stage, and other clinicopathological characteristics were not statistically significant (Figure 5A). Through survival analysis of the genes in the signature, we found that the high expression of BIRC5, SORT1, and INHBA was related to poor prognosis in MPM, while the high expression of CAT and TNFSF13B was closely related to a better OS (Figure 5B-5F). In addition, compared with patients with lymph node metastasis (Nx), the expression level of CAT in patients without lymph node metastasis (N0) was lower, and the expression level of SORT1 in patients without distant metastasis was higher than in patients with distant metastasis. BIRC5, INHBA, and risk score had higher expression levels in patients who died than in those who survived (Figure 5G-5K).
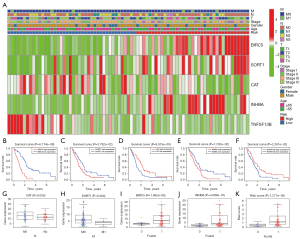
Immune infiltration analysis
By analyzing the data of immune infiltration results downloaded from TIMER, we found that, according to the difference of high- and low-risk, the different types of immune cells calculated by algorithms in different databases (TIMER, QUANTISEQ, MCPCOUNTER, XCELL, and EPIC) were also different (Figure 6A). To further explore the correlation between the DEIRG signature and the level of immune infiltration, ssGSEA was used to calculate ten immune cell infiltration scores. The high-risk group was positively correlated with the scores of three immune cells, including activated CD4 T cells, immature dendritic cells, and type 2 T helper cells, and negatively associated with natural killer cell (P<0.05, Figure 6B). We further explored the correlation between the expression level of genes in the signature and the level of immune cell infiltration, and the results showed that the gene in the signature had a significant correlation with natural killer cells and immature dendritic cells except for CAT. In addition, the correlation between BIRC5 and type 2 T helper cells was as high as 0.93, and there was a negative correlation between CAT and activated CD4 T cell and type 2 T helper cells (Figure 6C-6G).
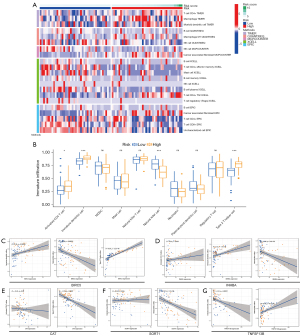
GSEA analysis between high-risk and low-risk groups
We also performed GSEA to explore the biological signal pathway between the high-risk and low-risk groups. The results showed that the significantly enriched pathways in the high-risk group involved cell cycle, DNA replication, extracellular matrix (ECM) receptor interaction, focal adhesion, homogeneous recombination, the P53 signaling pathway, regulation of actin cytoskeleton, and the transforming growth factor-β (TGF-β) signaling pathway (FDR <0.01) (Figure 7).
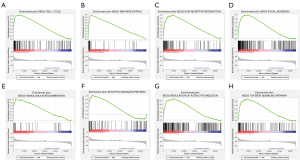
Discussion
MPM is a very rare malignant tumor, for which current treatments of surgery, chemotherapy, and radiotherapy provide limited clinical efficacy and a poor prognosis. As exploring molecular biomarkers is of great significance to the early diagnosis, prognosis prediction, and treatment strategies of MPM, we constructed a prognostic signature in this study consisting of five immune genes (INHBA, CAT, SORT1, TNFSF13B, and BIRC5).
Based on the relevant data of MPM in TCGA, we used univariate and multivariate cox regression analysis to build a prognostic signature by five genes, including INHBA, CAT, SORT1, TNFSF13B, and BIRC5. In this signature, the ROC values of 1, 2, and 3 years were 0.853, 0.881, and 0.914, respectively, showing good accuracy. In comparing the prediction performance of multiple signatures, these results show the prediction performance of our signature is superior to those previously published. Furthermore, low-risk patients had better OS than those in the high-risk group, and compared with high-risk patients, low-risk patients also showed the advantage of longer survival in RFS, PFS, and DFI. We also used an independent GEO dataset to verify the predictability of the signature, and a nomogram was drawn to provide a personalized estimate of the potential survival rate and contribute to the personalized management of MPM. Tumor-infiltrating cells have been proven to have vital functions in cancer, and we analyzed the immune infiltration levels of patients in different risk groups. This showed they were in activated CD4 T cells, immature dendritic cells, and type 2 T helper cells, and the fraction of the high-risk group was higher than that of the low-risk group. In natural killer T cells, the fraction of the low-risk group was higher than that of the high-risk group, while GSEA revealed that patients in high-risk and low-risk groups had different levels of enrichment pathways. Compared with the prognostic characteristics of MPM previously constructed, the signature established in this study had higher predictive performance.
Furthermore, in this prognostic signature, five genes (INHBA, CAT, SORT1, TNFSF13B, and BIRC5) were selected as crucial genes. SORT1 is a protein of the sortilin family, and according to existing reports, has different roles in different types of cancer. Targeted SORT1 has been reported to inhibit tumor metastasis and promotes tumor apoptosis in breast (27) and ovarian (28) cancer, while high expression of SORT1 may promote tumor progression in gastric cancer (29) and lung cancer (30). TNFSF13B is a member of the TNF ligands family and has been shown to play an important role in the proliferation and differentiation of B cells (31). While previous research on the TNFSF13B mainly focused on the immune system and hematological tumors, one study showed that as a B cell-activating cytokine, TNFSF13B could regulate T cell function by increasing T cell activation and TH1 polarization, enhancing the expression of pro-inflammatory leukocyte trafficking chemokine CCR6 and promoting memory phenotype, thereby enhancing antitumor immunity. Furthermore, TNFSF13B also has an apparent immune regulation function, promoting CD4+Foxp3+ spleen and Treg in the tumor microenvironment (32). In this study, TNFSF13B was used as a protective factor in MPM, and in the TISIDB database (33), we found that belimumab is an intravenous immunosuppressant for adjuvant treatment of systemic lupus erythematosus by acting on the target of TNFSF13B, which also provides new ideas for treating the disease. BIRC5, also known as survivin, is a negative regulatory protein that prevents apoptosis (34) and can regulate cancer development by inhibiting cell apoptosis and inducing cell proliferation (35). The abnormal amplification of BIRC5 has been observed in a variety of malignant tumors, and its overexpression is closely related to tumor occurrence and development, radiotherapy and chemotherapy resistance, and the poor prognosis of cancer patients (36-38). Recently, BIRC5 has attracted much attention as a molecular target for cancer therapy. CAT (catalase) is a key enzyme for H2O2 metabolism, and its expression and localization changes significantly in tumors (39). It can also ameliorate this hypoxic environment and reverse the malignant phenotype of tumor cells (40). INHBA is a member of the TGF-β superfamily of the gene encoding protein, and increasing reports have demonstrated it is a crucial factor in some solid malignant tumors, including prostate cancer (41), esophagus squamous cell carcinoma (42), and bladder cancer (43). Chen’s study found that knocking down the expression of INHBA could inhibit gastric cancer progression through the TGF-β signaling pathway (44), and another study found that interfering with the expression of INHBA could significantly reduce the degree of cell proliferation, invasion, and migration of colon cancer (45). In this study, we found a high expression of INHBA was related to the poor prognosis of MPM patients, which raises the question of whether reducing its expression could prolong their survival.
The GSEA showed patients in the high-risk group concentrated on the P53 signaling pathway, which is probably the most commonly inactivated protein in cancer (46,47), and TP53 mutations are associated with poor OS (48). Related study has shown that miR-320a is significantly inhibited in MPM samples, P53 can target PD-L1 by mediating miR-320a (49), and PD-L1 is highly expressed in mesothelioma and tumor stroma. P53 is associated with poor survival (50-52), providing a new direction for treating MPM. In addition, microRNA-215-5p can be used to suppress the progression of mesothelioma through the MDM2-p53-signal axis (53).
Inevitably, this study has some limitations. Firstly, the prognostic model proposed was established and verified using data from public databases and requires verification in clinical trials. Secondly, there remains a need for in vivo and in vitro experiments to further understand the biological function of the role of the DEIRG signature in MPM.
Conclusions
We constructed a prognostic signature and nomogram of MPM patients which can predict the prognosis of individual cases. Moreover, exploring the relationship between high and low-risk group immune cell infiltration and clinicopathologic features provides a new direction for guiding individualized and accurate treatment.
Acknowledgments
We are very grateful to the TCGA and GEO databases for providing valuable data resources to enable us to perform this study.
Funding: None.
Footnote
Reporting Checklist: The authors have completed the TRIPOD reporting checklist. Available at https://atm.amegroups.com/article/view/10.21037/atm-22-527/rc
Conflicts of Interest: All authors have completed the ICMJE uniform disclosure form (available at https://atm.amegroups.com/article/view/10.21037/atm-22-527/coif). The authors have no conflicts of interest to declare.
Ethical Statement: The authors are accountable for all aspects of the work in ensuring that questions related to the accuracy or integrity of any part of the work are appropriately investigated and resolved. The study was conducted in accordance with the Declaration of Helsinki (as revised in 2013).
Open Access Statement: This is an Open Access article distributed in accordance with the Creative Commons Attribution-NonCommercial-NoDerivs 4.0 International License (CC BY-NC-ND 4.0), which permits the non-commercial replication and distribution of the article with the strict proviso that no changes or edits are made and the original work is properly cited (including links to both the formal publication through the relevant DOI and the license). See: https://creativecommons.org/licenses/by-nc-nd/4.0/.
References
- Woolhouse I, Bishop L, Darlison L, et al. British Thoracic Society Guideline for the investigation and management of malignant pleural mesothelioma. Thorax 2018;73:i1-i30. [Crossref] [PubMed]
- Signorelli D, Macerelli M, Proto C, et al. Systemic approach to malignant pleural mesothelioma: what news of chemotherapy, targeted agents and immunotherapy? Tumori 2016;102:18-30. [Crossref] [PubMed]
- Vogelzang NJ, Rusthoven JJ, Symanowski J, et al. Phase III study of pemetrexed in combination with cisplatin versus cisplatin alone in patients with malignant pleural mesothelioma. J Clin Oncol 2003;21:2636-44. [Crossref] [PubMed]
- Milano MT, Zhang H. Malignant pleural mesothelioma: a population-based study of survival. J Thorac Oncol 2010;5:1841-8. [Crossref] [PubMed]
- Arora S, Velichinskii R, Lesh RW, et al. Existing and Emerging Biomarkers for Immune Checkpoint Immunotherapy in Solid Tumors. Adv Ther 2019;36:2638-78. [Crossref] [PubMed]
- Xu X, Cheng L, Fan Y, et al. Tumor Microenvironment-Associated Immune-Related Genes for the Prognosis of Malignant Pleural Mesothelioma. Front Oncol 2020;10:544789. [Crossref] [PubMed]
- Désage AL, Karpathiou G, Peoc'h M, et al. The Immune Microenvironment of Malignant Pleural Mesothelioma: A Literature Review. Cancers (Basel) 2021;13:3205. [Crossref] [PubMed]
- Mansfield AS, Peikert T, Smadbeck JB, et al. Neoantigenic Potential of Complex Chromosomal Rearrangements in Mesothelioma. J Thorac Oncol 2019;14:276-87. [Crossref] [PubMed]
- Carbone M, Yang H, Gaudino G. Does Chromothripsis Make Mesothelioma an Immunogenic Cancer? J Thorac Oncol 2019;14:157-9. [Crossref] [PubMed]
- Yoshikawa Y, Emi M, Hashimoto-Tamaoki T, et al. High-density array-CGH with targeted NGS unmask multiple noncontiguous minute deletions on chromosome 3p21 in mesothelioma. Proc Natl Acad Sci U S A 2016;113:13432-7. [Crossref] [PubMed]
- Anraku M, Cunningham KS, Yun Z, et al. Impact of tumor-infiltrating T cells on survival in patients with malignant pleural mesothelioma. J Thorac Cardiovasc Surg 2008;135:823-9. [Crossref] [PubMed]
- Yamada N, Oizumi S, Kikuchi E, et al. CD8+ tumor-infiltrating lymphocytes predict favorable prognosis in malignant pleural mesothelioma after resection. Cancer Immunol Immunother 2010;59:1543-9. [Crossref] [PubMed]
- Ujiie H, Kadota K, Nitadori JI, et al. The tumoral and stromal immune microenvironment in malignant pleural mesothelioma: A comprehensive analysis reveals prognostic immune markers. Oncoimmunology 2015;4:e1009285. [Crossref] [PubMed]
- Hiltbrunner S, Mannarino L, Kirschner MB, et al. Tumor Immune Microenvironment and Genetic Alterations in Mesothelioma. Front Oncol 2021;11:660039. [Crossref] [PubMed]
- Bueno R, De Rienzo A, Dong L, et al. Second generation sequencing of the mesothelioma tumor genome. PLoS One 2010;5:e10612. [Crossref] [PubMed]
- Suraokar MB, Nunez MI, Diao L, et al. Expression profiling stratifies mesothelioma tumors and signifies deregulation of spindle checkpoint pathway and microtubule network with therapeutic implications. Ann Oncol 2014;25:1184-92. [Crossref] [PubMed]
- Bhattacharya S, Andorf S, Gomes L, et al. ImmPort: disseminating data to the public for the future of immunology. Immunol Res 2014;58:234-9. [Crossref] [PubMed]
- Ritchie ME, Phipson B, Wu D, et al. limma powers differential expression analyses for RNA-sequencing and microarray studies. Nucleic Acids Res 2015;43:e47. [Crossref] [PubMed]
- Zhang Z, Kattan MW. Drawing Nomograms with R: applications to categorical outcome and survival data. Ann Transl Med 2017;5:211. [Crossref] [PubMed]
- Bai Y, Wang X, Hou J, et al. Identification of a Five-Gene Signature for Predicting Survival in Malignant Pleural Mesothelioma Patients. Front Genet 2020;11:899. [Crossref] [PubMed]
- Zhou JG, Zhong H, Zhang J, et al. Development and Validation of a Prognostic Signature for Malignant Pleural Mesothelioma. Front Oncol 2019;9:78. [Crossref] [PubMed]
- Hänzelmann S, Castelo R, Guinney J. GSVA: gene set variation analysis for microarray and RNA-seq data. BMC Bioinformatics 2013;14:7. [Crossref] [PubMed]
- Bindea G, Mlecnik B, Tosolini M, et al. Spatiotemporal dynamics of intratumoral immune cells reveal the immune landscape in human cancer. Immunity 2013;39:782-95. [Crossref] [PubMed]
- Mootha VK, Lindgren CM, Eriksson KF, et al. PGC-1alpha-responsive genes involved in oxidative phosphorylation are coordinately downregulated in human diabetes. Nat Genet 2003;34:267-73. [Crossref] [PubMed]
- Subramanian A, Tamayo P, Mootha VK, et al. Gene set enrichment analysis: a knowledge-based approach for interpreting genome-wide expression profiles. Proc Natl Acad Sci U S A 2005;102:15545-50. [Crossref] [PubMed]
- Liu J, Lichtenberg T, Hoadley KA, et al. An Integrated TCGA Pan-Cancer Clinical Data Resource to Drive High-Quality Survival Outcome Analytics. Cell 2018;173:400-416.e11. [Crossref] [PubMed]
- Rhost S, Hughes É, Harrison H, et al. Sortilin inhibition limits secretion-induced progranulin-dependent breast cancer progression and cancer stem cell expansion. Breast Cancer Res 2018;20:137. [Crossref] [PubMed]
- Ghaemimanesh F, Ahmadian G, Talebi S, et al. The effect of sortilin silencing on ovarian carcinoma cells. Avicenna J Med Biotechnol 2014;6:169-77. [PubMed]
- Liang M, Yao W, Shi B, et al. Circular RNA hsa_circ_0110389 promotes gastric cancer progression through upregulating SORT1 via sponging miR-127-5p and miR-136-5p. Cell Death Dis 2021;12:639. [Crossref] [PubMed]
- Al-Akhrass H, Naves T, Vincent F, et al. Sortilin limits EGFR signaling by promoting its internalization in lung cancer. Nat Commun 2017;8:1182. [Crossref] [PubMed]
- Ferraccioli G, Gremese E. B cell activating factor (BAFF) and BAFF receptors: fakes and facts. Clin Exp Immunol 2017;190:291-2. [Crossref] [PubMed]
- Yarchoan M, Ho WJ, Mohan A, et al. Effects of B cell-activating factor on tumor immunity. JCI Insight 2020;5:136417. [Crossref] [PubMed]
- Ru B, Wong CN, Tong Y, et al. TISIDB: an integrated repository portal for tumor-immune system interactions. Bioinformatics 2019;35:4200-2. [Crossref] [PubMed]
- Yamamoto H, Ngan CY, Monden M. Cancer cells survive with survivin. Cancer Sci 2008;99:1709-14. [Crossref] [PubMed]
- Ungvári I, Hadadi E, Virág V, et al. Implication of BIRC5 in asthma pathogenesis. Int Immunol 2012;24:293-301. [Crossref] [PubMed]
- Erpolat OP, Gocun PU, Akmansu M, et al. High expression of nuclear survivin and Aurora B predicts poor overall survival in patients with head and neck squamous cell cancer. Strahlenther Onkol 2012;188:248-54. [Crossref] [PubMed]
- Jia G, Wang Y, Yu Y, et al. Long non-coding RNA NR2F1-AS1 facilitates the osteosarcoma cell malignant phenotype via the miR-485-5p/miR-218-5p/BIRC5 axis. Oncol Rep 2020;44:1583-95. [Crossref] [PubMed]
- Yan L, Zhao Q, Liu L, et al. Expression of SIRT1 and survivin correlates with poor prognosis in esophageal squamous cell carcinoma. Medicine (Baltimore) 2020;99:e21645. [Crossref] [PubMed]
- Glorieux C, Calderon PB. Catalase, a remarkable enzyme: targeting the oldest antioxidant enzyme to find a new cancer treatment approach. Biol Chem 2017;398:1095-108. [Crossref] [PubMed]
- Song X, Xu J, Liang C, et al. Self-Supplied Tumor Oxygenation through Separated Liposomal Delivery of H2O2 and Catalase for Enhanced Radio-Immunotherapy of Cancer. Nano Lett 2018;18:6360-8. [Crossref] [PubMed]
- Zhao Z, Wang K, Tan S. microRNA-211-mediated targeting of the INHBA-TGF-β axis suppresses prostate tumor formation and growth. Cancer Gene Ther 2021;28:514-28. [Crossref] [PubMed]
- Lyu S, Jiang C, Xu R, et al. INHBA upregulation correlates with poorer prognosis in patients with esophageal squamous cell carcinoma. Cancer Manag Res 2018;10:1585-96. [Crossref] [PubMed]
- Lee HY, Li CC, Huang CN, et al. INHBA overexpression indicates poor prognosis in urothelial carcinoma of urinary bladder and upper tract. J Surg Oncol 2015;111:414-22. [Crossref] [PubMed]
- Chen ZL, Qin L, Peng XB, et al. INHBA gene silencing inhibits gastric cancer cell migration and invasion by impeding activation of the TGF-β signaling pathway. J Cell Physiol 2019;234:18065-74. [Crossref] [PubMed]
- Guo J, Liu Y. INHBA promotes the proliferation, migration and invasion of colon cancer cells through the upregulation of VCAN. J Int Med Res 2021;49:3000605211014998. [Crossref] [PubMed]
- Pentimalli F. Updates from the TP53 universe. Cell Death Differ 2018;25:10-2. [Crossref] [PubMed]
- Levine AJ. Reviewing the future of the P53 field. Cell Death Differ 2018;25:1-2. [Crossref] [PubMed]
- Quetel L, Meiller C, Assié JB, et al. Genetic alterations of malignant pleural mesothelioma: association with tumor heterogeneity and overall survival. Mol Oncol 2020;14:1207-23. [Crossref] [PubMed]
- Costa C, Indovina P, Mattioli E, et al. P53-regulated miR-320a targets PDL1 and is downregulated in malignant mesothelioma. Cell Death Dis 2020;11:748. [Crossref] [PubMed]
- Currie AJ, Prosser A, McDonnell A, et al. Dual control of antitumor CD8 T cells through the programmed death-1/programmed death-ligand 1 pathway and immunosuppressive CD4 T cells: regulation and counterregulation. J Immunol 2009;183:7898-908. [Crossref] [PubMed]
- Mansfield AS, Roden AC, Peikert T, et al. B7-H1 expression in malignant pleural mesothelioma is associated with sarcomatoid histology and poor prognosis. J Thorac Oncol 2014;9:1036-40. [Crossref] [PubMed]
- Awad MM, Jones RE, Liu H, et al. Cytotoxic T Cells in PD-L1-Positive Malignant Pleural Mesotheliomas Are Counterbalanced by Distinct Immunosuppressive Factors. Cancer Immunol Res 2016;4:1038-48. [Crossref] [PubMed]
- Singh A, Bhattacharyya N, Srivastava A, et al. MicroRNA-215-5p Treatment Suppresses Mesothelioma Progression via the MDM2-p53-Signaling Axis. Mol Ther 2019;27:1665-80. [Crossref] [PubMed]
(English Language Editor: B. Draper)