Non-targeted metabonomic analysis of plasma in patients with atherosclerosis by liquid chromatography-mass spectrometry
Introduction
Cardiovascular disease (CVD) has the highest incidence and mortality rates worldwide (1). The most important risk factors for CVD are dyslipidemia, hypertension, smoking, stress, diabetes, obesity (especially abdominal fat distribution), a lack of exercise, unhealthy eating habits, and excessive drinking (2). The primary pathological basis of most CVD is atherosclerosis (AS) (3). AS is a progressive disease that mainly involves the large and middle arteries (4), and is characterized by the accumulation of lipid and inflammatory factors in the arterial wall (5). Inflammation and lipid metabolism accompany and cooperate with each other to promote the progression of AS. Inflammatory mediators can affect changes in lipid metabolism, and inflammation obviously damages the process of reverse cholesterol transport. Cholesterol itself is an inflammatory factor. Excessive free cholesterol can activate the p38MAPK signaling pathway through the TLR3 or TLR4 pathway to induce inflammation. Abnormal lipid metabolism is another key pathogenic factor of AS. Previous studies have confirmed that elevated plasma low-density lipoprotein and cholesterol levels are highly correlated with AS (6). Sphingolipids and sphingolipid synthesis intermediates also play a key role in the pathogenesis of AS. The pathogenesis of AS (7) and the relationship between a series of external factors, such as diet, environment, susceptibility genes, and AS (8), have been extensively studied, but there is still no AS evaluation system.
Metabonomics is an essential part of systems biology after genomics, transcriptomics, and proteomics. It is also a hot topic in the field of metabolomics. The British Nicholson Research Group put forward the concept of metabonomics when analyzing rat urine components from the perspective of toxicology (9). Metabonomics is a technology used to study the metabolic pathway of a biological system by investigating changes in metabolites or changes in metabolites over time after the disturbance or stimulation of a biological system (e.g., a specific gene variation or environmental change) (9). Compared to traditional metabolic research, metabonomics combines knowledge of physics, biology, and analytical chemistry. It uses modern advanced instruments and analytical techniques to detect changes to the whole metabolite spectrum under specific conditions and studies overall biological function using a unique multivariate statistical analysis method. Metabonomics analyzes all the metabolites of organisms. Metabolites are produced by the reaction of endogenous substances. Thus, metabolite change also reveals endogenous substances or gene levels, which changes the research object from micro-genes to macro-metabolites. The study of macro-metabolic phenotypes makes research objects more intuitive (10). Metabonomics has become a powerful tool for the study of AS with complex pathophysiological characteristics. Many epidemiological studies have used this technique to accurately and comprehensively assess the effects of environmental factors on health outcomes, which used to be challenging to study. Metabolites are the intermediate products of various enzyme-catalyzed metabolic reactions in cells. Due to interactions between genetic variations and environments, metabonomics enables us to explore gene-environment interactions, and better understand AS and other multifactorial diseases (11). According to different research methods, metabolomics is divided into targeted metabolomics and non-targeted metabolomics. Non-targeted metabolomics can mainly analyze the ionization mode and stationary phase to produce the largest number of chromatographic features. With the improvement of non-targeted data processing software, the precision and qualitative accuracy of non-targeted analysis continue to improve. High substance detection coverage is the main feature and advantage of non-targeted omics.
Liquid chromatography-mass spectrometry (LC-MS) started later then, but has obvious advantages over, other metabonomic technologies. LC-MS is suitable for the analysis of metabolites with high throughput, high resolution, and high sensitivity. In MS scanning mode, the tandem-mass spectrometer can quickly switch between high- and low-collision energy, simultaneously collect the primary and secondary mass-spectrum information of metabolites, and when combined with the analysis of mass spectrum information by the metabonomic data processing software Progenesis QI v2.3 (Nonlinear Dynamics, Newcastle, UK), hundreds to thousands of metabolites can be detected and identified in a single analysis (12). The application of this technology to the research of coronary heart disease has achieved some results. Studies have confirmed that in patients with coronary heart disease, serum sphingomyelin (SM) is directly related to plasma cholesterol levels through non-targeted MS lipidomics studies of 2,998 serum samples (6). The results of this study confirm that sphingolipids play an important role in regulating blood lipid levels, and the biological markers in the sphingolipid metabolism pathway are new targets for the treatment of coronary heart disease (6).
Metabolic factors play an essential role in the pathogenesis and development of AS (13). Metabonomics comprehensively characterizes small molecule metabolites, such as amino acids, organic acids, nucleic acids, and lipids, providing an overview of the metabolic state and any pathophysiological changes, cells or biological systems. LC-MS, which is a high-throughput technique, has been introduced in epidemiological studies, and will extend understandings of the consequences of gene-environment interactions, and help to find novel biomarkers for the prevention and detection of AS and CVD (14-17). Changes in blood lipid metabolism are closely related to AS. This study used LC-MS technology to analyze the non-targeted plasma metabolites of patients with AS to provide insights into how to identify diagnostic target molecules and study the mechanism of AS. We present the following article in accordance with the MDAR reporting checklist (available at https://atm.amegroups.com/article/view/10.21037/atm-22-118/rc).
Methods
Samples
The morning fasting plasma of patients with AS treated at the Taihe Hospital from June 2019 to June 2020 was collected (the patient group). The morning fasting plasma of healthy individuals matched in terms of age and sex was collected at the health examination center (the control group). All the procedures in this study involving human participants were conducted in accordance with the Declaration of Helsinki (as revised in 2013). The study was approved by the Ethics Committee of the Taihe Hospital, Hubei University of Medicine (No. 2020-TH-055), and informed consent was obtained from all the patients.
The individuals in the healthy control group underwent coronary angiography, and were shown to have normal coronary arteries. Those in the patient group were diagnosed with CAD by angiography, and the complications and medications were evaluated based on self-reported information and written medical reports. Two experienced interventional cardiologists analyzed the evaluation and classification of the syntax scores. Except for age and gender, the other analysis variables were blind. Syntax scores were valuated with the internet-based SYNTAX calculator (version 2.28; https://www.syntaxcore.com/). Consistent with previous studies on the effects of different parameters on the complexity of CAD, we screened patients with a SYNTAX score ≥23 according to the low- and high-risk complexity of CAD, and excluded patients with acute myocardial infarction. The patient group comprised 38 males and 26 females, with an average age of 60.57±9.05 years. The control group comprised 14 males and 26 females, with an average age of 54.52±8.66 years.
Quality control (QC) samples
All samples were mixed equally as QC samples. In the process of MS, the QC samples were interspersed among the samples. The QC samples were used to evaluate the stability of the mass MS platform during the experiment.
Reagent
Methanol, formic acid, water, and acetonitrile were purchased from the Thermo company. L-2-chlorophenyl alanine was purchased from Shanghai Hengchung Biotechnology Co., Ltd. All chemicals and solvents were analytically pure or chromatographic grade.
Instrument
See Table 1 for information on the instruments used in the experiment.
Table 1
Instrument | Model and specification | Manufacturer |
---|---|---|
Ultrasonic cleaning machine | F-060SD | Shenzhen Fuyang Technology Group Co., Ltd. |
Vortex oscillator | TYXH-I | Shanghai Hannuo Instrument Co., Ltd. |
High-speed refrigerated centrifuge | TGL-16MS | Shanghai Luxianyi Centrifuge Instrument Co., Ltd. |
Freeze concentration centrifugal dryer | LNG-T98 | Taicang Huamei Biochemical Instrument Factory |
High-resolution mass spectrometer | QE plus | Thermo Fisher Scientific |
High-performance LC | Dionex U3000 UHPLC | Thermo Fisher Scientific |
Chromatographic column | ACQUITY UPLC HSS T3 | Waters |
LC, liquid chromatography.
Pretreatment
The samples were stored at −80 °C, and thawed at room temperature. One hundred µL of the samples were transferred, and 10 µL of internal standard solution (L-2-chlorophenyl alanine, 0.3 mg/mL, methanol configuration) was added. The samples were then vortexed for 10 s. Three hundred µL of protein precipitant methanol-acetonitrile (V:V =2:1) was added, and the samples were then vortexed for 1 min. Ultrasonic extraction was performed in an ice-water bath for 10 min, and the samples were then left to stand at −20 °C for 30 min, and then centrifuged for 10 min (13,000 rpm; 4 °C). Three hundred µL of the supernatant was added to the LC-MS sample vial and evaporated to dryness, after which it was reconstituted with 300 µL of methanol-water (V:V =1:4) (vortexed for 30 s, ultrasound for 3 min). The samples were allowed to stand at −20 °C for 2 hours, and then centrifuged for 10 min (13,000 rpm; 4 °C). One hundred and fifty µL of supernatant was taken with a syringe and filtered with a 0.22 µm organic phase pinhole filter, and then transferred to the LC injection vial. The samples were stored at −80 °C until the LC-MS analysis.
The QC samples were prepared by mixing the extracts of all the samples in equal volumes, and the volume of the QC was the same as that of the samples. All extraction reagents were pre-cooled at −20 °C before use.
LC-MS analysis conditions
The analytical instrument used in this experiment was the LC-MS system composed of Dionex U3000 UHPLC ultra-performance LC (UPLC)-tandem QE plus a high-resolution mass spectrometer. The chromatographic conditions were as follows: column: ACQUITY UPLC HSS T3 (100 mm × 2.1 mm; 1.8 µm). The column temperature was 45 °C. The mobile phase conditions were as follows: A-water (containing 0.1% formic acid), B-acetonitrile (containing 0.1% formic acid); flow rate: 0.35 mL/min; and injection volume: 2 µL. The MS conditions were as follows: used ion source: electrospray ionization (ESI); and mode for sample mass spectrum signal acquisition: positive and negative ion scanning.
Data analysis
Based on the QC samples, the pre-treatment of the LC-MS experimental part, samples on the computer, and the stability of the MS system were analyzed and evaluated. The ion peak with a relative standard deviation (RSD) >0.4 in the QC group was deleted. The RSD is the ratio of the standard deviation, and the arithmetic mean of the measurement results. It is a standard for measuring the dispersion degree of data distribution and the deviation degree of the data value from the arithmetic mean. Before pattern recognition, the original data were processed by metabonomic software Progenesis QI v2.3 to determine baseline filtering, peak recognition, integration, retention time correction, peak alignment, and normalization. The precursor tolerance’s main parameters were as follows: precursor tolerance: 5 ppm; product tolerance: 10 ppm; and product ion threshold: 5%. The identification of the compounds was based on the accurate mass number, secondary fragments, and isotopic distribution, and the Electron Microscopy Data Bank (EMDB) database was used for the qualitative analysis. The EMDB is a dedicated metabolite database for humans and animals. The database contains 3,600+ metabolites, including amino acids, lipids, nucleotides, carbohydrates, vitamins, cofactors, hormones, etc., including metabolite structure, and MS data, and is aimed at solving biological problems through metabonomics in a more professional way.
In the multivariate statistical analysis, an unsupervised principal component analysis (PCA) was conducted to observe the overall distribution of each sample and the stability of the whole analysis process. Next, a supervised partial least squares-discriminant analysis (PLS-DA) and an orthogonal partial least squares-discriminant analysis (OPLS-DA) were conducted to distinguish among the overall differences of the metabolic profiles among the groups and identify different metabolites in the groups. 7-fold cross-validation and 200 response permutation testing were used to evaluate the quality of the model. To avoid the risk of the supervised learning method obtaining the classification, we established a random ranking method for the OPLS-DA model to evaluate the accuracy of the OPLS model. We tested the OPLS-DA model for 200 times of response ranking, fixed the X matrix, and randomly arranged the variables (e.g., 0 or 1) of the previously defined classification y matrix, n times (n=200). The corresponding OPLS-DA model was established to obtain the R2 and Q2 values of the random model. Linear regression was conducted with the R2Y and Q2Y of the original model. The regression line and Y-axis intercept values (R2 and Q2, respectively) were used to measure whether the model was overfitted. Finally, a Loading diagram and Splot diagram were drawn to show the effect intensity and characteristics of metabolites on the comparison group. The Student’s t-test and fold change analysis were used to compare the metabolites between the two groups. The Kyoto Encyclopedia of Genes and Genomes (KEGG; https://www.kegg.jp/) database was used for path enrichment analysis. The KEGG IDs of different metabolites were used for the pathway enrichment analyses, and the results of the metabolic pathway enrichment analyses were obtained. A hypergeometric test was used to identify the pathway items that were significantly enriched in the significantly differentially expressed metabolites compared to the whole background. The following formula was used:
where N is the total number of metabolites, n is the number of differentially expressed metabolites in N, M is the number of metabolites annotated as a specific pathway, and M is the number of different metabolites annotated as a particular pathway. A P value ≤0.05 was set as the threshold; any pathway that met this condition was deemed to be significantly enriched in the different metabolites. The KEGG pathway mapper function was used to display the differential metabolic pathways, and the differential metabolites were colored according to the up-down information. The data analysis process is shown in Figure 1.
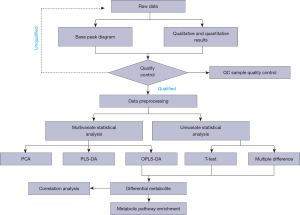
Statistical analysis
In this study, R software (V3.5.1) and related R packages were used for statistical analysis. P<0.05 indicates statistical significance.
Results
Results of sample statistical analysis
There were no significant differences in terms of age and gender between the patient group and the control group (see Table 1). The interference of age and gender was excluded.
QC results
In the QC sample, we detected various material peaks, which are illustrated in a Base Peak Chromatogram (BPC) diagram of the QC sample’s positive and negative ion mode QC1 in this experiment (see Figure 2A,2B). The instrument detection stability was good in the experimental process (see Figure 2C,2D). The PCA model diagram obtained by a 7-fold cross-validation (i.e., 7 cycles of cross-validation) showed that the QC samples were closely clustered, and the metabolite intensity of the QC samples was relatively strong. To more intuitively establish the relationship between the QC samples and the other samples, and the stability between the QC samples and the other samples, we performed a hierarchical clustering analysis of the expression of all the metabolites (see Figure 2E), which further confirmed that the detection process was stable and reliable.
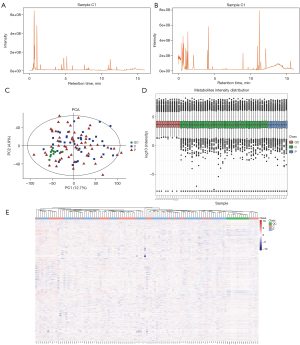
Qualitative and quantitative results
According to the extracted data, the ion peaks with missing values (0 value) >50% in the group were deleted, and the ‘0’ value was replaced by half of the minimum value. The qualitative compounds were screened according to the scores of the compound qualitative results. The screening standard was 36 points (the full score was 60 points). Qualitative results with a score <36 were regarded as inaccurate and were deleted. Finally, the positive and negative ion data were combined into a data matrix table containing all the information extracted from the original data used for the analysis. The subsequent investigation was based on the data matrix. In total, 14,348 types of substance peaks were detected, of which 6,917 types decreased, and 7,431 increased in the patient group. There were 1,126 types of metabolites, of which 411 types decreased, and 715 increased in the patient group (see Figure 3).
Multivariate statistical analysis results
The PCA chart showed that most of the original data of the patient group and the control group were gathered in the 95% confidence interval. The few discrete points proved that the repeatability of the same group of samples in the detection process was increased (see Figure 4A). The corresponding PLS-DA models had good quality and good prediction ability (see Figure 4B). There was a significant difference in the plasma metabolites between the patient and control groups. To filter out noise irrelevant to the classification information, improve the analytical ability and effectiveness of the model, and maximize the differences among the different groups within the model, we modified the PLS-DA by drawing an OPLS-DA score chart (see Figure 4C). The two groups of the samples differed significantly in the OPLS-DA score chart, and we found various variable weight in the projection (VIP) >1, P value <0.05. This study used a 7-fold cross-validation and 200 response ranking tests (see Figure 4D). R2 and Q2 were 0.918 and −0.634, respectively. The results showed that the model was stable without overfitting. To identify the effect intensity and correlations of metabolites in the comparison group, we created a load map and plots of the patient group and the control group (see Figure 4E,4F), and confirmed that the selected metabolites differed significantly between patients with AS and healthy individuals. These differential metabolites may be potential biomarkers of AS.
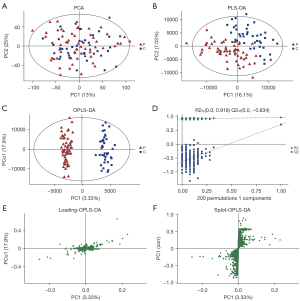
Screening results of different metabolites
According to the results of the quantitative analysis, to screen the differential metabolites, we visualized the P value and fold change value and drew a volcano map of the upregulated and downregulated metabolites (see Figure 5A). Four hundred and eleven metabolites in the patient group were more downregulated than those in the control group, and 715 metabolites in the patient group were more downregulated than those in the control group. On this basis, we set the screening criteria as the VIP value of the first principal component (PC) of the OPLS-DA model >1, P value of t-test <0.05, and screened 61 types of metabolites of biological significance and with significant differences (see Figure 5B-5D). More than half of the metabolites [34] were lipids, 10 types of carbon and oxygen compounds, 8 types of organic acids and derivatives, 4 types of organic heterocyclic compounds, 3 types of nitrogen-containing organic compounds, and 2 types of nucleotides and analogs. Among the 61 different metabolites, this study used |log2fold change| >1; P <0.05 as the standard, and screened 9 metabolites with the most significant differences, including 2-tetradecanone, pantothenol, all-trans-13,14-dihydroretinol, linoleoyl ethanolamide, n-oleoylethanolamine, 4-methyl-2-pentenal, Cer (d18:1/14:0), chenodeoxycholic acid glycine conjugate, and 5-acetamidovalerate. The 9 most significant metabolites may be potential markers of AS. Figure 5E shows the close correlations between the top 50 significant differences in the VIP values and the metabolites.
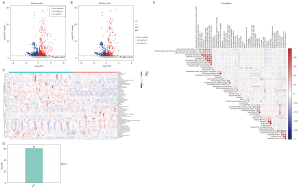
Enrichment analysis of metabolic pathways
By screening the 61 significant metabolites, we identified 17 enriched metabolic pathways that were significantly different between the patient and control groups (P<0.05), including choline metabolism in cancer, sphingolipid signaling pathway, glycerophospholipid metabolism, linoleic acid metabolism, neuroactive ligand-receptor interaction, basal cell carcinoma. There were significant differences between the patient and control groups (P<0.01; see Figure 6A,6B).
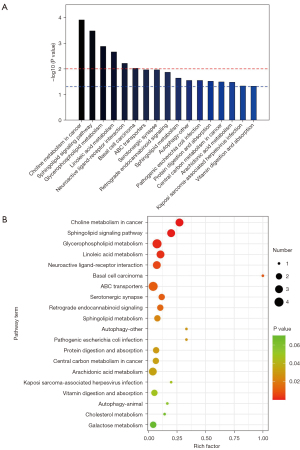
Discussion
Non-targeted metabonomics is a comprehensive analytical method that can detect, identify, and quantify metabolites in as many biological samples as possible (18,19). To this end, non-targeted methods aim to distinguish unique metabolite characteristics related to genotype, drug therapy, clinical subgroup, or other comparison groups, usually via comparisons to an appropriate control group. The broad coverage provided by non-targeted methods can identify new metabolic pathways, disease biomarkers, and drug-derived metabolites (20,21). However, non-targeted metabolomics approaches lack specific fragments for target metabolites. Thus, improper sample processing leads to biases in non-targeted metabolomic analysis results. Strict QC samples are essential if reliable results are to be obtained (11). In metabonomics, control measurement errors and deviations, such as sample collection, processing, transportation, and storage, can be occur at the pre-analysis stage. Hirayama et al. studied the effects of sampling procedures and storage conditions on the stability of plasma and serum metabonomics by capillary electrophoresis-MS (CE-MS), and noted that the spectrum in plasma was more stable than that in serum (22). Based on this, we collected the fasting venous plasma of the patients in the morning. We excluded the interference of age and gender factors in the patient group through the statistical analysis. As discussed above, we implemented QC measures to ensure the accuracy and credibility of the analysis results.
In this study, we compared a patient group to a healthy control group and identified 1,126 different metabolites. Of these metabolites, 411 were downregulated, and 715 were upregulated in the patient group. To further confirm these results, PCA, PLS-DA, and OPLS-DA models were used to verify that the selected metabolites differed significantly between patients with AS and healthy individuals. These differential metabolites may be potential biomarkers of AS.
Individual lipids are specific in their biological functions. Thus, quantitative lipidomics, which provides the structural details of single lipids is very important to revealing the precise lipid targets involved in disease pathogenesis. Based on a VIP >1 and a P<0.05, we identified 61 different metabolites between the two groups, of which 47 were upregulated and 14 were downregulated in the patient group. Among these metabolites, 34 (>50%) were lipid metabolites. The results suggest that lipid metabolites play an essential role in the pathogenesis of AS. Previous serum metabolomic studies showed that patients with coronary heart disease metabolize lipids, especially phospholipids, very differently to healthy people (23-25), which is consistent with our research results.
The changes of lipid metabolites will significantly affect the fluidity, thickness, and accumulation of cell membranes, and the dynamics and function of membrane proteins (26-29). Ganna et al. found that lysophosphatidylcholine (LPC) 18:1, LPC 18:2, monoglyceride (MG) 18:2, and SM were associated with coronary heart disease and were not associated with major cardiovascular risk factors. They not only found that these metabolites improved risk reclassification beyond traditional risk factors to a certain extent, but also suggested that there was an association between coronary heart disease-related single nucleotide polymorphism (SNP) and some LPCs (30). Another Italian-based lipidomic study measured 8 lipids [i.e., phosphatidylcholine (PC), LPC, cholesteryl ester (CE), SM, phosphatidylserine (PS), phosphatidylethanolamine (PE), lysophosphatidylethanolamine (LPE), and triglyceride (TAG)], and conducted a 10-year follow-up study of 702 participants, and found that 28 types of lipids were significantly associated with CVD. These lipids had an extensive overlap with those previously associated with advanced atherosclerotic plaques, such as PC (38:3). The Cox proportional hazards model was used to identify 3 lipids significantly associated with incidental CVD, including TAG (54:2), CE (16:1), and PE (36:5). Further, an analysis revealed that adding these 3 lipids to risk factors (e.g., age, gender, diabetes, smoking, systolic blood pressure, total cholesterol, and high-density lipoprotein cholesterol) significantly improved the risk identification and classification ability of the risk prediction model (31). A lipidomic analysis of the sarcoplasmic reticulum showed that the imbalance between PC and PE was related to decreased sarcoplasmic reticulum calcium triphosphatase activity, resulting in abnormal calcium homeostasis and coronary heart disease.
We conducted a KEGG pathway enrichment analysis to clarify the possible mechanism of the 61 differential metabolites screened in this study in the pathogenesis of AS. The results showed that the differentially expressed metabolites were significantly enriched in the choline metabolism, lipid metabolism, autophagy, amino acid metabolism, vitamin digestion, and absorption pathways. Notably, lipid metabolites, susceptible genes related to metabolic disorders, and lipid metabolic pathways have been shown to play important roles in atherosclerotic diseases (32,33). Similarly, studies have shown that the plasma choline pathway is highly correlated with cardiovascular risk (34-36). These results are consistent with our findings.
Our research had a number of limitations. First, the sample size was small, and needs to be expanded for further verification. Second, in vivo and in vitro experimental verification was not undertaken to confirm our results. Finally, the screening of differential metabolites and the investigation of the mechanism pathways need to be further studied to clarify the critical pathogenic metabolites and their mechanism.
Through the non-targeted metabolic analysis of the blood of patients with AS, our research can provide more strategies for the screening of AS markers and the study of the mechanism of occurrence, and provide more targets for treatment. For example, the differential metabolites we screened may be used as marker molecules for AS. Pathway enrichment results show that, in addition to the recognized lipid metabolism is closely related to its occurrence, it suggests that choline metabolism, autophagy pathway, amino acid metabolism, and vitamin digestion and absorption may all be closely related to the occurrence of CAD. It can provide more strategies for the study of its occurrence mechanism and provide more targets for treatment. The digestion and absorption of vitamins suggests that the intestinal flora may be closely related to it.
We found significant differences in the plasma non-targeted metabolites between patients with AS and healthy individuals. Differential metabolites may be potential biomarkers of AS and play important roles in the pathogenesis of AS. Our research identified some key differential expressed metabolites. The metabolites could be used to detect AS and as potential therapeutic targets. We intend to further explore and clarify the pathogenesis of AS in future studies.
Acknowledgments
Funding: This research was supported by the 2021 Guiding Scientific Research Project of Shiyan Science and Technology Bureau (No. 21Y02).
Footnote
Reporting Checklist: The authors have completed the MDAR reporting checklist. Available at https://atm.amegroups.com/article/view/10.21037/atm-22-118/rc
Data Sharing Statement: Available at https://atm.amegroups.com/article/view/10.21037/atm-22-118/dss
Conflicts of Interest: All authors have completed the ICMJE uniform disclosure form (available at https://atm.amegroups.com/article/view/10.21037/atm-22-118/coif). The authors have no conflicts of interest to declare.
Ethical Statement: The authors are accountable for all aspects of the work, including ensuring that any questions related to the accuracy or integrity of any part of the work have been appropriately investigated and resolved. All the procedures in this study involving human participants were conducted in accordance with the Declaration of Helsinki (as revised in 2013). The study was approved by the Ethics Committee of Taihe Hospital, Hubei University of Medicine (No. 2020-TH-055), and informed consent was obtained from all the patients.
Open Access Statement: This is an Open Access article distributed in accordance with the Creative Commons Attribution-NonCommercial-NoDerivs 4.0 International License (CC BY-NC-ND 4.0), which permits the non-commercial replication and distribution of the article with the strict proviso that no changes or edits are made and the original work is properly cited (including links to both the formal publication through the relevant DOI and the license). See: https://creativecommons.org/licenses/by-nc-nd/4.0/.
References
- Shaito A, Thuan DTB, Phu HT, et al. Herbal Medicine for Cardiovascular Diseases: Efficacy, Mechanisms, and Safety. Front Pharmacol 2020;11:422. [Crossref] [PubMed]
- Schenck-Gustafsson K. Risk factors for cardiovascular disease in women. Maturitas 2009;63:186-90. [Crossref] [PubMed]
- Emamat H, Totmaj AS, Tangestani H, et al. The effect of egg and its derivatives on vascular function: A systematic review of interventional studies. Clin Nutr ESPEN 2020;39:15-21. [Crossref] [PubMed]
- Skuratovskaia D, Vulf M, Komar A, et al. Promising Directions in Atherosclerosis Treatment Based on Epigenetic Regulation Using MicroRNAs and Long Noncoding RNAs. Biomolecules 2019;9:226. [Crossref] [PubMed]
- McLaren JE, Michael DR, Ashlin TG, et al. Cytokines, macrophage lipid metabolism and foam cells: implications for cardiovascular disease therapy. Prog Lipid Res 2011;50:331-47. [Crossref] [PubMed]
- Zalloua P, Kadar H, Hariri E, et al. Untargeted Mass Spectrometry Lipidomics identifies correlation between serum sphingomyelins and plasma cholesterol. Lipids Health Dis 2019;18:38. [Crossref] [PubMed]
- Yang X, Li Y, Li Y, et al. Oxidative Stress-Mediated Atherosclerosis: Mechanisms and Therapies. Front Physiol 2017;8:600. [Crossref] [PubMed]
- Frohlich J, Dobiasova M, Lear S, et al. The role of risk factors in the development of atherosclerosis. Crit Rev Clin Lab Sci 2001;38:401-40. [Crossref] [PubMed]
- Nicholson JK, Lindon JC, Holmes E. 'Metabonomics': understanding the metabolic responses of living systems to pathophysiological stimuli via multivariate statistical analysis of biological NMR spectroscopic data. Xenobiotica 1999;29:1181-9. [Crossref] [PubMed]
- Cui L, Lu H, Lee YH. Challenges and emergent solutions for LC-MS/MS based untargeted metabolomics in diseases. Mass Spectrom Rev 2018;37:772-92. [Crossref] [PubMed]
- Iida M, Harada S, Takebayashi T. Application of Metabolomics to Epidemiological Studies of Atherosclerosis and Cardiovascular Disease. J Atheroscler Thromb 2019;26:747-57. [Crossref] [PubMed]
- Vogeser M, Parhofer KG. Liquid chromatography tandem-mass spectrometry (LC-MS/MS)--technique and applications in endocrinology. Exp Clin Endocrinol Diabetes 2007;115:559-70. [Crossref] [PubMed]
- Barrington WT, Lusis AJ. Atherosclerosis: Association between the gut microbiome and atherosclerosis. Nat Rev Cardiol 2017;14:699-700. [Crossref] [PubMed]
- Griffin JL, Atherton H, Shockcor J, et al. Metabolomics as a tool for cardiac research. Nat Rev Cardiol 2011;8:630-43. [Crossref] [PubMed]
- Lewis GD, Asnani A, Gerszten RE. Application of metabolomics to cardiovascular biomarker and pathway discovery. J Am Coll Cardiol 2008;52:117-23. [Crossref] [PubMed]
- McGarrah RW, Crown SB, Zhang GF, et al. Cardiovascular Metabolomics. Circ Res 2018;122:1238-58. [Crossref] [PubMed]
- Shah SH, Kraus WE, Newgard CB. Metabolomic profiling for the identification of novel biomarkers and mechanisms related to common cardiovascular diseases: form and function. Circulation 2012;126:1110-20. [Crossref] [PubMed]
- Schrimpe-Rutledge AC, Codreanu SG, Sherrod SD, et al. Untargeted Metabolomics Strategies-Challenges and Emerging Directions. J Am Soc Mass Spectrom 2016;27:1897-905. [Crossref] [PubMed]
- Zeki ÖC, Eylem CC, Reçber T, et al. Integration of GC-MS and LC-MS for untargeted metabolomics profiling. J Pharm Biomed Anal 2020;190:113509. [Crossref] [PubMed]
- Wang R, Li B, Lam SM, et al. Integration of lipidomics and metabolomics for in-depth understanding of cellular mechanism and disease progression. J Genet Genomics 2020;47:69-83. [Crossref] [PubMed]
- Cheng S, Shah SH, Corwin EJ, et al. Potential Impact and Study Considerations of Metabolomics in Cardiovascular Health and Disease: A Scientific Statement From the American Heart Association. Circ Cardiovasc Genet 2017;10:e000032. [Crossref] [PubMed]
- Hirayama A, Sugimoto M, Suzuki A, et al. Effects of processing and storage conditions on charged metabolomic profiles in blood. Electrophoresis 2015;36:2148-55. [Crossref] [PubMed]
- Polonskaya YV, Shramko VS, Morozov SV, et al. Balance of Fatty Acids and Their Correlations with Parameters of Lipid Metabolism and Markers of Inflammation in Men with Coronary Atherosclerosis. Bull Exp Biol Med 2017;164:33-5. [Crossref] [PubMed]
- Yang J, Li X, Xu D. Research Progress on the Involvement of ANGPTL4 and Loss-of-Function Variants in Lipid Metabolism and Coronary Heart Disease: Is the "Prime Time" of ANGPTL4-Targeted Therapy for Coronary Heart Disease Approaching? Cardiovasc Drugs Ther 2021;35:467-77. [Crossref] [PubMed]
- Cai F, Ren F, Zhang Y, et al. Screening of lipid metabolism biomarkers in patients with coronary heart disease via ultra-performance liquid chromatography-high resolution mass spectrometry. J Chromatogr B Analyt Technol Biomed Life Sci 2021;1169:122603. [Crossref] [PubMed]
- Katsoulieris E, Mabley JG, Samai M, et al. alpha-Linolenic acid protects renal cells against palmitic acid lipotoxicity via inhibition of endoplasmic reticulum stress. Eur J Pharmacol 2009;623:107-12. [Crossref] [PubMed]
- Martín V, Fabelo N, Santpere G, et al. Lipid alterations in lipid rafts from Alzheimer's disease human brain cortex. J Alzheimers Dis 2010;19:489-502. [Crossref] [PubMed]
- Rysman E, Brusselmans K, Scheys K, et al. De novo lipogenesis protects cancer cells from free radicals and chemotherapeutics by promoting membrane lipid saturation. Cancer Res 2010;70:8117-26. [Crossref] [PubMed]
- Lam SM, Tian H, Shui G. Lipidomics, en route to accurate quantitation. Biochim Biophys Acta Mol Cell Biol Lipids 2017;1862:752-61. [Crossref] [PubMed]
- Ganna A, Salihovic S, Sundström J, et al. Large-scale metabolomic profiling identifies novel biomarkers for incident coronary heart disease. PLoS Genet 2014;10:e1004801. [Crossref] [PubMed]
- Stegemann C, Pechlaner R, Willeit P, et al. Lipidomics profiling and risk of cardiovascular disease in the prospective population-based Bruneck study. Circulation 2014;129:1821-31. [Crossref] [PubMed]
- Zhang LN, Liu PP, Zhou J, et al. Positive correlation between variants of lipid metabolism-related genes and coronary heart disease. Mol Med Rep 2013;8:260-6. [Crossref] [PubMed]
- Mathes P, Thiery J. The role of lipid metabolism in the prevention of coronary heart disease. Z Kardiol 2005;94 Suppl 3:III/43-55.
- Papandreou C, Bulló M, Hernández-Alonso P, et al. Choline Metabolism and Risk of Atrial Fibrillation and Heart Failure in the PREDIMED Study. Clin Chem 2021;67:288-97. [Crossref] [PubMed]
- Millard HR, Musani SK, Dibaba DT, et al. Dietary choline and betaine; associations with subclinical markers of cardiovascular disease risk and incidence of CVD, coronary heart disease and stroke: the Jackson Heart Study. Eur J Nutr 2018;57:51-60. [Crossref] [PubMed]
- Meyer KA, Shea JW. Dietary Choline and Betaine and Risk of CVD: A Systematic Review and Meta-Analysis of Prospective Studies. Nutrients 2017;9:711. [Crossref] [PubMed]
(English Language Editor: L. Huleatt)