BATF2 and PDK4 as diagnostic molecular markers of sarcoidosis and their relationship with immune infiltration
Introduction
Sarcoidosis (SA), a multisystem granulomatous disease with elusive etiology, is characterized histologically by non-caseating granulomas (1). In most cases, SA affects the lungs, but it is possible that any organ can be involved (2). In the past 30 years, SA-related mortality has risen, and respiratory failure is highly linked to SA-related deaths (3). The severity of pulmonary SA ranges from imaging abnormalities detected accidentally in patients presenting no symptoms, to chronic diseases that are difficult to treat (4). It is difficult to diagnose because SA can mimic many other diseases, including lymphoproliferative diseases and granulomatous infections. There is no special examination for its diagnosis, which depends on the correlation of clinical radiology and histopathological characteristics (5,6). For patients who require systemic treatment to manage their condition, clinicians, in most cases, administer corticosteroids as the first-line treatment. Antimetabolites are usually administered as alternative drugs for patients who do not respond to or are intolerant to corticosteroids (7). In fact, corticosteroid treatment is related to toxic effects, and toxic effects are related to cumulative dose and treatment time (8). The scarcity of reliable predictors for disease progression, lack of truly effective therapies, and individuality of patients pose great challenges to managing SA (9). Therefore, it is critical to identify early diagnostic biomarkers of SA.
A large number of studies have recently indicated that SA progression is highly linked to the infiltration of immune cells and inflammation. Studies on cellular players in the SA mechanism include both innate and adaptive immune cells (10). During granulomatous responses, several cytokines plus other mediators are released by T lymphocytes and activated macrophages (11). Granulomas are the pathological characteristic of SA. They are tightly packed cell clusters, forming the primary core of multinucleated giant cells, epithelioid histiocytes, and macrophages enclosed in a lymphocyte collar (12). Although CD4+ T-cells are majorly localized within the lymphocyte collar, a few B cells, CD8+ T-cells, fibroblasts, and plasma cells are also present in granulomas (13). The CD4+ T lymphocytes are crucial in SA progression as they recruit leukocytes, eventually generating granulomas, whose interaction with B cells stimulates the production of antibodies (14). Hence, from an immunological point of view, assessing the degree of immune cell infiltration and revealing how various infiltrating immune cell components are vital in determining the underlying molecular mechanism of SA and the development of novel immunotherapeutic targets. Cell-type Identification by Estimating Relative Subsets of RNA Transcripts (CIBERSORT) is a biological tool that adopts extensive deconvolution of data for the expression of genes and a sophisticated algorithm for quantifying various immune cells in different disease samples and substrates, in silico (15). To date, no previous studies have attempted to explore the infiltration of immune cells in SA using CIBERSORT.
Herein, we retrieved the microarray dataset of SA from the Gene Expression Omnibus (GEO) database, then carried out weighted gene co-expression network analysis (WGCNA) to find the key module. Then, machine learning approaches were used for extensive filtration and to uncover the diagnostic molecular markers of SA. SA, is easily confused with other pulmonary disease, such as tuberculosis. We validated the recommended diagnostic molecular markers in our clinical samples. Next, we applied CIBERSORT to evaluate the immune infiltration difference between SA patients and healthy control’s blood samples in 22 immune cell subsets. Consequently, the association of markers with infiltrating immune cells was studied to comprehend the immune mechanisms present in SA. We present the following article in accordance with the STARD reporting checklist (available at https://atm.amegroups.com/article/view/10.21037/atm-22-180/rc).
Methods
Data
Using the GEO database (https://www.ncbi.nlm.nih.gov/geo/), we chose 2 datasets relevant to SA for subsequent analyses. Notably, the GSE83456 (16) dataset based on the GPL10558 platform, including 49 SA patients and 61 normal individual blood samples, was used to investigate the immunologic mechanism in SA. Additionally, the GSE42834 (17) dataset includes 61 SA patients and 113 blood samples from normal individuals, which are also based on the GPL10558 platform, were used for the verification test. The arrays function within the limma package (https://bioconductor.org/packages/limma/) was explored for normalizing gene expression profiles in GSE83456 and GSE42834 (18). Besides, we employed the impute package (http://bioconductor.org/packages/impute/) as a supplement to the missing data.
Differentially expressed genes (DEGs) screening
To demonstrate the impact of inter-sample correction, we used a principal component analysis (PCA) cluster plot with 2 dimensions. The DEGs between SA and control were also revealed via lmFit and eBayes functions of the limma package. A heatmap and the volcano map of DEGs were generated via the ggplot2 (https://cran.r-project.org/ggplot2/index.html) and pheatmap (https://cran.r-project.org/web/packages/pheatmap/index.html) package was used to illustrate the differential expression of DEGs. The DEGs showing P<0.05 upon adjustment using the false discovery rate (FDR), and |log2fold change|>0.5 were regarded as significant.
WGCNA
A WGCNA explores necessary modules and key genes of overlapping genes. It adopts the topological overlapping measurements to reveal the corresponding expression modules and describe the pattern of gene correlation between different samples (19). The expression profile of DEGs, universally down-regulated or up-regulated in the SA and control groups, were extracted to perform WGCNA in GSE83456. Firstly, we used hclust function for hierarchical clustering analysis. Secondly, we utilized the pick soft threshold function to screen for the soft thresholding power value when constructing the module. Using candidate power (1 to 20), we determined the average connectivity degrees of various modules in addition to their independent traits. For any degree of independence above 0.8, a suitable power value was chosen. The WGCNA R package was employed in establishing the co-expression net-work (modules), with the minimum-sized module set to 30, whereas we issued a unique color label to each module. In WGCNA, we identified gene significance (GS) as the relationship between genes and phenotypes. Then, a module membership (MM): MM (i) = cor(x i, ME) was highlighted to evaluate essential gene functions in the module. Notably, the highest correlation index between Module membership and gene significance was used to screen key module.
Functional correlation analysis of key module
The Database for Annotation, Visualization and Integrated Discovery (DAVID; http://david.abcc.ncifcrf.gov/), which incorporates a comprehensive biological knowledge base and a employs series of analytic tools when extracting biological themes for proteins or genes (20), was used for Gene Ontology (GO) and Kyoto Encyclopedia of Genes and Genomes (KEGG) pathway enrichment analyses of the genes in the key module. The Benjamini-Hochberg (BH) method was used for adjusting the P values, and the adjusted P value <0.05 acted as the threshold for significant results. Visualization of results was compiled using the R ggplot2 package.
Screening and verifying diagnostic markers
The least absolute shrinkage and selection operator (LASSO) logistics regression and support vector machine (SVM) recursive feature elimination (SVM-RFE) are both classic methods in machine learning, and were executed to conduct feature selection for screening the diagnostic markers for SA. Moreover, the GSE42834 was used to verify the diagnostic efficiency of the obtained diagnostic markers. We used the LASSO algorithm via the glmnet package (https://cran.r-project.org/web/packages/glmnet/index.html). Additionally, SVM-RFE, which is a machine learning technique that relies on an SVM, was employed to reveal the optimal variations by eliminating SVM-generated eigenvectors (15). An SVM module was constructed to determine the diagnostic value of molecular markers in sarcoidosis using e1071 (https://cran.r-project.org/web/packages/e1071/index.html) and kernlab package (https://cran.r-project.org/web/packages/kernlab/index.html). Consequently, we choose an interaction of genes derived from SVM-RFE or LASSO algorithm for subsequent analyses. We considered a 2-sided P<0.05 to represent statistical significance.
Evaluating immune cell infiltration
By uploading of the GSE83456 data to CIBERSORT, samples with P>0.05 were eliminated and we obtained the immune cell infiltration matrix data. Furthermore, the ggplot2 package was used for the analysis of PCA clustering about immune cell infiltration quantitative data to generate a PCA clustering map with 2 dimensions. Next, we used corrplot package (https://cran.r-project.org/web/packages/corrplot/vignettes/corrplot-intro.html) to create a correlation heatmap to identify any relationships between 22 infiltrating immune cell types; thereafter, ggpolt2 package was employed to visualize violin plots to observe any difference in the immune cell infiltration.
Relationship analysis of diagnostic molecular markers with infiltrating immune cells
The corrplot package of R software was adopted for Spearman’s correlation analysis of the infiltrating immune cells as well as the diagnostic markers. The threshold was set as |r| >0.3 and P<0.05. The former result was visualized by the ggplot2 package.
Clinical specimen verification
Specimen source
The serum samples of 30 patients with active pulmonary SA were collected from the Department of Respiratory and Critical Care Medicine, the First Affiliated Hospital of Chengdu Medical College from January 2016 to December 2020. At the same time, the serum samples of 30 tuberculosis (TB) and 30 healthy patients were collected as the control group. The peripheral blood samples of all participant’s (10 mL) were collected by anticoagulant tube. After 30 min in a 4 °C refrigerator, the samples were centrifuged for 15 min at room temperature at 3,000 r, and the supernatant was retained and stored in the refrigerator at −80 °C. The inclusion criteria for patients with active pulmonary SA were as follows: (I) age greater than 18 years old, endobronchial ultrasound-guided transbronchial needle aspiration (EBUS-TBNA) biopsy, pathological diagnosis of pulmonary SA; (II) patients with good compliance and ability to cooperate with the examination. The exclusion criteria were as follows: (I) having been given glucocorticoid treatment; (II) complicated with infectious diseases, tumorous diseases, or immunodeficiency diseases; (III) severe cardiopulmonary insufficiency or blood coagulation disturbance. Patients with pulmonary TB were selected as control group 1, whose inclusion criteria were as follows: (I) met the diagnostic criteria in the Guidelines for Diagnosis and Treatment of Tuberculosis. Those complicated by connective tissue disorders, neoplastic disease, or immunocompromised disease were excluded. Healthy people who visited the hospital at the same period were recruited to control group 2.
This study was approved by the Ethics Committee of The First Affiliated Hospital of Chengdu Medical College (approval number 2021CYFYIRB-BA-14-01). All participants provided their written informed consent. All procedures performed in this study involving human participants were in accordance with the Declaration of Helsinki (as revised in 2013).
Detection of messenger RNA transcription level in clinical tissue samples of BATF2 and PDK4 by reverse transcription-quantitative polymerase chain reaction (RT-qPCR)
Total RNA was isolated using Trizol (Invitrogen, Grand Island, NY, USA). According to the instructions of complementary DNA (cDNA) synthesis kit (TaKaRa Bio, Shiga, Japan), the reverse transcription conditions of cDNA were as follows: 37 °C, 30 min, 95 °C, 5 min, −20 °C. The RT-qPCR preparation system was conducted as follows: 10 μL SYBR Green (TaKaRa), upstream and downstream primers 0.8 μL, 2 μL cDNA, and 6.4 μL without enzyme water. The RT-qPCR reaction was carried out in ABI 8000 real-time quantitative PCR (Thermo Fisher Scientific, Waltham, MA, USA), with glyceraldehyde 3-phosphate dehydrogenase (GAPDH) as the internal reference, and the reaction conditions were set as follows: pre-denaturation at 95 °C, 30 s at 95 °C, 5 s at 60 °C, 34 s at 60 °C, 40 cycles, and annealing at 60 °C for 30 s. The BATF2 upstream primer was 5'-CCTCTCCCGACAACCCTTC, downstream primer was GTGGACTTGAGCAGAGGAGA-3'; the PDK4 upstream primer was 5'-TTGGCTGGTTTTGGTTACGG, downstream primer was CACCAGTCAGCCTCAGA-3'; the GAPDH upstream primer was 5'-CCATCTTCCAGGAGCGAGAT, and downstream primer was TGCTGATGATCTTGAGGCTG-3'. Gene expression levels were calculated relative to the housekeeping gene GAPDH. The receiver operating characteristic (ROC) curve was drawn by MedCalc software (MedCalc Software Ltd., Ostend, Belgium), and the area under the ROC curve (AUC) was used to evaluate the diagnostic value of serum BATF2 messenger RNA (mRNA), PDK4 mRNA, and their combination in pulmonary SA.
Statistical analysis
The data were analyzed with GraphPad Prism 8 (GraphPad Inc., La Jolla, CA, USA), MedCalc 19.0.4, and R 3.6.1 (R Foundation for Statistical Computing, Vienna, Austria). Measurement data conforming to normal distribution were expressed as mean±standard deviation (x±s). Multiple group comparisons were performed by one-way analysis of variance (ANOVA) followed by the Bonferroni procedure for pairwise comparison, and the comparison between groups were performed using the Student’s t-test. Non-normally distributed data were expressed as the median and interquartile range, and the comparison between groups was conducted using the Wilcoxon-test. Kruskall-Wallis H test was used for comparison of multiple groups of non-normal data, and Nemenyi method was used for pairwise comparison thereon. The ROC curves were drawn and the AUC was used to evaluate the diagnostic value of serum BATF2 mRNA and PDK4 mRNA for active pulmonary SA. P value <0.05 was considered statistically significant.
Results
Differential expression analysis
After the 47,230 probes in GSE83456 were preprocessed, 20,936 genes were obtained. The results of PCA demonstrated that the clustering of the 2 sample groups was remarkable, suggesting the reliability of the sample source (Figure 1). After preprocessing the data, the R software was used to retrieve 764 DEGs from GSE83456, as depicted in the volcano map and heatmap (Figure 2A,2B).
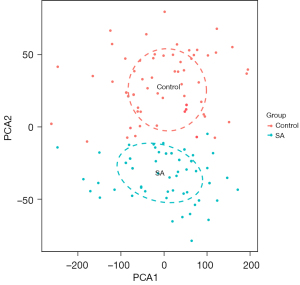
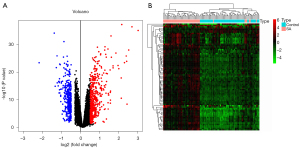
Sarcoidosis-associated module
To reveal the key module most associated with SA, WGCNA was conducted utilizing the expression profiles of the 764 DEGs. After merging the similar modules, we could identify a total of 6 modules and each module was displayed by distinct colors to distinguish different modules (Figure 3A). The blue module was most negatively associated with sarcoidosis [correlation coefficient =−0.92, P=3E-46; Figure 3B). Based on the absolute value of the GS in each module, the key module in SA was selected (blue module) (Figure 3C), and the module membership in the blue module was positively correlated with GS for SA (correlation coefficient =0.94, P<1E-200; Figure 3D). Thus, the blue module was classified a key module containing 580 genes.
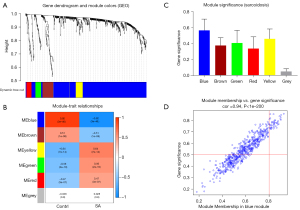
Functional correlation analysis of genes in the key module
The 580 genes of the key module were grouped into the following categories: biological process (BP; 8 BP terms were significantly enriched), molecular function (MF; 9 MF terms were significantly enriched), and cellular component (CC; 12 CC terms were significantly enriched) (Figure 4). The results of GO analysis indicated that the genes were primarily associated with “immune response”, “type I interferon signaling pathway”, “immunological synapse”, “innate immune response”, and so on. The KEGG pathway enrichment analysis (8 KEGG terms were statistically significantly enriched, Figure 5) showed that a major proportion of the genes were enriched in pathways such as “primary immunodeficiency” and “cytokine-cytokine receptor interaction.” These findings suggest that immune response may play a significant role in the progression of SA.
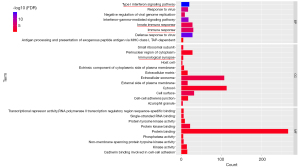
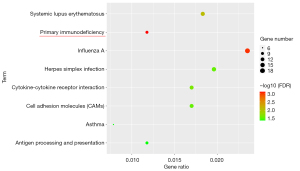
Screening and validating the diagnostic markers
We identified 22 genes from the key module as diagnostic markers for SA using the LASSO logistic regression algorithm method (Figure 6A), and lambda value (min =0.00436). A total of 25 genes were screened from the key module employing the SVM-RFE algorithm as diagnostic markers (Figure 6B). Notably, we overlapped gene markers found by 2 algorithms and eventually confirmed 2 diagnostic-related genes (PDK4 and BATF2) (Figure 6C). In GSE83456, the diagnostic power of BATF2 was 0.980 [AUC =0.980, 95% confidence interval (CI): 0.933 to 0.997], and the diagnostic power of PDK4 was 0.942 (AUC =0.942, 95% CI: 0.880 to 0.977) (Figure 7A). The accuracy and efficiency of the 2 diagnostic markers were validated using GSE42834 as an external validation test. The diagnostic power of BATF2 was 0.896 (AUC =0.896, 95% CI: 0.841 to 0.937), and that of PDK4 was 0.772 (AUC =0.942, 95% CI: 0.703 to 0.832) (Figure 7B). The summary of the 2 diagnostic markers is shown in Table 1.
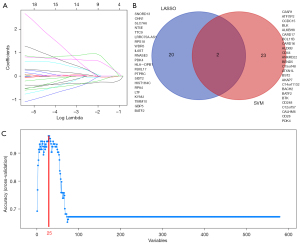
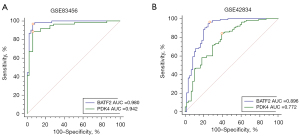
Table 1
Diagnostic markers | AUC | Youden index | Sensitivity (%) | Specificity (%) | 95% CI | |
---|---|---|---|---|---|---|
Lower limit | Upper limit | |||||
PDK4 (GSE83456) | 0.980 | 0.824 | 88.52 | 93.88 | 0.880 | 0.977 |
BATF2 (GSE83456) | 0.942 | 0.906 | 96.72 | 93.88 | 0.933 | 0.997 |
PDK4 (GSE42834) | 0.772 | 0.447 | 84.07 | 60.66 | 0.703 | 0.832 |
BATF2 (GSE42834) | 0.896 | 0.702 | 96.46 | 73.77 | 0.841 | 0.937 |
CI, confidence interval; AUC, area under the curve.
Immune cell infiltration
The PCA cluster analysis can be utilized to examine whether the biological repetition and the diversity of various groups concur. Herein, the PCA cluster assessment revealed a significant immune cell infiltration difference between the SA samples and the control samples (Figure 8A). The heatmap of the 22 types of immune cells suggested that activated natural killer (NK) cells, eosinophils, and mast cells resting had a positive correlation (Figure 8B). In addition, the violin plot of the immune cell infiltration difference indicated significant differences of immune infiltration between SA patients and the normal people’ blood samples. Quite notably, monocytes, NK cells activated, macrophages M0, macrophages M1, dendritic cells (DC) activated, mast cells resting, T cells regulatory (Tregs), and mast cells activated infiltrated more in SA patient than that in normal people. Conversely, T cell CD8, T cells CD4 naïve, T cells follicular helper, and neutrophils infiltrated less in SA patients than that in normal people (Figure 8C).
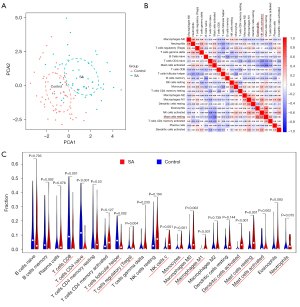
Correlation analysis between PDK4, BATF2, and infiltrating immune cells
We found that the BATF2 had a positive correlation with DC activated (r=0.378, P=0.007), macrophages M1 (r=0.376, P=0.008), monocytes (r=0.302, P=0.035) (Figure 9A-9C) and negatively correlated with macrophages M0 (r=0.508, P=1.975E-04) (Figure 9D); PDK4 was negatively correlated with T cells CD4 naive (r=−0.304, P=0.034), and positively correlated with DC resting (r=0.328, P=0.021), mast cells resting (r=0.307, P=0.032) (Figure 10A-10C), NK cells activated (r=0.306, P=0.033) (Figure 10D).
Expression of PDK4 and BATF2 in clinical serum specimens
Finally, there were 30 patients with pulmonary SA (13 males and 17 females) aged 46.2±10.4 years. A total of 30 patients (14 males and 16 females) were included in the TB control group, mean age, 48.8±12.3 years. A total of 30 healthy patients (15 males and 15 females) were included in the healthy control group, mean age, 50.4±10.8 years. There was no significant difference in age and gender between the 3 groups, which were comparable. Serum BATF2 mRNA and PDK4 mRNA of the 32 groups are shown in Figure 11. Serum PDK4 mRNA in the active pulmonary SA group was significantly higher than that in TB control group and healthy control group (P<0.05), but there was no significant difference between the TB control group and healthy control group (P>0.05). Serum BATF2 mRNA in patients with active pulmonary SA was higher than that in the tuberculosis control group, the difference was statistically significant (P<0.05), and the former 2 were higher than that in the healthy control group (P<0.05) (Figure 11A,11B).
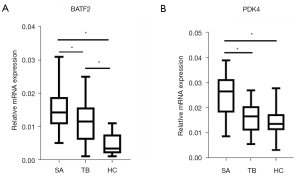
Compared with non-SA participants (TB + healthy population), the AUC of serum BATF2 mRNA, PDK4 mRNA, and their combination for the diagnosis of active pulmonary SA were 0.798 (95% CI: 0.701 to 0.876), 0.895 (95% CI: 0.813 to 0.950) and 0.910 (95% CI: 0.831 to 0.960) (Figure 12A). The sensitivity and specificity of the combination of BATF2 mRNA and PDK4 mRNA for the diagnosis of active pulmonary SA were 84% and 79%. It demonstrated that the combination of the 2 markers can be diagnostic biomarkers for active pulmonary SA. Compared with tuberculosis patients (TB), the AUC of serum BATF2 mRNA, PDK4 mRNA, and their combination for the diagnosis of active pulmonary SA were 0.654 (95% CI: 0.520 to 0.772), 0.796 (95% CI: 0.672 to 0.889) and 0.821 (95% CI: 0.703 to 0.908) (Figure 12B). The sensitivity and specificity of the combination of BATF2 mRNA and PDK4 mRNA for the diagnosis of active pulmonary SA were 75% and 70%. This indicated that the combination of the 2 markers can distinguish active pulmonary SA and tuberculosis well.
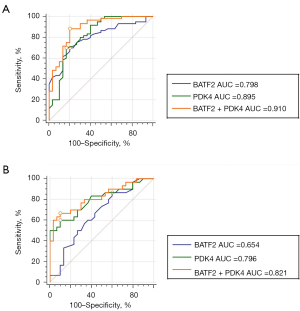
Discussion
As a granulomatous disease with multi-system and multi-organ involvement, SA often causes damage to the lungs, heart, liver, kidney, and central nervous system. The incidence of SA in women is higher compared to that in men (21). In recent years, due to the continuous deepening of clinicians’ understanding of SA and the gradual improvement of examination techniques, its rate of diagnosis has risen significantly. The diagnosis of SA often depends on radiological and clinical imaging, which are related to the histology of epithelioid granulomas; nevertheless, granulomas are not pathognomonic of SA (22). In particular, the imaging features of pulmonary SAs are similar to those of pulmonary TB and mediastinal lymph node TB (23). Therefore, pulmonary SA might be easily missed in diagnosis or misdiagnosed. Remarkably, there have recently been studies suggesting that immune cell infiltration serves a vital role in SA development (24,25). Thus, identifying special molecular biomarkers and exploring the immune cell infiltration pattern in SA has become urgent, which may be valuable to improve SA prognosis. With the rapid development of genome-sequencing technology, bioinformatics also provides strong support for the screening of molecular biomarkers, and CIBERSORT tools also provide favorable conditions for exploring immune cell infiltration patterns for various diseases. Herein, we attempted to reveal blood biomarkers capable of diagnosing SA. Meanwhile, the role of immune cell infiltration to SA will be further explored.
In this paper, we obtained the microarray gene expression data from GEO database and identified 764 DEGs between SA patients and 61 normal people’s blood samples. Besides, using WGCNA, we found the key module, the blue module, to be significantly correlated with SA. Based on the blue module, 580 genes of the module were screened. The GO analysis result indicated that these 580 genes were mainly associated with “type I interferon signaling pathway”, “innate immune response”, “immune response”, “immunological synapse”, and so on. The KEGG pathway enrichment analysis demonstrated that the majority of these genes were enriched in pathways such as “cytokine-cytokine receptor interaction” and “primary immunodeficiency”. Taken together, these results strongly implicate that the immune response is essential for SA. Greaves et al. demonstrated that one of the defining features of pulmonary SA is infiltration of activated CD4+ T cells in the lung tissue (24). Previously, Grunewald et al. had reported that SA is a systemic inflammatory disorder characterized by tissue infiltration of mononuclear phagocytes and lymphocytes which are associated with the formation of non-caseating granuloma (26). The results of our analysis data were in line with the above previous studies, confirming the robustness and reliability of the present findings.
The SVM-RFE, a wrapper technique employed in big data mining, utilizes a backward feature, which recursively eliminates insignificant traits from a larger subset (27). It has been shown to be a powerful tool in the identification of potential alterations and thus, the classification of healthy and SA groups. The LASSO logistic regression is a widely applied method for the regression of high-dimensional data. It imposes a constraint, λ, on the size of the regression coefficients β in ordinary least squares (ordinary least squares regression). Based on WGCNA analysis, we used 2 algorithms to screen feature variables and establish a reliable classification model. The PDK4 and BATF2 genes were identified as diagnostic markers of SA. Although PDK4 and BATF2 were selected simply by combining SVM-RFE and LASSO, their diagnostic powers were reliable upon GSE42834 validation. These findings were also validated in clinical samples, suggesting that the presence of PDK4 and BATF2 in peripheral blood has significant value in the diagnosis of SA. Pulmonary SA and TB are the 2 major diseases that clinicians need to distinguish as pathological manifestations of granulomatous diseases. This study included 30 patients with TB for differential diagnosis. The results showed that the serum BATF2 level of pulmonary SA patients were higher than that of the TB control group and healthy control group, and the TB patients also had higher levels than those of the healthy control group. At the same time, the serum PDK4 level of pulmonary SA patients were higher than that of the 2 control groups, and the difference between the 2 control groups was not statistically significant. When BATF2 and PDK4 were combined in diagnosis, pulmonary SA and TB could be better differentiated. Gong et al. (28) showed that BATF2 combined with UBE2L6, SERPING1, and VAMP5 could be used for the screening of TB, because BATF2 was highly expressed in the TB patients compared with the healthy control group. This conclusion is consistent with the results of the present study. In this study, the level of BATF2 in serum of patients with pulmonary SA was higher than that of TB patients, the possible reason is that pulmonary SA and TB have different pathogenesis, but the specific mechanism still needs to be further studied.
Pyruvate dehydrogenase kinase 4 (PDK4), an important mitochondrial enzyme, impedes the acetyl-CoA production via selective inhibition of pyruvate dehydrogenase activity via phosphorylation. It can regulate the glycolysis pathway and affects cell metabolism, proliferation, and apoptosis (29). Some studies have reported that inactivation of the pyruvate dehydrogenase complex by overexpression of PDK4 contributes to hyperglycemia. Therefore, the serious health problems associated with diabetes and PDK may play an essential role in hyper catecholamine-induced insulin resistance in the periadrenal adipose tissues of pheochromocytoma patients (30,31). Another study revealed that PDK4 is indispensable in dictating the fate of tumor necrosis factor/nuclear factor-κB (TNF/NF-κB)-mediated hepatocyte apoptosis. Meanwhile, this pro-survival pathway switches to pro-apoptosis mediated by PDK4-deficiency (32). It could be that TNF exerts significant effects in SA development through the maintenance of chronic pro-inflammatory status in macrophages. Activated macrophages can be transformed into epithelioid cells and multinucleated macrophages, resulting in granuloma formation (33). Given that PDK4 interacts with inflammatory factors, we propose the hypothesis that PDK4 potentially contributes to the regulation of the pathological process of sarcoidosis. A transcription factor belonging to the BATF family, BATF2 has previously been characterized and reported to inhibit tumor growth by suppressing AP-1 activity (34). Recent reports have highlighted BATF2 as a vital agent in innate immune responses and are essential as a transcription factor during gene regulation. It also exhibits effector functions in classical activation of macrophages (35). Functional studies by Guler et al. revealed a predominant role of BATF2 in regulating Th2 cell functions and lineage development of T lymphocytes (36). Kayama et al. also reported that BATF2 in innate myeloid cells was a crucial molecule that suppressed interleukin (IL)-23/IL-17 pathway-mediated adaptive intestinal pathology (34). Studies have revealed several T-cell-associated cytokines in SA immunopathogenesis; however, increasing reports showed that IL-12 cytokine family members such as IL-12, IL-23, IL-27, and IL-35 were closely associated with SA (37). Findings from earlier studies showed that PDK4 and BATF2 might be related to the development of SA, thus could be utilized as potential diagnostic markers for SA. However, additional clinical reports are warranted to validate the diagnostic capability of PDK4 and BATF2. Corticosteroids are the mainstay of treatment for sarcoidosis. To our knowledge, treating sarcoidosis with targeted therapy has not been widely considered. Therefore, this study might at least provide some objective basis for the discussion of the relationship between targeted therapy and Sarcoidosis.
Sarcoidosis is a chronic granulomatous disease with an aberrant immune response to undefined environmental or infectious triggers (7). To further assess how immune cell infiltration is linked to SA, we employed CIBERSORT for in-depth exploration of SA immune infiltration. It was demonstrated that infiltration of T cells regulatory (Tregs), NK cells activated, monocytes, macrophages M0, macrophages M1, DC activated, mast cells resting, and mast cells activated were elevated, and a decreased infiltration of T cell CD8, T cells CD4 naïve, T cells follicular helper, and neutrophils may lead to SA. Understanding the role of immune responses in the pathogenesis of pulmonary sarcoidosis is important. The immune system response initiates with the activation of antigen-presenting cells (APCs), especially alveolar macrophages, in the lungs. Alveolar macrophages present the specific antigenic peptides in association with human leukocyte antigen molecules to specific T cells (38). In addition to the alveolar macrophages which play a critical role in granuloma formation, increased activation of T cells, NK cells and mast cells could promote this process as well (39). The cytokine IL-33 ameliorates the regulatory effects of T cells in the occurrence of pulmonary SA. Previous studies have shown that the pulmonary SA exerts a Th1/Th17/regulatory T cells (Tregs)-driven inflammatory process in the lung, inducing noncaseating granulomas that comprise CD4+ T cells (14). Tøndell et al. found that healthy control participants' NK T cell fractions of leucocyte were lower than that in SA patients (40). These conclusions are consistent with those of the present study. Additionally, Lepzien et al. suggested that mast cells, monocytes, and DC-are likely critical in SA through the initiation and maintenance of T cell activation, thereby participating in granuloma formation driven via cytokine production (41). By combining results obtained in this study, we propose that SA is closely related to inflammation and immune dysregulation. Moreover, the present findings uncovered a detailed mechanism of 22 immune cell types in SA. Activated NK cells and eosinophils infiltration are tightly linked to mast cells resting infiltration. Immunophenotyping and functional analysis of these cells may enhance our understanding of the immune biology of sarcoidosis and elucidate novel immune subsets that could be targeted in new treatment approaches. However, the actual mechanisms underlying the highlighted correlations should be confirmed via experimental assessments. When we analyzed the correlation between PDK4, BATF2, and immune cells, notably, BATF2 had a remarkably positive correlation with DC, macrophages M1, and monocytes, whereas it had a negative correlation with macrophages M0. Additionally, PDK4 showed a significantly negative correlation with T cells CD4 naive and significantly positively correlated with NK cells activated, DC resting, and mast cells resting. Researchers have found that DC, macrophages, monocytes, NK cells, and mast cells are critically important in SA. Studies have shown that there was a significantly higher proportion of M1 in SA when compared with other interstitial lung diseases (ILDs) (42). Roy et al. illustrated that BATF2 (an activation marker gene for M1) mediates the regulation of genes in interferon (IFN)-γ-activated classical macrophages and LPS/HKTB-induced macrophage modulation (43). The polarization of M1 macrophages in the lung potentially aggravates the granuloma formation. As a result, we deduced that BATF2 could raise DC and monocytes or reduce M0 macrophages cells, and PDK4 can raise dendritic cells, NK cells, and mast cells, or reduce T cells naive to participate in SA progression. PDK4 and BATF2 might be molecules of immune microenvironment. Although the precise mechanisms have not been well elucidated, the association between the immune microenvironment and inflammatory status is pivotal in the pathogenesis of sarcoidosis. However, these hypotheses need more research to elucidate the unknown reciprocal relationship between immune cells, BATF2, and PDK4.
Conclusions
This study revealed that PDK4 and BATF4 are diagnostic markers of SA. We also found that Tregs, NK cells activated, monocytes, macrophages, DC activated, mast cells resting, and mast cells activated may contribute to the pathogenesis of SA. Besides, PDK4 and BATF4 are closely related to the immune cells, which may have an important role in SA. Further exploration of the immune cells may determine new targets of SA immunotherapy and enhance the efficacy of immunomodulatory therapies for SA patients.
Acknowledgments
Funding: None.
Footnote
Reporting Checklist: The authors have completed the STARD reporting checklist. Available at https://atm.amegroups.com/article/view/10.21037/atm-22-180/rc
Data Sharing Statement: Available at https://atm.amegroups.com/article/view/10.21037/atm-22-180/dss
Conflicts of Interest: All authors have completed the ICMJE uniform disclosure form (available at https://atm.amegroups.com/article/view/10.21037/atm-22-180/coif). The authors have no conflicts of interest to declare.
Ethical Statement: The authors are accountable for all aspects of the work in ensuring that questions related to the accuracy or integrity of any part of the work are appropriately investigated and resolved. This study was approved by the Ethics Committee of the First Affiliated Hospital of Chengdu Medical College (approval number 2021CYFYIRB-BA-14-01). All participants provided their written informed consent. All procedures performed in this study involving human participants were in accordance with the Declaration of Helsinki (as revised in 2013).
Open Access Statement: This is an Open Access article distributed in accordance with the Creative Commons Attribution-NonCommercial-NoDerivs 4.0 International License (CC BY-NC-ND 4.0), which permits the non-commercial replication and distribution of the article with the strict proviso that no changes or edits are made and the original work is properly cited (including links to both the formal publication through the relevant DOI and the license). See: https://creativecommons.org/licenses/by-nc-nd/4.0/.
References
- Judson MA. Environmental Risk Factors for Sarcoidosis. Front Immunol 2020;11:1340. [Crossref] [PubMed]
- Soto-Gomez N, Peters JI, Nambiar AM. Diagnosis and Management of Sarcoidosis. Am Fam Physician 2016;93:840-8. [PubMed]
- Criado E, Sánchez M, Ramírez J, et al. Pulmonary sarcoidosis: typical and atypical manifestations at high-resolution CT with pathologic correlation. Radiographics 2010;30:1567-86. [Crossref] [PubMed]
- Spagnolo P, Rossi G, Trisolini R, et al. Pulmonary sarcoidosis. Lancet Respir Med 2018;6:389-402. [Crossref] [PubMed]
- Carmona EM, Kalra S, Ryu JH. Pulmonary Sarcoidosis: Diagnosis and Treatment. Mayo Clin Proc 2016;91:946-54. [Crossref] [PubMed]
- Culver DA. Sarcoidosis. Immunol Allergy Clin North Am 2012;32:487-511. [Crossref] [PubMed]
- West SG. Current management of sarcoidosis I: pulmonary, cardiac, and neurologic manifestations. Curr Opin Rheumatol 2018;30:243-8. [Crossref] [PubMed]
- Baughman RP, Grutters JC. New treatment strategies for pulmonary sarcoidosis: antimetabolites, biological drugs, and other treatment approaches. Lancet Respir Med 2015;3:813-22. [Crossref] [PubMed]
- Korsten P, Strohmayer K, Baughman RP, et al. Refractory pulmonary sarcoidosis - proposal of a definition and recommendations for the diagnostic and therapeutic approach. Clin Pulm Med 2016;23:67-75. [Crossref] [PubMed]
- Lee S, Birnie D, Dwivedi G. Current perspectives on the immunopathogenesis of sarcoidosis. Respir Med 2020;173:106161. [Crossref] [PubMed]
- Talreja J, Bauerfeld C, Sendler E, et al. Derangement of Metabolic and Lysosomal Gene Profiles in Response to Dexamethasone Treatment in Sarcoidosis. Front Immunol 2020;11:779. [Crossref] [PubMed]
- Larsson J, Graff P, Bryngelsson IL, et al. Sarcoidosis and increased risk of comorbidities and mortality in sweden. Sarcoidosis Vasc Diffuse Lung Dis 2020;37:104-35. [PubMed]
- Zhou ER, Arce S. Key Players and Biomarkers of the Adaptive Immune System in the Pathogenesis of Sarcoidosis. Int J Mol Sci 2020;21:7398. [Crossref] [PubMed]
- Zhang B, Zhao F, Mao H, et al. Interleukin 33 ameliorates disturbance of regulatory T cells in pulmonary sarcoidosis. Int Immunopharmacol 2018;64:208-16. [Crossref] [PubMed]
- Liu Z, Mi M, Li X, et al. A lncRNA prognostic signature associated with immune infiltration and tumour mutation burden in breast cancer. J Cell Mol Med 2020;24:12444-56. [Crossref] [PubMed]
- Blankley S, Graham CM, Turner J, et al. The Transcriptional Signature of Active Tuberculosis Reflects Symptom Status in Extra-Pulmonary and Pulmonary Tuberculosis. PLoS One 2016;11:e0162220. [Crossref] [PubMed]
- Bloom CI, Graham CM, Berry MP, et al. Transcriptional blood signatures distinguish pulmonary tuberculosis, pulmonary sarcoidosis, pneumonias and lung cancers. PLoS One 2013;8:e70630. [Crossref] [PubMed]
- Deng YJ, Ren EH, Yuan WH, et al. GRB10 and E2F3 as Diagnostic Markers of Osteoarthritis and Their Correlation with Immune Infiltration. Diagnostics (Basel) 2020;10:171. [Crossref] [PubMed]
- Pascut D, Pratama MY, Gilardi F, et al. Weighted miRNA co-expression networks analysis identifies circulating miRNA predicting overall survival in hepatocellular carcinoma patients. Sci Rep 2020;10:18967. [Crossref] [PubMed]
- Lin T, Qiu Y, Peng W, et al. Heat Shock Protein 90 Family Isoforms as Prognostic Biomarkers and Their Correlations with Immune Infiltration in Breast Cancer. Biomed Res Int 2020;2020:2148253. [Crossref] [PubMed]
- Niewiadomska E, Kowalska M, Skrzypek M, et al. Incidence and economic burden of sarcoidosis in years 2011-2015 in Silesian voivodeship, Poland. Sarcoidosis Vasc Diffuse Lung Dis 2020;37:43-52. [PubMed]
- Vijayaraj M, Abhinand PA, Venkatesan P, et al. An ANN model for the differential diagnosis of tuberculosis and sarcoidosis. Bioinformation 2020;16:539-46. [Crossref] [PubMed]
- Węcławek M, Ziora D, Jastrzębski D. Imaging methods for pulmonary sarcoidosis. Adv Respir Med 2020;88:18-26. [Crossref] [PubMed]
- Greaves SA, Atif SM, Fontenot AP. Adaptive Immunity in Pulmonary Sarcoidosis and Chronic Beryllium Disease. Front Immunol 2020;11:474. [Crossref] [PubMed]
- Matsuyama H, Isshiki T, Chiba A, et al. Activation of mucosal-associated invariant T cells in the lungs of sarcoidosis patients. Sci Rep 2019;9:13181. [Crossref] [PubMed]
- Grunewald J, Spagnolo P, Wahlström J, et al. Immunogenetics of Disease-Causing Inflammation in Sarcoidosis. Clin Rev Allergy Immunol 2015;49:19-35. [Crossref] [PubMed]
- Chang H, Yang X, You K, et al. Integrating multiple microarray dataset analysis and machine learning methods to reveal the key genes and regulatory mechanisms underlying human intervertebral disc degeneration. PeerJ 2020;8:e10120. [Crossref] [PubMed]
- Gong Z, Gu Y, Xiong K, et al. The Evaluation and Validation of Blood-Derived Novel Biomarkers for Precise and Rapid Diagnosis of Tuberculosis in Areas With High-TB Burden. Front Microbiol 2021;12:650567. [Crossref] [PubMed]
- Leem J, Lee IK. Mechanisms of Vascular Calcification: The Pivotal Role of Pyruvate Dehydrogenase Kinase 4. Endocrinol Metab (Seoul) 2016;31:52-61. [Crossref] [PubMed]
- Jeon JH, Thoudam T, Choi EJ, et al. Loss of metabolic flexibility as a result of overexpression of pyruvate dehydrogenase kinases in muscle, liver and the immune system: Therapeutic targets in metabolic diseases. J Diabetes Investig 2021;12:21-31. [Crossref] [PubMed]
- Wu C, Zhang H, Lin X, et al. Role of PDK4 in insulin signaling pathway in periadrenal adipose tissue of pheochromocytoma patients. Endocr Relat Cancer 2020;27:583-9. [Crossref] [PubMed]
- Wu J, Zhao Y, Park YK, et al. Loss of PDK4 switches the hepatic NF-κB/TNF pathway from pro-survival to pro-apoptosis. Hepatology 2018;68:1111-24. [Crossref] [PubMed]
- Besnard V, Calender A, Bouvry D, et al. G908R NOD2 variant in a family with sarcoidosis. Respir Res 2018;19:44. [Crossref] [PubMed]
- Kayama H, Tani H, Kitada S, et al. BATF2 prevents T-cell-mediated intestinal inflammation through regulation of the IL-23/IL-17 pathway. Int Immunol 2019;31:371-83. [Crossref] [PubMed]
- Bondar G, Togashi R, Cadeiras M, et al. Association between preoperative peripheral blood mononuclear cell gene expression profiles, early postoperative organ function recovery potential and long-term survival in advanced heart failure patients undergoing mechanical circulatory support. PLoS One 2017;12:e0189420. [Crossref] [PubMed]
- Guler R, Roy S, Suzuki H, et al. Targeting Batf2 for infectious diseases and cancer. Oncotarget 2015;6:26575-82. [Crossref] [PubMed]
- Ringkowski S, Thomas PS, Herbert C. Interleukin-12 family cytokines and sarcoidosis. Front Pharmacol 2014;5:233. [Crossref] [PubMed]
- Cinetto F, Scarpa R, Dell'Edera A, et al. Immunology of sarcoidosis: old companions, new relationships. Curr Opin Pulm Med 2020;26:535-43. [Crossref] [PubMed]
- Jabbari P, Sadeghalvad M, Rezaei N. An inflammatory triangle in Sarcoidosis: PPAR-γ, immune microenvironment, and inflammation. Expert Opin Biol Ther 2021;21:1451-9. [Crossref] [PubMed]
- Tøndell A, Rø AD, Børset M, et al. Activated CD8+ T cells and natural killer T cells in bronchoalveolar lavage fluid in hypersensitivity pneumonitis and sarcoidosis. Sarcoidosis Vasc Diffuse Lung Dis 2015;31:316-24. [PubMed]
- Lepzien R, Rankin G, Pourazar J, et al. Mapping mononuclear phagocytes in blood, lungs, and lymph nodes of sarcoidosis patients. J Leukoc Biol 2019;105:797-807. [Crossref] [PubMed]
- Wojtan P, Mierzejewski M, Osińska I, et al. Macrophage polarization in interstitial lung diseases. Cent Eur J Immunol 2016;41:159-64. [Crossref] [PubMed]
- Roy S, Guler R, Parihar SP, et al. Batf2/Irf1 induces inflammatory responses in classically activated macrophages, lipopolysaccharides, and mycobacterial infection. J Immunol 2015;194:6035-44. [Crossref] [PubMed]