Identification of a seven-gene prognostic signature using the gene expression profile of osteosarcoma
Introduction
Osteosarcoma often occurs in children and adolescents with an average age of about 16 years (1-3) and is a leading cause of cancer-related deaths among adolescents (2). Osteosarcoma is often diagnosed at the late stage of the disease, which further contributes to poor prognosis (1-3). If diagnosed at an early stage, about 68% of osteosarcoma patients will have primary and localized tumor lesions with a high 5-year survival rate (70%) (4,5). However, the 5-year survival rate of patients with metastatic osteosarcoma is approximately 19–30% (4-6). Therefore, the development of early diagnostic methods and novel therapeutic strategies will facilitate the effective control of the invasion and metastasis of osteosarcomas.
Increasingly, studies have shown that messenger RNAs (mRNAs), as a molecular biomarker, has prognostic value in patients with potentially high-risk osteosarcoma (7,8). For example, positive expression of CD133 is related to local recurrence, low cancer stage, metastasis, and a high 5-year overall survival in osteosarcoma patients (9). The expression of ferritin light chain (FTL) and inosine 5'-monophosphate dehydrogenase type II (IMPDH2) can also be used to classify osteosarcoma patients into low-risk or high-risk groups, and may be potential therapeutic targets (7). In recent years, based on the development of bioinformatics techniques, multiple gene profiles serve as important prognostic indicators for osteosarcoma. For example, Liu et al. demonstrated that 7 hub primary-term DEGs (CDK1, CDK20, CCNB1, MTIF2, MRPS7, VEGFA and EGF) were eventually selected as potential biomarker for osteosarcoma (10). Yang et al. identified three glycolysis-related genes (P4HA1, ABCB6, and STC2) for the establishment of a risk signature for osteosarcoma (11). Although bioinformatics analysis is a feasible method to identify specific genes in osteosarcoma, the current research has not been effectively applied to the clinic. While, in this work, 7 genes identified, are associated with osteosarcoma, they have not been reported as prognostic markers for osteosarcoma. Compared with using a single gene marker, the combination of multiple genes maybe more effectively and comprehensively improve the identification of differences in the prognosis of patients with osteosarcomas.
Since a wide variety of signaling pathways and genes are involved in osteosarcoma, this current study developed a gene model based on multiple differentially expressed mRNAs to improve the prediction of recurrence in osteosarcoma patients.
The public databases Gene Expression Omnibus (GEO) (12) and The Cancer Genome Atlas (TCGA) (13) provide comprehensive sequence- and array-based data, allowing quick and easy access to a large amount of data using bioinformatics methods. This study screened the important differentially expressed genes (DEGs) in osteosarcoma samples and normal tissues or para-cancer samples from the GEO and TARGET dataset, respectively. The minimum absolute contraction and selection operator (LASSO) regression was used to develop a mRNAs-based signature to evaluate the prognosis of patients with osteosarcoma. Related pathways and markers were detected with Gene Ontology (GO) enrichment analysis and Kyoto Encyclopedia of Genes and Genomes (KEGG) pathway analysis. These findings provide an effective method to predict the recurrence rate of osteosarcoma and to facilitate personalized treatment for patients with osteosarcoma.
We present the following article in accordance with the TRIPOD reporting checklist (available at https://atm.amegroups.com/article/view/10.21037/atm-21-6276/rc).
Methods
Acquisition of data
The RNA-seq data of 101 osteosarcoma tissue samples and the clinical information of 274 osteosarcoma patients were obtained from the TARGET dataset (https://ocg.cancer.gov/). The RNA-seq data was normalized using the transcripts per million (TPM) method that scales gene length and sequencing depth (M). Since the TARGET database did not provide normal healthy samples for the screening of differential genes, three datasets from the GEO (14) database [GSE39058 (15), GSE21257 (16), and GSE42352] were used to screen the DEGs (17,18). The GSE39058 dataset includes two sub-series, the GSE39055 and GSE39057 datasets. The Illumina HumanHT-12 WG-DASL V4.0 R2 expression beadchip was used as the expression profiling platform. The expression profiling contained 29,377 probes and 47 samples, including 37 osteosarcoma biopsy samples and 5 pairs of biopsy and surgical resection samples, and the clinical and survival data of the patients. The GSE21257 expression profiling (in the Illumina human-6 v2.0 expression beadchip platform) contained 48,701 probes, 34 samples from patients with osteosarcoma metastasis within 5 years, and 19 samples from patients without metastasis within 5 years. The GSE42352 expression profiling platform used was the Illumina human-6 v2.0 expression beadchip. The expression profiling contained 24,998 probes and 118 samples, including 15 normal tissue samples and 103 cancer tissue samples. The data analysis process is shown in Figure 1. The study was conducted in accordance with the Declaration of Helsinki (as revised in 2013).
Screening of the DEGs
The limma package in the R software (19) was applied to detect the differential gene expression. According to the threshold value of P<0.05, a gene with a |log2(fold change)| >0.263 was defined as a differentially expressed gene of osteosarcoma, and was depicted using volcano maps. The common differences obtained from the three datasets were extracted as candidate genes for further analysis.
Construction of the protein interaction networks and extraction of subnets
Human protein interaction information was obtained by integrating five protein interaction databases, including the Biomolecular Interaction Network Database (BIND), the Database of Interacting Proteins (DIP), the Human Protein Reference Database (HPRD), IntAc, and the Molecular INTeraction Database (MINT) for developing a protein-protein interaction (PPI) network. The DEGs were merged into the PPI as seed genes, and the nodes adjacent to them were obtained with the differentially expressed gene to construct subnets, thereby revealing the potential tumor-related genes. The network topological properties were investigated.
Functional analysis of the genes
The functional interpretation of genes listed in g:Profiler (20) were used to conduct enrichment analyses of GO, KEGG pathways, and human protein figure profiles of candidate genes related to osteosarcoma. EnrichmentMap (21), a cytoscape plug-in, was used to visualize the results of the gene enrichment analysis in network form.
Risk model construction
Univariate Cox regression analysis was used to identify genes showing significant correlation with the prognosis of osteosarcoma (P<0.05) in 80% of the TARGET dataset (Figure S1A). Those genes were then filtered by dimension reduction using LASSO regression analysis, which is a compression estimation that refines a model through a penalty function to compress dataset coefficients close to zero. In this way, it retains subset contraction, and is considered a biased estimation method in data processing with complex collinearity, thereby realizing variable selection under parameter estimation and reducing multicollinearity problem during regression analysis. Multivariate Cox regression analysis (P<0.05) was conducted and Cox proportional risk regression models were developed using the “coxph” function in the R package “survival”. LASSO analysis was conducted using cv.glmnet in the R package “glmnet”. The risk model is as follows:
where N is the sum of the prognostic genes, Expk refers to the prognostic gene expression level, and HR is the estimated regression coefficient of the genes in the multivariate Cox regression analysis.
Survival analysis
The osteosarcoma samples were grouped according to the z-score into a high-risk and a low-risk group. Kaplan-Meier (KM) curves and time-dependent receiver operating characteristic (ROC) curves were used to showed patient survival status. These analyses were all performed with the “survival” R package.
External data validation datasets
To verify the reliability of the prognostic risk markers identified in this study, the GEO dataset GSE16091 was used as the external validation set, and internal validation was performed using the GSE39058 and GSE21257 datasets. In addition, the GSE19276, GSE42352, and GSE36001 datasets were used to verify the differential expression of the genes.
Statistical analysis
The R software (version: 3.5.2) was used for performing all statistical analyses and graphical representations. The Kaplan-Meier survival plots showed the survival rates of patients. The effectiveness of the model for predicting survival was assessed using the AUC of the ROC curve. Independent prognostic factors were identified by univariate and multivariate Cox regression analyses. Unless stated otherwise, statistical significance was considered at P<0.05.
Results
Screening of the prognostic genes for osteosarcoma
The working flow chart is shown in Figure 1. A total of 758 DEGs were identified from the GSE39058 dataset, including 158 upregulated genes and 600 downregulated genes. In the GSE42352 dataset, a total of 4,821 differential genes were identified, including 2,455 upregulated and 2,366 downregulated genes. In the GSE21257 dataset, there were 552 DEGs, of which, 221 were upregulated and 331 were downregulated (Figure 2A). The DEGs that were identified in at least 2 datasets were retained, resulting in a total of 242 differentially regulated genes, including 94 upregulated genes (Figure 2B) and 148 downregulated genes (Figure 2C).
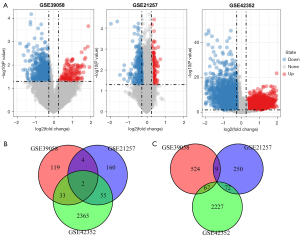
Construction of the protein-protein interaction network and identification of the subnets
Due to the lack of normal control samples in this study, the human protein interaction information from 5 databases were integrated to construct a background network, consisting of 80,977 pairs of interactions and 13,368 genes. The 242 previously obtained DEGs were merged into the network as seed genes and a total of 185 nodes were mapped. These nodes and their neighboring nodes were extracted to construct a subnet consisting of 1,240 nodes. The node degree conformed to the power law distribution in the network (Figure 3A) and satisfied the characteristics of the molecular biology network. The large nodes in the network were hub nodes, generally considered as more important key nodes in the network. The node with the highest degree in this sub-network was the retinoblastoma 1 gene (RB1 gene; degree 150). Previous studies have demonstrated that the RB1 gene mutation is closely related to the development and formation of human osteosarcoma, and that the loss of RB1 function resulted in a 1.62-fold increase in the mortality of osteosarcoma patients (22). The second largest node is the growth factor receptor-bound protein 2 (GRB2; degree 117), which has been shown to be positively expressed in bone cancer, rhabdomyosarcoma, and synovial sarcoma (23). The third largest gene node is the E1A binding protein P300 (EP300; degree112), which has been associated with esophageal cancer (24), breast cancer (25), and lymphoma (26). These results confirmed that identifying osteosarcoma-related genes through the network method is an effective approach. Herein, nodes with a moderate degree greater than 15 (380 genes) and the above 242 DEGs (242 genes) were selected as candidate genes for further analysis. In total, 590 unique candidate genes were selected (Figure 3B).
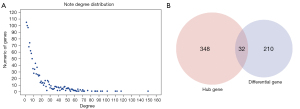
Functional analysis of tumor-related genes
Functional enrichment analysis of the 590 candidate genes was performed. A total of 2,499 pathways, including 1,218 GO biological pathways, 420 transcription factors, and 133 KEGG pathways, were enriched (Figure 4A), suggesting that these genes were involved in a large number of biological pathways and molecular functions. Crosstalk analysis on these pathways revealed that the main core regulatory pathways included non-coding (nc)RNA processing, metabolic processes, bone marrow, and hematopoietic cells (Figure 4B). These genes were also enriched in bone and bone cell development, abnormal differentiation of osteoclasts and osteoporosis, as well as bone marrow which is involved in cell metabolism and bone tissue differentiation (Figure 4C). These results demonstrated that these candidate genes are directly or indirectly involved in bone tissue growth, differentiation, and pathological changes, and that their abnormal expression may result in lesions of bone tissues.
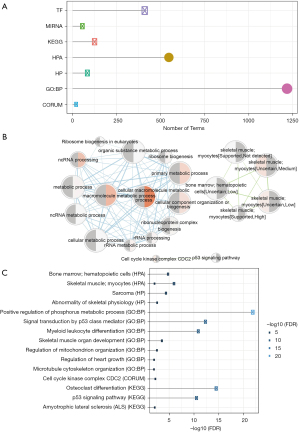
Development of a seven-gene signature and prognosis analysis
Here, 80% of the TARGET data were randomly included into the training dataset, and the DEGs and hub genes identified were analyzed with the R package survival coxph function. A total of 43 genes showed significant differences in prognosis.
LASSO Cox regression analysis was performed on the 43 genes identified to be related to overall survival (OS) in osteosarcoma patients. Analysis of the change trajectory of each independent variable revealed that the gradual increase of lambda was positively related to a gradual increase in the number of independent variable coefficients approaching 0 (Figure 5A). The model was constructed by using a 3-fold cross-validation, and the confidence interval under each lambda was analyzed. When lambda =0.09518779, the model was optimized (Figure 5B) and 12 genes were determined to be target genes.
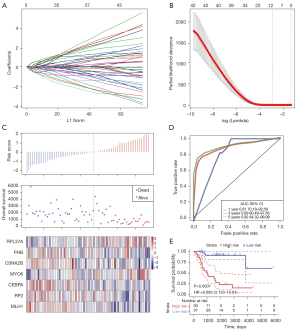
Furthermore, multivariate Cox survival analysis on the 12 genes identified 7 genes with the minimum Akaike information criterion (AIC) value of 146.28, and these genes were used in the final model (Table 1).
Table 1
Gene | Coefficient | P | HR | Lower 0.95 | Upper 0.95 |
---|---|---|---|---|---|
RP2 | −0.8445 | 0.0025 | 0.4298 | 0.2487 | 0.7426 |
PHB | −0.7205 | 0.0523 | 0.4865 | 0.2350 | 1.0071 |
MYO6 | 0.8332 | 0.0005 | 2.3006 | 1.4360 | 3.6859 |
MLH1 | −0.9115 | 0.0713 | 0.4019 | 0.1493 | 1.0823 |
CSNK2B | −0.6136 | 0.0990 | 0.5414 | 0.2612 | 1.1224 |
RPL37A | 0.6677 | 0.0127 | 1.9498 | 1.1535 | 3.2959 |
CEBPA | −0.5183 | 0.0797 | 0.5955 | 0.3335 | 1.0633 |
MYO6, myosin VI; RPL37A, ribosomal protein L37A; RP2, retinitis pigmentosa 2; PHB, polyhydroxybutyrate; MLH1, mutL homolog 1; CSNK2B, Casein kinase 2 beta; CEPBA, CCAAT/enhancer binding protein alpha.
The formula is as follows: RiskScore7 = −0.8445 × RP2 − 0.7205 × PHB + 0.8332 × MYO6 − 0.9115 × MLH1 − 0.6136 × CSNK2B + 0.6677 × RPL37A − 0.5183 × CEBPA.
The GSE19276 dataset containing normal healthy samples was used to evaluate the expressions of the 7 key genes (Figure S1B). Significant differences in the expression of 5 genes were observed and this was consistent with the trend of the study. Significant differential expression of the 7 genes was also observed in the GSE42352 dataset that contains high-grade osteosarcoma cell lines and mesenchymal stem cells (Figure S1C). Further comparison of expression differences between osteosarcoma cell lines and normal osteoblast cell lines in the GSE36001 dataset revealed significant differential expression of the 7 genes (Figure S1D). In addition, reverse transcription polymerase chain reaction (RT-PCR) analysis on osteosarcoma cell lines and osteoblast cell lines showed significant differential expression of the 7 genes as expected (Figure S1E). These results verified the reliability of the 7 key genes.
The TARGET training dataset samples were divided into a high-group and a low-risk group according to the Z-score. An increase in the patient’s risk score was negatively associated with the survival time, and most deaths were observed in the high-risk group. The expression of both myosin VI (MYO6) and ribosomal protein L37A (RPL37A) was upregulated with an increase in the risk value, indicating that high expression of these 2 genes was associated with higher risk, and indeed, high expression of MYO6 and RPL37A may be significant risk factors. The expression of retinitis pigmentosa 2 (RP2), polyhydroxybutyrate (PHB), mutL homolog 1 (MLH1), Casein kinase 2 beta (CSNK2B), and CCAAT/enhancer binding protein alpha (CEPBA) were all downregulated with an increase in risk value, indicating that lower risk was related to high expression of these 5 genes, and indeed, these genes may be considered protective factors (Figure 5C). The ROC curve analysis demonstrated that the AUC (area under the ROC curve) of the 1-, 3-, and 5-year survival status were all above 0.81 (Figure 5D). In the TARGET training dataset sample, 37 patients were classified into the low-risk group and another 30 patients were classified into the high-risk group. The Kaplan-Meier survival curve prognostic analysis demonstrated that the survival time of patients in the high-risk group was shorter than that in low-risk group (Figure 5E). Multivariate survival analysis was applied to evaluate the influence of clinical factors on the model. Age, gender, and therapy were included for comparison with risk score, and univariate analysis showed that only risk score had significant prognostic value (Figure S2A). Multivariate analysis also demonstrated that only the risk score had significant prognostic value (Figure S2B), indicating that the risk score was a prognostic factor independent of other clinical features.
The robustness of seven-gene signature
To determine the robustness of the signature, the model and coefficient used for the training dataset was verified using the GSE39058 and GSE21257 datasets. Similarly, the changes in the expression of the 7 genes were associated with increased risk. Furthermore, higher expression of MYO6 and RPL37A was associated with an increase in the risk value, indicating that high expression of MYO6 and RPL37A was positively correlated with a higher risk and therefore, these genes are risk factors. The expression of RP2, PHB, MLH1, CSNK2B, and CEPBA were all downregulated with an increase in risk, indicating that the high expression of these 5 genes was associated with a lower risk, and are therefore protective factors (Figure 6A). The ROC analysis of the prognostic classification of the risk score showed that the model had high 5 years AUC values of 0.72 for the GSE39058 dataset (Figure 6B). In the GSE39058 dataset, Kaplan-Meier survival curve showed that the prognosis of the high-risk group tended to be poorer than that of the low-risk group, however, the difference was not statistically different (P=0.057, Figure 6C). In GSE21257 dataset, the above genes expressions had similar trend with those in GSE39058 dataset (Figure 6D). ROC analysis indicated that the 5 years AUC was 0.78 in GSE21257 dataset (Figure 6E). In contrast, in GSE21257 dataset, patients in the high-risk group had significantly poorer survival than patients in the low-risk group (P=0.013, Figure 6F).
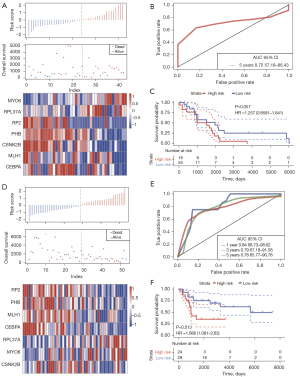
The expression profiles of the 7 genes were extracted from the GSE16091 dataset. The risk scores of each patient were calculated using the same method to classify patients into a high-risk group and a low-risk group. Kaplan-Meier analysis showed a significantly poorer prognostic outcome in the high-risk group compared to the low-risk group (Figure S3A), and the ROC analysis showed a 1-year AUC of 0.88, a 3-year AUC of 0.89, and a 5-year AUC of 0.83 (Figure S3B).
Discussion
After chemotherapy, the 5-year survival rate of patients with osteosarcoma can be improved from 10–20% to 60–80%, but the prognosis of patients with osteosarcoma is still relatively poor, and the prognosis of osteosarcoma is related to a variety of factors, such as age, tumor size, location, treatment time, response to chemotherapy, lung metastasis, height at diagnosis and birth-weight (27-29). This study identified 242 differentially expression genes through screening the gene expression profile of osteosarcomas in the training dataset. There was a close relationship between these genes and the differential response of the osteosarcoma to treatment, as well as the development, progression, disease subtypes, and prognosis of the cancer. Construction of the human PPI network identified 380 central node genes that were associated with osteosarcoma. These genes, along with 242 differentially expression genes, were considered candidate genes. These candidate genes were involved in biological processes such as osteoclast differentiation and bone physiology abnormalities. The study further successfully developed a signature consisting of 7 genes, namely, RP2, PHB, MYO6, MLH1, CSNK2B, RPL37A, and CEBPA, for predicting the prognosis of patients with osteosarcoma. External datasets were applied to verify the reliability of signature. The signature effectively divided the patients into a high-risk group and a low-risk group, which showed significantly different survival times.
Previous studies have applied bioinformatics analyses to identify the DEGs, potential target genes and transcription factors, and gene functions in osteosarcoma. Understanding the related underlying biological processes in the progression of osteosarcoma can facilitate better clinical decisions for the patients. Liu et al. built a 2-gene signature, involving PML and EPB41, according to clinical treatment outcomes (30). Although bio-reliability analyses and verification were performed, the AUC in the training cohort for the 5-year survival was only 0.72. Another study designed a risk signature with three genes (MYC, LY86, and CPE) based on metastasis-associated genes for osteosarcoma (31). Despite validation across multiple datasets, the AUC remained low, with an AUC of 0.82 for the 5-year survival in the target dataset. Zhang et al. identified 8 clinically significant genes and constructed an 8-gene signature for osteosarcoma with an overall AUC of 0.88 (32). All these reports indicate that bioinformatics-based methods are effective in identifying prognostic markers of osteosarcoma. However, none of these models are currently used in clinical practice. Therefore, further biomarker studies are required for clinical selection and validation.
This study constructed a 7-gene signature based on the prognosis of osteosarcoma patients to estimate overall prognosis. Gene signatures can be used to predict the risk and prognosis of osteosarcoma. Compared with the published gene signature, the 5-year AUC of 0.92 was higher in training dataset. In addition, the 7-gene signature demonstrated a strong predictive performance in multiple verification sets. These 7 genes are abnormally expressed in the process of tumorigenesis and play an important role in the development of osteosarcoma. This information provides a basis for further research into understanding the molecular mechanisms of osteosarcomas and the development of novel treatment strategies.
Among the signature genes, some are involved in the genesis and development of disease. RP-2 is a gene that is activated in thymic cells undergoing apoptosis (33). Moreover, Northern blot analyses have demonstrated that this protein is mainly expressed in skeletal muscles (34). A protein similar to RP-2, namely, P2XM, may have an important role in the differentiation and/or proliferation of skeletal muscle cells, and altered expression of P2XM may contribute to the development of certain sarcomas (34). PHB is an adriamycin resistance-associated gene that can suppress the proliferation of human osteosarcoma MG-63 cells through the interaction with oncogenes or tumor suppressor genes, c-fos, p53, c-myc, and Rb (35). MYO6, which is highly expressed in cancers such as colorectal cancer, non-small cell lung cancer, stomach cancer, and prostate cancer, is regarded as an oncogene (36-38). In endometrial cancer, MLH1 gene methylation can cause complete mismatch repair or MLH1 defects (39). CSNK2B enhances nuclear factor (NF)-κB reporter activity in hepatocellular carcinoma cells in a dose-dependent manner (40). Bioinformatics studies have shown that RPL37A can serve as a gene signature by acting as a molecular marker of tumors (41,42). Interleukin (IL)-34 contributes to the proliferation and migration of hepatoma cells via CEBPA (43). Although some genes have not been reported in relation to the progression of osteosarcoma, the above studies support the biological relevance of gene signatures in tumor biology.
While these 7 genes are associated with osteosarcoma, they have not been reported as prognostic markers for osteosarcoma. Compared with using a single gene marker, the combination of multiple genes can more effectively and comprehensively improve the identification of differences in the prognosis of patients with osteosarcomas.
Some limitations in the current research should be noted. While multiple GEO datasets were included for analysis, the results should be confirmed in other independent cohorts. Also, the prognostic value of mRNAs was evaluated using gene chips, and the results should be verified with biological experiments such as real-time quantitative polymerase chain reaction (RT-qPCR). Moreover, the specific clinical significance, biological function, and potential mechanisms of action of each identified mRNAs in our model should be examined. Further experiments are warranted to determine the functions of these prognostic mRNAs in osteosarcoma.
Conclusions
This study determined the prognostic markers and constructed a seven-gene signature for evaluating the OS of patients with osteosarcoma using bioinformatics methods. The current findings enrich the current understanding of the role of mRNAs in the pathogenesis, progression, and prognosis of osteosarcomas. This information may contribute to the development of novel therapeutic and diagnostic biomarkers for clinical practice.
Acknowledgments
Funding: This work was supported by the Guangxi Natural Science Foundation under Grant No. 2016GXNSFBA380202.
Footnote
Reporting Checklist: The authors have completed the TRIPOD reporting checklist. Available at https://atm.amegroups.com/article/view/10.21037/atm-21-6276/rc
Conflicts of Interest: All authors have completed the ICMJE uniform disclosure form (available at https://atm.amegroups.com/article/view/10.21037/atm-21-6276/ciof). The authors have no conflicts of interest to declare.
Ethical Statement: The authors are accountable for all aspects of the work in ensuring that questions related to the accuracy or integrity of any part of the work are appropriately investigated and resolved. The study was conducted in accordance with the Declaration of Helsinki (as revised in 2013).
Open Access Statement: This is an Open Access article distributed in accordance with the Creative Commons Attribution-NonCommercial-NoDerivs 4.0 International License (CC BY-NC-ND 4.0), which permits the non-commercial replication and distribution of the article with the strict proviso that no changes or edits are made and the original work is properly cited (including links to both the formal publication through the relevant DOI and the license). See: https://creativecommons.org/licenses/by-nc-nd/4.0/.
References
- Sakamoto A, Iwamoto Y. Current status and perspectives regarding the treatment of osteo-sarcoma: chemotherapy. Rev Recent Clin Trials 2008;3:228-31. [Crossref] [PubMed]
- Brown HK, Tellez-Gabriel M, Heymann D. Cancer stem cells in osteosarcoma. Cancer Lett 2017;386:189-95. [Crossref] [PubMed]
- Kager L, Zoubek A, Potschger U, et al. Primary metastatic osteosarcoma: presentation and outcome of patients treated on neoadjuvant Cooperative Osteosarcoma Study Group protocols. J Clin Oncol 2003;21:2011-8. [Crossref] [PubMed]
- Huang J, Ni J, Liu K, et al. HMGB1 promotes drug resistance in osteosarcoma. Cancer Res 2012;72:230-8. [Crossref] [PubMed]
- Mialou V, Philip T, Kalifa C, et al. Metastatic osteosarcoma at diagnosis: prognostic factors and long-term outcome--the French pediatric experience. Cancer 2005;104:1100-9. [Crossref] [PubMed]
- Thayanithy V, Sarver AL, Kartha RV, et al. Perturbation of 14q32 miRNAs-cMYC gene network in osteosarcoma. Bone 2012;50:171-81. [Crossref] [PubMed]
- Fellenberg J, Bernd L, Delling G, et al. Prognostic significance of drug-regulated genes in high-grade osteosarcoma. Mod Pathol 2007;20:1085-94. [Crossref] [PubMed]
- Gu Z, Wu S, Xu G, et al. miR-487a performs oncogenic functions in osteosarcoma by targeting BTG2 mRNA. Acta Biochim Biophys Sin (Shanghai) 2020;52:631-7. [Crossref] [PubMed]
- Xu N, Kang Y, Wang W, et al. The prognostic role of CD133 expression in patients with osteosarcoma. Clin Exp Med 2020;20:261-7. [Crossref] [PubMed]
- Liu J, Wu S, Xie X, et al. Identification of potential crucial genes and key pathways in osteosarcoma. Hereditas 2020;157:29. [Crossref] [PubMed]
- Yang M, Ma X, Wang Z, et al. Identification of a novel glycolysis-related gene signature for predicting the prognosis of osteosarcoma patients. Aging (Albany NY) 2021;13:12896-918. [Crossref] [PubMed]
- Edgar R, Domrachev M, Lash AE. Gene Expression Omnibus: NCBI gene expression and hybridization array data repository. Nucleic Acids Res 2002;30:207-10. [Crossref] [PubMed]
- Deng M, Bragelmann J, Schultze JL, et al. Web-TCGA: an online platform for integrated analysis of molecular cancer data sets. BMC Bioinformatics 2016;17:72. [Crossref] [PubMed]
- Barrett T, Wilhite SE, Ledoux P, et al. NCBI GEO: archive for functional genomics data sets--update. Nucleic Acids Res 2013;41:D991-5. [Crossref] [PubMed]
- Kelly AD, Haibe-Kains B, Janeway KA, et al. MicroRNA paraffin-based studies in osteosarcoma reveal reproducible independent prognostic profiles at 14q32. Genome Med 2013;5:2. [Crossref] [PubMed]
- Buddingh EP, Kuijjer ML, Duim RA, et al. Tumor-infiltrating macrophages are associated with metastasis suppression in high-grade osteosarcoma: a rationale for treatment with macrophage activating agents. Clin Cancer Res 2011;17:2110-9. [Crossref] [PubMed]
- Kuijjer ML, Peterse EF, van den Akker BE, et al. IR/IGF1R signaling as potential target for treatment of high-grade osteosarcoma. BMC Cancer 2013;13:245. [Crossref] [PubMed]
- Kuijjer ML, van den Akker BE, Hilhorst R, et al. Kinome and mRNA expression profiling of high-grade osteosarcoma cell lines implies Akt signaling as possible target for therapy. BMC Med Genomics 2014;7:4. [Crossref] [PubMed]
- Ritchie ME, Phipson B, Wu D, et al. limma powers differential expression analyses for RNA-sequencing and microarray studies. Nucleic Acids Res 2015;43:e47. [Crossref] [PubMed]
- Reimand J, Kull M, Peterson H, et al. g:Profiler--a web-based toolset for functional profiling of gene lists from large-scale experiments. Nucleic Acids Res 2007;35:W193-200. [Crossref] [PubMed]
- Merico D, Isserlin R, Stueker O, et al. Enrichment map: a network-based method for gene-set enrichment visualization and interpretation. PLoS One 2010;5:e13984. [Crossref] [PubMed]
- Ren W, Gu G. Prognostic implications of RB1 tumour suppressor gene alterations in the clinical outcome of human osteosarcoma: a meta-analysis. Eur J Cancer Care (Engl) 2017; [Crossref] [PubMed]
- Al-Husseinawi E, Bui MM, Ahmed AA. Grb2-associated binding protein-1 as a biomarker in bone and soft tissue sarcomas. Pathology 2019;51:610-4. [Crossref] [PubMed]
- Bi Y, Kong P, Zhang L, et al. EP300 as an oncogene correlates with poor prognosis in esophageal squamous carcinoma. J Cancer 2019;10:5413-26. [Crossref] [PubMed]
- Sobczak M, Pitt AR, Spickett CM, et al. PARP1 Co-Regulates EP300-BRG1-Dependent Transcription of Genes Involved in Breast Cancer Cell Proliferation and DNA Repair. Cancers (Basel) 2019;11:1539. [Crossref] [PubMed]
- Mottok A, Hung SS, Chavez EA, et al. Integrative genomic analysis identifies key pathogenic mechanisms in primary mediastinal large B-cell lymphoma. Blood 2019;134:802-13. [Crossref] [PubMed]
- Sadykova LR, Ntekim AI, Muyangwa-Semenova M, et al. Epidemiology and Risk Factors of Osteosarcoma. Cancer Invest 2020;38:259-69. [Crossref] [PubMed]
- Zhang C, Guo X, Xu Y, et al. Lung metastases at the initial diagnosis of high-grade osteosarcoma: prevalence, risk factors and prognostic factors. A large population-based cohort study. Sao Paulo Med J 2019;137:423-9. [Crossref] [PubMed]
- Mirabello L, Pfeiffer R, Murphy G, et al. Height at diagnosis and birth-weight as risk factors for osteosarcoma. Cancer Causes Control 2011;22:899-908. [Crossref] [PubMed]
- Liu S, Liu J, Yu X, et al. Identification of a Two-Gene (PML-EPB41) Signature With Independent Prognostic Value in Osteosarcoma. Front Oncol 2020;9:1578. [Crossref] [PubMed]
- Shi Y, He R, Zhuang Z, et al. A risk signature-based on metastasis-associated genes to predict survival of patients with osteosarcoma. J Cell Biochem 2020;121:3479-90. [Crossref] [PubMed]
- Zhang H, Guo L, Zhang Z, et al. Co-Expression Network Analysis Identified Gene Signatures in Osteosarcoma as a Predictive Tool for Lung Metastasis and Survival. J Cancer 2019;10:3706-16. [Crossref] [PubMed]
- Woloschak GE, Chang-Liu CM, Chung J, et al. Expression of enhanced spontaneous and gamma-ray-induced apoptosis by lymphocytes of the wasted mouse. Int J Radiat Biol 1996;69:47-55. [Crossref] [PubMed]
- Urano T, Nishimori H, Han H, et al. Cloning of P2XM, a novel human P2X receptor gene regulated by p53. Cancer Res 1997;57:3281-7. [PubMed]
- Du MD, He KY, Qin G, et al. Adriamycin resistance-associated prohibitin gene inhibits proliferation of human osteosarcoma MG63 cells by interacting with oncogenes and tumor suppressor genes. Oncol Lett 2016;12:1994-2000. [Crossref] [PubMed]
- Yang Q. MicroRNA-5195-3p plays a suppressive role in cell proliferation, migration and invasion by targeting MYO6 in human non-small cell lung cancer. Biosci Biotechnol Biochem 2019;83:212-20. [Crossref] [PubMed]
- Wei AW, Li LF. Long non-coding RNA SOX21-AS1 sponges miR-145 to promote the tumorigenesis of colorectal cancer by targeting MYO6. Biomed Pharmacother 2017;96:953-9. [Crossref] [PubMed]
- Lei C, Du F, Sun L, et al. miR-143 and miR-145 inhibit gastric cancer cell migration and metastasis by suppressing MYO6. Cell Death Dis 2017;8:e3101. [Crossref] [PubMed]
- Tandon N, Hudgens C, Fellman B, et al. Variable Expression of MSH6 in Endometrial Carcinomas With Intact Mismatch Repair and With MLH1 Loss Due to MLH1 Methylation. Int J Gynecol Pathol 2020;39:507-13. [Crossref] [PubMed]
- Xiao Y, Huang S, Qiu F, et al. Tumor necrosis factor alpha-induced protein 1 as a novel tumor suppressor through selective downregulation of CSNK2B blocks nuclear factor-kappaB activation in hepatocellular carcinoma. EBioMedicine 2020;51:102603. [Crossref] [PubMed]
- Barros Filho MC, Katayama ML, Brentani H, et al. Gene trio signatures as molecular markers to predict response to doxorubicin cyclophosphamide neoadjuvant chemotherapy in breast cancer patients. Braz J Med Biol Res 2010;43:1225-31. [Crossref] [PubMed]
- El Hadi H, Abdellaoui-Maane I, Kottwitz D, et al. Development and evaluation of a novel RT-qPCR based test for the quantification of HER2 gene expression in breast cancer. Gene 2017;605:114-22. [Crossref] [PubMed]
- Kong F, Zhou K, Zhu T, et al. Interleukin-34 mediated by hepatitis B virus X protein via CCAAT/enhancer-binding protein alpha contributes to the proliferation and migration of hepatoma cells. Cell Prolif 2019;52:e12703. [Crossref] [PubMed]
(English Language Editor: J. Teoh)