Development and validation of a prognostic nomogram for 2018 FIGO stages IB1, IB2, and IIA1 cervical cancer: a large multicenter study
Introduction
In 2018, the International Union of Gynecology and Obstetrics (FIGO 2018) published a revised cervical cancer staging system. For the first time, imaging and pathology were used to assess staging. One of the updates is the classification of stage IB disease into three substages based on the two cutoff values of 2 and 4 cm in tumor diameter: stage IB1 (≤2 cm), stage IB2 (>2 to ≤4 cm), and IB3 (>4 cm). Another update was to classify patients with lymph node metastases as stage IIIC (1). However, the predictive efficacy of FIGO 2018 staging for overall oncological outcomes has yet to be supported by clinical evidence from multiple centers and large samples. Current staging systems fail to identify which patients are at the highest risk for death or recurrence. Therefore, it is essential to locate a method to accurately predict the prognosis of cervical cancer patients based on FIGO 2018 staging.
Nomograms are predictive models that provide a score or a probability that a patient will be disease-free or alive within a specific timeframe (2). Recently, Nomograms have been widely used in predicting the individual prognosis of patients with malignancies (3-7). In Yang et al., locally advanced cervical cancer patients with the 2018 FIGO stage recommended to undergo radical chemoradiotherapy in the guidelines were included, and the survival was effectively predicted (8). However, there is no nomogram to predict the prognosis of cervical cancer patients with surgical treatment as standard treatment based on FIGO 2018 staging. The guidelines recommend surgery for patients with stages IB1, IB2, and IIA1 (9).
The present study aimed to develop and validate a nomogram to predict 5-year overall survival (OS) and 5-year disease-free survival (DFS) in cervical cancer patients with FIGO 2018 stages IB1, IB2, and IIA1 disease. In addition, we established a risk stratification system to effectively identify high-risk patients and assist doctors in personalized treatment and follow-up of patients.
We present the following article in accordance with the STROBE reporting checklist (available at https://atm.amegroups.com/article/view/10.21037/atm-21-6367/rc).
Methods
Data sources
The present study cases were selected from the Chinese Cervical cancer Clinical Diagnosis and Treatment Database, which included a total of 63,926 cases of cervical cancer diagnosed from 47 hospitals (10-13). All procedures performed in this study involving human participants were in accordance with the Declaration of Helsinki (as revised in 2013). The Ethics Committee of Nanfang Hospital affiliated with Southern Medical University (Guangzhou, China) approved the study (ethics No. NFEC-2017-135). Individual consent for this retrospective analysis was waived. The study protocol was registered in the International Clinical Trials Registry Platform Search Portal (Identifier: CHiCTR1800017778).
Inclusion and exclusion criteria
The inclusion criteria were as follows: (I) 18 years or older; (II) FIGO stages IB1, IB2, and IIA1(FIGO 2018); (III) histological type of squamous cell carcinoma or adenocarcinoma; (IV) surgical approach was abdominal; (V) upfront the Querleu-Morrow classification (Q-M) type B or type C radical hysterectomy + pelvic lymphadenectomy ± para-aortic lymphadenectomy; (VI) intact postoperative pathological outcomes; and (VII) >5 years’ follow up.
Patients were excluded if they had the following special conditions: (I) cervical cancer during pregnancy; (II) cervical stump cancer; (III) cervical cancer concomitant with other malignant tumors; and (IV) use of neoadjuvant therapy.
Statistical analysis
Grouping
Enrolled patients were divided by surgical hospitals at a 3:1 ratio; the groups included 3,074 patients from 7 hospitals as the development cohort and 991 patients from 13 hospitals as the validation cohort.
Independent prognostic factors for OS/DFS in the development cohort
Candidate prognostic variables included clinical and pathological findings or potential prognostic factors for cervical cancer based on prior studies, such as age, FIGO stage, histological type, stromal invasion, surgical margins, and lymphovascular space invasion (LVSI) in the surgical specimen. The Cox proportional hazards regression model was used in the univariable and multivariable analyses to identify independent predictors of OS/DFS in enrolled patients that could be incorporated into nomograms.
Observation indicators
The patients included in this study were followed up through telephone follow-up and the reporting system of the hospital. The last follow-up was on December 31, 2018. 5-year OS/DFS were the primary endpoints of this study. OS was defined as the time from diagnosis to death from any cause or the last follow-up. DFS was defined as the time from diagnosis to death, recurrence, or last follow-up.
Construction and validation of a nomogram
The OS and DFS nomograms were established based on Cox regression analyses. The score of each variable and the survival probability corresponding to the total score was automatically calculated. The performance of the nomogram was assessed through estimates of discrimination and calibration (1000 bootstrap resamples). Internal and external validation was performed in the development and validation cohorts, respectively. The discrimination performance of the nomogram was evaluated by concordance index (C-index) and receiver-operating characteristic curve. We used calibration curves to assess the consistency between the predicted and actual 5-year OS/DFS probabilities.
Furthermore, a risk-stratification system was developed using the best cut-off value of the total score in the development cohort. The cut-off value of the total score was obtained from X-tile plots. Patients were assigned to three risk subgroups: low, intermediate, and high risk. Survival differences among the three groups were analyzed by Kaplan-Meier analysis. Quantitative variables were compared between groups using Student’s t test. All statistical analyses were conducted using IBM SPSS statistics version 25.0 (SPSS, Armonk, NY, USA), R software version 4.04 (www.r-project.org), and X-tile Software (Yale University School of Medicine, New Haven, CT, USA). Differences were considered significant at P<0.05.
Results
Baseline characteristics
A total of 4,065 consecutive patients between 2004 and 2013 were included in the present study (Figure 1). The median follow up in the development and validation cohorts was 40 and 51 months, respectively. All patients had all nomogram variables available and were included in the model. Table 1 shows the characteristics of patients in the development and validation cohorts. There was no significant difference in age, stromal invasion, and the margin between the development and the validation cohorts (P>0.05).
Table 1
Variable | Overall (n=4,065) | Development cohort (n=3,074) | Validation cohort (n=991) | P value |
---|---|---|---|---|
Age | 47.96±9.86 | 47.46±9.76 | 0.165 | |
FIGO 2018 stage (%) | 0.015 | |||
IB1 | 703 (17.3) | 507 (16.5) | 196 (19.8) | |
IB2 | 2,338 (57.5) | 1,804 (58.7) | 534 (53.9) | |
IIA1 | 1,024 (25.2) | 763 (24.8) | 261 (26.3) | |
Histological type (%) | 0.028 | |||
SCC | 3,596 (88.5) | 2,735 (89) | 861 (86.9) | |
AC | 370 (9.1) | 275 (8.9) | 95 (9.6) | |
SAC | 99 (2.4) | 64 (2.1) | 35 (3.5) | |
Stromal invasion (%) | 0.458 | |||
≤1/2 | 2,281 (56.1) | 1,735 (56.4) | 546 (55.1) | |
>1/2 | 1,784 (43.9) | 1,339 (43.6) | 445 (44.9) | |
LVSI (%) | 0.001 | |||
Negative | 3,506 (86.2) | 2,621 (85.3) | 885 (89.3) | |
Positive | 559 (13.8) | 453 (14.7) | 106 (10.7) | |
Margin (%) | 0.645 | |||
Negative | 3,986 (98.1) | 3,016 (98.1) | 970 (97.9) | |
Positive | 79 (1.9) | 58 (1.9) | 21 (2.1) |
AC, adenocarcinoma; FIGO, International Federation of Gynecology and Obstetrics; LVSI, lymphatic vascular invasion; SAC, adenosquamous carcinoma; SCC, squamous cell carcinoma.
Independent predictors in the development cohort
The results from the univariate analysis were as follows: age, FIGO stage, stromal invasion, and LVSI were significantly associated with OS; age, FIGO stage, histological type, stromal invasion, and LVSI were prognostic factors of DFS. The multivariable analysis showed that age, FIGO stage, stromal invasion, and LVSI were independent prognostic factors for OS; FIGO stage, histological type, stromal invasion, and LVSI were independent prognostic factors for DFS (Tables 2,3).
Table 2
Variable | Univariate analysis | Multivariate analysis | |||
---|---|---|---|---|---|
HR (95% CI) | P value | HR (95% CI) | P value | ||
Age | 1.039 (1.02–1.059) | <0.001*** | 1.025 (1.005–1.046) | 0.012* | |
FIGO 2018 stage | |||||
IB1 | Reference | Reference | |||
IB2 | 4.497 (1.638–12.35) | 0.004 | 3.2 (1.149–8.912) | 0.026* | |
IIA1 | 7.856 (2.833–21.786) | <0.001*** | 4.145 (1.449–11.857) | 0.008** | |
Histological type | |||||
SCC | Reference | Reference | |||
AC | 1.047 (0.547–2.005) | 0.89 | 1.429 (0.74–2.76) | 0.287 | |
SAC | 1.79 (0.842–3.806) | 0.226 | 2.386 (0.872–6.529) | 0.09 | |
Stromal invasion | |||||
≤1/2 | Reference | Reference | |||
>1/2 | 3.025 (2.035–4.498) | <0.001*** | 2.109 (1.392–3.196) | <0.001*** | |
LVSI | |||||
Negative | Reference | Reference | |||
Positive | 2.095 (1.389–3.161) | <0.001*** | 1.695 (1.118–2.572) | 0.013* | |
Margin | |||||
Negative | Reference | Reference | |||
Positive | 1.377 (0.438–4.335) | 0.584 | 0.865 (0.271–2.759) | 0.807 |
*P<0.05; **P<0.01; ***P<0.001. AC, adenocarcinoma; CI, confidence interval; FIGO, International Federation of Gynecology and Obstetrics; HR, hazard ratio; LVSI, lymphatic vascular invasion; SAC, adenosquamous carcinoma; SCC, squamous cell carcinoma.
Table 3
Variable | Univariate analysis | Multivariate analysis | |||
---|---|---|---|---|---|
HR (95% CI) | P value | HR (95% CI) | P value | ||
Age | 1.018 (1.005–1.031) | 0.008** | 1.008 (0.995–1.023) | 0.231 | |
FIGO 2018 stage | |||||
IB1 | Reference | Reference | |||
IB2 | 1.662 (1.058–2.612) | 0.027* | 1.298 (0.814–2.068) | 0.274 | |
IIA1 | 2.469 (1.542–3.954) | <0.001*** | 1.744 (1.059–2.874) | 0.029* | |
Histological type | |||||
SCC | Reference | Reference | |||
AC | 1.503 (1.012–2.232) | 0.044* | 1.779 (1.191–2.657) | 0.005** | |
SAC | 1.655 (0.779–3.516) | 0.19 | 1.79 (0.842–3.806) | 0.131 | |
Stromal invasion | |||||
≤1/2 | Reference | Reference | |||
>1/2 | 2.321 (1.778–3.03) | <0.001*** | 2.04 (1.535–2.71) | <0.001*** | |
LVSI | |||||
Negative | Reference | Reference | |||
Positive | 1.58 (1.156–2.159) | 0.004** | 1.379 (1.003–1.895) | 0.048* | |
Margin | |||||
Negative | Reference | Reference | |||
Positive | 1.627 (0.767–3.452) | 0.205 | 1.214 (0.565–2.607) | 0.619 |
*P<0.05; **P<0.01; ***P<0.001. AC, adenocarcinoma; CI, confidence interval; FIGO, International Federation of Gynecology and Obstetrics; HR, hazard ratio; LVSI, lymphatic vascular invasion; SAC, adenosquamous carcinoma; SCC, squamous cell carcinoma.
Model development
Based on the independent prognostic factors obtained by the multivariate Cox regression model, OS and DFS nomograms were constructed (Figure 2). Internal validation was performed using the bootstrapping correction technique. The estimated 5-year OS/DFS probability can be obtained by summing up the points of each variable to find the corresponding point on the total points axis and drawing a vertical line from that point down. The higher the overall score calculated from the nomograms, the higher the likelihood of death or recurrence.
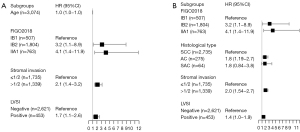
Model validation
Discrimination
Both internal and external validation was performed for the nomograms. Both the OS and DFS nomograms showed greater discrimination than the FIGO staging system in the development cohort [OS nomogram vs. FIGO 2018: C-index =0.69 vs. 0.61, area under the curve (AUC): 69.8 vs. 60.3; DFS nomogram vs. FIGO 2018: C-index =0.64 vs. 0.57, AUC: 62.6 vs. 56.9]. Results were the same for the validation cohort (Figure 3).
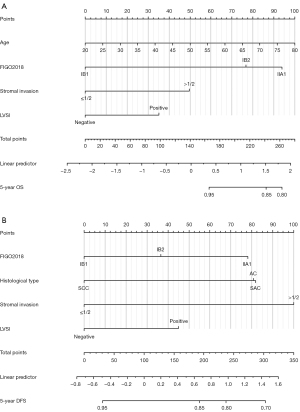
Calibration
The 5-year OS/DFS probability predicted by the nomograms and the actual 5-year OS/DFS probability are shown in the calibration plots (Figure 4). Calibration plots demonstrated good agreement between the predicted and actual probabilities of 5-year OS/DFS.
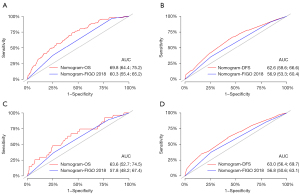
Risk-stratification system
The risk-stratification system was established by calculating the total score of patients in the developmental cohort and stratifying them according to the optimal cut-off value. Patients were classified into three risk subgroups according to the OS nomogram: low risk (<141.22), intermediate risk (≥141.22, <206.32), and high risk (≥206.32); patients were classified into three risk subgroups according to the DFS nomogram: low risk (<119.53), intermediate risk (≥119.53, <226.98), and high risk (≥226.98). Each risk subgroup presented a distinct prognosis, and this system accurately distinguished the OS/DFS of the three subgroups (P<0.05) (Figure 5). According to the grouping criteria, survival analysis was performed for each risk subgroup in the development and validation cohorts. The results indicated that the risk-stratification system accurately differentiated the three subgroups according to OS/DFS (Figure 6).
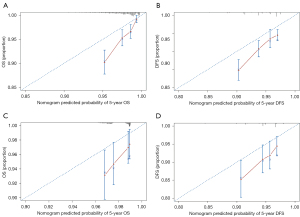
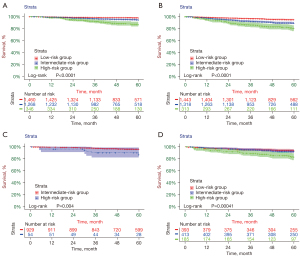
Discussion
We developed and validated a robust prediction model that can be used to predict 5-year OS and 5-year DFS for stages IB1, IB2, and IIA1 cervical cancer patients using a Chinese database. The nomogram showed good prediction accuracy in the development and validation cohorts. The nomogram can predict patient prognosis individually and showed better predictive performance than the FIGO staging system. In addition, the established risk-stratification system differentiates patients with different prognosis, making it easier to identify high-risk patients and improve treatment strategies and follow-up plans.
Individual prognosis is essential for both patients and doctors, but FIGO staging alone is too simplified to predict prognosis. Therefore, patients with equivalent anatomical spread, yet variable, outcomes (recurrence or mortality) are categorized into the same stage, introducing heterogeneity. At the same time, the FIGO 2018 guidelines included imaging or pathological evidence to form a new stage of cervical cancer. All cases not exceeding FIGO 2009 stage IIIB are classified into the new stage IIIC stage, as long as lymph node metastasis is positive (1). However, the extent of stage migration and the changes in survival outcomes are not clear. In addition to FIGO staging, many other prognostic factors affect the oncological outcome of patients with cervical cancer (14-22).
One of the main advantages of a nomogram is that it can estimate the risk of individual endpoint events according to the characteristics of patients and diseases. As far as we know, a nomogram has been used to predict the prognosis of various cancers (23-41). Although other researchers have created predictive models for cervical cancer, these nomograms have not been widely accepted or put into extensive clinical practice (6,42-46). Few nomograms for estimating the outcome of patients with FIGO 2018 stages IB1, IB2, and IIA1 cervical cancer have been published.
Nomograms have been used for risk stratification in several other diseases (6,7,33,47). The risk-stratification system established in the present study can accurately divide patients into three risk subgroups with significantly distinct prognoses to help clinicians evaluate a particular patient to determine that individual’s prognosis and conduct strict follow up.
To our knowledge, our study is the first to develop a multifactorial prognostic model in FIGO 2018 stages IB1, IB2, and IIA2 cervical cancer that has been externally validated. To date, a total of three nomograms have been published predicting the outcome of cervical cancer in the FIGO stage 2018 (8,48,49). Tang et al. included patients with FIGO stage IA1-IIA2 cervical cancer who received surgical treatment (48). As we all know, both surgical approach and hysterectomy type are known as risk factors affecting the prognosis of cervical cancer patients. However, in Tang’s study, there is no data screening for these two variables, leading to the fact that not all the cases included are those receiving standard treatment. Therefore, these confounding variables will affect the accuracy of the model and reduce the reliability of the prediction results. In our nomogram, all patients were screened by strict inclusion and exclusion criteria to ensure the reliability of the research results and a high reference value. The other two studies predicted the prognosis of locally advanced cervical cancer who received radical chemoradiotherapy (8,49). However, both were single-center studies without external validation. One of the advantages of our research is the analysis of a significant amount of data using a multicenter approach. This convenient and straightforward nomogram was established with clinically available variables, and clinicians can use this nomogram to predict the prognosis of patients immediately.
However, there are some limitations to our study. First, this was a retrospective study, which affects the accuracy of restaging to a certain extent. Second, there might be differences in preoperative imaging examinations and medical record report writing standards at different hospitals, resulting in a lack of clinical data to a certain extent. Therefore, some cases will be lost because they cannot be restaged. Even if the follow up was multilevel, the follow-up rate was still lower than the international standard follow-up rate of malignant tumors (50).
In the present study, we successfully developed a novel nomogram for predicting the 5-year OS and 5-year DFS of stages IB1, IB2, and IIA1 (FIGO 2018) cervical cancer patients for the first time. Internal and external validation showed that the model had good prediction performance and was superior to the currently utilized FIGO staging system. The nomogram is a ready-to-use tool, making it widely used in clinical practice. However, the model needs to be further verified in prospective studies to evaluate the prediction accuracy.
Acknowledgments
We are grateful to Min Hao (Department of Obstetrics and Gynecology, The Second Hospital of ShanXi Medical University), Wuliang Wang (Department of Obstetrics and Gynecology, The Second Affiliated Hospital of Zhengzhou University), Ying Yang (Department of Obstetrics and Gynecology, Xinqiao Hospital, Third Military Medical University), Shan Kang (Department of Obstetrics and Gynecology, The Forth Hospital of Hebei Medical University & The Tumor Hospital of Hebei Province), Bin Ling (Department of Obstetrics and Gynecology, China-Japan Friendship Hospital), Xinli Sun (Department of Gynecology, Shanxi Provincial Cancer Hospital), Hongwei Zhao (Department of Gynecology, Shanxi Provincial Cancer Hospital), Yu Guo (Department of Gynecology, Anyang Tumor Hospital), Li Wang (Department of Gynecology, The Affiliated Tumor Hospital of Zhengzhou University), Weidong Zhao (Department of Gynecology and Oncology, Anhui Provincial Cancer Hospital), Wentong Liang (Department of Obstetrics and Gynecology, Guizhou Provincial People’s Hospital), Jianxin Guo (Department of Obstetrics and Gynecology, Daping Hospital, The Third Military 18 Medical University), Mingwei Li (Department of Gynecology, Jiangmen Central Hospital), Weifeng Zhang (Department of Obstetrics and Gynecology, Ningbo Women & Children’s Hospital), Peiyan Du (Department of Gynecological Oncology, Cancer Center of Guangzhou Medical University), Ziyu Fang (Department of Obstetrics and Gynecology, Liuzhou Workers’ Hospital), Long Chen (Department of Obstetrics and Gynecology, Qingdao Municipal Hospital), Ruilei Liu (Department of Obstetrics and Gynecology, Linyi People’s Hospital), Mubiao Liu and (Department of Obstetrics and Gynecology, Zhujiang Hospital, Southern Medical University), Yuanli He (Department of Obstetrics and Gynecology, Zhujiang Hospital, Southern Medical University), Jilong Yao (Department of Obstetrics and Gynecology, Shenzhen Maternity & Child Healthcare Hospital), Zhihua Liu (Department of Obstetrics and Gynecology, Shenzhen Maternity & Child Healthcare Hospital), Xueqin Wang (Department of Obstetrics and Gynecology, The Fifth Affiliated Hospital of Southern Medical University), Yan Xu (Department of Obstetrics and Gynecology, Guangzhou Panyu Central Hospital), Ben Ma (Department of Obstetrics and Gynecology, Guangzhou First People’s Hospital), Zhonghai Wang (Department of Obstetrics and Gynecology, Shenzhen Nanshan People’s Hospital), Lin Zhu (Department of Gynecology, The Second Hospital of Shandong University), Hongxin Pan (Department of Obstetrics and Gynecology, the Third Affiliated Hospital of Shenzhen University, Luohu People’s Hospital), Qianyong Zhu (Department of Obstetrics and Gynecology, No.153. Center Hospital of 398 Liberation Army, Hospital No. 988 of the Chinese People’s Liberation Army Joint Support Force), Xiaohong Wang (Department of Obstetrics and Gynecology, Jinan City People’s Hospital, Former Laiwu People’s Hospital), Dingyuan Zeng (Department of Obstetrics and Gynecology, Maternal and Child Health Care Hospital of Liuzhou), Zhong Lin (Department of Obstetrics and Gynecology, Maternal and Child Health Care Hospital of Liuzhou), Shaoguang Wang (Department of Obstetrics and Gynecology, Yantai Yuhuangding Hospital), Bin Zhu (Department of Obstetrics and Gynecology, Yiwu Maternal and Child Health Hospital), Anwei Lu (Department of Obstetrics and Gynecology, Maternal and Child Health Care Hospital of Guizhou Province), Mei Ji (Department of Obstetrics and Gynecology, the First Affiliated Hospital of Zhengzhou University), Qianqing Wang (Department of Obstetrics and Gynecology, Central Hospital Affiliated to Xinxiang Medical University), Zhumei Cui (Department of Gynecology, The Affiliated Hospital of Qingdao University Medical College), Biliang Chen (Department of Gynecology, Xijing Hospital of Air Force Medical University), Qinghuang Xie (Department of Gynecology, Foshan Women and Children Hospital), Qiubo Lv (Department of Gynecology, Beijing Hospital), Chang Liu (Department of Gynecology, the First Hospital of Lanzhou University), and Yi Zhang (Department of Gynecology, the First Hospital of China Medical University) for providing medical records.
Funding: This study received funding from the National Science and Technology Support Program of China (No. 2014BAI05B03), the Natural Science Fund of Guangdong Province (No. 2015A030311024), and the Science and Technology Plan of Guangzhou (No. 158100075).
Footnote
Provenance and Peer Review: This article was commissioned by the Guest Editor (Danbo Wang) for the series “New Progress and Challenge in Gynecological Cancer” published in Annals of Translational Medicine. The article has undergone external peer review.
Reporting Checklist: The authors have completed the STROBE reporting checklist. Available at https://atm.amegroups.com/article/view/10.21037/atm-21-6367/rc
Data Sharing Statement: Available at https://atm.amegroups.com/article/view/10.21037/atm-21-6367/dss
Conflicts of Interest: All authors have completed the ICMJE uniform disclosure form (available at https://atm.amegroups.com/article/view/10.21037/atm-21-6367/coif). The series “New Progress and Challenge in Gynecological Cancer” was commissioned by the editorial office without any funding or sponsorship. The authors have no other conflicts of interest to declare.
Ethical Statement: The authors are accountable for all aspects of the work in ensuring that questions related to the accuracy or integrity of any part of the work are appropriately investigated and resolved. All procedures performed in this study involving human participants were in accordance with the Declaration of Helsinki (as revised in 2013). The Ethics Committee of Nanfang Hospital affiliated with Southern Medical University (Guangzhou, China) approved the study (ethics No. NFEC-2017-135). Individual consent for this retrospective analysis was waived.
Open Access Statement: This is an Open Access article distributed in accordance with the Creative Commons Attribution-NonCommercial-NoDerivs 4.0 International License (CC BY-NC-ND 4.0), which permits the non-commercial replication and distribution of the article with the strict proviso that no changes or edits are made and the original work is properly cited (including links to both the formal publication through the relevant DOI and the license). See: https://creativecommons.org/licenses/by-nc-nd/4.0/.
References
- Corrigendum to. "Revised FIGO staging for carcinoma of the cervix uteri" Int J Gynecol Obstet 145(2019) 129-135. Int J Gynaecol Obstet 2019;147:279-80. [Crossref] [PubMed]
- Iasonos A, Schrag D, Raj GV, et al. How to build and interpret a nomogram for cancer prognosis. J Clin Oncol 2008;26:1364-70. [Crossref] [PubMed]
- Huang YQ, Liang CH, He L, et al. Development and Validation of a Radiomics Nomogram for Preoperative Prediction of Lymph Node Metastasis in Colorectal Cancer. J Clin Oncol 2016;34:2157-64. [Crossref] [PubMed]
- Balachandran VP, Gonen M, Smith JJ, et al. Nomograms in oncology: more than meets the eye. Lancet Oncol 2015;16:e173-80. [Crossref] [PubMed]
- Wang Y, Li J, Xia Y, et al. Prognostic nomogram for intrahepatic cholangiocarcinoma after partial hepatectomy. J Clin Oncol 2013;31:1188-95. [Crossref] [PubMed]
- Briganti A, Larcher A, Abdollah F, et al. Updated nomogram predicting lymph node invasion in patients with prostate cancer undergoing extended pelvic lymph node dissection: the essential importance of percentage of positive cores. Eur Urol 2012;61:480-7. [Crossref] [PubMed]
- Valentini V, van Stiphout RG, Lammering G, et al. Nomograms for predicting local recurrence, distant metastases, and overall survival for patients with locally advanced rectal cancer on the basis of European randomized clinical trials. J Clin Oncol 2011;29:3163-72. [Crossref] [PubMed]
- Yang X, An J, Zhang Y, et al. Prognostic Nomograms Predicting Survival in Patients With Locally Advanced Cervical Squamous Cell Carcinoma: The First Nomogram Compared With Revised FIGO 2018 Staging System. Front Oncol 2020;10:591700. [Crossref] [PubMed]
- Koh WJ, Abu-Rustum NR, Bean S, et al. Cervical Cancer, Version 3.2019, NCCN Clinical Practice Guidelines in Oncology. J Natl Compr Canc Netw 2019;17:64-84. [Crossref] [PubMed]
- Li W, Liu P, Zhao W, et al. Effects of preoperative radiotherapy or chemoradiotherapy on postoperative pathological outcome of cervical cancer--from the large database of 46,313 cases of cervical cancer in China. Eur J Surg Oncol 2020;46:148-54. [Crossref] [PubMed]
- Chen B, Ji M, Li P, et al. Comparison between robot-assisted radical hysterectomy and abdominal radical hysterectomy for cervical cancer: A multicentre retrospective study. Gynecol Oncol 2020;157:429-36. [Crossref] [PubMed]
- Chen C, Wang W, Liu P, et al. Survival After Abdominal Q-M Type B versus C2 Radical Hysterectomy for Early-Stage Cervical Cancer. Cancer Manag Res 2019;11:10909-19. [Crossref] [PubMed]
- Liang C, Liu P, Cui Z, et al. Effect of laparoscopic versus abdominal radical hysterectomy on major surgical complications in women with stage IA-IIB cervical cancer in China, 2004-2015. Gynecol Oncol 2020;156:115-23. [Crossref] [PubMed]
- Rutledge FN, Mitchell MF, Munsell M, et al. Youth as a prognostic factor in carcinoma of the cervix: a matched analysis. Gynecol Oncol 1992;44:123-30. [Crossref] [PubMed]
- Dattoli MJ, Gretz HF 3rd, Beller U, et al. Analysis of multiple prognostic factors in patients with stage IB cervical cancer: age as a major determinant. Int J Radiat Oncol Biol Phys 1989;17:41-7. [Crossref] [PubMed]
- Chen F, Trapido EJ, Davis K. Differences in stage at presentation of breast and gynecologic cancers among whites, blacks, and Hispanics. Cancer 1994;73:2838-42. [Crossref] [PubMed]
- Katz A, Eifel PJ, Moughan J, et al. Socioeconomic characteristics of patients with squamous cell carcinoma of the uterine cervix treated with radiotherapy in the 1992 to 1994 patterns of care study. Int J Radiat Oncol Biol Phys 2000;47:443-50. [Crossref] [PubMed]
- Dunst J, Kuhnt T, Strauss HG, et al. Anemia in cervical cancers: impact on survival, patterns of relapse, and association with hypoxia and angiogenesis. Int J Radiat Oncol Biol Phys 2003;56:778-87. [Crossref] [PubMed]
- Toita T, Nakano M, Higashi M, et al. Prognostic value of cervical size and pelvic lymph node status assessed by computed tomography for patients with uterine cervical cancer treated by radical radiation therapy. Int J Radiat Oncol Biol Phys 1995;33:843-9. [Crossref] [PubMed]
- Perez CA, Grigsby PW, Nene SM, et al. Effect of tumor size on the prognosis of carcinoma of the uterine cervix treated with irradiation alone. Cancer 1992;69:2796-806. [Crossref] [PubMed]
- Hong JH, Tsai CS, Lai CH, et al. Risk stratification of patients with advanced squamous cell carcinoma of cervix treated by radiotherapy alone. Int J Radiat Oncol Biol Phys 2005;63:492-9. [Crossref] [PubMed]
- Tsai CC, Lin H, Huang EY, et al. The role of the preoperative serum carcinoembryonic antigen level in early-stage adenocarcinoma of the uterine cervix. Gynecol Oncol 2004;94:363-7. [Crossref] [PubMed]
- Gospodarowicz M, Benedet L, Hutter RV, et al. History and international developments in cancer staging. Cancer Prev Control 1998;2:262-8. [PubMed]
- Cho CS, Gonen M, Shia J, et al. A novel prognostic nomogram is more accurate than conventional staging systems for predicting survival after resection of hepatocellular carcinoma. J Am Coll Surg 2008;206:281-91. [Crossref] [PubMed]
- Kattan MW. Nomograms are superior to staging and risk grouping systems for identifying high-risk patients: preoperative application in prostate cancer. Curr Opin Urol 2003;13:111-6. [Crossref] [PubMed]
- Wong SL, Kattan MW, McMasters KM, et al. A nomogram that predicts the presence of sentinel node metastasis in melanoma with better discrimination than the American Joint Committee on Cancer staging system. Ann Surg Oncol 2005;12:282-8. [Crossref] [PubMed]
- Weiser MR, Gönen M, Chou JF, et al. Predicting survival after curative colectomy for cancer: individualizing colon cancer staging. J Clin Oncol 2011;29:4796-802. [Crossref] [PubMed]
- Ross PL, Gerigk C, Gonen M, et al. Comparisons of nomograms and urologists’ predictions in prostate cancer. Semin Urol Oncol 2002;20:82-8. [Crossref] [PubMed]
- Specht MC, Kattan MW, Gonen M, et al. Predicting nonsentinel node status after positive sentinel lymph biopsy for breast cancer: clinicians versus nomogram. Ann Surg Oncol 2005;12:654-9. [Crossref] [PubMed]
- Yu C, Zhang Y. Development and validation of prognostic nomogram for young patients with gastric cancer. Ann Transl Med 2019;7:641. [Crossref] [PubMed]
- Sjoquist KM, Renfro LA, Simes RJ, et al. Personalizing Survival Predictions in Advanced Colorectal Cancer: The ARCAD Nomogram Project. J Natl Cancer Inst 2018;110:638-48. [Crossref] [PubMed]
- Diao JD, Ma LX, Sun MY, et al. Construction and validation of a nomogram to predict overall survival in patients with inflammatory breast cancer. Cancer Med 2019;8:5600-8. [Crossref] [PubMed]
- Liang W, Zhang L, Jiang G, et al. Development and validation of a nomogram for predicting survival in patients with resected non-small-cell lung cancer. J Clin Oncol 2015;33:861-9. [Crossref] [PubMed]
- He Y, Mao M, Shi W, et al. Development and validation of a prognostic nomogram in gastric cancer with hepatitis B virus infection. J Transl Med 2019;17:98. [Crossref] [PubMed]
- Kattan MW, Zelefsky MJ, Kupelian PA, et al. Pretreatment nomogram for predicting the outcome of three-dimensional conformal radiotherapy in prostate cancer. J Clin Oncol 2000;18:3352-9. [Crossref] [PubMed]
- Kattan MW, Leung DH, Brennan MF. Postoperative nomogram for 12-year sarcoma-specific death. J Clin Oncol 2002;20:791-6. [Crossref] [PubMed]
- Kattan MW, Karpeh MS, Mazumdar M, et al. Postoperative nomogram for disease-specific survival after an R0 resection for gastric carcinoma. J Clin Oncol 2003;21:3647-50. [Crossref] [PubMed]
- Rouzier R, Preti M, Haddad B, et al. Development and validation of a nomogram for predicting outcome of patients with vulvar cancer. Obstet Gynecol 2006;107:672-7. [Crossref] [PubMed]
- Wang X, Ke X, Min J. A prognostic nomogram for women with primary ovarian signet-ring cell carcinoma. Ann Transl Med 2021;9:525. [Crossref] [PubMed]
- Wang N, Liu F, Xi W, et al. Development and validation of risk and prognostic nomograms for bone metastases in Chinese advanced colorectal cancer patients. Ann Transl Med 2021;9:875. [Crossref] [PubMed]
- Kong TW, Kim J, Son JH, et al. Preoperative nomogram for prediction of microscopic parametrial infiltration in patients with FIGO stage IB cervical cancer treated with radical hysterectomy. Gynecol Oncol 2016;142:109-14. [Crossref] [PubMed]
- Wang T, Gao T, Guo H, et al. Preoperative prediction of parametrial invasion in early-stage cervical cancer with MRI-based radiomics nomogram. Eur Radiol 2020;30:3585-93. [Crossref] [PubMed]
- Benoit L, Balaya V, Guani B, et al. Nomogram Predicting the Likelihood of Parametrial Involvement in Early-Stage Cervical Cancer: Avoiding Unjustified Radical Hysterectomies. J Clin Med 2020;9:2121. [Crossref] [PubMed]
- Tseng JY, Yen MS, Twu NF, et al. Prognostic nomogram for overall survival in stage IIB-IVA cervical cancer patients treated with concurrent chemoradiotherapy. Am J Obstet Gynecol 2010;202:174.e1-7. [Crossref] [PubMed]
- Du W, Wang Y, Li D, et al. Preoperative Prediction of Lymphovascular Space Invasion in Cervical Cancer With Radiomics -Based Nomogram. Front Oncol 2021;11:637794. [Crossref] [PubMed]
- Sturdza AE, Pötter R, Kossmeier M, et al. Nomogram Predicting Overall Survival in Patients With Locally Advanced Cervical Cancer Treated With Radiochemotherapy Including Image-Guided Brachytherapy: A Retro-EMBRACE Study. Int J Radiat Oncol Biol Phys 2021;111:168-77. [Crossref] [PubMed]
- Hyder O, Marques H, Pulitano C, et al. A nomogram to predict long-term survival after resection for intrahepatic cholangiocarcinoma: an Eastern and Western experience. JAMA Surg 2014;149:432-8. [Crossref] [PubMed]
- Tang X, Guo C, Liu S, et al. A novel prognostic nomogram utilizing the 2018 FIGO staging system for cervical cancer: A large multicenter study. Int J Gynaecol Obstet 2021;155:86-94. [Crossref] [PubMed]
- Kim J, Cho Y, Kim N, et al. Magnetic resonance imaging-based validation of the 2018 FIGO staging system in patients treated with definitive radiotherapy for locally advanced cervix cancer. Gynecol Oncol 2021;160:735-741. [Crossref] [PubMed]
- Kim SI, Lee M, Lee S, et al. Impact of laparoscopic radical hysterectomy on survival outcome in patients with FIGO stage IB cervical cancer: A matching study of two institutional hospitals in Korea. Gynecol Oncol 2019;155:75-82. [Crossref] [PubMed]