Modular characteristics and the mechanism of Chinese medicine’s treatment of gastric cancer: a data mining and pharmacology-based identification
Introduction
Globally, gastric cancer (GC) is the fourth most common cancer and the second leading cause of cancer deaths (1). Current approaches to GC management largely consist of endoscopic detection followed by gastrectomy and chemotherapy (CT) or chemo-radiotherapy (CRT); however, the available treatments have adverse side effects and are associated with high recurrence rates (2). Therefore, there is a need to address the current limitations of the various therapeutic strategies to facilitate possible clinical applications.
With the development of personalized and complementary medicine, multi-compound and multi-targeting traditional Chinese medicine (TCM) has been shown to be clinically effective in treating GC (3,4). However, due to the lack of large-scale evidence-based medicine, the extensive application of TCM remains inhibited. The use of TCM as an adjuvant therapy is greatly subjective because understanding on GC treatment varies among physicians in terms of etiology, syndrome differentiation, and medicinal prescriptions. Generally, the principal theory of Chinese traditional medicine for GC is invigorating the Spleen and tonifying the vital-Qi, and eliminating blood stasis and removing toxins. The largely unknown mechanism of these empirical prescriptions is another limiting factor for the use of TCM. Therefore, it is important and innovative to screen clinical prescriptions with good efficacy, based on which the obtainment of a basic prescription with general applicability for treating GC could be achieved by machine learning. What’s more, the elucidation of prescription patterns by data mining may promote both clinical application and basic researches on herbal pairs. To the best of our knowledge, another study with such an aim has not been previously reported.
In this study, we proposed a method of combining data mining and network pharmacology to systematically elucidate the prescription patterns of TCM, and unravel the modular functions and potential action mechanisms of TCM for treating GC. Additionally, the effects of the machine learning-based preferred prescription were validated in vivo and in vitro. We present the following article in accordance with the ARRIVE reporting checklist (available at https://dx.doi.org/10.21037/atm-21-6301).
Methods
Big data mining and machine learning
Source of literature, inclusion and exclusion criteria
All literature was obtained from the China National Knowledge Infrastructure (CNKI) database, which is the world’s largest Chinese knowledge portal website. The sources of the literature included the Academic Journals Full-text Database, Doctoral Dissertations Full-text Database, and Masters’. These Full-text Database (01/1990–12/2020). The combinational search terms were “Gastric cancer and/or gastric malignancy” and “Traditional Chinese Medicine”. Literature with the following criteria were included: (I) relevant to clinical research on using TCM in treating GC; (II) containing randomized controlled trial (RCT) as the study design; (III) containing prescriptions with complete and specific names of Chinese herbs; (IV) studies must have reported one or more of the following efficacy endpoints: progression-free survival (PFS), overall survival (OS), objective response rate (ORR), or adverse events (AEs) (5). The exclusion criteria were as follows: (I) duplicate publications reporting the same group of participants; (II) non-clinical studies including experimental research on cell lines, xenografts or animal models, or theoretical studies; (III) where TCM and western medicines were integrated as a therapeutic regimen; (IV) non-oral administrations including injection and nasogastric tube nutrition; (V) non-decoction dosage types including Chinese patent medicines and TCM for external use; (VI) use of prescriptions composed of an unspecified or single herb.
Data extraction
Firstly, the names of the prescriptions (ancient prescriptions or recombinant personalized prescriptions) and their constituent herbs were extracted from the eligible literature. Secondly, we referred to the Chinese Pharmacopoeia (2020 Edition) Volume I to standardize the names of each herb (6). Furthermore, the basic information on each herb was extracted from the Chinese Pharmacopoeia, including its Latin name, property, taste, and meridian tropism. The 5 properties of TCM herbs include cold, hot, warm, cool, and neutral. The 5 tastes of TCM herbs include sour, bitter, sweet, pungent, and salty. The various combinations of property and taste determine the herbs’ specific attributes, which can influence the Yin and Yang of the body. For example, herbs with warm and hot properties are used to invigorate the Yang in patients with heat-deficiency disorders. Likewise, sour, bitter, and salty tastes are related to Yin, whereas pungent and sweet pertain to Yang. The meridian serves as the pathway for the transportation of Qi and Blood throughout the body, and its tropism represents the selective therapeutic effects of a medicinal herb on a certain region of the human body (7). Moreover, the principal function of each herb was classified according to the Chinese Pharmacy (8).
Association rule mining (ARM)
To investigate the rules of herbal combinations in the prescriptions used in various studies, ARM, an in-silico screening process, was applied. In this scheme, the dataset and the association rules are defined as follows: an association rule has the form left hand side (LHS) ⇒ right hand side (RHS), where LHS and RHS are sets of items, with the likely occurrence of the RHS whenever the LHS set occurs (9). The Apriori algorithm was used to extract the significant associations from all possible combinations of the items from the main dataset (10). There are 3 evaluation metrics which are critical in describing the power and significance of the rules generated by ARM (11). Support is the frequency of the rule occurrence in the total dataset, measuring whether an association between the LHS and the RHS happens by chance. Confidence is the frequency of rule occurrence in the cases of the dataset fulfilling the LHS of the rule, thus, representing the reliability of the association. Lift is the ratio of observed support to the expected support when the LHS and the RHS are independent, indicating the dependency of the occurrences of the 2 items when its value is larger than 1 (12). To establish a proper threshold, we detected the central tendency of the association rules to be more obvious at the support of 0.1 and confidence of 0.6 in the correlation analysis of herbal combination patterns. Then, the herbs were categorized in Microsoft Excel 2010 (Microsoft Corp., Redmond, WA, USA) according to their properties, tastes, meridian tropisms, and functions. The software platform IBM SPSS Modeler 18.1 (IBM Corp., Armonk, NY, USA) was used to analyze the categorization-based frequency and the correlations of the prescription patterns and to generate a visual network diagram.
Cluster analysis
Clustering is central to many data-driven bioinformatics research and serves a powerful computational method. Deep learning can be effective means to transform mappings from a high-dimensional data space into a lower-dimensional feature space, leading to improved clustering results (13). In this study, we used IBM SPSS Modeler software platform to perform deep learning-based cluster analysis to identify the preferred regroups of the most frequently used herbs based on their attributes (14). In our study, k-means cluster analysis was considered since the variables were quantitative at the interval or ratio level rather than being binary or counts. To avoid unreliable results through omitted variable bias, we included all the attributes, including the 5 properties, 5 tastes, and meridian tropism, and investigated the therapeutic preferences of the candidate clusters. To assess the reliability of a given solution, we compared the results from analyses with different permutations of the initial center values to ensure an appropriate number of clusters.
Mechanism investigation of the candidate formulae by network pharmacology
Compounds library construction and active components screening of the candidate formulae
To build a compound library of the core herbs for GC, we extracted all the compounds of the candidate formulae from the Traditional Chinese Medicines for Systems Pharmacology Database and Analysis Platform (TCMSP; http://lsp.nwu.edu.cn/index.php), Traditional Chinese Medicines Integrated Database (TCMID; http://bionet.ncpsb.org/batman-tcm/), Bioinformatics Analysis Tool for Molecular Mechanism of Traditional Chinese Medicine (BATMAN-TCM; http://bionet.ncpsb.org/batman-tcm/), and wide-scale literature mining (15,16). To optimize the use of the high cost and time-consuming biological experiments and clinical research, absorption, distribution, metabolism, and excretion (ADME) evaluations are critical procedures for active components screening (17). In this study, oral bioavailability (OB) ≥30% and drug-likeness (DL) ≥0.18 were set as the threshold; however, compounds that did not meet these inclusion criteria but were supported by the literature were retained.
Therapeutic targets prediction of the candidate formulae
Computational predictions of bioactive molecule targets based on similarity with known ligands are powerful in narrowing down the number of potential targets and the rationalization of possible side effects of the known molecules (18). The prediction algorithms of the ligand-based strategies include systematic drug targeting (SysDT) (19) and weighted ensemble similarity (WES) models (20). The SysDT model was developed based on random forest (RF) and support vector machine (SVM), which performed impressively on systematic predictions for drug-target associations and interactions involving enzymes, ion channels, nuclear receptors, and G-protein coupled receptors (19). In the WES model, the standardized ensemble similarities (Z score) by Bayesian network are utilized and the targets are predicted using the multi-variate kernel approach (21). In our study, the predictive therapeutic targets of the candidate formulae were obtained from web tools including Search Tool for Interacting Chemicals (STITCH; http://stitch.embl.de/), similarity ensemble approach (SEA; http://sea.bkslab.org/) and SwissTargetPrediction (www.swisstargetprediction.ch) (22-24). Targets with RF ≥0.7, SVM ≥0.8, or Z score ≥7 were considered for further analysis and standardized to corresponding genes for homo sapiens through the UniProt database (https://www.uniprot.org/uploadlists/).
To evaluate the performances of the candidate formulae in treating GC, we mapped the predictive therapeutic targets to the GC-related genes/proteins, which were comprehensively collected from online databases including MalaCards (https://www.malacards.org/), Online Mendelian Inheritance in Man (OMIM; https://omim.org/), and DisGeNET v7.0 (https://www.disgenet.org/home/) (25,26). We visualized the results and generated an additional protein-protein interaction (PPI) network using Metascape (https://metascape.org/gp/index.html).
Construction and topological analysis of the compound-target network of the preferred prescription
Cytoscape v3.7.2 (https://cytoscape.org/) was used to construct a compound-target (C-T) network of the preferred prescription, and to analyze its degree, a key topological parameter for evaluation (15). In the C-T network, compounds sharing interactions with GC-related genes were determined as components that were beneficial for GC. Moreover, we considered the targets (compounds) with degree values equal to or above the mean value to be the predominant therapeutic targets (crucial substances).
Gene Ontology (GO) and pathway enrichment analysis of the preferred prescription
GO analysis, Kyoto Encyclopedia of Genes and Genomes (KEGG), and Reactome pathway enrichment of the preferred prescription were carried out using the Database for Annotation, Visualization, and Integrated Discovery system v6.8 (DAVID; https://david.ncifcrf.gov/) (27). We also used ClueGO, a Cytoscape v3.7.2 plug-in to identify the interactions among the various signaling pathways by generating a functionally grouped network (28,29). Based on the mechanism of GC, we further constructed a multi-regulation map of KEGG pathways of the crucial components in the core herbs.
Moreover, the modular functional characteristics of TCM in GC treatment were demonstrated in PPI networks, which visualized the interactions among significant targets that could be regulated by the crucial components in the preferred prescription. The PPI networks were generated by the GeneMANIA web site (http://genemania.org/) which offers a fast prediction on the functions of the given gene sets through the application of a guilt-by-association approach (30).
Molecular docking
The three-dimensional (3D) structures of the predominant targets of the preferred prescription were collected from protein data bank (PDB; http://www.rcsb.org). AutoDock Tools 1.5.6 software (https://autodock.scripps.edu/) was used to remove the water molecules, isolate proteins, add nonpolar hydrogen, and calculate Gasteiger charges for the structure (31). The preprocessed structures were saved as PBD with partial changes and AutoDock 4 atom types (PDBQT) files. The PubChem database (https://pubchem.ncbi.nlm.nih.gov/) was applied to download the two-dimensional (2D) structures of the crucial substances of the preferred prescription. The 2D structure was processed and transformed into PDB format via Open Babel (32), and then saved in PDBQT format as docking ligands in AutoDock Tools 1.5.6 software. The target proteins were used as receptors while the substances were used as ligands. The active site of molecular docking was determined by the complex of ligand and target protein. Autodock Vina 1.1.2 (https://vina.scripps.edu/) was used to dock small molecules with their target proteins. The conformation with the best affinity was selected as the final docking conformation and visualized in Pymol 2.5 (https://pymol.org/2/).
Experimental validation
Preparation of the preferred prescription and components identification
Crude TCM herbs [dried roots of Atractylodes macrocephala Koidz. 12 g, dried roots of Astragalus membranaceus (Fisch.) Bge. 30 g, dried mature pericarp of Citrus reticulata Blanco 10 g, dried tuber of Pineilia ternate (Thunb.) Breit. 9 g, dried root of Aucklandia lappa Decne. 6 g, dried mature fruits of Amomum villosum Lour. 3 g, dried immature fruits of Citrus aurantium L. 10 g, dried gizzard lining of Gallus gallus domesticus Brisson 10 g, dried mature fruits of Crataegus pinnatifida Bge. 12 g, dried mature fruits of Hordeum vulgare L. 15 g, Radix Actinidiae Chinensis 15 g, and Herba Hedyotidis Diffusae 15 g] were provided by Sanyue Chinese Traditional Medicine Co. (Nantong, China). All the herbs were soaked for 30 min in 1,800 mL double-distilled water and then boiled at minimum temperature for 30 min before being refluxed and extracted. The boiling process was repeated with 1,800 mL double-distilled water for 30 min. Then, 2 parts of the extracted solutions were mixed and vaporized to 60 mL. The decoction was finally concentrated to 1 g/mL and stored at −20 °C after being sterilized and filtered through a 0.22 µm filter. The extracts of the preferred prescription were detected and analyzed using high-performance liquid chromatography diode array detection (HPLC-DAD) (detailed information shown in the Supplementary materials).
In vitro, to determine the decoction dose, the half-maximal inhibitory concentration (IC50) of different GC cell lines were assessed by 3-(4,5-Dimethyl-2-thizolyl)-2,5-diphenyltetrazolium bromide (MTT) assay (detailed information shown in the Supplementary materials), and the dose range of 2, 4, 8 mg/mL was selected.
Cell apoptosis and cell cycle analyses
Human GC cell lines AGS, HGC27, MKN28, and SGC7901 were purchased from the Cell Bank of the Chinese Academy of Sciences (Shanghai, China). All cell lines were kept in a humidified atmosphere of 5% CO2 at 37 °C. For apoptosis analysis, the cells were measured using Annexin V-FITC/PI apoptosis detection kit (Keygen Biotech Co., Nanjing, China) by flow cytometry [Becton, Dickinson, and Co. (BD) Biosciences, Franklin Lakes, NJ, USA] according to the manufacturer’s instructions. Cell cycle distributions were determined using a cell cycle and apoptosis analysis kit (Beyotime Biotech Co., Shanghai, China) by flow cytometry (BD Biosciences).
Wound-healing assay
Cells (1,000×103 cells/well) were seeded into 6-well plates for 24 h, and scraped with a sterile pipette tip when 80% of the cells were adherent to the walls. Cells were treated with various concentrations of the preferred prescription after removing debris by phosphate-buffered saline (PBS). The scratch area was observed by microscopy at 0, 12, 24, and 48 h, respectively.
Invasion assay
The upper surface of the Transwell inserts (8 µm pore size, Merck & Millipore, Darmstadt, Germany) were coated with Matrigel (100 µL, diluted 1:29 with PBS) (Corning, Corning, NY, USA) before serum-free medium containing 2×105 cells were loaded. The lower chamber included 500 µL media containing 10% fasting blood sugar (FBS) and various concentrations of the preferred prescription. After 48 h, the chambers were removed, and nonpenetrative cells were washed from the top chamber with PBS. The invaded cells were fixed with 95% ethanol and stained with crystal violet. Image J (https://imagej.nih.gov/ij/) was applied to count the number of cells in images randomly taken under a microscope.
Western blot assay
Protein lysates were separated using sodium dodecyl sulfate polyacrylamide gel electrophoresis (SDS-PAGE) and transferred to polyvinylidene difluoride (PVDF) membranes. Membranes were blocked with 5% bovine serum albumin (BSA) for 1 h and incubated with primary antibodies at 4 °C overnight. The primary antibodies included β-actin, Bax, Bcl2, N-cadherin, Snail, Slug, hTERT, MDM2, p53, p21, cyclinE, and CDK2 [all antibodies were purchased from Cell Signaling Technology (CST) Danvers, MA, USA]. The secondary goat anti-rabbit horseradish peroxidase-conjugated antibody (ZSGB-BIO, Beijing, China) was incubated at room temperature for 1 h. Signals were examined using the Image Lab system, version 5.1 (Bio-Rad, Hercules, CA, USA).
In vivo study
Male BALB/c athymic nude mice (4–6 weeks old, 18–20 g) obtained from Charles River Co. (Beijing, China) were housed in a specific pathogen-free (SPF) environment. An appropriate amount of the preferred prescription extracts was collected and prepared into the 0.735 g/mL solution with distilled water, and used for the intragastric administration of the experimental animals. The MKN28 cells were collected and cultured in the logarithmic growth phase, and the density was adjusted to 5×107/mL. Each mouse was inoculated with 0.2 mL of cell suspension in the right armpit after disinfection. After 10 days, the diameter of the induration reached 3−7 mm, suggesting the establishment of a successful model. The 20 nude mice were divided into 4 groups (n=5 each) as follows: (I) model group with transplanted tumors given the gavage of distilled water; (II) 5-fluorouracil (5-FU) group with transplanted tumors given the intraperitoneal injection at a dose of 20 mg/kg body weight (BW) every 3 days; (III) preferred prescription group with transplanted tumors given the gavage of decoction at a dose of 14.7 g/kg BW every day for 14 days; (IV) the 5-FU+ preferred prescription group with transplanted tumors given the intraperitoneal injection at a dose of 20 mg/kg BW every 3 days and gavage of decoction at a dose of 14.7 g/kg BW every day for 14 days. To calculate the volume of the tumors, the dimension was measured by length (L) and width (W) using a caliper every 3 days. Mice were sacrificed by cervical dislocation, and the tumors were excised and weighed.
Ethical statement
This study was conducted in accordance with the Declaration of Helsinki (as revised in 2013). Animal experiment was performed under a project license (No. 2021DW-35-01) granted by the Animal Ethics Committee of Affiliated Hospital of Nanjing University of Chinese Medicine (Nanjing, China), in compliance with the recommendations in the Guide for the Care and Use of Laboratory Animals of the National Institutes of Health. A protocol was prepared before the study without registration.
Statistical analysis
The data were described as means ± standard error of the mean (SEM). Statistical significance was determined using one-way analysis of variance (ANOVA, comparison between multiple groups) and Tukey multiple comparison processing (comparison between the two groups), with a P value <0.05 indicating statistical significance. All experiments were repeated at least three times under the same conditions. The statistical analyses were performed using GraphPad Prism software (GraphPad Software, La Jolla, CA, USA).
Results
Screening of eligible literature, clinical study-based prescriptions, core herbs, and frequency distributions according to herbal attributes and principal functional categorizations
The framework of this study can be summarized as follows: (I) screening of clinical study based TCM prescriptions for GC treatment; (II) data mining of the treatment principles, prescription patterns, and generation of candidate formulae by deep machine learning; (III) prediction of the action mechanism of the preferred prescription by network pharmacology; (IV) validation of the antitumor effects of the preferred prescription by experiments in vivo and in vitro (Figure 1A).
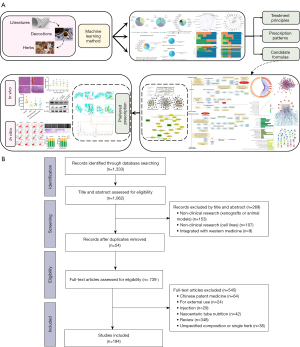
A total of 194 eligible prescriptions and 148 herbs with standardized names were screened from clinical studies spanning from January 1990 to December 2020. The screening process is summarized as a PRISMA flow diagram (33) (Figure 1B). The total cumulative occurrences of the 148 herbs in 194 prescriptions were 2,103 times. Herbs with over 20 times frequency of occurrence were selected as predominant ones used in clinic. The top 24 core herbs and their functional categorizations are listed in Table S1. Descriptive statistics of herbal attributes are shown in Figure 2A. In terms of the 5 properties; herbs with warm property were the most frequently prescribed. With regards to the 5 tastes, herbs with bitter (44.59%), pungent (39.86%), and sweet (36.49%) tastes ranked the top 3 in clinical application. In terms of meridian tropism, herbs with a propensity for the Liver (LR) (43.24%), Stomach (ST) (39.19%), and Spleen (SP) meridians were the most frequently used. The top 3 principal functions of the core herbs are demonstrated in Figure 2B. In summary, the treatment principle of TCM in GC is mainly invigorating the Spleen and tonifying the vital-Qi.
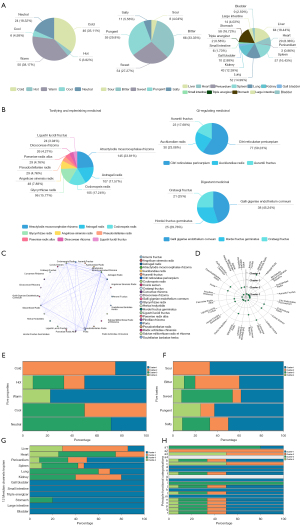
To facilitate better application of the core herbs in clinic, we summarized their clinical indications (Table S2). Particularly, herbs with the 3 major functions that embody TCM treatment principles for GC are listed in Table 1.
Table 1
Herbal nature | Principal functional categorizations | Number of prescriptions using the herbs | Frequency of use (%) | Syndromes | Key signs & symptoms | Treatment principles | Representative herbs |
---|---|---|---|---|---|---|---|
Sweet, warm | Qi-tonifying | 182 | 93.81 | Middle-Jiao Deficiency | Poor appetite, dislike to talk, lassitude, weak limbs, borborygmus, loose stools, heavy descending sensation in abdominal cavity, prolapse of rectum. | Tonify Middle-Jiao Qi | Atractylodis Macrocephalae Rhizoma, Astragali Radix, Codonopsis Radix, Glycyrrhizae Radix, Pseudostellariae Radix, Dioscoreae Rhizoma |
Pungent, warm/bitter, warm | Qi-regulating | 119 | 61.34 | Stagnation of Liver Qi | Mental depression, restlessness, sighing, distension, wandering pain in the costal and hypochondriac region, distress in epigastrium, poor appetite or vomiting, irregular bowel movements, thin greasy tongue coating, wiry pulse. | Disperse Liver Qi | Citri Reticulatae Pericarpium, Aucklandiae Radix, Aurantii Fructus, Fructus Evodiae |
Qi stagnation transforming into Fire | Irritability, stuffiness in the chest, hypochondriac distension, acid regurgitation, dry & bitter mouth, constipation or headache, tinnitus, red tongue & yellow coating, wiry-rapid pulse. | Purge Fire from Liver | |||||
Sweet, neutral | Food abating | 55 | 28.35 | Stomach excessive | Epigastric and abdominal distension and fullness or pain, which are aggravated by food intake, belching with foul smell, anorexia, constipation, acidic regurgitation, nausea, vomiting, diarrhea with foul smell or fermented contents or constipation | Dissolve the stagnation | Galli Gigeriae Endothelium Corneum, Hordei Fructus Germinatus, Crataegi Fructus |
TCM, traditional Chinese medicine; GC, gastric cancer.
Frequently prescribed herbal combination patterns by ARM and novel candidate formula prediction by cluster analysis
The ARM method was applied to analyze the combination patterns of the 194 prescriptions. Guided by the theory of synergy and attenuation in TCM, couplet herbs are 2 herbs administered together to enhance therapeutic effects or reduce toxicity. With a threshold of minimum support of 0.1 and confidence of 0.6, the prescribed pairs of couplet herbs with the top 3 confidence included; Atractylodis Macrocephalae Rhizoma (Bai Zhu) paired with Dioscoreae Rhizoma (Shan Yao; 92.31%), Atractylodis Macrocephalae Rhizoma paired with Aucklandiae Radix (Mu Xiang; 90%), and Atractylodis Macrocephalae Rhizoma paired with Codonopsis Radix (Dang Shen; 88.57%) (Table 2). Triplet herbs are a combination of 3 herbs, which interact with each other and are usually contained in a decoction or used as an independent decoction. Based on the established threshold above, the triplet combinations of herbs with the top 3 confidence included Poria (Fu Ling)-Aucklandiae Radix-Glycyrrhizae Radix (Gan Cao) (100%), Atractylodis Macrocephalae Rhizoma-Aucklandiae Radix-Codonopsis Radix (100%), and Atractylodis Macrocephalae Rhizoma-Galli Gigeriae Endothelium Corneum (Ji Nei Jin)-Codonopsis Radix (100%) (Table S3). A network diagram was generated to visualize the association rules among the core herbs (Figure 2C).
Table 2
Herb (LHS) | Number of prescriptions | Herb (RHS) | Number of occurrences | Support (LHS) (%) | Confidence (LHS ≥ RHS) (%) | LIFT | |
---|---|---|---|---|---|---|---|
Atractylodis Macrocephalae Rhizoma | 145 | Dioscoreae Rhizoma | → | 26 | 13.40 | 92.31 | 1.24 |
Atractylodis Macrocephalae Rhizoma | 145 | Aucklandiae Radix | → | 30 | 15.46 | 90.00 | 1.20 |
Atractylodis Macrocephalae Rhizoma | 145 | Codonopsis Radix | → | 105 | 54.12 | 88.57 | 1.19 |
Astragali Radix | 107 | Ligustri Lucidi Fructus | → | 24 | 12.37 | 87.50 | 1.59 |
Atractylodis Macrocephalae Rhizoma | 145 | Poria | → | 126 | 64.95 | 87.30 | 1.17 |
Atractylodis Macrocephalae Rhizoma | 145 | Coicis Semen | → | 63 | 32.47 | 87.30 | 1.17 |
Poria | 126 | Pseudostellariae Radix | → | 29 | 14.95 | 86.21 | 1.33 |
Poria | 126 | Amomi Fructus | → | 28 | 14.43 | 85.71 | 1.32 |
Atractylodis Macrocephalae Rhizoma | 145 | Amomi Fructus | → | 28 | 14.43 | 85.71 | 1.15 |
Codonopsis Radix | 105 | Hordei Fructus Germinatus | → | 25 | 12.89 | 84.00 | 1.55 |
LHS, left hand side; RHS, right hand side.
Additionally, the core herbs in the 194 prescriptions were regrouped into 4 clusters by machine learning. The cluster analysis result was presented in a 2D scatter diagram (Figure 2D). Cluster 1 (candidate formula 1, CF 1) included; Atractylodis Macrocephalae Rhizoma, Astragali Radix (Huang Qi), Pinelliae Rhizoma (Zhi Ban Xia), Citri Reticulatae Pericarpium (Chen Pi), Herba Hedyotidis (Bai Hua She She Cao), Galli Gigeriae Endothelium Corneum, Aucklandiae Radix, Amomi Fructus (Sha Ren), Hordei Fructus Germinatus (Mai Ya), Aurantii Fructus (Zhi Ke), Radix Actinidiae Chinensis (Mi Hou Tao Gen), Crataegi Fructus (Shan Zha); cluster 2 (candidate formula 2, CF 2) included; Paeoniae Radix Alba (Bai Shao), Scutellariae Barbatae Herba (Ban Zhi Lian), Salviae Miltiorrhizae Radix et Rhizoma (Dan Shen), Ligustri Lucidi Fructus (Nu Zhen Zi); cluster 3 (candidate formula 3, CF 3) included; Poria, Codonopsis Radix, Glycyrrhizae Radix, Coicis Semen (Yi Yi Ren), Pseudostellariae Radix (Tai Zi Shen), Dioscoreae Rhizoma; and cluster 4 (candidate formula 4, CF 4) included; Angelicae Sinensis Radix (Dang Gui), Curcumae Rhizoma (E Zhu). For a clearer understanding of the functions of the candidate formulae, the herbal attributes preferences of the 4 candidate formulae are shown in a distribution histogram (Figure 2E-2H). The CF 1 and CF 2 were composed of more herbs with warm and cold properties respectively, while the property of CF 3 appeared to be milder. With regards to the 5 tastes, the majority of the herbs in the CF 1 possessed sour and pungent tastes, while most herbs with sweet tastes were clustered in CF 3. Meridian tropism represents the selective therapeutic effects of a Chinese herb on a certain region of the human body (7). The CF 1, CF 2, and CF 3 prescribed more herbs belonging to SP (ST), LR, and KI meridians, respectively. Specifically, the CF 1 and CF 3 clusters were distinguished for tonifying Qi and invigorating the Spleen, and regulating Qi and resolving dampness, which meant both of them could increase appetite, alleviate lassitude, fullness sensation in the upper abdomen, and loose stools, as well as help GC patients feel less depressive. While the CF 2 and CF 4 seemed to play a significant role in tonifying the Blood and promoting blood circulation, which indicated they are more applicable for GC patients with symptoms like pale complexion, dizziness, insomnia, distending pain of the hypochondrium, and so on.
Active components library construction and therapeutic targets prediction of the candidate formulae
Complied with OB ≥30% and DL ≥0.18, 305 compounds of the 24 core herbs were screened out as bioactive components (Table S4). The numbers of active components in CF 1-4 were 74, 106, 154, and 5, respectively. With 35 compounds hitting no corresponding targets, a total of 2,128 predictive targets were retrieved and normalized via prediction databases and UniProt, with the potential to interact with 305 active components.
To investigate the relationship between the predictive targets and GC, 429 GC-related genes were screened, and 136 targets overlapped. As shown in Figure 3A, CF 1 targeted the most GC-related genes and was defined as the “preferred prescription” in our study. The CF 1-4 contributed to 135, 12, 13, and 4 genes/proteins, respectively. As shown in Figure 3B, the shared genes/proteins among the 4 formulae included human telomerase reverse transcriptase (hTERT), tyrosine-protein phosphatase non-receptor type 11 (PTPN11), estrogen receptor (ESR1), and sonic hedgehog protein (SHH), G2/mitotic-specific cyclin-B1 (CCNB1), fibroblast growth factor 2 (FGF2), and so on.
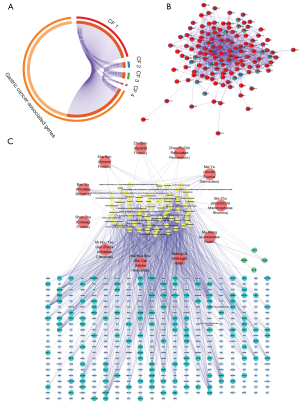
Construction and topological analysis of the compound-target network of the preferred prescription
Topological analysis of the C-T network was conducted to identify the crucial components and targets in the preferred prescription. As shown in Figure 3C, the network embodied 505 nodes (11 herbs, 61 active components, and 429 target genes/proteins), and 952 C-T interactions. The mean degree of the active components was 15.61. There were 23 compounds with a degree value higher than 15.61. In this network, crucial substances quercetin (Astragali Radix, Herba Hedyotidis, Radix Actinidiae Chinensis, Crataegi Fructus), kaempferol (Astragali Radix), baicalein (Pinelliae Rhizoma), and nobiletin (Citri Reticulatae Pericarpium, Aurantii Fructus) targeted 256, 191, 144, and 131 GC-related genes, respectively. There were 49 targets with degree values higher than 6.57, the mean degree of the predicted targets. The TP53, hTERT, vascular endothelial growth factor A (VEGFA), caspase-3 (CASP3), murine double minute 2 (MDM2), matrix metalloproteinase 2 (MMP2), and apoptosis regulator Bcl-2 (BCL2) genes were targeted by 26, 19, 16, 16, 14, 14, and 12 compounds respectively, which indicated they may be involved in the underlying mechanisms of the preferred prescription.
GO and pathway enrichment analysis of the preferred prescription
To explore the potential mechanism of the preferred prescription, we utilized the DAVID database to decipher the information related to gene ontology. The GO analysis on the targets of the preferred prescription is shown in Figure 4A. The significant biological processes (BP) (P<0.05) included apoptotic process (GO: 0006915), cell adhesion (GO: 0007155), cell cycle arrest (GO: 0007050), and signal transduction (GO: 0007165). The significant molecular functions (MF) (P<0.05) included protein kinase activity (GO: 0004672), cadherin binding involved in cell-cell adhesion (GO:0098641), enzyme binding (GO:0019899), and ubiquitin-protein ligase binding (GO:0031625). The significant cellular components (CC) (P<0.05) included nucleus (GO:0005634), cytoplasm (GO:0005737), plasma membrane (GO:0005886), and cytosol (GO:0005829).
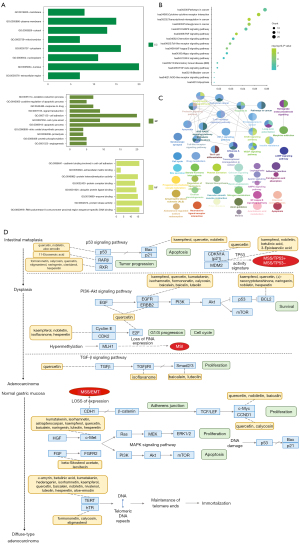
The significant KEGG pathways were mainly the pathways in cancer, T cell receptor signaling pathway, Toll-like receptor signaling pathway, apoptosis, and the VEGF signaling pathway (Figure 4B). Cross-talk pathways network of the preferred prescription is shown in Figure 4C. Moreover, a multi-regulation map of KEGG pathways was demonstrated, indicating the preferred prescription may exert inhibition on both tumorigenesis and progression of GC (Figure 4D).
Modular characteristics and molecular mechanism of the preferred prescription for GC treatment
To elucidate modular characteristics of the preferred prescription, we summarized the significantly enriched BP, KEGG signaling, and reactome pathway of the decoction, which was mainly distributed in the modules of immune regulation, epithelial-mesenchymal transition (EMT), and cell apoptosis/cell cycle (Table 3). Then, an herb-crucial compound-biological functional module-molecule network was constructed to determine the relationships among these elements (Figure 5A). For example, Atractylodis Macrocephalae Rhizoma, a core herb in different combinational patterns, is known to invigorate the Spleen and tonify the vital-Qi, which predominantly regulates the immune module. Astragali Radix, Pinelliae Rhizoma, Citri Reticulatae Pericarpium, Amomi Fructus, Hordei Fructus Germinatus, and Aurantii Fructus are known to regulate the movement of Qi, promote blood circulation, and disperse blood stasis, which mainly regulate the EMT module. In addition, GeneMANIA was used to analyze the interactions among the significant targets, which were enriched in the pathways of each functional biological module. The results indicated that the preferred prescription has the exact substance basis to regulate the biological modules related to the pathophysiology of GC.
Table 3
Module | Type | GO biological process/KEGG signaling pathway/reactome pathway | P value (Benjamini adjusted) |
---|---|---|---|
Immune | Reactome | Immune system | 6.18E-05 |
KEGG | T cell receptor signaling pathway | 5.71E-09 | |
KEGG | Toll-like receptor signaling pathway | 8.98E-08 | |
KEGG | NOD-like receptor signaling pathway | 2.66E-04 | |
Cell apoptosis/cell cycle | KEGG | Apoptosis | 1.35E-06 |
KEGG | Cell cycle | 9.49121E-08 | |
Epithelial mesenchymal transition | GO | Angiogenesis | 0.000915735 |
Reactome | Extracellular matrix organization | 3.78222E-05 | |
KEGG | Focal adhesion | 4.85619E-08 | |
KEGG | Wnt signaling pathway | 0.005181823 |
GO, Gene Ontology; KEGG, Kyoto Encyclopedia of Genes and Genomes; NOD, nucleotide-binding and oligomerization domain.
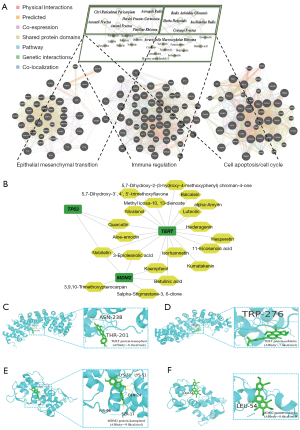
To confirm the molecular mechanism underlying the preferred prescription, molecular docking was performed. Based on the sub-network between the crucial substances and predominant targets of the preferred prescription (Figure 5B), we found that both nobiletin and kaempferol have strong affinity with TERT and MDM2 molecules, and p53 may function as a downstream target (Figure 5C-5F).
The preferred prescription suppressed GC proliferation and induced cell apoptosis
The typical HPLC-DAD chromatogram of all 12 major components in the preferred prescription is shown in Figure 6. The calycosin 7-O-glucoside, rutin, narirutin, naringin, hesperidin, neohesperidin, calycosin, naringenin, kaempferol, formononetin, nobiletin and atractylenolide II contents in the decoction were determined as 0.010, 0.014, 0.229, 0.214, 0.253, 0.239, 0.002, 0.002, 0.004, 0.001, 0.003, and 0.002 mg/g, respectively.
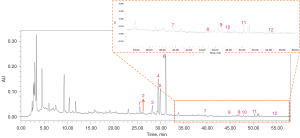
To determine the effect of the preferred prescription on GC cells, the AGS, HGC27, MKN28, and SGC7901 cell activities were assessed by MTT assay. As shown in Figure 7A, the cellular viabilities of the 4 GC cell lines were all significantly inhibited. In nude mouse xenograft models, we further validated that the preferred prescription-treated mice showed dramatically decreased tumor weights compared to the control (Figure 7B,7C). Notably, body mass did not change over the preferred prescription treatment time courses and mice appeared healthy over the duration of the experiments, suggesting that no significant adverse side-effects were experienced (Figure 7D, Figure S1). These results suggested that the preferred prescription treatment suppressed GC proliferation in vivo and in vitro.
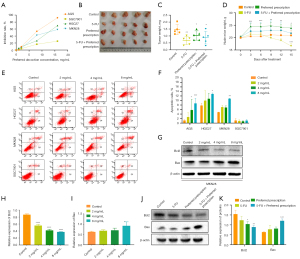
Inducing the apoptosis of cancer cells is a vital way for anticancer drugs to take effect. Therefore, the GC cells were treated with different concentrations of the preferred prescription, and Annexin V-positive cells were detected by FITC analysis to evaluate whether the preferred prescription could induce apoptotic cell death. As shown in Figure 7E,7F, the preferred prescription significantly and dose-dependently increased the apoptosis rates of GC cells compared to the control. We also detected that the preferred prescription significantly increased the expression of pro-apoptotic protein Bax and decreased the expression of antiapoptotic Bcl2 protein in a concentration-dependent manner (Figure 7G-7I). Similar results were found in vivo (Figure 7J,7K). Taken together, our findings indicated that the preferred prescription inhibited the growth of GC by inducing apoptosis both in vivo and in vitro.
The preferred prescription induced GC cell cycle arrest via hTERT/MDM2-p53 signaling pathway
From the results of KEGG pathway enrichment and the sub-network among the crucial substances and the predominant targets, the effects of the preferred prescription were evaluated on the hTERT/MDM2-p53 signaling pathway. The activation of the p53 protein initiates a program of cell cycle arrest, cellular senescence, or apoptosis (34). During different phases of cell cycle, p53 controls both the G1 and G2/M checkpoints (35). Therefore, we performed flow cytometry assay to evaluate whether the preferred prescription modulated the cell cycle of GC cells. As shown in Figure 8A,8B, the proportion of MKN28 cells in the G1 phase was increased and the proportion of cells in the S phase was decreased, while there was a significant increment in G2/M in AGS, HGC-27, SGC-7901 cells.
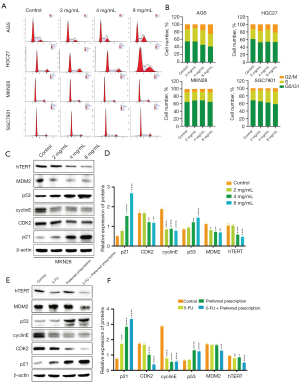
By binding to p53, MDM2 inactivates the suppressive function of the tumor in p53 and prevents it from intervening in the cell cycle (36). Cells lacking TERT possessed elevated p53 levels and transcriptional signatures were consistent with p53 up-regulation. Thus, we examined the lysates of the MKN28 cells treated with different concentrations of the preferred prescription using western blot assay. As shown in Figure 8C, the preferred prescription treatment significantly and dose-dependently decreased the expressions of hTERT and MDM2, and significantly increased the expression of p53. A major player in the p53-mediated G1 arrest is the p21 gene product that inhibits cyclin E-cdk2 (34). Therefore, we further detected the expressions of p21, cyclinE, and CDK2. It was found that the preferred prescription significantly increased the expression ratio of p21 and reduced the ratios of cyclinE and CDK2 in MKN28 cells (Figure 8C,8D). Similar results were verified in nude mouse xenograft models (Figure 8E,8F). The above findings implied that the preferred prescription induced cell cycle arrest in GC cells via hTERT/MDM2-p53 signaling pathway. It also supported the causal link between the elevated p53 by the preferred prescription and the induction of pro-apoptosis proteins of Bax, and the depletion of anti-apoptosis proteins Bcl-2.
The preferred prescription inhibited EMT of GC cells via the hTERT/MDM2-p53 signaling pathway
In cancer, EMT is associated with tumor initiation, invasion, metastasis, and resistance to therapy (37). The role of p53 in EMT has been well studied (38). Recently, it has been reported that p53, p21, and MDM2 bind to the EMT-inducing transcriptional factors Snail/Slug, and promote its ubiquitin-mediated proteasomal degradation (39,40). Based on these existing studies and our findings above, we hypothesized that the preferred prescription also exerted inhibition on EMT of GC via the hTERT/MDM2-p53 signaling pathway. We performed wound healing assay, which revealed that the preferred prescription remarkably suppressed the migration of MKN28 cells in a concentration-dependent manner, and notably the wound healing area of the preferred prescription (8 mg/mL) group was still large after culturing for 48 h (Figure 9A,9B). Transwell assay revealed that the preferred prescription dramatically inhibited GC invasion, even at the lowest concentration of 2 mg/mL (Figure 9C,9D). Further, we examined the EMT-related markers, and found that after the preferred prescription treatment, N-cadherin, Snail, and Slug expression ratios were significantly reduced in MKN28 GC cells (Figure 9E,9F) and in vivo (Figure 9G,9H) compared to the controls. Collectively, these data suggest that the preferred prescription may suppress EMT of GC via the hTERT/MDM2-p53 signaling pathway.
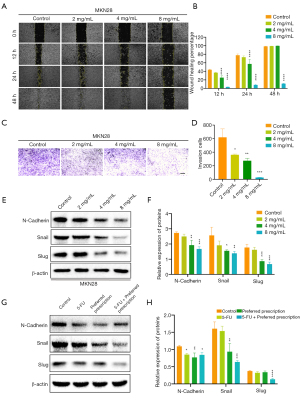
Discussion
Globally, GC is the most common cancer and is the leading cause of cancer deaths (1). Therefore, the discovery of novel therapeutic strategies is urgent to enhance the therapeutic effects of existing drugs. Recently, with the development of bioinformatics and network pharmacology, more researchers have applied these methods to unravel the therapeutic effects of TCM formulae (4,41). Elaborately prescribed herbal formulae are being increasingly beneficial for GC patients in relieving adverse events caused by CT or CRT, expediting postoperative recovery, and reducing recurrence or metastasis incidence. However, the modular functional characteristics and molecular mechanisms of TCM in ameliorating GC have remained unclear. In the current study, we conducted a comprehensive data mining of clinical prescriptions, based on which, we obtained a basic TCM prescription with general applicability for GC treatment via machine learning. Based on network pharmacology exploration, the pharmacological mechanism of this preferred prescription against GC was also clarified via experimental verification.
In this study, all the TCM prescriptions for GC treatment from eligible clinical studies over the past 3 decades were collected via CNKI, which is a predominant academic database containing the most comprehensive and authoritative information on TCM. A total of 194 prescriptions were retrieved, among which the most common used couplet herb pairs and triplet herbal combinations were analyzed through ARM. The top 3 recommended herb pairs were all led by Atractylodis Macrocephalae Rhizoma. As one of the most potent herbs to invigorate the Spleen and tonify the Qi, it is especially indicated for poor appetite, loose stools, and diarrhea, which are the common manifestations in GC patients, especially after postoperative CT or CRT. When paired with Dioscoreae Rhizoma, with the potential to nourish the Yin and tonify the Lung and Kidney, it improves appetite, and mitigates loose stools. When paired with Aucklandiae Radix, it promotes digestion and relieves pain. Codonopsis Radix can be used with Atractylodis Mcacrocephalae Rhizoma in GC patients with weak physique and lassitude, or those experiencing cold pain in the stomach and abdomen, vomiting, or diarrhea (8). In addition, we found that Poria-Pseudostellariae Radix-Atractylodis Mcacrocephalae Rhizoma was one of the most recommended triplet herbal combinations. Notably, they are the major compositions of Si Jun Zi decoction, a classic traditional Chinese herbal prescription, which is well known for treating digestive function disorders (42). A deep machine learning method-based cluster analysis was further conducted to obtain an optimized prescription. In this preferred prescription, Atractylodis Macrocephalae Rhizoma and Astragali Radix were found to be major herbs responsible for tonifying the Middle-Jiao and invigorating the Spleen, which could relieve fatigue, poor appetite, loose stools, and other symptoms in GC patients. Pinelliae Rhizoma, Citri Reticulatae Pericarpium, Aucklandiae Radix, Amomi Fructus, and Aurantii Fructus worked cooperatively to alleviate symptoms like fullness in the abdomen, belching, nausea, and vomiting in GC patients with dampness stagnation. Dyspepsia is very common among GC patients, especially after surgery. Thus, Galli Gigeriae Endothelium Corneum, Hordei Fructus Germinatus, and Crataegi Fructus in the preferred prescription were used to improve digestive dysfunction. For patients enduring a long course of the disease, Herba Hedyotidis and Radix Actinidiae Chinensis could help in the elimination of internal toxins. Generally, the treatment principles and clinical indications of the preferred prescription are summarized in Figure S2.
Next, we identified the bioactive components and the potential molecule targets of the preferred prescription by network pharmacology. A total of 74 bioactive components were acquired from literature and various public databases, 2,128 genes relevant to the preferred prescription were obtained via target prediction, and 429 GC-related targets were retrieved from disease databases. Eventually, 135 overlapping genes were identified as disease-associated targets. The disease of GC is heterogeneous, whereby the presence of molecular heterogeneity has been described based on anatomic histopathology, the anatomic site, gene expression, and so on (43). The multi-compound and multi-target characteristics of the preferred prescription potentiate its multiple biological functions in treating GC. To acquire an in-depth understanding of the overlapping targets, GO, KEGG, and reactome pathway enrichment were performed. The modular functional network revealed part of the combinational rules of herbs in the context of biological functional molecules (Figure 5A). In this study, the crucial compounds ranked by degree were quercetin, kaempferol, baicalein, nobiletin, and luteolin. Existing studies on these bioactive substances have shown diverse anti-GC mechanisms. For example, luteolin could shift the Bax/Bcl ratio in human GC cells by increasing the expressions of pro-apoptotic proteins (44-46). Treatment with luteolin was also observed to up-regulate p21/cip1 (CDKN1A), a TP53 activity signature (47). The mixture of Radix Actinidiae Chinensis could down-regulate the expressions of stromal cell-derived factor-1 (SDF-1), MMP-2, and MMP-9 in SGC-7901 cells (48). Quercetin was found to restrain transforming growth factor (TGF)-β1-induced EMT by inhibiting Twist1 and regulating E-cadherin expression (49). Also, quercetin-3-methyl ether (Q3ME) is a natural flavonoid compound capable of inhibiting esophageal carcinogenesis by targeting the receptor tyrosine kinases (RTKs) (50). Although there was no literature on some crucial components associated with GC, the efficacy was noteworthy in other cancers. All literature, together with the experimental studies, provided a valuable hint in identifying the action mechanism of the preferred prescription against GC.
From the in vivo results, it was revealed that treatment with the preferred prescription significantly suppressed tumor growth compared to the control. Notably, the preferred prescription did not compromise the mice’s body weights compared to the 5-FU group, indicating that it has a better safety profile, or at least in part, is favorable for patients who are intolerant of 5-FU treatment. To explore the potential mechanism, multiple biological function assays were conducted in vitro in GC cell lines, including AGS, HGC27, MKN28, and SGC-7901. It was demonstrated that the preferred prescription promoted cellular apoptosis and attenuated the metastatic capability in GC cells.
From the results of target prediction and pathway analysis, the preferred prescription might suppress the survival and metastasis of GC cells via the hTERT/MDM2-p53 signaling pathway. The TERT protein is often overexpressed in tumor cells and mediates cellular immortalization (51). Recent research revealed that cells lacking TERT possessed elevated p53 levels and transcriptional signatures were consistent with p53 up-regulation. The up-regulation of the MDM2 oncogene plays a role in the diffuse type of GC (52). By binding to p53, MDM2 inactivates the anti-tumor function of p53 and prevents it from intervening in the cell cycle (36). The activation of p53 induces p53-dependent cell death and p53- and p21-dependent cell cycle arrests, which is characterized by depletion of the S-phase cells and accumulation at the G1/S and/or G2/M phase boundaries of the cell cycle (53). In the present study, the flow cytometry and western blot results supported the prediction. After treatment with the preferred prescription, the proportion of MKN28 cells in the G1 phase was increased and the proportion of cells in the S phase was decreased, while there was a significant increment in G2/M in AGS, HGC-27, and SGC-7901 cells. Additionally, we validated that the preferred prescription exerted negative modulation on the expressions of hTERT and MDM2, and positively modulated the expressions of p53 and p21. The activation of p53 stimulates the synthesis of the p21 protein, which inhibits cyclin E-cdk2 activity, and this in turn acts upon the retinoblastoma (Rb)-MDM2 complex that promotes p53 activity and apoptosis (34). In this study, we demonstrated that after the preferred prescription treatment, increased p53 activity induced the pro-apoptosis protein Bax and depleted the anti-apoptosis protein Bcl-2. We also detected decreased expressions of Slug and Snail under the preferred prescription treatment, which was possibly due to p53, p21, and MDM2 interacting with the EMT-inducing transcriptional factors, and leading to their ubiquitination (39). Taken together, the preferred prescription might play a role in inducing cell cycle arrest, cellular apoptosis, and inhibiting EMT process of GC via the hTERT/MDM2-p53 signaling pathway.
Meanwhile, our research had several limitations. Firstly, the eligible literature in our study was drawn only from Chinese databases. With the development of TCM, we will be able to include more information from other Asian countries like Japan and South Korea. Secondly, the bioactive substances of the Chinese herbs screened in the existing databases need further preclinical and clinical verification. Lastly, a clinical trial on the preferred prescription is required to reliably assess the roles of TCM in the recurrence and metastasis of GC.
Conclusions
To conclude, data mining and machine learning combined with network pharmacology analysis and experimental verification may elucidate the modular functions and pharmacological mechanisms of TCM on GC from an innovative perspective. It was demonstrated that the preferred prescription may suppress the survival and metastasis of GC cells via modulating the hTERT/MDM2-p53 signaling pathway. Meanwhile, in-depth pharmacological mechanisms by which the preferred prescription ameliorates GC need to be further explored. Also, as the core concept of TCM, syndrome differentiation cannot be completely replaced by the results of machine learning. Hence, clinically effective combinations of herbs should also be encouraged as individualized strategies for GC patients. This study will facilitate the application of TCM in GC treatment with the purpose of improving therapeutic strategy in clinic.
Acknowledgments
Funding: This study was supported by the National Natural Science Foundation of China (81973609, 81704031, 81973782); Jiangsu Provincial Medical Youth Talent (QNRC2016641); Jiangsu Provincial Hospital of Traditional Chinese Medicine Academic Talent Program (Y2018RC33); the Open Projects of the Discipline of Chinese Medicine of Nanjing University of Chinese Medicine Supported by the Subject of Academic priority discipline of Jiangsu Higher Education Institutions (ZYX03KF019, ZYX03KF021, ZYX03KF029); Jiangsu Province Scientific Research and Practice Innovation Program (SJCX21_0738, SJCX20_0568).
Footnote
Reporting Checklist: The authors have completed the ARRIVE reporting checklist. Available at https://dx.doi.org/10.21037/atm-21-6301
Data Sharing Statement: Available at https://dx.doi.org/10.21037/atm-21-6301
Conflicts of Interest: All authors have completed the ICMJE uniform disclosure form (available at https://dx.doi.org/10.21037/atm-21-6301). The authors have no conflicts of interest to declare.
Ethical Statement: The authors are accountable for all aspects of the work in ensuring that questions related to the accuracy or integrity of any part of the work are appropriately investigated and resolved. This study was conducted in accordance with the Declaration of Helsinki (as revised in 2013). Animal experiment was performed under a project license (No. 2021DW-35-01) granted by the Animal Ethics Committee of Affiliated Hospital of Nanjing University of Chinese Medicine (Nanjing, China), in compliance with the recommendations in the Guide for the Care and Use of Laboratory Animals of the National Institutes of Health.
Open Access Statement: This is an Open Access article distributed in accordance with the Creative Commons Attribution-NonCommercial-NoDerivs 4.0 International License (CC BY-NC-ND 4.0), which permits the non-commercial replication and distribution of the article with the strict proviso that no changes or edits are made and the original work is properly cited (including links to both the formal publication through the relevant DOI and the license). See: https://creativecommons.org/licenses/by-nc-nd/4.0/.
References
- Jemal A, Bray F, Center MM, et al. Global cancer statistics. CA Cancer J Clin 2011;61:69-90. [Crossref] [PubMed]
- Guo S, Shang MY, Dong Z, et al. A nomogram for predicting cancer-specific survival in different age groups for operable gastric cancer: a population-based study. Transl Cancer Res 2020;9:2758-68. [Crossref]
- Zhao Y, Wang XW, Lu Y, et al. Effect of Xiaotan Sanjie Decoction treatment based on syndrome differentiation on quality of life of patients with intermediate and advanced gastric cancer. Ti Erh Chun I Ta Hsueh Hsueh Pao 2016;37:1333-7.
- Pan B, Wang Y, Wu C, et al. A Mechanism of Action Study on Danggui Sini Decoction to Discover Its Therapeutic Effect on Gastric Cancer. Front Pharmacol 2020;11:592903. [Crossref] [PubMed]
- Catenacci DV, Chao J, Muro K, et al. Toward a Treatment Sequencing Strategy: A Systematic Review of Treatment Regimens in Advanced Gastric Cancer/Gastroesophageal Junction Adenocarcinoma. Oncologist 2021;26:e1704-29. [Crossref] [PubMed]
- Pharmacopoeia Committee of the People’s Republic of China (Beijing). Chinese Pharmacopoeia. 2020 ed. Beijing: Chinese Medical Science and Technology Press, 2020.
- Liu P, Liu S, Chen G, et al. Understanding channel tropism in traditional Chinese medicine in the context of systems biology. Front Med 2013;7:277-9. [Crossref] [PubMed]
- Gao XM. Chinese Pharmacy. 8th ed. Beijing: China Traditional Chinese Medicine Publishing Press, 2007.
- Creighton C, Hanash S. Mining gene expression databases for association rules. Bioinformatics 2003;19:79-86. [Crossref] [PubMed]
- Agrawal R, Tomasz, Arun S. Mining association rules between sets of items in large databases. Acm Sigmod Conference on Management of Data 1993;22:207-15.
- Vougas K, Sakellaropoulos T, Kotsinas A, et al. Machine learning and data mining frameworks for predicting drug response in cancer: An overview and a novel in silico screening process based on association rule mining. Pharmacol Ther 2019;203:107395. [Crossref] [PubMed]
- Leem J, Jung W, Kim Y, et al. Exploring the combination and modular characteristics of herbs for alopecia treatment in traditional Chinese medicine: an association rule mining and network analysis study. BMC Complement Altern Med 2018;18:204. [Crossref] [PubMed]
- Karim MR, Beyan O, Zappa A, et al. Deep learning-based clustering approaches for bioinformatics. Brief Bioinform 2021;22:393-415. [Crossref] [PubMed]
- Jiang F, Jiang Y, Zhi H, et al. Artificial intelligence in healthcare: past, present and future. Stroke Vasc Neurol 2017;2:230-43. [Crossref] [PubMed]
- Ru J, Li P, Wang J, et al. TCMSP: a database of systems pharmacology for drug discovery from herbal medicines. J Cheminform 2014;6:13. [Crossref] [PubMed]
- Liu Z, Guo F, Wang Y, et al. BATMAN-TCM: a Bioinformatics Analysis Tool for Molecular mechANism of Traditional Chinese Medicine. Sci Rep 2016;6:21146. [Crossref] [PubMed]
- Su X, Kong L, Lei X, et al. Biological fingerprinting analysis of traditional Chinese medicines with targeting ADME/Tox property for screening of bioactive compounds by chromatographic and MS methods. Mini Rev Med Chem 2007;7:87-98. [Crossref] [PubMed]
- Gfeller D, Michielin O, Zoete V. Shaping the interaction landscape of bioactive molecules. Bioinformatics 2013;29:3073-9. [Crossref] [PubMed]
- Yu H, Chen J, Xu X, et al. A systematic prediction of multiple drug-target interactions from chemical, genomic, and pharmacological data. PLoS One 2012;7:e37608. [Crossref] [PubMed]
- Zheng C, Guo Z, Huang C, et al. Large-scale Direct Targeting for Drug Repositioning and Discovery. Sci Rep 2015;5:11970. [Crossref] [PubMed]
- Li J, Zhao P, Li Y, et al. Systems pharmacology-based dissection of mechanisms of Chinese medicinal formula Bufei Yishen as an effective treatment for chronic obstructive pulmonary disease. Sci Rep 2015;5:15290. [Crossref] [PubMed]
- Szklarczyk D, Santos A, von Mering C, et al. STITCH 5: augmenting protein-chemical interaction networks with tissue and affinity data. Nucleic Acids Res 2016;44:D380-4. [Crossref] [PubMed]
- Keiser MJ, Roth BL, Armbruster BN, et al. Relating protein pharmacology by ligand chemistry. Nat Biotechnol 2007;25:197-206. [Crossref] [PubMed]
- Daina A, Michielin O, Zoete V. SwissTargetPrediction: updated data and new features for efficient prediction of protein targets of small molecules. Nucleic Acids Res 2019;47:W357-64. [Crossref] [PubMed]
- Rappaport N, Twik M, Plaschkes I, et al. MalaCards: an amalgamated human disease compendium with diverse clinical and genetic annotation and structured search. Nucleic Acids Res 2017;45:D877-87. [Crossref] [PubMed]
- Piñero J, Bravo À, Queralt-Rosinach N, et al. DisGeNET: a comprehensive platform integrating information on human disease-associated genes and variants. Nucleic Acids Res 2017;45:D833-9. [Crossref] [PubMed]
- Dennis G Jr, Sherman BT, Hosack DA, et al. DAVID: Database for Annotation, Visualization, and Integrated Discovery. Genome Biol 2003;4:3. [Crossref] [PubMed]
- Kanehisa M, Goto S. KEGG: kyoto encyclopedia of genes and genomes. Nucleic Acids Res 2000;28:27-30. [Crossref] [PubMed]
- Bindea G, Mlecnik B, Hackl H, et al. ClueGO: a Cytoscape plug-in to decipher functionally grouped gene ontology and pathway annotation networks. Bioinformatics 2009;25:1091-3. [Crossref] [PubMed]
- Warde-Farley D, Donaldson SL, Comes O, et al. The GeneMANIA prediction server: biological network integration for gene prioritization and predicting gene function. Nucleic Acids Res 2010;38:W214-20. [Crossref] [PubMed]
- Morris GM, Huey R, Lindstrom W, et al. AutoDock4 and AutoDockTools4: Automated docking with selective receptor flexibility. J Comput Chem 2009;30:2785-91. [Crossref] [PubMed]
- O'Boyle NM, Banck M, James CA, et al. Open Babel: An open chemical toolbox. J Cheminform 2011;3:33. [Crossref] [PubMed]
- Moher D, Liberati A, Tetzlaff J, et al. Preferred reporting items for systematic reviews and meta-analyses: the PRISMA statement. PLoS Med 2009;6:e1000097. [Crossref] [PubMed]
- Wu D, Prives C. Relevance of the p53-MDM2 axis to aging. Cell Death Differ 2018;25:169-79. [Crossref] [PubMed]
- Agarwal ML, Agarwal A, Taylor WR, et al. p53 controls both the G2/M and the G1 cell cycle checkpoints and mediates reversible growth arrest in human fibroblasts. Proc Natl Acad Sci U S A 1995;92:8493-7. [Crossref] [PubMed]
- Momand J, Zambetti GP, Olson DC, et al. The mdm-2 oncogene product forms a complex with the p53 protein and inhibits p53-mediated transactivation. Cell 1992;69:1237-45. [Crossref] [PubMed]
- Pastushenko I, Blanpain C. EMT Transition States during Tumor Progression and Metastasis. Trends Cell Biol 2019;29:212-26. [Crossref] [PubMed]
- Powell E, Piwnica-Worms D, Piwnica-Worms H. Contribution of p53 to metastasis. Cancer Discov 2014;4:405-14. [Crossref] [PubMed]
- Wang SP, Wang WL, Chang YL, et al. p53 controls cancer cell invasion by inducing the MDM2-mediated degradation of Slug. Nat Cell Biol 2009;11:694-704. [Crossref] [PubMed]
- Kim J, Bae S, An S, et al. Cooperative actions of p21WAF1 and p53 induce Slug protein degradation and suppress cell invasion. EMBO Rep 2014;15:1062-8. [Crossref] [PubMed]
- Wang T, Feng Y, Wang H, et al. The Mechanisms of Sijunzi Decoction in the Treatment of Chronic Gastritis Revealed by Network Pharmacology. Evid Based Complement Alternat Med 2020;2020:8850259. [Crossref] [PubMed]
- Liu L, Han L, Wong DY, et al. Effects of Si-Jun-Zi decoction polysaccharides on cell migration and gene expression in wounded rat intestinal epithelial cells. Br J Nutr 2005;93:21-9. [Crossref] [PubMed]
- Cristescu R, Lee J, Nebozhyn M, et al. Molecular analysis of gastric cancer identifies subtypes associated with distinct clinical outcomes. Nat Med 2015;21:449-56. [Crossref] [PubMed]
- Lu J, Li G, He K, et al. Luteolin exerts a marked antitumor effect in cMet-overexpressing patient-derived tumor xenograft models of gastric cancer. J Transl Med 2015;13:42. [Crossref] [PubMed]
- Liu JF, Ma Y, Wang Y, et al. Reduction of lipid accumulation in HepG2 cells by luteolin is associated with activation of AMPK and mitigation of oxidative stress. Phytother Res 2011;25:588-96. [Crossref] [PubMed]
- Lee WJ, Wu LF, Chen WK, et al. Inhibitory effect of luteolin on hepatocyte growth factor/scatter factor-induced HepG2 cell invasion involving both MAPK/ERKs and PI3K-Akt pathways. Chem Biol Interact 2006;160:123-33. [Crossref] [PubMed]
- Imran M, Rauf A, Abu-Izneid T, et al. Luteolin, a flavonoid, as an anticancer agent: A review. Biomed Pharmacother 2019;112:108612. [Crossref] [PubMed]
- Zhang F, Zhang N, Liang F, et al. Effects of Tengligen Mixture on Expression of MMP-2,MMP-9 and SDF-1 in Gastric Cancer SGC-7901 Cells. Acta Chinese Medicine 2018;33:175-80.
- Feng J, Song D, Jiang S, et al. Quercetin restrains TGF-β1-induced epithelial-mesenchymal transition by inhibiting Twist1 and regulating E-cadherin expression. Biochem Biophys Res Commun 2018;498:132-8. [Crossref] [PubMed]
- Zhao S, Jiang Y, Zhao J, et al. Quercetin-3-methyl ether inhibits esophageal carcinogenesis by targeting the AKT/mTOR/p70S6K and MAPK pathways. Mol Carcinog 2018;57:1540-52. [Crossref] [PubMed]
- Konnikova L, Simeone MC, Kruger MM, et al. Signal transducer and activator of transcription 3 (STAT3) regulates human telomerase reverse transcriptase (hTERT) expression in human cancer and primary cells. Cancer Res 2005;65:6516-20. [Crossref] [PubMed]
- Günther T, Schneider-Stock R, Häckel C, et al. Mdm2 gene amplification in gastric cancer correlation with expression of Mdm2 protein and p53 alterations. Mod Pathol 2000;13:621-6. [Crossref] [PubMed]
- Shangary S, Wang S. Small-molecule inhibitors of the MDM2-p53 protein-protein interaction to reactivate p53 function: a novel approach for cancer therapy. Annu Rev Pharmacol Toxicol 2009;49:223-41. [Crossref] [PubMed]