Integrated transcriptomic and metabolomic analysis of rat serum to investigate potential target of puerarin in the treatment post-traumatic stress disorder
Introduction
Post-traumatic stress disorder (PTSD) is a serious and persistent disorder that occurs after individuals are exposed to traumatic experiences (war, natural disaster, injury or sexual assault, etc.) and experience tremendous fear, helplessness or fright (1). PTSD is a highly prevalent public health disease worldwide: the adult population with PTSD is around 3% and the lifetime prevalence is 1.9–8.8% in the whole world (2). It has been reported that PTSD correlates highly with other diseases, such as coronary heart disease and cancer, that severely affect the patients’ quality of life and impose a heavy burden on health systems (3,4). Effective treatments for PTSD are urgently required.
The pathogenesis of PTSD is complex and its development and phenotype may be affected by a variety of factors such as genetic vulnerability, epigenetics, nervous system, inflammation, and metabolism (5). Animal studies have shown that the brain regions associated with PTSD symptoms are involved in the formation and recovery of strong emotion and fear memories, such as the medial prefrontal cortex, anterior cingulate cortex, amygdala and hippocampus (6-8). It is reported that patients with PTSD have lesions in the nervous system, and magnetic resonance imaging (MRI) scans of the brain have revealed that the volume of the hippocampus and anterior cingulate cortex is reduced in patients compared with healthy subjects (9). Another study showed an increase in inflammatory markers in PTSD subjects who had experienced war, suggesting that PTSD may be associated with immune activation (10). Metabolomic analysis of PTSD patients revealed significant changes in various pathways, such as glycolysis, fatty acid uptake and metabolism, that are associated with mitochondrial lesions or dysfunction (11).
At present, the commonly used drugs for PTSD include selective serotonin reuptake inhibitors and benzodiazepines, which have side effects such as dependence and withdrawal reactions (12,13). In recent years, researchers have attempted to find drugs for PTSD from natural sources, such as cannabinoids; however, the use of cannabinoids leads to more severe mental illness with poor efficacy (14). Therefore, the development of new drugs with fewer side effects is critical and necessary for the treatment of PTSD. Puerarin is the main active ingredient extracted from pueraria root and its chemical structure is 7, 4-dihydroxy-8-C-glucosylisoflavone (molecular formula: C21H20O9), which has been widely used in clinical treatment of cardiovascular and cerebrovascular diseases, diabetes and its complications, osteonecrosis, Parkinson’s disease, Alzheimer’s disease, endometriosis, cancer, etc. (15). Our previous study revealed that puerarin could alleviate behavioral disorders of PTSD rats, regulate the levels of hypothalamic-pituitary-adrenal (HPA) axis hormones in the rats, and partially alleviate their PTSD symptoms (16). However, the specific mechanism of puerarin on alleviation of PTSD remains unclear.
Studies have demonstrated that part of the medicinal function of puerarin is through its metabolites. Luo et al. identified two glucuronic acid metabolites (puerarin-7-O-glucuronide and puerarin-4’-O-glucuronide) of puerarin in the blood, liver and urine of treated rats by liquid chromatography tandem mass spectrometry (LC-MS/MS), and explored the pharmacokinetics of puerarin in rats (17). However, the effects of puerarin on serum metabolomics in normal and PTSD rats have not been explored. Metabolomics is a newly developed science after genomics and proteomics to quantitatively describe endogenous metabolites and their responses to internal and external factors. For example, LC-MS/MS-based Metabolomics revealed that PE (18:1/0:0) and PC (18:1/0:0) were significantly different in peripheral blood of control subjects and PTSD patients, and could serve as potential biomarkers for PTSD (18). 1H NMR-based dynamic metabolomics revealed the protective effect of Shenfu injection on laparoscopic hysterectomy (19). Importantly, the transcriptomic and metabolomic multi-omics analysis have been widely used in exploring the pathogenesis of diseases and mechanisms of drug treatments (20). In the present study, transcriptomics and metabolomics were integrated to uncover the molecular mechanisms of puerarin’s efficacy in the treatment of PTSD from a systemic perspective. We present the following article in accordance with the ARRIVE reporting checklist (available at https://dx.doi.org/10.21037/atm-21-6009).
Methods
Animals
Eight-week-old male Sprague-Dawley rats (180–200 g, SPF) were obtained from the Laboratory Animal Center of Guangdong (Guangzhou, China, no. SCXK (Guangzhou) 2013-0002), and were housed under 12 h-light/dark cycle conditions with water and food freely available, and maintained at a constant humidity at 45–55% and temperature of 22–24 °C. Experiments were performed under a project license (No. NFYY-2021-0033) granted by institutional ethics board of Southern Medical University, in compliance with Southern Medical University guidelines for the care and use of animals.
Rat model of PTSD, drug treatment and sample collection
After 1 week for adaption, the rats were divided into three groups (Figure S1): normal control group (Control, n=6), PTSD group (n=6) and PTSD + puerarin group (PTSD + puerarin, n=6). The rats in PTSD groups and PTSD + puerarin groups were given a single prolonged stress (SPS) to construct the PTSD model according to the method described in our previous publication (16), and then were administrated puerarin (purity ≥95%, Sigma-Aldrich) or equal volume of normal saline (NS) by intragastric gavage once daily for a total of 10 days (from days 2 to 11 after SPS). The selected dose of puerarin (50 mg/kg) was based on our previous study (16). The rats in normal control groups were administered the equal volume of NS by intragastric gavage once per day for a total of 10 days, but were not treated with SPS. After 10 days of administration, blood samples were taken from the caudal vein. Some were placed in sterile non-anticoagulant tubes for serum collection by centrifugation and stored at −80 °C for metabolomic analysis. Total RNA was extracted from the remaining whole blood samples and stored at −80 °C for later transcriptome analysis and gene expression verification.
Metabolite analysis
Extraction of serum metabolites
Rats were killed humanely and blood was collected to isolate the serum, of which 100 µL was placed in the ethylene propylene (EP) tube, and 400 µL methanol (mass spectrometry grade) was added for vortex mixing. The supernatant was collected by centrifugation at 15,000×g and 4 °C for 10 min. A certain amount of supernatant was transferred to a new centrifuge tube and mass spectrometry-grade water was added to dilute the methanol to 53%. Subsequently, the supernatant was collected by centrifugation at 15,000×g and 4 °C for 10 min before LC-MS analysis. Quality control (QC) samples were pooled by taking equal volume samples from each experimental sample, and blank samples were 53% methanol aqueous solution containing 0.1% formic acid instead of the experimental samples. The pre-process of the QC and blank samples was the same as for the experimental samples.
Untargeted metabolomics analysis by UHPLC-MS/MS
The untargeted metabolomics was conducted by Novogene Bioinformatics Technology Co., Ltd (Beijing, China). The liquid chromatography-tandem mass spectrometry (LC-MS/MS) analyses were performed using a Vanquish ultra-high-performance liquid chromatography (UHPLC) system (Thermo Fisher) coupled with an Orbitrap Q Exactive series mass spectrometer (Thermo Fisher). Samples were injected onto a Hyperil Gold column (100 mm ×2.1 mm, 1.9 µm) using a 16-min linear gradient at a flow rate of 0.2 mL/min. The eluent A (0.1% formic acid) and eluent B (methanol) were the positive polarity mode eluents. The eluent A (5 mM ammonium acetate, pH 9.0) and eluent B (methanol) were the negative polarity mode eluents. The solvent gradient settings were as follows: 2% B, 1.5 min; 2–100% B, 12.0 min; 100% B, 14.0 min; 2–100% B, 14.1 min; 2% B, 17 min. The Q Exactive series mass spectrometer was performed in positive/negative polarity mode with a spray voltage of 3.2 kV, capillary temperature of 320 °C, sheath gas flow rate of 35 arb and aux gas flow rate of 10 arb.
Metabolite identification
The Compound Discoverer 3.1 (CD3.1, Thermo Fisher) was used to conduct the original data files obtained from UHPLC-MS/MS, and peak comparison, peak extraction and quantitation were performed for each metabolite. The main parameters settings were as follows: 0.2 min retention time tolerance, 5 ppm actual mass tolerance, 30% signal strength tolerance,3 signal-noise ratio, and 100,000 minimum strength. Subsequently, the total spectral intensity was normalized by the peak intensity, and then the molecular formula was predicted according to additive ions, molecular ion peaks and fragment ions. Next, peaks matching was performed against the mzCloud (https://www.mzcloud.org/), mzVault and MassList databases to gain accurate qualitative and relative quantitative results. Finally, statistical analyses were conducted by the statistical software R (R version R-3.4.3), Python (Python 2.7.6 version) and CentOS (CentOS release 6.6), but area normalization method is used for normal transformations when obtained data were not normally distributed.
Metabolomic data analysis
These metabolites were annotated using the Kyoto Encyclopedia of Genes and Genomes (KEGG) database (https://www.genome.jp/kegg/), HMDB database (https://www.hmdb.ca/) and Lipidmaps database (https://www.lipidmaps.org/).
For multivariate statistical analysis, principal components analysis (PCA) and partial least squares discriminant analysis (PLS-DA) were performed at metaX (a flexible and comprehensive software for processing metabolomic data). Variable Importance in the Projection (VIP) value of the first principal component of the PLS-DA model was calculated, which represented the contribution rate of metabolite to the differences in different groups. T-test was used to calculate the statistical significance (P value) for the univariate analysis. In addition, the fold change (FC) was calculated by the ratio of the mean of all biological repeated quantification values of each metabolite in pairwise groups. The metabolites with VIP >1, P<0.05 and FC ≥1.5 or ≤0.67 were considered as differentially expressed metabolites, which were plotted by volcano plots based on log2 (FC) and −log10 (P value) of the metabolites. For clustering heat maps, the data were normalized using z-scores of the intensity areas of the differential metabolites and plotted by P heatmap package in R language.
The functions of these metabolites were analyzed using the KEGG database. Metabolic pathway enrichment of differential metabolites was performed; when the ratio was satisfied by x/n > y/N, the metabolic pathway was considered as enriched; when the P value of the metabolic pathway was <0.05, the pathway was considered as having statistically significant enrichment.
Transcriptome analysis
RNA extraction, library construction, sequencing and RNA sequencing data analysis
The next-generation sequencing experiments were conducted by Novogene Bioinformatics Technology Co., Ltd (Beijing, China). Briefly, the total RNA from the rat blood samples was extracted by TRIzol (Thermo Scientific, USA), and the purity and integrity of the RNA were detected by agarose gel electrophoresis analysis. Next, the purity of the RNA (OD 260/280) was detected using Nanodrop (Thermo Scientific, USA), the RNA concentration was accurately quantified using Qubit 2.0 (Thermo Scientific, USA), and the integrity of RNA was accurately detected by Agilent 2100 (Agilent, USA). Further, a cDNA library was constructed using NEBNext1 Ultra™ RNA Library Prep Kit for Illumina (E7530, NEB, USA), and high-throughput sequencing was then performed using the HiSeq™ 2500 system (Illumina). Clean data were aligned to the rat reference genome by TopHat software and gene expression levels were assessed using fragments per kilobase of transcript per million mapped fragments (FPKM). The limma package in R was used to analyze the difference of expression matrix of the sequencing data. Differentially expressed genes were identified with the threshold of P<0.05 and |log2 FC| >0.5.
Functional enrichment analysis
The KEGG pathway enrichment and Gene Ontology datasets were used to analyze the differentially expressed genes with R package cluster Profiler, and terms with P<0.05 was considered to be statistically significant.
Identifying potential hub genes in puerarin’s action through protein-protein interaction (PPI) network analysis
PPI networks between the differentially expressed genes were performed by Search Tool for the Retrieval of Interacting Genes (STRING) database (https://string-db.org/) (21). Only these interactors with combined confidence score ≥0.4 were shown in the bitmap, and visualized with the Cytoscape software (Version 3.6.1) (22). Next, a plugin CytoHubba of the Cytoscape software (Version 3.6.1) was used to identify the hub genes in the PPI networds by calculating the degree of connectivity as previously reported (22,23). In accordance with node degree, the top 30 hub genes were visualized with the Cytoscape software (Version 3.6.1).
Validation of hub genes by RT-qPCR
Total RNA was extracted with TRIzol Reagent (Thermo Scientific, USA) according to the manufacturer’s instructions. The RNAs were reverse-transcribed into cDNA using PrimeScript™ RT reagent kit with gDNA Eraser (Takara, Dalian, China). Finally, RT-PCR was performed using TB Green® Fast qPCR Mix (Takara, Dalian, China) in an ABI 7500 Fast Real-Time PCR system (Applied Biosystems). GAPDH was used as an internal control for the mRNAs. RT-PCR was performed in triplicate, and the relative expression of each gene was calculated by the 2−ΔΔCt method. The primer sequences for RT-PCR are given in Table 1.
Table 1
Gene name | Sequencing (3'-5') | Product size (bp) |
---|---|---|
PKN2 | Forward primer: GTGGCACGAGATGAAGTAGACAGC | 144 |
Reverse primer: AGCAGCGTACTCCATCACAAAGC | ||
CD59 | Forward primer: GTTCCACAGGTGTTAGCCTCAGATG | 91 |
Reverse primer: GCATCCAGGTTAGGAGAGCAAGTG | ||
HBS1L | Forward primer: CGCCGTGCCTGATGACATACTG | 129 |
Reverse primer: CACACTGCTCGCTCACTCTTCTC | ||
CD36 | Forward primer: CGGCGATGAGAAAGCAGAAATGTTC | 91 |
Reverse primer: TCCAACACCAAGTAAGACCATCTCAAC | ||
PPP1CB | Forward primer: GCTGGTGGTATGATGAGTGTGGATG | 136 |
Reverse primer: GATTAGCTGTTCGAGGCGGAGTG | ||
DYNC1H1 | Forward primer: GCAGATGAGCAGTTTGGCATTTGG | 123 |
Reverse primer: AATCAGGAGTAGGCGGTGGATGG | ||
FUS | Forward primer: AGAACCAGTACAACAGCAGCAGTG | 114 |
Reverse primer: TCCAACACCAAGTAAGACCATCTCAAC | ||
BPTF | Forward primer: CCTGATTGCCATAACCACCACCAC | 124 |
Reverse primer: GGACTCATCGTATGGCGTCTTGC | ||
GAPDH | Forward primer: GTTGTCTCCTGCGACTTCA | 98 |
Reverse primer: GCCCCTCCTGTTATTATGG |
PKN2, Protein kinase N2; CD59, CD59 molecule; HBS1L, HBS1-like protein; CD36, CD36 molecule; Protein phosphatase 1 catalytic subunit beta; DYNC1H1, Dynein cytoplasmic 1 heavy chain 1; FUS, FUS RNA binding protein; BPTF, Bromodomain PHD finger transcription factor; GAPDH, Glyceraldehyde-3-phosphate dehydrogenase.
Hub genes verified in GEO dataset
The dataset of the mRNAs expression profile was collected from the GEO website (https://www.ncbi.nlm.nih.gov/geo/), and the accession number was GSE81761 (24). The dataset consisted of 66 samples, including 39 peripheral blood samples of patients with PTSD (PTSD group) and 27 peripheral blood samples of patients without PTSD (control). The limma package in R was used to select out differentially expressed genes between the PTSD and control groups. P<0.05 and |log2(FC)| >0 were considered as the threshold. The volcano plot of the mRNAs’ expression profiles was visualized using the ggplot2 package in the R software. In addition, the receiver operating characteristic (ROC) curves and the area under the ROC curve (AUC) were performed by calculating the sensitivity and specificity of expression levels to screen potential diagnostic and therapeutic targets in patients with PTSD.
Integrated pathway analysis
Integrated analysis of the transcriptome and metabolome was performed using the MetaboAnalysis 4.0 plugin of R. The official gene symbols of the mRNA and metabolites enriched KEGG IDs were input into the joint pathway analysis module for topological analysis.
Statistical analysis
All data are presented as mean ± SD. Statistical analysis and statistical graphing were conducted with GraphPad Prim 7.0. The statistical significance was assessed by Student’s t-test or one-way ANOVA for two comparisons and multiple comparisons, respectively. A P<0.05 was considered significant.
Results
Protective mechanism of puerarin against PTSD based on metabolomics
Metabolite profile analysis
The clinical and preclinical data have shown that metabolomic changes and metabolic dysregulation would highlight probable interrelated features of PTSD pathophysiology (25). Our previous study showed that puerarin could alleviate PTSD-like effects in a rat model of PTSD (16), but whether puerarin affects the serum metabolic expression profile of PTSD rats was unclear. In order to explore this, we used UHPLC-MS/MS to detect the serum metabolomic changes of PTSD rats treated with and without puerarin. First, the unsupervised PCA scores plot indicated good separation between the control group, PTSD group and puerarin-treated group in positive ion mode, in which the puerarin-treated group was well divide from the PTSD group and adjacent to the control group, showing that the metabolic disturbances in PTSD were markedly ameliorated with puerarin treatment (Figure 1A). In negative ion mode, the results were slightly worse than those in positive ion mode (Figure 1B). Supervised PLS-DA demonstrated that the separation between the control group and the PTSD groups was more significant in both positive ion and negative ion modes (Figure 1C,1D), for which R2=0.99, Q2=0.64 in positive ion mode and R2=0.99, Q2=0.33 in negative ion mode showed satisfactory modeling and predictive abilities. The supervised PLS-DA proofed the separation between the PTSD group and the puerarin-treated group was also remarkably distinguished (Figure 1E,1F), for which R2=1.00, Q2=0.93 in positive ion mode and R2=0.99, Q2=0.87 in negative ion mode showed satisfactory modeling and predictive abilities.
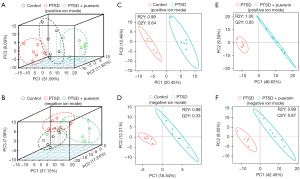
Identifying key metabolites in the treatment of PTSD by puerarin
With a threshold of P<0.05, |FC| >1.5 and VIP >1.0, a total of 63 differential metabolites were obtained from comparing the PTSD groups to the control group, 39 of which were upregulated and 24 downregulated [Figure 2A (left), Table S1, Table S2]. These differential metabolites were mainly enriched in nicotinate and nicotinamide metabolism, glutathione metabolism, protein digestion and absorption, and synthesis and degradation of ketone bodies (Figure S2A). Moreover, there were 153 differential metabolites in PTSD rats treated with puerarin compared with the PTSD rats, of which 44 were upregulated and 109 were downregulated [Figure 2A (right), Table S1, Table S2], and these differential metabolites were mainly enriched in biosynthesis of unsaturated fatty acids, nicotinate and nicotinamide metabolism, synthesis and degradation of ketone bodies, and protein digestion and absorption (Figure S2B). Further comprehensive analysis revealed 25 common metabolites between the PTSD groups vs. control group and the puerarin-treated group vs. PTSD group (Figure 2B). To better understand the expression pattern, the expression profiles of these potential metabolites were visualized by heatmap (Figure 2C). Exhilaratingly, it was noted that puerarin intervention restored the perturbation of 17 metabolites in PTSD, of which 13 upregulated and 4 downregulated metabolites in PTSD were reversed by puerarin treatment (Table 2), suggesting that these metabolites could serve as potential therapeutic targets in the treatment of PTSD by puerarin. Further, we used ROC analysis to evaluate whether these metabolites might be capable of distinguishing subjects with PTSD from those without PTSD, and the results indicated that the AUC of these metabolites ranged from 0.81 to 1.00. Among them, there were 11 metabolites with AUC >0.9 and the AUC of 3-succinoylpyridine (Com_1934_pos), 5-(2,5-dihydroxyhexyl) oxolan-2-one (Com_1761_pos), elaidic acid (Com_3_neg) and daidzein (Com_3159_pos) was 1.00 (Figure 3), suggesting that these four metabolites could be potential diagnostic targets for PTSD.
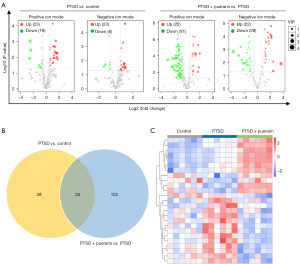
Table 2
ID | Name | PTSD vs. control | PTSD + pue vs. PTSD | |||||||
---|---|---|---|---|---|---|---|---|---|---|
log2FC | P value | VIP | Regulation | log2FC | P value | VIP | Regulation | |||
Com_6872_pos | 7α-Hydroxytestosterone | 1.77 | 1.29E-02 | 2.72 | Up | −1.43 | 4.23E-02 | 1.10 | Down | |
Com_3824_pos | N-(2-morpholinophenyl)-2-furamide | 1.71 | 5.18E-03 | 2.75 | Up | −1.98 | 2.27E-03 | 1.73 | Down | |
Com_571_pos | 3-phenyl-5-(trifluoromethyl)-4,5-dihydro-1H-pyrazol-5-ol | 1.67 | 9.85E-03 | 2.64 | Up | −2.35 | 1.28E-03 | 2.06 | Down | |
Com_2280_pos | 5-(6-hydroxy-6-methyloctyl)-2,5-dihydrofuran-2-one | 1.66 | 5.41E-03 | 2.76 | Up | −1.24 | 2.84E-02 | 1.22 | Down | |
Com_1934_pos | 3-Succinoylpyridine | 1.50 | 9.03E-04 | 2.68 | Up | −1.84 | 9.91E-07 | 1.73 | Down | |
Com_1891_pos | N-{2-[(2-furylmethyl)thio]ethyl}-2-[(3-methoxyphenyl)thio] acetamide | 1.44 | 5.24E-03 | 2.58 | Up | −1.63 | 1.47E-02 | 1.97 | Down | |
Com_1761_pos | 5-(2,5-dihydroxyhexyl) oxolan-2-one | 1.30 | 2.00E-03 | 2.12 | Up | −1.93 | 1.67E-04 | 1.74 | Down | |
Com_3836_neg | (±) -Abscisic acid | 1.06 | 1.55E-02 | 1.85 | Up | −1.26 | 5.33E-03 | 1.06 | Down | |
Com_6167_pos | N1-isopropyl-2-(1H-2-pyrrolylcarbonyl)-1-hydrazinecarboxamide | 0.99 | 2.39E-02 | 1.63 | Up | −2.20 | 2.19E-04 | 2.10 | Down | |
Com_5310_pos | Dl-Tropic acid | 0.72 | 3.19E-02 | 1.14 | Up | −1.70 | 1.80E-04 | 1.56 | Down | |
Com_8440_pos | 1-(2-furyl) pentane-1,4-dione | 0.64 | 3.33E-02 | 1.07 | Up | −1.33 | 3.44E-04 | 1.24 | Down | |
Com_3_neg | Elaidic acid | 0.54 | 5.59E-04 | 1.07 | Up | −1.14 | 4.02E-03 | 1.12 | Down | |
Com_1841_neg | FAHFA (22:5/22:3) | 0.51 | 4.93E-02 | 1.08 | Up | −1.44 | 1.00E-03 | 1.42 | Down | |
Com_1476_pos | DL-2-(acetylamino)-3-phenylpropanoic acid | −0.62 | 2.70E-02 | 1.03 | Down | 1.56 | 6.13E-05 | 1.51 | Up | |
Com_330_neg | L-Histidine | −1.17 | 1.83E-02 | 2.44 | Down | 1.23 | 1.00E-02 | 1.25 | Up | |
Com_3726_neg | N-(1,1-Dioxotetrahydro-1H-1λ6-thiophen-3-yl)-4-methoxybenzamide | −1.34 | 2.58E-02 | 2.29 | Down | 2.14 | 1.52E-05 | 2.05 | Up | |
Com_3159_pos | Daidzein | −2.57 | 1.17E-02 | 3.49 | Down | 2.32 | 2.51E-03 | 2.00 | Up |
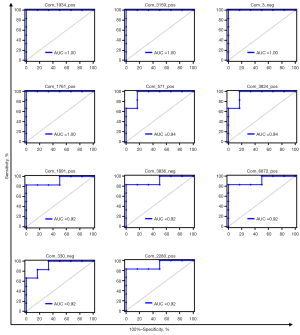
Protective mechanism of puerarin against PTSD based on transcriptomics
mRNA profile analysis
To further investigate the effect of puerarin on the mRNA expression profile of PTSD rats, we detected the mRNA changes of PTSD rats treated with or without puerarin by mRNA sequencing. The differentially expressed genes were obtained by limma package of R software with the threshold of P<0.05 and |log FC| >0.5. A total of 250 differentially expressed genes were identified by comparing the PTSD group to the control group, of which 210 were upregulated and 40 were downregulated [Figure 4A (left), https://cdn.amegroups.cn/static/public/atm-21-6009-1.pdf]. In addition, there were 1688 differentially expressed genes in the PTSD rats treated with puerarin compared with the PTSD rats, of which 683 were upregulated and 1,005 were downregulated [Figure 4A (right), Table S2]. A total of 126 overlapping differentially expressed genes were shared by the PTSD groups vs. control group and puerarin-treated group vs. PTSD group (Figure 4B), which were visualized in a heatmap (Figure 4C). Interestingly, 99 mRNAs with significant dysregulation in PTSD were reversed by puerarin treatment (Table 3), and these dysregulated genes were mainly enriched in the AMPK signaling pathway, hematopoietic cell lineage, PPAR signaling pathway, cellular senescence, and inflammatory mediator regulation of TRP channels (Figure 4D). Taken together, our data indicated that puerarin may improve the symptoms of PTSD in rats by regulating the mRNA expression profile, AMPK signaling pathway and PPAR signaling pathway, cellular senescence, and inflammatory mediator regulation of TRP channels.
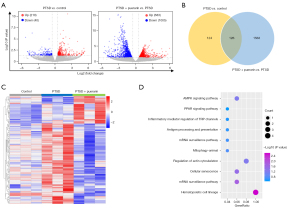
Table 3
DEG gene name | Full name | PTSD vs. control | PTSD + pue vs. PTSD | |||
---|---|---|---|---|---|---|
log2FC | P value | log2FC | P value | |||
Map2k3 | mitogen-activated protein kinase kinase 3 | 2.50 | 2.05E-02 | −3.11 | 1.14E-02 | |
LOC103689956 | LOC103689956 | 2.49 | 2.15E-02 | −3.16 | 1.00E-02 | |
Lgalsl | galectin like | 2.28 | 3.22E-11 | −1.55 | 1.47E-04 | |
Rplp2 | ribosomal protein lateral stalk subunit P2 | 2.03 | 8.87E-03 | −1.75 | 2.04E-03 | |
Gypc | glycophorin C | 1.97 | 3.83E-06 | −1.79 | 3.38E-03 | |
Ncoa4 | nuclear receptor coactivator 4 | 1.94 | 2.14E-02 | −1.56 | 2.78E-03 | |
LOC100911575 | LOC100911575 | 1.70 | 1.35E-04 | −1.46 | 8.76E-03 | |
Ret | ret proto-oncogene | 1.59 | 1.12E-04 | −1.42 | 9.90E-04 | |
Clic4 | chloride intracellular channel 4 | 1.32 | 4.35E-05 | −1.72 | 3.06E-05 | |
LOC103690020 | LOC103690020 | 1.27 | 1.85E-03 | −3.12 | 2.50E-08 | |
Als2cl | ALS2 C-terminal like | 1.25 | 8.08E-04 | −1.28 | 1.13E-02 | |
Spta1 | spectrin alpha | 1.16 | 3.83E-03 | −1.28 | 1.01E-03 | |
Ifit1bl | interferon-induced protein with tetratricopeptide repeats 1B-like | 1.15 | 2.11E-03 | −2.45 | 9.22E-08 | |
Cd36 | CD36 molecule | 1.14 | 3.32E-04 | −2.21 | 7.34E-07 | |
Cd226 | CD226 molecule | 1.09 | 1.37E-03 | −2.55 | 2.03E-08 | |
Tex2 | testis expressed 2 | 1.07 | 2.82E-03 | −1.56 | 5.80E-04 | |
Ell2 | elongation factor for RNA polymerase II 2 | 1.02 | 3.08E-03 | −1.78 | 3.60E-04 | |
Gng12 | G protein subunit gamma 12 | 1.02 | 2.63E-02 | −2.96 | 7.22E-08 | |
Hemgn | hemogen | 1.00 | 9.01E-07 | −1.01 | 1.38E-03 | |
Creg1 | cellular repressor of E1A stimulated genes 1 | 0.99 | 5.33E-04 | −1.48 | 2.88E-04 | |
Tacc3 | Transforming acidic coiled-coil containing protein 3 | 0.94 | 2.91E-02 | −2.09 | 1.14E-03 | |
Dpf3 | Double PHD fingers 3 | 0.93 | 2.02E-03 | −1.17 | 1.71E-02 | |
LOC100362738 | LOC100362738 | 0.92 | 2.84E-03 | −1.39 | 3.38E-05 | |
Mylk | Myosin light chain kinase | 0.92 | 5.18E-04 | −1.11 | 1.67E-03 | |
Kel | Kell metallo-endopeptidase | 0.91 | 5.85E-03 | −1.26 | 2.54E-03 | |
LIMS1 | LIM zinc finger domain containing 1 | 0.90 | 7.47E-03 | −1.04 | 1.41E-02 | |
KCNAB1 | Potassium voltage-gated channel subfamily A member regulatory beta subunit 1 | 0.90 | 2.22E-03 | −1.51 | 2.79E-04 | |
RGS18 | Regulator of G protein signaling 18 | 0.89 | 3.57E-02 | −3.66 | 7.64E-09 | |
RGD1359290 | LOC100362738 | 0.88 | 1.36E-04 | −2.32 | 3.16E-11 | |
PRAF2 | PRA1 domain family member 2 | 0.88 | 2.84E-03 | −0.94 | 3.79E-02 | |
USP15 | Ubiquitin specific peptidase 15 | 0.87 | 8.49E-03 | −2.09 | 5.47E-06 | |
PNPLA8 | Patatin like phospholipase domain containing 8 | 0.86 | 1.03E-02 | −1.55 | 3.77E-04 | |
TSC22D1 | TSC22 domain family member 1 | 0.84 | 5.55E-04 | −2.66 | 5.46E-15 | |
PPP1CB | Protein phosphatase 1 catalytic subunit beta | 0.82 | 4.73E-03 | −2.25 | 9.10E-08 | |
HMBS | Hydroxymethylbilane synthase | 0.82 | 7.32E-04 | −1.09 | 3.33E-03 | |
HBS1L | HBS1 like translational GTPase | 0.82 | 1.53E-02 | −1.12 | 2.49E-02 | |
MAST2 | Microtubule associated serine/threonine kinase 2 | 0.80 | 6.76E-03 | −1.26 | 1.87E-03 | |
RHAG | Rh associated glycoprotein | 0.80 | 3.24E-02 | −2.58 | 4.37E-07 | |
HIST1H1C | H1.2 linker histone, cluster member | 0.79 | 4.80E-03 | −0.93 | 3.51E-02 | |
PKN2 | Protein kinase N2 | 0.77 | 2.74E-02 | −1.29 | 5.81E-03 | |
USP46 | Ubiquitin specific peptidase 46 | 0.77 | 1.50E-02 | −1.96 | 4.21E-04 | |
CD9 | CD9 molecule | 0.77 | 5.42E-03 | −2.86 | 3.80E-10 | |
EXOC3 | Exocyst complex component 3 | 0.76 | 4.30E-02 | −1.46 | 1.85E-03 | |
GDA | Guanine deaminase | 0.76 | 2.40E-02 | −1.64 | 5.09E-04 | |
DYRK3 | Dual specificity tyrosine phosphorylation regulated kinase 3 | 0.76 | 2.03E-02 | −2.47 | 2.37E-08 | |
ZFAND5 | Zinc finger AN1-type containing 5 | 0.76 | 1.18E-02 | −1.95 | 9.09E-06 | |
UBXN2A | UBX domain protein 2A | 0.74 | 4.10E-02 | −4.57 | 1.69E-14 | |
CIR1 | Corepressor interacting with RBPJ | 0.74 | 1.93E-02 | −0.85 | 2.26E-02 | |
PPP1R13B | Protein phosphatase 1 regulatory subunit 13B | 0.74 | 3.31E-02 | −1.88 | 1.81E-04 | |
RTN4 | Reticulon 4 | 0.73 | 1.39E-02 | −1.68 | 5.27E-05 | |
Btrc | Beta-transducin repeat containing E3 ubiquitin protein ligase | 0.73 | 1.18E-02 | −1.49 | 1.05E-03 | |
Smg5 | SMG5 nonsense mediated mRNA decay factor | 0.73 | 6.54E-03 | −0.82 | 2.06E-02 | |
Tfdp2 | Transcription factor Dp-2 | 0.72 | 1.87E-02 | −2.61 | 5.10E-09 | |
Dennd5a | DENN domain containing 5A | 0.72 | 1.77E-02 | −1.04 | 1.52E-02 | |
Mmadhc | Metabolism of cobalamin associated D | 0.71 | 9.13E-03 | −1.12 | 8.99E-03 | |
Cep89 | Centrosomal protein 89 | 0.71 | 1.44E-02 | −1.35 | 7.12E-04 | |
Tinf2 | TERF1 interacting nuclear factor 2 | 0.71 | 3.23E-02 | −2.06 | 1.11E-05 | |
Ppm1a | Protein phosphatase, Mg2+/Mn2+ dependent 1A | 0.71 | 1.72E-02 | −1.23 | 1.33E-03 | |
RT1-T24-4 | RT1-T24-4 | 0.70 | 1.92E-02 | −1.00 | 3.65E-03 | |
LOC100361558 | LOC100361558 | 0.69 | 1.90E-02 | −1.65 | 4.47E-04 | |
Plcl2 | Phospholipase C like 2 | 0.69 | 1.37E-02 | −1.35 | 4.72E-04 | |
LPIN2 | Lipin 2 | 0.68 | 2.16E-02 | −1.36 | 1.07E-03 | |
Ranbp10 | RAN binding protein 10 | 0.67 | 7.74E-03 | −1.19 | 3.95E-03 | |
Tgfb1i1 | Transforming growth factor beta 1 induced transcript 1 | 0.67 | 3.76E-02 | −1.06 | 8.88E-03 | |
Smap1 | Small ArfGAP 1 | 0.66 | 3.69E-02 | −0.89 | 3.58E-02 | |
Bnip3l | BCL2 interacting protein 3 like | 0.65 | 1.24E-02 | −2.05 | 5.31E-08 | |
Arhgef37 | Rho guanine nucleotide exchange factor 37 | 0.64 | 2.40E-02 | −1.09 | 6.26E-03 | |
Fhdc1 | FH2 domain containing 1 | 0.64 | 2.81E-02 | −0.96 | 8.39E-03 | |
Usp4 | Ubiquitin specific peptidase 4 | 0.64 | 1.01E-02 | −1.40 | 8.12E-05 | |
Tmem140 | Transmembrane protein 140 | 0.63 | 3.18E-02 | −1.04 | 5.56E-03 | |
Caprin1 | Cell cycle associated protein 1 | 0.63 | 2.08E-02 | −0.71 | 4.94E-02 | |
Ppm1d | Protein phosphatase, Mg2+/Mn2+ dependent 1D | 0.63 | 2.72E-02 | −1.74 | 3.37E-06 | |
Sptb | Spectrin beta | 0.63 | 2.67E-02 | −0.89 | 1.33E-02 | |
Babam2 | BRISC and BRCA1 A complex member 2 | 0.63 | 1.01E-02 | −0.80 | 2.11E-02 | |
Mthfd2 | Methylenetetrahydrofolate dehydrogenase (NADP+ dependent) 2 | 0.63 | 4.24E-02 | −1.58 | 1.18E-04 | |
B2M | Beta-2-microglobulin | 0.62 | 9.47E-03 | −2.58 | 3.09E-15 | |
ANK1 | Ankyrin 1 | 0.61 | 3.02E-02 | −0.93 | 1.38E-02 | |
DCAF8 | DDB1 and CUL4 associated factor 8 | 0.60 | 4.89E-02 | −1.38 | 7.92E-04 | |
TAL1 | TAL bHLH transcription factor 1 | 0.60 | 1.91E-02 | −1.19 | 1.13E-03 | |
PTP4A2 | Protein tyrosine phosphatase 4A2 | 0.58 | 2.25E-02 | −1.33 | 1.22E-04 | |
TRIM10 | Tripartite motif containing 10 | 0.58 | 1.29E-02 | −1.93 | 8.30E-09 | |
ANKH | ANKH inorganic pyrophosphate transport regulator | 0.57 | 2.54E-02 | −0.75 | 3.92E-02 | |
CD59 | CD59 molecule | 0.56 | 2.33E-02 | −1.06 | 1.56E-02 | |
ITSN1 | Intersectin 1 | 0.55 | 2.51E-02 | −1.06 | 2.02E-03 | |
UBE2J1 | Ubiquitin conjugating enzyme E2 J1 | 0.55 | 3.68E-02 | −1.92 | 3.50E-07 | |
ELL | Elongation factor for RNA polymerase II | 0.55 | 1.45E-02 | −1.22 | 4.69E-04 | |
ITGA6 | Integrin subunit alpha 6 | 0.54 | 3.84E-02 | −0.81 | 3.73E-02 | |
STRADB | STE20 related adaptor beta | 0.52 | 4.81E-02 | −2.08 | 2.76E-07 | |
NARF | Nuclear prelamin A recognition factor | 0.50 | 4.52E-02 | −2.27 | 3.96E-10 | |
SH3GLB1 | SH3 domain containing GRB2 like | 0.50 | 3.02E-02 | −1.41 | 4.15E-05 | |
HUWE1 | HECT, UBA and WWE domain containing E3 ubiquitin protein ligase 1 | −0.57 | 4.09E-02 | 1.47 | 1.37E-05 | |
DYNC1H1 | Dynein cytoplasmic 1 heavy chain 1 | −0.59 | 3.09E-02 | 1.23 | 3.35E-04 | |
SCD2 | domain containing 2 | −0.61 | 2.13E-02 | 0.85 | 1.90E-02 | |
MADD | MAP kinase activating death domain | −0.62 | 3.51E-02 | 1.22 | 7.57E-04 | |
BPTF | Bromodomain PHD finger transcription factor | −0.65 | 2.94E-02 | 1.38 | 1.55E-04 | |
SETD2 | SET domain containing 2 | −0.68 | 4.32E-02 | 1.29 | 3.54E-04 | |
FUS | FUS RNA binding protein | −0.68 | 1.70E-02 | 1.05 | 8.27E-03 | |
KAT6A | Lysine acetyltransferase 6A | −0.70 | 1.77E-02 | 1.11 | 2.18E-03 | |
PPP1R14B | Protein phosphatase 1 regulatory inhibitor subunit 14B | −0.71 | 2.78E-02 | 0.93 | 2.24E-02 |
PTSD, post-traumatic stress disorder; FC, fold change.
Identifying key targets of puerarin in the treatment of PTSD
From the data from the STRING database analysis, the 99 mRNAs of significantly dysregulated genes were used to construct PPI networks, and we identified some hub genes among these significantly dysregulated mRNAs (Figure 5A). In accordance with node degree, the interactors of the top 30 hub genes were re-built using Cytoscape software (Figure 5B), and heatmaps showed the expression profiles of the top 30 hub genes in the control group, PTSD group, and puerarin-treated group (Figure 5C). Further, eight mRNAs among the top 30 hub genes were randomly selected for RT-PCR verification. The results showed that the expression levels of PKN2, CD36, CD59, HBS1L and PPP1CB were significantly increased and the expression levels of DYNC1H1, FUS and BPTF were markedly decreased in the peripheral blood of PTSD rats, and the dysregulation of these genes in PTSD was reversed by puerarin treatment (Figure 5D), which was consistent with the results of mRNA sequencing. Taken together, our data suggested that the eight hub genes may be potential targets for treating the development of PTSD in rats.
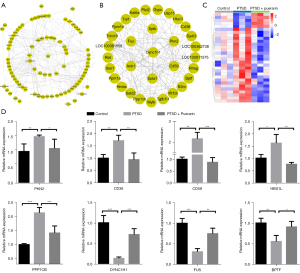
Verifying potential diagnostic and therapeutic targets for PTSD based on GEO datasets
Analysis of differentially expressed mRNAs in peripheral blood samples from patients with and without PTSD in the GSE81761 dataset was conducted using limma R software package (|log2FC| >0 and P<0.05). A total of 6,833 dysregulated mRNAs in peripheral blood samples from patients with PTSD were identified, of which 2,974 were significantly upregulated and 3,859 were markedly downregulated (Figure 6A). In order to further identify key genes in PTSD, the GSE81761 dataset was used to verify the expression levels of the eight genes (PKN2, CD36, CD59, HBS1L, PPP1CB, DYNC1H1, FUS and BPTF) identified above. The results indicated that the expression levels of CD59, HBS1L and CD36 were remarkably increased and the DYNC1H1 expression was markedly restrained in peripheral blood samples from patients with PTSD, compared with the peripheral blood samples from patients without PTSD (Figure 6B), while PKN2, SPTB, MADD and BPTF tended to be highly or lowly expressed in peripheral blood samples from patients with PTSD, but without significant difference (data not shown). Moreover, to evaluate whether specific genes might be capable of distinguishing patients with PTSD from patients without PTSD, we used ROC analysis for four different genes (CD59, HBS1L, DYNC1H1 and CD36) identified above. The results indicated that the AUC of CD59, HBS1L, CD36 and DYNC1H1 was 0.71, 0.70, 0.69 and 0.71, respectively (Figure 6C). To further evaluate whether the mRNA signatures date from supervised classification may enhance test sensitivity and specificity, we conducted the ROC combination analysis of the four selected genes (CD59, HBS1L, DYNC1H1 and CD36). The ROC analyses for all four genes as a single marker for PTSD showed extremely high diagnostic accuracy, with AUC of 0.79 (Figure 6D), indicating a significant amelioration compared with each single gene marker. Taken together, our results suggested that the four genes (CD59, HBS1L, DYNC1H1 and CD36) could act as potential diagnostic and therapeutic targets for PTSD.
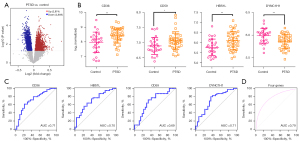
Integrated analysis of transcriptomics and metabolomics
To further explore the relationship between differentially expressed genes and metabolites, we performed joint pathway analysis for puerarin-regulated key genes and potential metabolic markers. As shown in Figure 7, the integrated analysis revealed that enriched pathways with a pathway impact >0.4 were antigen processing and presentation, synthesis and degradation of ketone bodies and one carbon pool by folate, which may be the main modulated pathways involved in the molecular mechanism of puerarin’s therapeutic effect in PTSD.
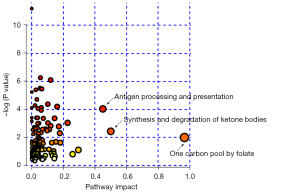
Discussion
Puerarin is an isoflavonoid extracted from Pueraria lobate, and has been reported to have antioxidant, anti-inflammatory, antidepressant-like, and protective effects in hippocampal injury (26,27). We also reported that puerarin could alleviate the behavioral disorders of PTSD rats (16). More importantly, compared with selective serotonin reuptake inhibitors and benzodiazepines, puerarin can go through the blood brain barrier into the central nervous system (CNS) (28). However, the underlying mechanisms have not been illuminated, so in our current study we used transcriptomic and metabolomic approaches to systematically reveal for the first time the molecular mechanisms underlying puerarin’s action in the treatment of PTSD.
Based on the metabolomics analysis, puerarin reversed the perturbance of 17 metabolites such as elaidic acid, daidzein and L-histidine in PTSD. Elaidic acid is a major trans fatty acid and has neurotoxic effects that have been reported to be related to the central nervous system disorders such as Parkinson’s disease, and it may increase cerebral pathological lesions, and deficits in learning and memory in mice (29,30). On the other hand, daidzein and L-histidine may have protective effects on neurons. Daidzein may ameliorate the inflammatory response and apoptosis to exert neuroprotective effects in traumatic brain injury (31,32), and L-histidine has been reported to protect neurons from the toxic effects of zinc, which are related to traumatic brain injury and ischemic stroke, and the plasma histidine level is decreased in obese trauma patients (33,34). Interestingly, the AUC of both daidzein and elaidic acid was 1.00, indicating that they could be potential diagnostic targets for PTSD.
By performing transcriptomic analysis, we found that the significant dysregulation of 99 mRNAs in PTSD was reversed by puerarin treatment, and these dysregulated genes were mainly enriched in the AMPK signaling pathway, PPAR signaling pathway, and cellular senescence. Several lines of evidence show that the AMPK and PPAR signaling pathways may play a role in the pathogenesis and therapy of PTSD. For example, it is reported that electroacupuncture pretreatment exerted neuroprotective effects, ameliorated PTSD-like behaviors and increased the AMPK activity in enhanced SPS-induced rat models (35). Another study revealed that the PPAR signaling pathway was dysregulated in a PTSD rat model, and CPT1B (a key enzyme in the PPAR pathway) was significantly upregulated in the amygdala and peripheral blood, which might contribute to PTSD pathogenesis (36). In addition, studies have demonstrated that PTSD is associated with cellular senescence, and PTSD severity has been strongly negatively associated with a biomarker of cellular senescence—telomere length (TL)—among relatively older subjects (37,38).
Furthermore, our integrated analysis based on data mining, bioinformatics and RT-PCR revealed that CD36, CD59, HBS1L and DYNC1H1 were not only key targets for puerarin in the treatment of PTSD, but also potential biomarkers for the diagnosis and treatment of PTSD. CD36 is a transmembrane protein present in various cell types and involved in multiple biological functions such as fatty acid translocation, inflammation and scavenging of oxidized fatty acids (39). CD36 has been implicated in various nervous system diseases such as Parkinson’s disease and Alzheimer’s disease (40,41). Besides, there is also evidence that CD36 is related to traumatic brain injury and its expression increased in trauma–hemorrhagic shock (42,43). CD59 has been identified as a membrane protein anchored by glycosylphosphatidylinositol, which acts as an inhibitor of membrane attack complex formation to regulate complement activation (44). The high expression of CD59 in many tumors not only aggravates the malignant behaviors of tumor cells but also affects the activities of immune cells, which could be a promising target for tumor immunotherapy (45). CD59 knockout enhanced the marked neuromyelitis optica spectrum disorder pathology (46), and Cheng et al. found that CD59 was upregulated in subjects with trait-anxiety (47). Consistent with those results, our data suggested that CD59 was significantly overexpressed in the peripheral blood of rats or humans with PTSD. In addition, many studies have demonstrated that mutations in the human DYNC1H1 gene are associated with neurological diseases (48), and a recurrent neonatal DYNC1H1 caudate mutation causes spinal muscular atrophy with lower extremity dominance, learning difficulties and mild brain abnormalities (49). However, the role of DYNC1H1 in PTSD has not been reported. In this study, we found for the first time that this gene was lowly expressed in the peripheral blood of PTSD rats, but its specific role needs to be further explored. Furthermore, the integrating transcriptomic and metabolomic analysis revealed that puerarin is mainly involved in antigen processing and presentation, synthesis, and degradation of ketone bodies and one carbon pool by folate the signaling pathway in PTSD that might be main mechanisms of puerarin in the treatment of PTSD. There is little evidence demonstrating a relationship of PTSD and the antigen processing and presentation pathway. However, the major histocompatibility complex I (MHCI) antigen processing and presentation pathway has been reported to contribute to age-related hippocampal dysfunction (50). These pathways and their related genes need further investigation, which may reveal potential targets of PTSD diagnosis and treatment.
Taken together, our data suggested that puerarin alleviated PTSD by regulating the expression of key genes (CD36, CD59, HBS1L and DYNC1H1) and metabolites (elaidic acid, daidzein, 3-succinoylpyridine and 5-(2,5-dihydroxyhexyl) oxolan-2-one), as well as modulating important pathways such as antigen processing and presentation, synthesis and degradation of ketone bodies and one carbon pool by folate. Further investigation is needed to verify the exact function of the puerarin-associated genes, metabolites, and modulated pathways in experimental animal PTSD models as well as in PTSD patients.
Conclusions
We integrated transcriptomics and metabolomics to illuminate the molecular mechanisms of puerarin in the treatment of PTSD. We found that four metabolites and four genes might be considered as novel biomarkers for the diagnosis and treatment of patients with PTSD. Our results provide us potential targets and a basis for exploring novel therapeutic drugs for PTSD.
Acknowledgments
Funding: This work was supported by the National Science and Technology Major Project of China (No. 2020ZX09201-017) and President Foundation of Nanfang Hospital, Southern Medical University (No. 2020C022).
Footnote
Reporting Checklist: The authors have completed the ARRIVE reporting checklist. Available at https://dx.doi.org/10.21037/atm-21-6009
Data Sharing Statement: Available at https://dx.doi.org/10.21037/atm-21-6009
Conflicts of Interest: All authors have completed the ICMJE uniform disclosure form (available at https://dx.doi.org/10.21037/atm-21-6009). The authors have no conflicts of interest to declare.
Ethical Statement: The authors are accountable for all aspects of the work in ensuring that questions related to the accuracy or integrity of any part of the work are appropriately investigated and resolved. Experiments were performed under a project license (No. NFYY-2021-0033) granted by institutional ethics board of Southern Medical University, in compliance with Southern Medical University guidelines for the care and use of animals.
Open Access Statement: This is an Open Access article distributed in accordance with the Creative Commons Attribution-NonCommercial-NoDerivs 4.0 International License (CC BY-NC-ND 4.0), which permits the non-commercial replication and distribution of the article with the strict proviso that no changes or edits are made and the original work is properly cited (including links to both the formal publication through the relevant DOI and the license). See: https://creativecommons.org/licenses/by-nc-nd/4.0/.
References
- Foa EB, Gillihan SJ, Bryant RA. Challenges and Successes in Dissemination of Evidence-Based Treatments for Posttraumatic Stress: Lessons Learned From Prolonged Exposure Therapy for PTSD. Psychol Sci Public Interest 2013;14:65-111. [Crossref] [PubMed]
- Bisson JI, Cosgrove S, Lewis C, et al. Post-traumatic stress disorder. BMJ 2015;351:h6161. [Crossref] [PubMed]
- Birk J, Kronish I, Chang B, et al. The Impact of Cardiac-induced Post-traumatic Stress Disorder Symptoms on Cardiovascular Outcomes: Design and Rationale of the Prospective Observational Reactions to Acute Care and Hospitalizations (ReACH) Study. Health Psychol Bull 2019;3:10-20. [Crossref] [PubMed]
- Clouston SAP, Kuan P, Kotov R, et al. Risk factors for incident prostate cancer in a cohort of world trade center responders. BMC Psychiatry 2019;19:389. [Crossref] [PubMed]
- Blacker CJ, Frye MA, Morava E, et al. A Review of Epigenetics of PTSD in Comorbid Psychiatric Conditions. Genes (Basel) 2019;10:140. [Crossref] [PubMed]
- Milad MR, Quirk GJ. Neurons in medial prefrontal cortex signal memory for fear extinction. Nature 2002;420:70-4. [Crossref] [PubMed]
- Bechara A, Tranel D, Damasio H, et al. Double dissociation of conditioning and declarative knowledge relative to the amygdala and hippocampus in humans. Science 1995;269:1115-8. [Crossref] [PubMed]
- Parsons RG, Ressler KJ. Implications of memory modulation for post-traumatic stress and fear disorders. Nat Neurosci 2013;16:146-53. [Crossref] [PubMed]
- Kitayama N, Vaccarino V, Kutner M, et al. Magnetic resonance imaging (MRI) measurement of hippocampal volume in posttraumatic stress disorder: a meta-analysis. J Affect Disord 2005;88:79-86. [Crossref] [PubMed]
- Lindqvist D, Dhabhar FS, Mellon SH, et al. Increased pro-inflammatory milieu in combat related PTSD - A new cohort replication study. Brain Behav Immun 2017;59:260-4. [Crossref] [PubMed]
- Mellon SH, Bersani FS, Lindqvist D, et al. Metabolomic analysis of male combat veterans with post traumatic stress disorder. PLoS One 2019;14:e0213839. [Crossref] [PubMed]
- Stockmann T, Odegbaro D, Timimi S, et al. SSRI and SNRI withdrawal symptoms reported on an internet forum. Int J Risk Saf Med 2018;29:175-80. [Crossref] [PubMed]
- Pinna G, Galici R, Schneider HH, et al. Alprazolam dependence prevented by substituting with the beta-carboline abecarnil. Proc Natl Acad Sci U S A 1997;94:2719-23. [Crossref] [PubMed]
- Steenkamp MM, Blessing EM, Galatzer-Levy IR, et al. Marijuana and other cannabinoids as a treatment for posttraumatic stress disorder: A literature review. Depress Anxiety 2017;34:207-16. [Crossref] [PubMed]
- Zhou YX, Zhang H, Peng C. Puerarin: a review of pharmacological effects. Phytother Res 2014;28:961-75. [Crossref] [PubMed]
- Su AS, Zhang JW, Zou J. The anxiolytic-like effects of puerarin on an animal model of PTSD. Biomed Pharmacother 2019;115:108978. [Crossref] [PubMed]
- Luo CF, Yuan M, Chen MS, et al. Metabolites of puerarin identified by liquid chromatography tandem mass spectrometry: similar metabolic profiles in liver and intestine of rats. J Chromatogr B Analyt Technol Biomed Life Sci 2010;878:363-70. [Crossref] [PubMed]
- Konjevod M, Nedic Erjavec G, Nikolac Perkovic M, et al. Metabolomics in posttraumatic stress disorder: Untargeted metabolomic analysis of plasma samples from Croatian war veterans. Free Radic Biol Med 2021;162:636-41. [Crossref] [PubMed]
- Wang X, Wang K, Wang H, et al. 1H NMR-based dynamic metabolomics delineates the therapeutic effects of Shenfu injection on laparoscopic hysterectomy. Medicine (Baltimore) 2020;99:e23336. [Crossref] [PubMed]
- Chen RH, Du WD, Wang Q, et al. Effects of Acanthopanax senticosus (Rupr. & Maxim.) Harms on cerebral ischemia-reperfusion injury revealed by metabolomics and transcriptomics. J Ethnopharmacol 2021;264:113212. [Crossref] [PubMed]
- Szklarczyk D, Franceschini A, Kuhn M, et al. The STRING database in 2011: functional interaction networks of proteins, globally integrated and scored. Nucleic Acids Res 2011;39:D561-8. [Crossref] [PubMed]
- Wang W, Lou W, Ding B, et al. A novel mRNA-miRNA-lncRNA competing endogenous RNA triple sub-network associated with prognosis of pancreatic cancer. Aging (Albany NY) 2019;11:2610-27. [Crossref] [PubMed]
- Lou W, Chen J, Ding B, et al. Identification of invasion-metastasis-associated microRNAs in hepatocellular carcinoma based on bioinformatic analysis and experimental validation. J Transl Med 2018;16:266. [Crossref] [PubMed]
- Rusch HL, Robinson J, Yun S, et al. Gene expression differences in PTSD are uniquely related to the intrusion symptom cluster: A transcriptome-wide analysis in military service members. Brain Behav Immun 2019;80:904-8. [Crossref] [PubMed]
- Mellon SH, Gautam A, Hammamieh R, et al. Metabolism, Metabolomics, and Inflammation in Posttraumatic Stress Disorder. Biol Psychiatry 2018;83:866-75. [Crossref] [PubMed]
- Tao J, Cui Y, Duan Y, et al. Puerarin attenuates locomotor and cognitive deficits as well as hippocampal neuronal injury through the PI3K/Akt1/GSK-3beta signaling pathway in an in vivo model of cerebral ischemia. Oncotarget 2017;8:106283-95. [Crossref] [PubMed]
- Liu X, Mo Y, Gong J, et al. Puerarin ameliorates cognitive deficits in streptozotocin-induced diabetic rats. Metab Brain Dis 2016;31:417-23. [Crossref] [PubMed]
- Liu S, Cao XL, Liu GQ, et al. The in silico and in vivo evaluation of puerarin against Alzheimer’s disease. Food Funct 2019;10:799-813. [Crossref] [PubMed]
- Ma WW, Zhao L, Yuan LH, et al. Elaidic acid induces cell apoptosis through induction of ROS accumulation and endoplasmic reticulum stress in SHSY5Y cells. Mol Med Rep 2017;16:9337-46. [Crossref] [PubMed]
- Zhang MA, Chen FH, Huang ZY, et al. Elaidic acid enhanced the simultaneous neurotoxicity attributable to the cerebral pathological lesion resulted from oxidative damages induced by acrylamide and benzo(a)pyrene. Toxicol Ind Health 2011;27:661-72. [Crossref] [PubMed]
- Zhang F, Ru N, Shang ZH, et al. Daidzein ameliorates spinal cord ischemia/reperfusion injury-induced neurological function deficits in Sprague-Dawley rats through PI3K/Akt signaling pathway. Exp Ther Med 2017;14:4878-86. [Crossref] [PubMed]
- Rui Q, Ni H, Lin X, et al. Astrocyte-derived fatty acid-binding protein 7 protects blood-brain barrier integrity through a caveolin-1/MMP signaling pathway following traumatic brain injury. Exp Neurol 2019;322:113044. [Crossref] [PubMed]
- Ralph DM, Robinson SR, Campbell MS, et al. Histidine, cystine, glutamine, and threonine collectively protect astrocytes from the toxicity of zinc. Free Radic Biol Med 2010;49:649-57. [Crossref] [PubMed]
- Jeevanandam M, Ramias L, Schiller WR. Altered plasma free amino acid levels in obese traumatized man. Metabolism 1991;40:385-90. [Crossref] [PubMed]
- Zhou CH, Xue F, Xue SS, et al. Electroacupuncture Pretreatment Ameliorates PTSD-Like Behaviors in Rats by Enhancing Hippocampal Neurogenesis via the Keap1/Nrf2 Antioxidant Signaling Pathway. Front Cell Neurosci 2019;13:275. [Crossref] [PubMed]
- Zhang L, Li H, Hu X, et al. Mitochondria-focused gene expression profile reveals common pathways and CPT1B dysregulation in both rodent stress model and human subjects with PTSD. Transl Psychiatry 2015;5:e580. [Crossref] [PubMed]
- Connolly SL, Stoop TB, Logue MW, et al. Posttraumatic Stress Disorder Symptoms, Temperament, and the Pathway to Cellular Senescence. J Trauma Stress 2018;31:676-86. [Crossref] [PubMed]
- Stein JY, Levin Y, Uziel O, et al. Traumatic stress and cellular senescence: The role of war-captivity and homecoming stressors in later life telomere length. J Affect Disord 2018;238:129-35. [Crossref] [PubMed]
- Niculite CM, Enciu AM, Hinescu ME. CD 36: Focus on Epigenetic and Post-Transcriptional Regulation. Front Genet 2019;10:680. [Crossref] [PubMed]
- Chew EGY, Liany H, Tan LCS, et al. Evaluation of novel Parkinson’s disease candidate genes in the Chinese population. Neurobiol Aging 2019;74:235.e1-e4. [Crossref] [PubMed]
- Yuan C, Aierken A, Xie Z, et al. The age-related microglial transformation in Alzheimer’s disease pathogenesis. Neurobiol Aging 2020;92:82-91. [Crossref] [PubMed]
- Deitch EA, Condon M, Feketeova E, et al. Trauma-hemorrhagic shock induces a CD36-dependent RBC endothelial-adhesive phenotype. Crit Care Med 2014;42:e200-10. [Crossref] [PubMed]
- Zhao Z, Zhou Y, Hilton T, et al. Extracellular mitochondria released from traumatized brains induced platelet procoagulant activity. Haematologica 2020;105:209-17. [Crossref] [PubMed]
- Zhang R, Liu Q, Peng J, et al. Pancreatic cancer-educated macrophages protect cancer cells from complement-dependent cytotoxicity by up-regulation of CD59. Cell Death Dis 2019;10:836. [Crossref] [PubMed]
- Zhang R, Liu Q, Liao Q, et al. CD59: a promising target for tumor immunotherapy. Future Oncol 2018;14:781-91. [Crossref] [PubMed]
- Yao X, Verkman AS. Marked central nervous system pathology in CD59 knockout rats following passive transfer of Neuromyelitis optica immunoglobulin G. Acta Neuropathol Commun 2017;5:15. [Crossref] [PubMed]
- Cheng LZ, Li CY, Deng GH. Effect on the Concentration on CD55 and CD59 in RBC Membrane Among New Recruits of Different Trait-anxiety Levels Under Ball Firing Examination. Chinese Journal of Clinical Psychology 2006;14:98-9.
- Hoang HT, Schlager MA, Carter AP, et al. DYNC1H1 mutations associated with neurological diseases compromise processivity of dynein-dynactin-cargo adaptor complexes. Proc Natl Acad Sci U S A 2017;114:E1597-606. [Crossref] [PubMed]
- Chan SHS, van Alfen N, Thuestad IJ, et al. A recurrent de novo DYNC1H1 tail domain mutation causes spinal muscular atrophy with lower extremity predominance, learning difficulties and mild brain abnormality. Neuromuscul Disord 2018;28:750-6. [Crossref] [PubMed]
- VanGuilder Starkey HD, Van Kirk CA, Bixler GV, et al. Neuroglial expression of the MHCI pathway and PirB receptor is upregulated in the hippocampus with advanced aging. J Mol Neurosci 2012;48:111-26. [Crossref] [PubMed]
(English Language Editor: K. Brown)