The establishment of a hub differential gene model to predict prognosis in stage II and III right- and left-sided colon cancer patients
Introduction
Colon cancer is the most common intestinal cancer and is now the third most common cancer globally. There are histological, embryological, and clinical differences in left- and right-sided colon cancer. Despite the variety of treatments available for colon cancer, the prognosis is poor when colon cancer metastasizes to the lymph nodes and distant organs (1). Compared with patients with left-sided colon cancer, right-sided colon cancer patients have been shown to have increased lymphovascular invasion and a more advanced tumor stage (2). There is growing consensus that right- and left-sided colon cancer have different molecular features (3). A growing number of differential genes have been discovered with the development of technology and extensive previous research, such as the KRAS, BRAF, and BRAC1 mutations. The molecular difference between left-sided and right-sided colon cancer patients is mainly caused by different tissue and embryo development. However, finding disease-related genes using methods such as sequencing alone is too time-consuming and costly. With the development of computer algorithms, machine learning occupies an important position in cancer prediction and prognosis (4). Wang et al. constructed a hub gene prognosis model by multiplying regression coefficient by expression value based on the combined analysis of transcriptome and methylation data (5). If an algorithm can be constructed to effectively identify the hub genes that differentiate left-sided from right-sided colon cancer, it will be of great significance in predicting the prognosis of these patients. In addition, the identified hub genes are likely to be biomarkers of the disease, and this information will be useful for subsequent experimental verification.
Analysis of colon cancer patients based on transcriptome data is currently a feasible approach. Liang et al. constructed a model which predicted the prognosis of right- and left-sided colon cancer patients using The Cancer Genome Atlas (TCGA) public database (6). The molecular differences between left- and right-sided colon cancer patients are essential differences in the expression of key genes. If genetic risk scoring can be used, it will significantly benefit clinical treatment and prognosis. For example, MMC, DMNC, and other algorithms can perform screening for transcriptome data. In conclusion, the use of relevant genetic and clinical information and the construction of a prognosis model can play a crucial role. High-throughput technology and bioinformatics make it possible to build this model (7).
To study the hub genes of left- and right-sided colon cancer patients and construct a prognostic scoring model, we used colon cancer patients’ data from the TCGA. Data from 243 colon cancer patients in stages II and III were selected to perform the differential gene analysis. By using MMC, DMNC, and other algorithms, we obtained several hub genes. A principal component analysis (PCA) was used to verify the screening validity. After Cox regression, the BST2 gene and associated clinical information were used to construct a prognostic model. Finally, we evaluated the efficacy of our model in predicting survival. Our findings may provide a valuable contribution to the prognosis and treatment of left- and right-sided colon cancer patients.
We present the following article in accordance with the TRIPOD reporting checklist (available at https://dx.doi.org/10.21037/atm-21-6163).
Methods
Data collection and preprocessing
The study was conducted in accordance with the Declaration of Helsinki (as revised in 2013). We downloaded the transcriptome data and clinical information from 523 patients from the TCGA public database on September 3rd. Of these, 453 people had both transcriptome information and clinical information. Next, 243 patients in stages II and III were divided into two groups by the International Oncology Code. The right-sided colon cancer (RCC) group comprised 154 patients with codes C18.0, C18.2, C18.3, and C18.4. The left-sided colon cancer (LCC) group included 89 patients with codes C18.5, C18.6, and C18.7. The remaining patients were excluded. According to an American epidemiological study, the ratio of left-sided and right-sided colon cancer patients is 1:2 (8). Our groupings were approximately equal to this ratio. Differential gene analysis was complete-case analysis. However, we used multiple imputation to supplement missing value in predictive variables with “mice” R software package.
Statistical analysis
All statistical analyses were performed using R software (version 4.0.3). We used the “limma” R software package to complete the differential analysis (9). The results of the differential screening were displayed in a volcano plot using the “ggplot2” package in R software (10). The preliminary results of the principal components were visualized by the “factoMineR” and “factoextra” R packages (11,12). The correlation analysis and visualization between the hub genes were completed by the “cor” R software function and “ggcorrplot” R software package (13). Univariate and multivariate Cox regressions were conducted by using the “survival” package in R. The overall survival in BST2 low group and high group were compared using the Kaplan-Meier method with a log rank-test. Receiver operating characteristic (ROC) curve analysis and the subsequent calculation of the area under the curve (AUC) were performed using the “pROC” and “survivalROC” packages in R. A P value of less than 0.05 was considered to be statistically significant.
Differential gene analysis and annotation
Differential transcription products with a fold change (FC) >1.5 and a P value <0.05 were considered to be differentially expressed products. We chose FC >1.5 to avoid missing key genes and made the screening more comprehensive. All the products corresponded to the genes to which they belonged. All differential genes were uploaded to the Database for Annotation, Visualization, and Integrated Discovery (DAVID) to perform the functional annotations (14). The results from the Kyoto Encyclopedia of Genes and Genomes (KEGG) pathway enrichment and the Gene Ontology (GO) analysis in DAVID were downloaded (15). After that, we used a bubble chart and bar chart with the “ggplot2” package and Excel software to visualize the KEGG and GO results. Subsequently, the hub genes were imported into the Funrich software program to perform the biological pathway and process analyses (16). The results of the analyses can be obtained directly from Funrich.
Differential protein analysis and network construction
After screening, the transcriptome product identification corresponded to the gene name. The identified genes were uploaded to the STRING database for the protein interaction analysis (17). Data from the protein node interactions were imported into Cytoscape software for calculation (https://cytoscape.org/what_is_cytoscape.html). According to 12 algorithms in cytoHubba which was a Cytoscape plugin, including MMC and DMNC, the first 30 genes of each algorithm were derived (18). Ultimately, 37 hub genes were obtained by de-duplicating. We constructed the protein-protein interaction (PPI) in the STRING database. In addition, we also constructed PPI in Cytoscape according to the nodes and the up-down relationships.
Relevant information analysis and model construction
We performed PCA for 37 hub genes with the “psych” R package (19). The proportion of variance of the first principal component reached 22%. Subsequently, we used the “coxph” function to perform the Cox regression analysis of differential genes, with genes having a P value <0.2 selected for the model building. Genes that reached significance in the Cox regression analysis were uploaded to the cBioPortal database for the mutation and survival analyses (20). We finally selected the BST2 gene as a predictive variable. The predictive variables included BST2, PC1, cancer stage, preoperative pretreatment carcinoembryonic antigen (CEA) levels, lymph node count, and KRAS gene mutation analysis results. We also explored the correlation between BST2 and the relevant clinical information of patients. Several boxplots were selected to show the correlations using the “ggboxlplot” R package. Finally, the probability of survival over several years was predicted, and the efficacy of the prediction was evaluated by a receiver operating characteristic (ROC) curve analysis (21).
Results
Screening of differential gene
The results of the differential gene screening are shown in the volcano map. Blue and red represent downregulation and upregulation of different genes, respectively (Figure 1). We found 167 upregulated genes and 115 downregulated genes. However, only 251 genes met the criteria of P<0.05 and FC >1.5. Of these 251 genes, 90 genes were downregulated, and 161 genes were upregulated.
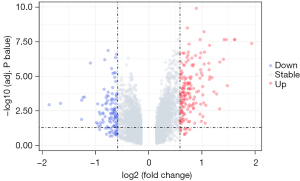
Hub gene PPI construction and differential gene related pathway analysis
Differential genes were screened by a variety of algorithms and regressions. The 37 key genes we selected represented most genes. After we uploaded 37 genes in the STRING database, we plotted the corresponding PPI (Figure 2A). The PPI showed us that MUC1 and its surrounding genes are more likely to be central. According to the text, the purple line shows the relationship that has been proved experimentally, and the green line shows the relationship that STRING mines. We also plotted PPI in Cytoscape according to node and regulation (Figure 2B). The larger the node, the greater the number of interactions. Green labels represent upregulation, and red labels represent downregulation (patients with left colon cancer were used as controls).
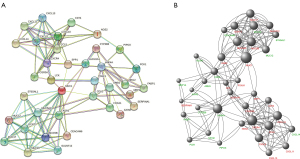
Bar charts were used to show the biological processes in the GO analysis. The analysis revealed that differences in genes were primarily located in immune response, cell-cell signaling, and O-glycan processing (Figure 3A). After we translated the results into a table, we presented the partial results of the KEGG pathway analysis using bubble diagrams. We still found marked differences in the genes involved in the metabolic and chemokine signaling pathways (Figure 3B). This proves that the gene differences we were looking for in left- and right-sided colon cancer were confirmed.
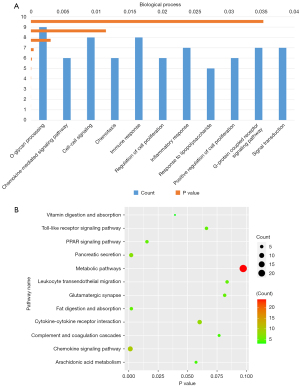
PC1 extraction of hub gene
We used a principal component scatter plot to reflect the relationship between the observed values and the principal components (Figure 4A). Most points were distributed around the center, and most of the observed values had a strong relationship with the first principal component. The redder the color, the more valuable it was. Moreover, we also explored the 37 hub genes’ contribution to the first and second principal components (Figure 4B). It can be seen that the first ten genes up to NR1I2 made significant contributions to the first and second principal components. We investigated the relationship between the PC1 and the expression values of the first ten hub genes (Figure 4C). We also explored whether PC1 could predict left- and right-sided colon cancer, and the area under the ROC curve (AUC) showed a good result (Figure 4D).
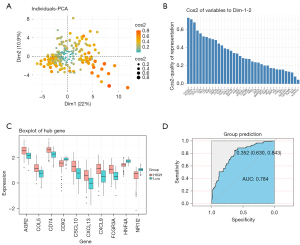
Gene enrichment and correlation analysis of hub gene
To explore whether the 37 hub genes had representative significance, we entered them into the Funrich software for analysis. The biological pathway showed that all 37 hub genes were significantly correlated with CXCR3-mediated signaling events and the chemokine receptors bind chemokines (Figure 5A). This suggested that relevant significant biological pathway may be related to the recognition between cells. The biological process still showed an immune response (Figure 5B). However, in the protein domains, SCY and signal peptide differed between the hub gene set and the differential gene set (Figure 5C). Therefore, the 37 hub genes represented a large proportion of the differential genes. We also created heat maps of the relationships between the 37 genes (Figure 5D), showing positive and negative correlations in different colors. For instance, FCGR3A and CXCL9 showed a high positive correlation.
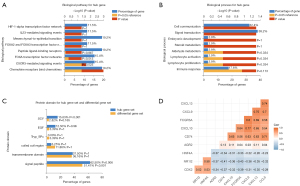
Relationship between BST2, PC1, and relevant clinical information
We explored the relationship between BST2, PC1, and relevant clinical information with the use of boxplots. The principal component value and BST2 expression value of the right-sided group were higher than those of the left-sided group (Figure 6A,6B). Additionally, the high BST2 group had a higher PC1 value than the low group (Figure 6C). People with high BST2 expression levels were relatively older (Figure 6D). It is worth noting that the BST2 expression value in people without sigmoid colon cancer was significantly higher than those with sigmoid colon cancer (Figure 6E).
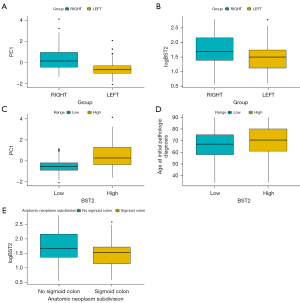
Construction of prognostic model
In the significant gene group identified by Cox regression, the mutation occurred mainly in patients with right-sided colon cancer (Figure 7A). Therefore, we decided that a prediction of prognosis was more appropriate in patients with right-sided colon cancer. We also plotted survival curves for the first three years between the two groups (Figure 7B). The survival of the Cox significant group was significantly worse than that of the non-significant group. To test whether our model effectively predicted right-sided patient survival, we used an ROC curve for verification. The ROC curve showed good predictions at 1 and 3 years. The AUC at 1 and 3 years was 0.76 and 0.765, respectively (Figure 7C,7D). In general, the prediction results were satisfactory.
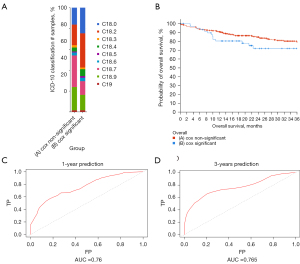
Discussion
There is now widespread consensus that molecular differences between left- and right-sided colon cancer are the more likely cause of differences in the disease. The mechanisms of difference in left- and right-sided colon cancer depends on their gene pathways. Genes associated with poor prognosis are significantly higher in right-sided colorectal cancer (22). Mukund et al. explored differences in molecular mechanisms between left- and right-sided colon cancer at the methylation and transcription levels (23). Their study provided a good explanation for the molecular differences that cause right- and left-sided colon cancer, but their results were limited and failed to translate to clinical application in diagnosis or prognosis. Chang et al. established a recurrence score (RS) to evaluate the relationship between differential genes and age. They concluded that differential gene expression did not differ by age or stage (II and III) (24). Their results provide strong evidence that molecular differences play an important role. However, our model did show better predictive performance with the addition of stage. After screening for differentially expressed genes in left- and right-sided colon cancer patients, we did not continue to use regression screening as other researchers have. Instead, the protein interaction data of the gene expressions were screened twice by 12 algorithms in Cytoscape to greatly improve our chances of identifying the hub genes. In constructing models, many researchers directly use Cox or LASSO regression analyses (25).
We borrowed the methods of other researchers who used principal components to intuitively show the characteristics of the high- and low-risk groups (26). We also used Cox regression to screen the genes that affected the survival of patients. Finally, after 37 hub genes were obtained through 12 algorithms, the first principal component value was used as one of the variables of our model for left- and right-sided colon cancer patients. Each patient received an independent value, which avoided the problem of establishing training sets and validation sets to verify the validity of the value. Our concept was similar to Xu, Xu, and Yin’s idea of quantifying immune cell infiltration (ICI) patterns in individual tumors by using PCA to obtain ICI scores in the colon cancer tumor microenvironment (27). We finally selected the BST2 gene as another predictor because it was both a differential gene and a Cox regression significant gene. Left- and right-sided colon cancer continue to lack unique biomarkers at present, so discovering new biomarkers is undoubtedly helpful in targeting therapies (28). The screening approach we used has the potential to help other researchers find biomarkers that are unique to the treatment of left- and right-sided colon cancer. Pathway analysis revealed that our hub genes were significantly associated with immunity and relevant metabolic pathways. We also found that genes identified as significant in the Cox regression were mainly mutated in right-sided colon cancer. As such, we considered the model was better for predicting right-sided colon cancer, although we used it for both sides. Previous studies have shown that the BST2 gene shows hypomethylation in colon cancer tissues relative to adjacent normal tissues (29). Additionally, relevant clinical information was associated with BST2 and PC1 levels. We used BST2, PC1, pretreatment CEA levels, lymph node counts, and KRAS gene mutation results to build a predictive survival model in right-sided colon cancer patients.
In our prediction, patients with left-sided colon cancer did better than those with right-sided colon cancer, consistent with previous studies (30). Our Cox regression analysis showed that most of the significant genes were enriched in right-sided colon cancer compared with left-sided colon cancer (31). This may be related to lower survival rates in patients with right- rather than left-sided colon cancer. Whether the disparate survival rates were related to these Cox significant genes needs further study. We were surprised to find a lower BST2 value in sigmoid colon cancer, which may be a specific question worth exploring in the future. As a differential gene and a Cox regression significant gene, BST2 is likely to be a biomarker of left- and right-sided colon cancer, including sigmoid colon cancer.
Conclusions
In conclusion, we screened for differentially expressed genes in stage II and III left- and right-sided colon cancer patients and constructed a model of differential genes using algorithms and other statistical methods. We also explored the relationship between relevant clinical information and predictive variables and demonstrated the predictive effect of our model. Our study also had limitations, which will be addressed in our future study. We encourage other researchers to further validate the correlation between the model and classical pathways or important molecules. Subsequent experiments should be carried out to verify that BST2 can be used as a biomarker. The reliability of our model will also require prospective cohort or case-control evaluation.
Acknowledgments
Funding: None.
Footnote
Reporting Checklist: The authors have completed the TRIPOD reporting checklist. Available at https://dx.doi.org/10.21037/atm-21-6163
Conflicts of Interest: All authors have completed the ICMJE uniform disclosure form (available at https://dx.doi.org/10.21037/atm-21-6163). The authors have no conflicts of interest to declare.
Ethical Statement: The authors are accountable for all aspects of the work in ensuring that questions related to the accuracy or integrity of any part of the work are appropriately investigated and resolved. The study was conducted in accordance with the Declaration of Helsinki (as revised in 2013).
Open Access Statement: This is an Open Access article distributed in accordance with the Creative Commons Attribution-NonCommercial-NoDerivs 4.0 International License (CC BY-NC-ND 4.0), which permits the non-commercial replication and distribution of the article with the strict proviso that no changes or edits are made and the original work is properly cited (including links to both the formal publication through the relevant DOI and the license). See: https://creativecommons.org/licenses/by-nc-nd/4.0/.
References
- Ait Ouakrim D, Pizot C, Boniol M, et al. Trends in colorectal cancer mortality in Europe: retrospective analysis of the WHO mortality database. BMJ 2015;351:h4970. [Crossref] [PubMed]
- Narayanan S, Gabriel E, Attwood K, et al. Association of Clinicopathologic and Molecular Markers on Stage-specific Survival of Right Versus Left Colon Cancer. Clin Colorectal Cancer 2018;17:e671-8. [Crossref] [PubMed]
- Dekker E, Tanis PJ, Vleugels JLA, et al. Colorectal cancer. Lancet 2019;394:1467-80. [Crossref] [PubMed]
- Jiang Y, Yan X, Liu K, et al. Discovering the molecular differences between right- and left-sided colon cancer using machine learning methods. BMC Cancer 2020;20:1012. [Crossref] [PubMed]
- Wang X, Zhang D, Zhang C, et al. Identification of epigenetic methylation-driven signature and risk loci associated with survival for colon cancer. Ann Transl Med 2020;8:324. [Crossref] [PubMed]
- Liang L, Zeng JH, Qin XG, et al. Distinguishable Prognostic Signatures of Left- and Right-Sided Colon Cancer: a Study Based on Sequencing Data. Cell Physiol Biochem 2018;48:475-90. [Crossref] [PubMed]
- Zhang X, Zhao H, Shi X, et al. Identification and validation of an immune-related gene signature predictive of overall survival in colon cancer. Aging (Albany NY) 2020;12:26095-120. [Crossref] [PubMed]
- Siegel RL, Miller KD, Goding Sauer A, et al. Colorectal cancer statistics, 2020. CA Cancer J Clin 2020;70:145-64. [Crossref] [PubMed]
- Ritchie ME, Phipson B, Wu D, et al. limma powers differential expression analyses for RNA-sequencing and microarray studies. Nucleic Acids Res 2015;43:e47. [Crossref] [PubMed]
- Wickham H. ggplot2. WIREs Comp Stat 2011;3:180-5. [Crossref]
- Kassambara A, Mundt F. Package ‘factoextra’. Extract and visualize the results of multivariate data analyses. 2017. Available online: https://cran.r-project.org/web/packages/factoextra/factoextra.pdf
- Husson F, Josse J, Le S, Mazet J, Husson MF. Package ‘FactoMineR’. An R Package 2016;96:698.
- Kassambara A, Kassambara MA. Package ‘ggcorrplot’. R package version 01. 2019. Available online: https://cran.r-project.org/web/packages/ggcorrplot/ggcorrplot.pdf
- Dennis G Jr, Sherman BT, Hosack DA, et al. DAVID: Database for Annotation, Visualization, and Integrated Discovery. Genome Biol 2003;4:3. [Crossref] [PubMed]
- Zhang T, Jiang M, Chen L, et al. Prediction of gene phenotypes based on GO and KEGG pathway enrichment scores. Biomed Res Int 2013;2013:870795. [Crossref] [PubMed]
- Pathan M, Keerthikumar S, Ang CS, et al. FunRich: An open access standalone functional enrichment and interaction network analysis tool. Proteomics 2015;15:2597-601. [Crossref] [PubMed]
- Szklarczyk D, Morris JH, Cook H, et al. The STRING database in 2017: quality-controlled protein-protein association networks, made broadly accessible. Nucleic Acids Res 2017;45:D362-8. [Crossref] [PubMed]
- Shannon P, Markiel A, Ozier O, et al. Cytoscape: a software environment for integrated models of biomolecular interaction networks. Genome Res 2003;13:2498-504. [Crossref] [PubMed]
- Revelle WR. psych: Procedures for personality and psychological research. 2017. Available online: https://www.scholars.northwestern.edu/en/publications/psych-procedures-for-personality-and-psychological-research
- Gao J, Aksoy BA, Dogrusoz U, et al. Integrative analysis of complex cancer genomics and clinical profiles using the cBioPortal. Sci Signal 2013;6:pl1. [Crossref] [PubMed]
- Fawcett T. An introduction to ROC analysis. Pattern Recognit Lett 2006;27:861-74. [Crossref]
- Jensen CE, Villanueva JY, Loaiza-Bonilla A. Differences in overall survival and mutation prevalence between right- and left-sided colorectal adenocarcinoma. J Gastrointest Oncol 2018;9:778-84. [Crossref] [PubMed]
- Mukund K, Syulyukina N, Ramamoorthy S, et al. Right and left-sided colon cancers - specificity of molecular mechanisms in tumorigenesis and progression. BMC Cancer 2020;20:317. [Crossref] [PubMed]
- Chang GJ, You YNY, Russell CA, et al. Young-Onset Colon Cancer and Recurrence Risk by Gene Expression. J Natl Cancer Inst 2020;112:1170-3. [Crossref] [PubMed]
- Xu J, Dai S, Yuan Y, et al. A Prognostic Model for Colon Cancer Patients Based on Eight Signature Autophagy Genes. Front Cell Dev Biol 2020;8:602174. [Crossref] [PubMed]
- Tang H, Mirshahidi S, Senthil M, et al. Down-regulation of LXR/RXR activation and negative acute phase response pathways in colon adenocarcinoma revealed by proteomics and bioinformatics analysis. Cancer Biomark 2014;14:313-24. [Crossref] [PubMed]
- Xu H, Xu Q, Yin L. Prognostic value of tumor immune cell infiltration patterns in colon adenocarcinoma based on systematic bioinformatics analysis. Cancer Cell Int 2021;21:344. [Crossref] [PubMed]
- Baran B, Mert Ozupek N, Yerli Tetik N, et al. Difference Between Left-Sided and Right-Sided Colorectal Cancer: A Focused Review of Literature. Gastroenterology Res 2018;11:264-73. [Crossref] [PubMed]
- Chu CH, Chang SC, Wang HH, et al. Prognostic Values of EPDR1 Hypermethylation and Its Inhibitory Function on Tumor Invasion in Colorectal Cancer. Cancers (Basel) 2018;10:393. [Crossref] [PubMed]
- Shen H, Yang J, Huang Q, et al. Different treatment strategies and molecular features between right-sided and left-sided colon cancers. World J Gastroenterol 2015;21:6470-8. [Crossref] [PubMed]
- Imperial R, Ahmed Z, Toor OM, et al. Comparative proteogenomic analysis of right-sided colon cancer, left-sided colon cancer and rectal cancer reveals distinct mutational profiles. Mol Cancer 2018;17:177. [Crossref] [PubMed]
(English Language Editor: D. Fitzgerald)