Identification of a novel pyroptosis-related gene signature correlated with the prognosis of diffuse glioma patients
Introduction
Gliomas, the most common primary tumors of the central nervous system, account for 81% of malignant brain tumors (1). Diffuse gliomas, which are characterized by extensive infiltration and growth into surrounding tissues, are the main type of gliomas that lead to poor prognosis, especially in adult patients. The World Health Organization (WHO) classifies diffuse gliomas into 3 grades (II, III and IV) based on the extent of mitotic activity, necrosis, and microvascular proliferation. These gliomas are classified as diffuse astrocytomas, oligodendrogliomas, oligoastrocytomas (tumors mixed astrocytic and oligodendroglial) according to histopathologic analysis. The survival rate of diffuse glioma patients has improved with the help of various treatment methods (including surgery, radiotherapy, and chemotherapy); however, the prognosis of patients is still far from reaching expectations (2). Due to treatment drug resistance and the high recurrence rate of gliomas, molecular-targeted therapy has gradually attracted attention. However, targeted therapy faces great challenges because of the heterogeneity of the molecular pathology of gliomas (3,4). The molecular characteristics of diffuse glioma vary greatly among histological subtypes. For instance, the mutation of isocitrate dehydrogenase 1/2 (IDH 1/2) is frequent in WHO grade II and III astrocytomas (>80%), oligodendrogliomas and ‘secondary’ glioblastomas. Primary glioblastoma is more likely to be IDH-wildtype, whose prognosis is worse than it of IDH-mutant type (5).
In recent years, the prognostic value of PCD related genes in gliomas has been extensively studied. Such as Xu et al. (6) established a fifteen autophagy-related genes signature associated with clinical prognosis and immune mircroenvironment. And Chen et al. (7) identified eleven ferroptosis-related genes highly correlated with the prognosis of glioma patient. Whereas, the related genes of pyroptosis, a novel type of PCD, whose prognostic value remains unknown in diffuse glioma.
Pyroptosis, an inflammatory form of programmed cell death (PCD), which is mediated by Caspase (CASP)-1 or CASP11/–4/–5, has similar features to apoptosis [e.g., deoxyribonucleic acid (DNA) fragmentation, and chromatin condensation]. Pyroptosis can be distinguished from other types of PCD by its specific characteristics, such as DNA laddering, cell swelling, and osmotic lysis (8). Typically, activated by the reaction to microbial infection and endogenous danger signals, CASP will cleave Gasdermin D (GSDMD) into the N-terminal (GSDMD-NT) and C-terminal (GSDMD-CT) fragment of GSDMD, while GSDMD-NT will attach to the cell membrane and oligomerize, leading to the formation of pores. This change in membrane morphology results in an imbalance inside and outside the cell, which leads to cell lysis and death, accompanied by the release of abundant inflammatory factors [e.g., interleukin (IL)-1β and IL-18 (IL18), which ripped by the active GSDMD] (9). Interestingly, caspase-3, which has traditionally been considered a key executor of apoptosis, can also induce pyroptosis via Gasdermin E (GSEME) (10).
Previous research has shown that pyroptosis plays an important role in promoting and resisting cancer. Pyroptosis can inhibit the proliferation and metastasis of cancer cells by PCD; however, the inflammatory microenvironment formed by pyroptosis provides suitable conditions for tumor growth (11). Recently, Jiang et al. found that CASP1 is upregulated in glioma tissues and the glioma cell lines U87 and T98G, while micro ribonucleic acid (miRNA)-214 inhibits the proliferation and migration of glioma cells by decreasing the expression of CASP1, which suggests that pyroptosis plays an essential role in the progression of glioma (12). However, the mechanism of pyroptosis in glioma and the clinical value of pyroptosis-related genes (PRGs) remain unknown. An in-depth study on the molecular mechanism of pyroptosis will help to identify novel targets for disease treatment.
In this study, we examined the prognostic value of 33 PRGs in patients with diffuse glioma for the first time, and constructed a risk signature to predict patients’ overall survival (OS) time. RNA-sequencing data and matched clinical information from 625 diffuse glioma samples from 620 patients in The Cancer Genome Atlas (TCGA) and 1018 diffuse glioma samples from 2 independent cohorts in the Chinese Glioma Genome Atlas (CGGA) were obtained for this study. Ultimately, CASP3 and IL18 were significantly differentially expressed between normal and tumor tissues, and highly related to the prognosis of diffuse glioma patients. Based on CASP3 and IL18, a 2-PRG signature was established with a time-dependent receiver operating characteristic (ROC) curve. The signature had excellent capability in predicting patients’ OS time. Patients were divided into high- and low-risk subgroups based on the median risk score, and difference in patients’ survival outcomes and the immune microenvironment were investigated. In addition, we predicted and visualized the regulatory network of competing endogenous RNA (ceRNA) associated with IL18 and CASP3. We present the following article in accordance with the STREGA reporting checklist (available at https://dx.doi.org/10.21037/atm-21-6011).
Methods
Data source
The study was conducted in accordance with the Declaration of Helsinki (as revised in 2013). Through the Xena platform (http://xena.ucsc.edu/) (13), the RNA-sequencing data and clinical information [e.g., age, gender, WHO grade, and isocitrate dehydrogenase (IDH) mutation] of TCGA-Glioma [TCGA-low-grade glioma (LGG) and TCGA-glioblastoma (GBM)] tumor samples were obtained on July 11, 2021. Similarly, the relevant data of brain samples from the Genome Tissue Expression (GTEx) (14) database (www.gtexportal.org) were downloaded and matched as normal tissues via the Xena platform. To match the normal and tumor samples, the RNA-sequencing of TCGA-Glioma and GTEx-Brain were merged and normalized using the “limma” R package. Additionally, normalized gene expression was measured as fragments per kilobase of transcript per million (FPKM) mapped reads and log2-based transformation [log2(FPKM + 1)]. We also downloaded the related information of 2 independent patient cohorts (mRNAseq_693 and mRNAseq_325) from the CGGA (http://www.cgga.org.cn/) (15) as validation sets. Patient information is shown in Table 1.
Table 1
Variable | Training cohort | Validation cohort | ||
---|---|---|---|---|
TCGA | CGGA mRNAseq_693 | CGGA mRNAseq_325 | ||
Age | ||||
≥50 | 254 (41.0%) | 202 (29.1%) | 98 (30.2%) | |
<50 | 351 (56.6%) | 490 (70.7%) | 227 (69.8%) | |
NA | 15 (2.4%) | 1 (0.2%) | 0 (0.0%) | |
Gender | ||||
Male | 343 (55.3%) | 398 (57.4%) | 203 (62.5%) | |
Female | 262 (42.3%) | 295 (42.6%) | 122 (37.5%) | |
NA | 15 (2.4%) | 0 (0.0%) | 0 (0.0%) | |
LGG/GBM | ||||
LGG | 473 (76.3%) | 443 (63.9%) | 182 (56.0%) | |
GBM | 147 (23.7%) | 249 (35.9%) | 139 (42.8%) | |
NA | 0 (0.0%) | 1 (0.2%) | 4 (1.2%) | |
WHO grade | ||||
II | 229 (36.9%) | 188 (27.1%) | 103 (31.7%) | |
III | 244 (39.4%) | 255 (36.8%) | 79 (24.3%) | |
IV | 147 (23.7%) | 249 (35.9%) | 139 (42.8%) | |
NA | 0 (0.0%) | 1 (0.2%) | 4 (1.2%) | |
IDH mutation | ||||
Wildtype | 155 (25.0%) | 286 (41.3%) | 149 (45.8%) | |
Mutant | 95 (15.3%) | 356 (51.4%) | 175 (53.8%) | |
NA | 370 (59.7%) | 51 (7.3%) | 1 (0.4%) | |
Total of patients | 620 | 693 | 325 |
NA, not available; LGG, low-grade glioma; GBM, glioblastoma; IDH, isocitrate dehydrogenase.
Identification of DE_PRGs
Recent studies have identified 33 PRGs (11,16-18). Differentially expressed genes (DEGs) (|Log2FoldChange >1| and adj.P<0.05) between TCGA-LGG/GBM and GTEx-Brain samples were identified via the “limma” R package. After intersecting DEGs with PRGs, DE_PRGs were obtained. Additionally, to determine the functional annotation of DE_PRGs, Gene Ontology (GO) [including biological process (BP), molecular function (MF), and cellular component (CC)] enrichment analyses were conducted using the Database for Annotation Visualization and Integrated Discovery (DAVID) (https://david.ncifcrf.gov/home.jsp) (19). The Top 20 genes that interacted most closely with the DE_PRGs were predicted via GeneMANIA (http://genemania.org/search/) (20).
Development and validation of the PRG prognostic model
The prognostic values of DE_PRGs were determined by a univariate and multivariate Cox regression analysis u the “survival” and “survminer” R packages. Based on these prognostic DE_PRGs (P<0.05, both in the univariate and multivariate Cox regression analyses), we constructed the following model:
where Exp.(Genei) and Coef.(Genei) are the expression of Genei in a particular patient, and coefficients of the Genei in the multivariate cox regression analysis.
The risk score for each TCGA patient was calculated, and patients were then categorized into the low- or high-risk subgroups based on the median value. To observe the distribution of patients’ survival status in different risk subgroups, risk curves were plotted. We also reduced the dimension of the sample through a principal component analysis (PCA). Using the “pheatmap” R package, we visualized the gene expression and clinical traits of the samples. Simultaneously, by plotting Kaplan-Meier survival curves with the help of the “survival” and “survminer” R packages, we evaluated whether the predictive power of the model was statistically significant (Log rank P<0.05). Additionally, a time-dependent ROC curve (using the “survivalROC” R package) was used to assess the predictive effects of this model at different endpoints (0.5, 1, 2, 3, 4, or 5 years). External validation was performed in 2 separate cohorts of diffuse glioma patients from the CGGA database based on the median risk score in TCGA cohort. To confirm the independent prognostic value of the novel risk signature, we executed univariate and multivariate Cox regression analysis on training and validation cohorts respectively.
Gene set enrichment analysis (GSEA)
To better elucidate the molecular mechanisms of the prognostic signature, a GSEA (21) was conducted to examine discrepancies, related pathways, and molecular mechanisms in the high- and low-risk subgroups in TCGA cohort. A GSEA was performed in javaGSEA v.4.1.0, and the selected gene sets were “c5.go.bp.v7.4”, “c5.go.cc.v7.4”, “c5.go.mf.v7.4”, and “c2.cp.kegg.v7.4”. A |NES| >1 and a P<0.05 were considered statistically significant.
ssGSEA
According to the RNA-sequencing data, Immune Cell Abundance Identifier (ImmuCellAI) (22) (http://bioinfo.life.hust.edu.cn/ImmuCellAI#!/), a Web tool for assessing the abundance of immune cells (including 18 T-cell subtypes and 6 other immune cell types) in each sample, was used in both TCGA and the CGGA cohorts. We also visualized the results with the “ggplot2” and “ggsignif” R packages to examine the differences in the immune microenvironments between the high- and low-risk subgroups.
The construction of ceRNA network
The starBase v2.0 database (http://starbase.sysu.edu.cn/) provides the highly credible information of interactions between mRNA, microRNA (miRNA), and long non-coding RNA (lncRNA) based on high-throughput crosslinking-immunoprecipitation sequencing (CLIP-Seq) data (23). Thus, we obtained the information of interactions between miRNAs, lncRNAs, IL18 and CASP3 via starBase v2.0. Meanwhile, Cytoscape v3.8.0 software was used to visualize the ceRNA network.
Protein levels of prognostic signature
The Human Protein Atlas Database (HPA; www.proteinatlas.org) provides access to the protein-expression profiles of 32 human tissues and immunohistochemical results that accurately locate the protein in each tissue and organ (24). HPA was used to explore the protein-expression levels of the prognostic signatures in normal, and low- and high-grade glioma tissues.
Statistical analysis
All the statistical analyses and visualizations were carried out using R v3.6.1 software. Missing survival information and other missing clinical information are represented as not available (NA). To compare the OS time of patients between the groups, we employed the Kaplan-Meier method with a 2-sided log-rank test. Additionally, univariate and multivariate Cox regression models were used to assess the prognostic values of genes. The T-test was used between two groups for parameter tests, and an analysis of variance was used between multiple groups for the parameter tests.
Results
Identification of DE_PRGs in normal and tumor tissues
To better describe the process of our study, a flow diagram was constructed (see Figure 1). TCGA cohort comprised 625 samples from 620 diffuse glioma patients. RNA-sequencing data and clinical information from 325 and 693 patients in the CGGA cohorts were included in a further study (see Table 1). By comparing 477 TCGA-LGG and 148 TCGA-GBM samples to 207 GTEx-Brain samples, we identified 4575 and 5704 DEGs (|Log2FoldChange| >1 & adj. P<0.05; see Figure 2A,2B). After intersecting DEGs with 33 PRGs (see Figure 2C), 4 DE_PRGs were idented, including CASP3, IL18, gasdermin B (GSDMB), NLR family pyrin domain containing 1 (NLRP1), among which CASP3 and IL18 were upregulated and GSDMB and NLRP1 were downregulated in diffuse glioma tissues. Using DAVID, we explored the enrichment of GO (BP, MF and CC) in DE_PRGs and found that they were all located in the cytoplasm. Notably, CASP3 and NLRP1 are key molecules in the process of apoptosis, and IL18 and NLRP1 are mostly involved in the inflammatory response (see Figure 2D). The top 20 genes that had obvious interaction relationships with the DE_PRGs are shown in Figure 2E.
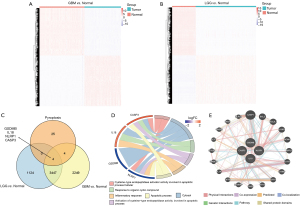
Prognostic value of DE_PRGs and the construction of predictive models for OS in TCGA cohort
First, we conducted a univariate Cox regression analysis (see Figure 3A), and found that CAPS3 and IL18 were high-risk genes, while GSDMB was a low-risk gene. Notably, the result of the multivariate Cox regression analysis was only the same for CASP3 and IL18 (see Figure 3B). Additionally, higher grade tumors had higher mRNA expressions of CASP3 and IL18 (see Figure 3C). Thus, according to the coefficients of the multivariate Cox regression analysis, we constructed the following risk-score formula:
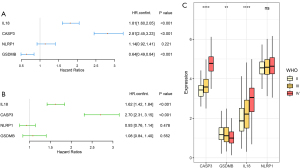
Based on the median risk score, 625 TCGA patients were categorized into the low- and high-risk subgroups (see Figure 4A). Patients in the high-risk subgroup had shorter survival times and more deaths than those in the low-risk subgroup (see Figure 4B). The PCA showed that patients at different risks could be further divided into two subgroups (see Figure 4C). A remarkable difference in OS time was observed between the two risk subgroups (P<0.0001; see Figure 4D). Patient risk scores and clinical traits are displayed in a heatmap (see Figure 4E), and we found that patients with IDH mutations tended to be in the high-risk subgroup. The time-dependent ROC curve indicates the excellent sensitivity and specificity of the prognostic model, while the areas under the curve (AUCs) for 0.5-, 1-, 2-, 3-, 4- and 5-year survival were 0.841, 0.856, 0.852, 0.855, 0.809, and 0.796, respectively (see Figure 4F). In addition, univariate and multivariate Cox regression analyses were performed in the TCGA cohort which showed the independent prognostic value of the 2-gene signature (P<0.001; see Table 2).
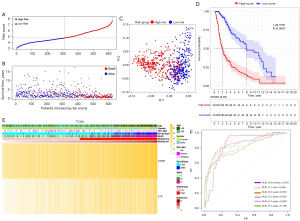
Table 2
Variable | Univariate Cox regression analysis | Multivariate Cox regression analysis | |||||||||||||||
---|---|---|---|---|---|---|---|---|---|---|---|---|---|---|---|---|---|
TCGA | CGGA693 | CGGA325 | TCGA | CGGA693 | CGGA325 | ||||||||||||
HR.confint | P value | HR.confint | P value | HR.confint | P value | HR.confint | P value | HR.confint | P value | HR.confint | P value | ||||||
Risk score | 1.81 (1.67–1.96) | <0.001 | 1.31 (1.15–1.49) | <0.001 | 1.18 (1.12–1.24) | <0.001 | 1.09 (1.04–1.14) | <0.001 | 1.54 (1.42–1.69) | <0.001 | 1.43 (1.29–1.58) | <0.001 | |||||
Age | 1.07 (1.06–1.08) | <0.001 | 1.03 (1.01–1.05) | <0.001 | 1.02 (1.01–1.03) | <0.001 | 1.02 (1.01–1.03) | <0.001 | 1.03 (1.02–1.04) | <0.001 | 1.01 (1.00–1.02) | 0.066 | |||||
Gender (male/female) | 1.24 (0.95–1.61) | 0.099 | 1.03 (0.71–1.49) | 0.886 | 1.06 (0.87–1.29) | 0.563 | 1.07 (0.87–1.32) | 0.506 | 0.94 (0.71–1.24) | 0.66 | 0.84 (0.63–1.12) | 0.238 | |||||
IDH_Mut (mutant-/wild-type) | 0.09 (0.05–0.17) | <0.001 | 0.25 (0.11–0.55) | 0.001 | 0.32 (0.26–0.40) | <0.001 | 0.38 (0.30–0.47) | <0.001 | 0.35 (0.26–0.47) | <0.001 | 0.64 (0.45–0.90) | 0.011 |
External validation in the CGGA cohorts
Based on the median risk score in TCGA cohort, patients from the CGGA693 cohort were assigned to the high- and low-risk subgroups (see Figure 5A). The distribution of survival status for each patient indicated that high-risk patients had a worse prognosis than low-risk patient (see Figure 5B) and the PCA result of CGGA693 cohort (see Figure 5C) showed that the risk model could divide diffuse glioma patients into two parts. As the Kaplan-Meier survival curve suggested, the median OS time of low-risk patients was significantly longer than that of high-risk patients (P<0.0001, see Figure 5D). The heatmap of the CGGA693 cohort showed that the patients with IDH wild-type or high-grade glioma were more likely to be in the high-risk subgroup (see Figure 5E). Additionally, the 0.5-, 1-, 2-, 3-, 4- and 5-year survival AUCs of the CGGA693 cohort were 0.688, 0.609, 0.69, 0.679, 0.693, and 0.703 (see Figure 5F). The same external validation was performed in CGGA325 cohort. Respectively, Figure 6A,6B showed the distribution of patients based on median risk score in TCGA cohort and the distribution of survival status for each patient of CGGA325 cohort. In the PCA result of CGGA325 cohort (see Figure 6C), it can be seen that the patients were divided into two different subgroups obviously. Furthermore, the Kaplan-Meier survival curve (see Figure 6D) and the heatmap of CGGA325 cohort (see Figure 6E) showed the similar results as TCGA and CGGA693 cohorts. Notably, the 0.5-, 1-, 2-, 3-, 4- and 5-year survival AUCs of the CGGA325 cohort (see Figure 6F) were 0.645, 0.717, 0.796, 0.805, 0.829 and 0.809 which shows the excellent predictive value of the prognostic model. Besides, the results of univariate and multivariate Cox regression analyses in both two CGGA cohorts confirmed the novel risk signature could be regarded as an independent prognostic factor (P<0.001; see Table 2).
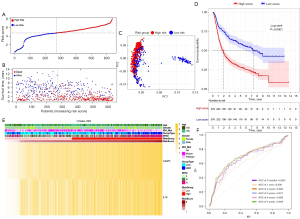
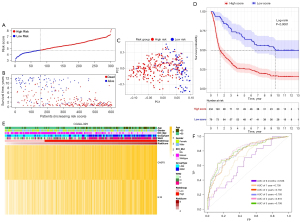
Functional analysis via GSEA in TCGA cohort
Based on the phenotypes (i.e., high- or low-risk) determined by the risk score, a GSEA was conducted to explore the relevant molecular mechanisms by applying the following criteria: |NES| >1 and P<0.05. The results showed that processes, such as the negative regulation of the immune processes, were enriched in the high-risk phenotypes, as were the proliferation, differentiation, and activation of immune cells, such as T cells (the unabridged GSEA results are shown in https://cdn.amegroups.cn/static/public/atm-21-6011-1.xlsx).
Comparison of immune cell infiltration between subgroups
Compared to the low-risk subgroup, the infiltration of immune cells in the high-risk subgroup was generally more obvious, especially in cytotoxic T cells, CD8+T cells, dendritic cells (DC), γδ T cells, macrophages, natural killer T cell (NKT), induced regulatory T cells (iTreg), naturally occurring regulatory T cells (nTreg) and IL-17-producing T helper (Th17) cells, which were increased in the high-risk subgroups of TCGA (see Figure 7A) and the CGGA693 (see Figure 7B) and the CGGA325 cohorts (see Figure 7C), while the abundance of B cells and Th1 cells was reduced.
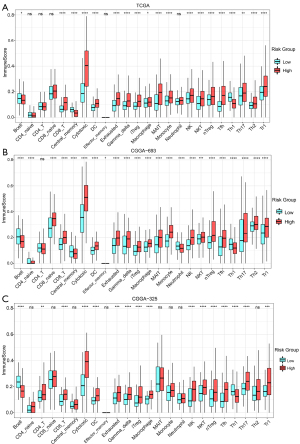
The ceRNA network associated with CASP3 and IL18
The ceRNA network were constructed with 36 miRNAs and 47 lncRNAs associated with CASP3 and IL18 (see Figure 8). The color of the strokes in the network represented the number of supporting CLIP-Seq experiments (clipExpNum). With the high clipExpNum, the interaction would be more credible.
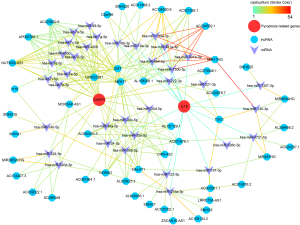
Immunohistochemical results of CASP3 and IL18
The results of the HPA showed that the protein expression of CASP3 and IL18 increased from normal brain to low-grade glioma and high-grade glioma samples (see Tables 3,4).
Table 3
No. | Image | Information |
---|---|---|
1 | ![]() |
Cerebral cortex, antibody: HPA002643, female, aged 45, cerebral cortex (T-X2020), normal tissue, NOS (M-00100), patient id: 1539 |
2 | ![]() |
Glioma, antibody: HPA002643, male, aged 42, brain (T-X2000), glioma, malignant, low grade (M-938031), patient id: 2878 |
3 | ![]() |
Glioma, antibody: HPA002643, male, aged 76, cerebral cortex (T-X2020), glioma, malignant, high grade (M-938033), patient id: 1589 |
Table 4
No. | Image | Information |
---|---|---|
1 | ![]() |
Cerebral cortex, antibody: CAB007772, male, aged 45, cerebral cortex (T-X2020), normal tissue, NOS (M-00100), patient id: 2521 |
2 | ![]() |
Glioma, antibody: CAB007772, male, aged 58, brain (T-X2000), glioma, malignant, low grade (M-938031), patient id: 156 |
3 | ![]() |
Glioma, antibody: CAB007772, male, aged 71, brain (T-X2000), glioma, malignant, high grade (M-938033), patient id: 3091 |
Discussion
In this study, an analysis of mRNA-sequencing data from TCGA and GTEx databases showed that 4 PRGs (i.e., IL18, CASP3, GSDMB, and NLRP1) were significantly differentially expressed between LGG and GBM tumor samples and normal tissue samples. After further evaluating the prognostic value of these 4 genes, univariate and multivariate Cox analyses showed that IL18 and CASP3 were highly correlated with the poor prognosis of diffuse glioma patients. Thus, based on IL18 and CASP3, we constructed a novel risk-score formula related to PRGs and validated it in two independent external cohorts of diffuse glioma patients in the CGGA database. The model showed remarkable predictive efficiency in relation to patients’ OS time. The functional analyses indicated that immune-related pathways were affected in the phenotype of the high- and low-risk subgroups. Further, we explored differences in immune cell infiltration between the high- and low-risk subgroups, and found that despite an increase in infiltration of most immune cells, patients’ prognoses were worse. Further, while patients in the high-risk subgroup had higher levels of cytotoxic T cells and dendritic cells, some immunosuppressive cells [e.g., iTreg, nTreg, and NKT cells (25,26)] also had higher infiltration levels, which indicate that the immune microenvironment of glioma patients is suppressed. In addition, we constructed a highly credible ceRNA network associated with IL18 and CASP3 for the further study.
IL-18, a proinflammatory cytokine produced by the cleavage of proIL-18 by CASP (cysteine-aspartic proteases,) particularly CASP1, was initially thought to promote interferon-gamma (IFN-γ) production in T-helper 1 (Th1) cells, especially in combination with IL-12. However, Th1 cells are not the only target of IL-18, which can also act on NK cells, NKT cells, B cells, DCs and macrophages to produce IFN-γ (27,28). Surprisingly, IL-18 promotes the polarization of Th1 or T-helper 2 (Th2), and its pleiotropy depends on underlying genetic factors and the surrounding cytokine environment (29). The abundance of Th2 cells was higher in the high-risk TCGA subgroup than the low-risk TCGA subgroup, and similar increases were observed in both the high-risk CGGA693 and CGGA325 subgroups. Th1 cells can participate in cell-mediated immunity and phagocyte-dependent inflammation by producing INF-γ, IL-2 and tumor necrosis factor beta (TNF-β), while Th2 cells, which can produce IL-4/-5/-6/-9/-10/-13, are involved in antibody responses, the inhibition of phagocytic cells, and the recruitment of eosinophil simultaneously (30). Our results possibly suggest that the maturation of Th1/Th2 cells is inhibited in the glioma immune environment, or the Th1 effect is transformed to the Th2 effect. Regardless of which occurs, both would be a disaster to the anti-tumor effects in glioma. Additionally, Shimato et al. (31) reported a Th2 bias in patients with malignant glioma, while Kumar et al. (32) found an imbalance of Th1/Th2 cytokines in brain tumor patients, which provide further evidence that the transformation of anti-tumor immunity to the Th2 effect is detrimental to patient prognosis. Pyroptosis results in the release of a large number of inflammatory factors, such as IL-18, and the recruitment of immune cells, which do not play an anti-tumor role, but lead to the suppression of the diffuse glioma immune microenvironment.
CASP3, which was traditionally considered to be a key molecule in the execution of apoptosis, has recently been found to be involved in pyroptosis. Unlike the traditional CASP1 pathway, CASP1 cleaves GSDME into GSDME-NT and -CT, while GSDME-NT forms pores in the cell membranes and leads to pyroptosis (10,33). Inducing apoptosis through the CASP3 pathway has been reported to inhibit glioma proliferation and migration (34,35). Thus, a higher expression of CASP3 can improve the prognosis of patients; however, the high expression of CASP3 in TCGA and the CGGA sample cohorts in our study was associated with poor prognosis. Thus, further consideration should be given to the role of the CASP3 pathway in pyroptosis for diffuse glioma patients. Unfortunately, to date, no existing studies have reported on this aspect.
In summary, our study showed that pyroptosis was closely related to the progression of diffuse glioma. Pyroptosis has the effect of eliminating tumor cells; however, significantly upregulated PRGs in glioma patients indicate a poor prognosis. The inflammatory effects of pyroptosis lead to the recruitment of immune cells, but may also exacerbate the suppression of the immune microenvironment. It should be noted that in this study, we did not specifically explore the landscape of the inflammatory factors released by pyroptosis in diffuse glioma. The novel risk-score model based on the 2 PRGs remarkably predicted the OS time of diffuse glioma patients. We have identified a novel genetic marker for predicting the prognosis of diffuse glioma patients and have provided a perspective for future studies on the relationship between pyroptosis and the establishment of the glioma immune microenvironment.
Acknowledgments
We would like to express our sincere gratitude to Professor Libin Deng for his constant encouragement and technical guidance.
Funding: This work was supported by funding from the National Natural Science Foundation of China (No. 82060474, Xiaoli Tang); the Nanchang University Innovation and Entrepreneurship Training Program for College Students (No. 202110403006, Yize Zhang); the Provincial Postgraduate Innovation Special Fund Project, Jiangxi Province, China (No. YC2020-S047, Ning Su); and the Climbing Program for Postgraduate Education of Nanchang University (Weiming Lou, Ziqiang Zeng, Jianying Gao).
Footnote
Reporting Checklist: The authors have completed the STREGA reporting checklist. Available at https://dx.doi.org/10.21037/atm-21-6011
Conflicts of Interest: All authors have completed the ICMJE uniform disclosure form (available at https://dx.doi.org/10.21037/atm-21-6011). The authors have no conflicts of interest to declare.
Ethical Statement: The authors are accountable for all aspects of the work in ensuring that questions related to the accuracy or integrity of any part of the work are appropriately investigated and resolved. The study was conducted in accordance with the Declaration of Helsinki (as revised in 2013).
Open Access Statement: This is an Open Access article distributed in accordance with the Creative Commons Attribution-NonCommercial-NoDerivs 4.0 International License (CC BY-NC-ND 4.0), which permits the non-commercial replication and distribution of the article with the strict proviso that no changes or edits are made and the original work is properly cited (including links to both the formal publication through the relevant DOI and the license). See: https://creativecommons.org/licenses/by-nc-nd/4.0/.
References
- Ostrom QT, Bauchet L, Davis FG, et al. The epidemiology of glioma in adults: a "state of the science" review. Neuro Oncol 2014;16:896-913. [Crossref] [PubMed]
- Ho VK, Reijneveld JC, Enting RH, et al. Changing incidence and improved survival of gliomas. Eur J Cancer 2014;50:2309-18. [Crossref] [PubMed]
- Lin L, Cai J, Jiang C. Recent Advances in Targeted Therapy for Glioma. Curr Med Chem 2017;24:1365-81. [Crossref] [PubMed]
- Richardson LG, Choi BD, Curry WT. (R)-2-hydroxyglutarate drives immune quiescence in the tumor microenvironment of IDH-mutant gliomas. Transl Cancer Res 2019;8:S167-70. [Crossref] [PubMed]
- Kristensen BW, Priesterbach-Ackley LP, Petersen JK, et al. Molecular pathology of tumors of the central nervous system. Ann Oncol 2019;30:1265-78. [Crossref] [PubMed]
- Xu Y, Li R, Li X, et al. An Autophagy-Related Gene Signature Associated With Clinical Prognosis and Immune Microenvironment in Gliomas. Front Oncol 2020;10:571189. [Crossref] [PubMed]
- Chen Z, Wu T, Yan Z, et al. Identification and Validation of an 11-Ferroptosis Related Gene Signature and Its Correlation With Immune Checkpoint Molecules in Glioma. Front Cell Dev Biol 2021;9:652599. [Crossref] [PubMed]
- Fang Y, Tian S, Pan Y, et al. Pyroptosis: A new frontier in cancer. Biomed Pharmacother 2020;121:109595. [Crossref] [PubMed]
- Gong W, Shi Y, Ren J. Research progresses of molecular mechanism of pyroptosis and its related diseases. Immunobiology 2020;225:151884. [Crossref] [PubMed]
- Wang Y, Gao W, Shi X, et al. Chemotherapy drugs induce pyroptosis through caspase-3 cleavage of a gasdermin. Nature 2017;547:99-103. [Crossref] [PubMed]
- Xia X, Wang X, Cheng Z, et al. The role of pyroptosis in cancer: pro-cancer or pro-"host"? Cell Death Dis 2019;10:650. [Crossref] [PubMed]
- Jiang Z, Yao L, Ma H, et al. miRNA-214 Inhibits Cellular Proliferation and Migration in Glioma Cells Targeting Caspase 1 Involved in Pyroptosis. Oncol Res 2017;25:1009-19. [Crossref] [PubMed]
- Goldman MJ, Craft B, Hastie M, et al. Visualizing and interpreting cancer genomics data via the Xena platform. Nat Biotechnol 2020;38:675-8. [Crossref] [PubMed]
- GTEx Consortium. Human genomics. The Genotype-Tissue Expression (GTEx) pilot analysis: multitissue gene regulation in humans. Science 2015;348:648-60. [Crossref] [PubMed]
- Zhao Z, Zhang KN, Wang Q, et al. Chinese Glioma Genome Atlas (CGGA): A Comprehensive Resource with Functional Genomic Data from Chinese Glioma Patients. Genomics Proteomics Bioinformatics 2021;19:1-12. [Crossref] [PubMed]
- Karki R, Kanneganti TD. Diverging inflammasome signals in tumorigenesis and potential targeting. Nat Rev Cancer 2019;19:197-214. [Crossref] [PubMed]
- Wang B, Yin Q. AIM2 inflammasome activation and regulation: A structural perspective. J Struct Biol 2017;200:279-82. [Crossref] [PubMed]
- Man SM, Kanneganti TD. Regulation of inflammasome activation. Immunol Rev 2015;265:6-21. [Crossref] [PubMed]
- Huang da W. Sherman BT, Lempicki RA. Systematic and integrative analysis of large gene lists using DAVID bioinformatics resources. Nat Protoc 2009;4:44-57. [Crossref] [PubMed]
- Warde-Farley D, Donaldson SL, Comes O, et al. The GeneMANIA prediction server: biological network integration for gene prioritization and predicting gene function. Nucleic Acids Res 2010;38:W214-20. [Crossref] [PubMed]
- Subramanian A, Tamayo P, Mootha VK, et al. Gene set enrichment analysis: a knowledge-based approach for interpreting genome-wide expression profiles. Proc Natl Acad Sci U S A 2005;102:15545-50. [Crossref] [PubMed]
- Miao YR, Zhang Q, Lei Q, et al. ImmuCellAI: A Unique Method for Comprehensive T-Cell Subsets Abundance Prediction and its Application in Cancer Immunotherapy. Adv Sci (Weinh) 2020;7:1902880. [Crossref] [PubMed]
- Li JH, Liu S, Zhou H, et al. starBase v2.0: decoding miRNA-ceRNA, miRNA-ncRNA and protein-RNA interaction networks from large-scale CLIP-Seq data. Nucleic Acids Res 2014;42:D92-7. [Crossref] [PubMed]
- Uhlén M, Fagerberg L, Hallström BM, et al. Proteomics. Tissue-based map of the human proteome. Science 2015;347:1260419. [Crossref] [PubMed]
- Vandenberk L, Van Gool SW. Treg infiltration in glioma: a hurdle for antiglioma immunotherapy. Immunotherapy 2012;4:675-8. [Crossref] [PubMed]
- Tang B, Wu W, Wei X, et al. Activation of glioma cells generates immune tolerant NKT cells. J Biol Chem 2014;289:34595-600. [Crossref] [PubMed]
- Yasuda K, Nakanishi K, Tsutsui H. Interleukin-18 in Health and Disease. Int J Mol Sci 2019;20:649. [Crossref] [PubMed]
- Gracie JA, Robertson SE, McInnes IB. Interleukin-18. J Leukoc Biol 2003;73:213-24. [Crossref] [PubMed]
- Nakanishi K, Yoshimoto T, Tsutsui H, et al. Interleukin-18 regulates both Th1 and Th2 responses. Annu Rev Immunol 2001;19:423-74. [Crossref] [PubMed]
- Romagnani S. T-cell subsets (Th1 versus Th2). Ann Allergy Asthma Immunol 2000;85:9-18; quiz 18, 21.
- Shimato S, Maier LM, Maier R, et al. Profound tumor-specific Th2 bias in patients with malignant glioma. BMC Cancer 2012;12:561. [Crossref] [PubMed]
- Kumar R, Kamdar D, Madden L, et al. Th1/Th2 cytokine imbalance in meningioma, anaplastic astrocytoma and glioblastoma multiforme patients. Oncol Rep 2006;15:1513-6. [Crossref] [PubMed]
- Vaughan AT, Betti CJ, Villalobos MJ. Surviving apoptosis. Apoptosis 2002;7:173-7. [Crossref] [PubMed]
- Fan R, Wang H, Zhang L, et al. Nanocrystallized Oleanolic Acid Better Inhibits Proliferation, Migration and Invasion in Intracranial Glioma via Caspase-3 Pathway. J Cancer 2020;11:1949-58. [Crossref] [PubMed]
- Han W, Yu F, Wang R, et al. Valproic Acid Sensitizes Glioma Cells to Luteolin Through Induction of Apoptosis and Autophagy via Akt Signaling. Cell Mol Neurobiol 2021;41:1625-34. [Crossref] [PubMed]
(English Language Editor: L. Huleatt)