Identification of novel hub genes associated with lymph node metastasis of head and neck squamous cell carcinoma by completive bioinformatics analysis
Introduction
Head and neck squamous cell carcinomas (HNSCC) are the most common malignant tumors originating in the epithelial cells of the mucosal linings of the upper airway including the oral cavity, oropharynx, and larynx or hypopharynx, with about 600,000 new cases a year and 40–50% mortality (1,2). Although significant advances in treatment of HNSCC have been made, the recurrence rate is still high due to its anatomical location and biological characteristics (3). Occult metastases in lymph nodes are very easily spread following predictable pathways, which is a key pathological feature and a predictor of poor survival of HNSCC. Based on traditional biopsies or imaging-based diagnoses, several nomograms and indexes have been used to predict the occurrence of lymph node metastasis in HNSCC patients. However, most of them have limited accuracy and sensitivity. Therefore, determining the potential key biomarkers may help to further uncover the biological basis of lymph node metastasis in HNSCC and improve clinical therapy via potential therapeutic targets.
In consideration of the important role of lymph node metastasis in the progression of HNSCC, elucidating its mechanism is critical for facilitating the development of novel therapeutics and improving the patient survival rate (4). However, the molecular changes and mechanism of lymph node metastasis is largely unknown. Owing to increasing advances in microarray and next-generation sequencing technologies, novel therapeutic targets and prognostic biomarkers have been explored to develop treatment for various cancers in the past decade (5).
In this study, 2 datasets of messenger RNAs (mRNAs) expression of normal and tumor tissues in HNSCC were selected from the Gene Expression Omnibus (GEO) and differentially expressed genes (DEGs) were distinguished by the related R package (https://www.r-project.org/). Functional annotation analysis of DEGs was conducted to assess the functional pathways via Gene Ontology (GO) annotation and Kyoto Encyclopedia of Genes and Genomes (KEGG) pathway analyses. A protein-protein interaction (PPI) network of DEGs was constructed by Search Tool for the Retrieval of Interacting Genes/Proteins (STRING) and the Molecular Complex Detection (MCODE) of Cytoscape (https://apps.cytoscape.org/apps/mcode) was used to identify the hub genes. Moreover, the Gene Expression Profiling Analysis 2 (GEPIA2) database was used to analyze the expression differences, overall survival (OS), and disease-free survival (DFS) of hub genes to better understand the function of those genes. Immunohistochemistry (IHC) results from the Human Protein Atlas (HPA) and receiver operating characteristic (ROC) analysis showed that these hub genes had good diagnostic and prognostic value for HNSCC, and correlation of hub genes and immune infiltration was analyzed by Tumor Immune Estimation Resource (TIMER). In conclusion, we firstly identified AURKA, CCNB1, CKS1B, SERPINH1, and TGFBI as hub genes which were associated with lymph node metastasis in HNSCC by completive bioinformatics analysis. We presented the following article in accordance with the STREGA reporting checklist (available at https://dx.doi.org/10.21037/atm-21-5704).
Methods
Genomic data and DEGs identification
The workflow of the present study was shown in Figure 1. We selected 2 datasets (GES6631 and GES58911) from GEO (https://www.ncbi.nlm.nih.gov/geo/) using R package GEO query (6). The platform for GSE6631 was GPL8300, including 22 paired (from the same patient) samples of HNSCC. The platform for GSE58911 was GPL6244, containing 15 paired (from the same patient) samples. The DEGs between HNSCC tumor and normal tissues were identified using the Bioconductor Limma package (7). The DEGs were screened with |log2fold change| >2 and adjusted P value <0.05, and were listed in https://cdn.amegroups.cn/static/public/atm-21-5704-1.xlsx and https://cdn.amegroups.cn/static/public/atm-21-5704-2.xlsx. The study was conducted in accordance with the Declaration of Helsinki (as revised in 2013).
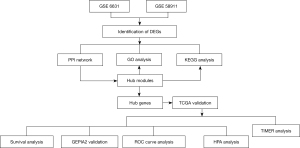
Functional annotation analysis of DEGs
The clusterProfiler R package was used to perform GO enrichment analysis and KEGG pathway enrichment analysis of DEGs. The P value <0.05 was considered statistically significant (8).
PPI network construction
A PPI network was built using the STRING database (version 10.5) and Cytoscape (3.8.2; https://manual.cytoscape.org/en/latest/) was used to visualize it. The MCODE plugin of Cytoscape was utilized to analyze the significant modules with parameter settings of degree cutoff =2, node score cutoff =0.2, k-core =2 and max. depth =100 (9).
Hub gene selection and validation
The Cytohubba plugin of Cytoscape was used to rank nodes in the PPI network, and the hub genes were selected through the 11 available computing methods in Cytohubba (10). The scores of selected genes were listed in the https://cdn.amegroups.cn/static/public/atm-21-5704-3.xlsx. Gene expression was validated using the GEPIA2 (https://gepia2.cancer-pku.cn/) online database (11). The IHC of the hub genes was validated from HPA (https://www.proteinatlas.org/). Moreover, ROC curves of hub genes were plotted using ‘pROC’ R package to evaluate whether those hub genes had good diagnostic and prognostic or not in GSE 6631and GSE 58911.
Survival analysis of hub genes
The GEPIA2 database was used to perform OS and DFS analysis based on the data from The Cancer Genome Atlas (TCGA) database. Statistical significance was considered when P<0.05 (12).
Correlation Analysis of hub gene and immune cell infiltration
TIMER (https://cistrome.shinyapps.io/timer/) (13), which included diverse types of cancer samples from the TCGA database, was used to analyze the correlation between the expression of hub genes and tumor-infiltrating immune cells (B cells, CD8+ T cells, CD4+ T cells, macrophages, neutrophils and dendritic cells).
Statistical analysis
Statistical analysis was performed by R packages. All data in the study were presented as mean ± standard deviation (SD). Differences between two groups were determined using Student’s t-test and the significance value was determined when P<0.05.
Results
Identification of DEGs in HNSCC
The original data of 2 datasets (GES 6631 and GES 58911) with a total of 37 pairs of normal and tumor samples were downloaded from GEO by using R package GEOquery. In total, 913 DEGs including 476 upregulated and 437 downregulated genes were identified after data processing and 24 DEGs were in both GES 6631 and GES 58911 (Figure 2). The DEGs were listed in https://cdn.amegroups.cn/static/public/atm-21-5704-1.xlsx and https://cdn.amegroups.cn/static/public/atm-21-5704-2.xlsx.
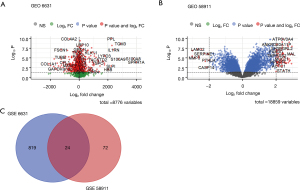
GO and KEGG pathway enrichment analysis
In order to analyze the biological function of DEGs, GO annotation and KEGG pathway enrichment analyses were conducted in the up- and downregulated DEGs by the clusterProfiler R package. The GO categories of molecular function (MF), biological process (BP), and cellular component (CC) for DEGs were significantly enriched, and the top 10 GO terms of the DEGs with upregulation and downregulation were presented. For BP, the upregulated DEGs were significantly enriched in response to extracellular matrix (ECM) organization, extracellular structure organization, external encapsulating structure organization, and the downregulated DEGs were significantly enriched in muscle system process, epidermis development, and epidermal cell differentiation. For MF, the upregulated DEGs were significantly enriched in ECM structural constituents, and ATPase activity and binding such as cadherin and growth factor, and the downregulated DEGs were significantly enriched in enzyme activity inhibition and actin filament binding. Concerning CC, the upregulated DEGs were significantly enriched in collagen-containing ECM and endoplasmic reticulum lumen, and the downregulated DEGs were significantly enriched in cell-cell junction, apical plasma membrane, and contractile fiber. We then used KEGG pathway analysis for further investigation of all DEGs. The upregulated DEGs were significantly enriched in the PI3K-Akt signaling pathway, focal adhesion, and ECM-receptor interaction, which were related to tumorigenesis and migration, and the downregulated DEGs were most significantly enriched in thyroid hormone signaling pathway, fatty acid degradation, and tyrosine metabolism (Figure 3).
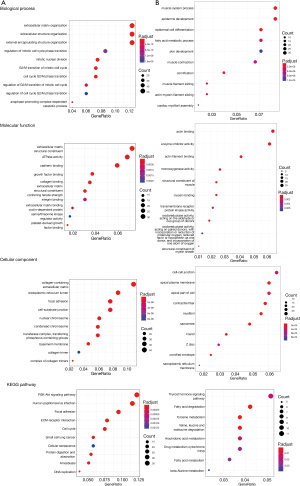
PPI interaction analysis
To demonstrate the molecular mechanisms that regulated HNSCC progression, the interactions among the DEGs were predicted using the STRING database with a combined score >0.9 at the protein level. Cytoscape was used to build the PPI network which included of 448 nodes and 1435 edges (Figure 4). The top 3 modules from the PPI network were selected after analysis of MCODE with the following default parameters: node score cut-off =0.2, cut-off =2, k-core =2, and max depth =100 (Figure 5).
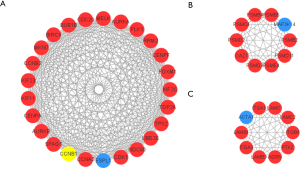
Hub genes selection associated with lymph node metastasis and analysis
Based on the results of the top 6 modules in MCODE analysis from PPI network, the 11 available computing methods in Cytohubba were used to screen hub genes synthetically. The scores of genes were listed in https://cdn.amegroups.cn/static/public/atm-21-5704-3.xlsx, and hub genes associated with lymph node metastasis were screened from GO and KEGG analysis (Table 1). Among these hub genes, AURKA, CCNB1, CKS1B, SERPINH1, and TGFBI were highly associated with lymph node metastasis in HNSCC, which had rarely been reported and were thus utilized for further analysis. The expression differences of hub genes between tumors and normal tissues were verified using GEPIA2. As shown in Figure 6, the expression levels of hub genes were significantly elevated in tumor samples, which was consistent with our results. Moreover, the protein levels of AURKA, CCNB1, CKS1B, SERPINH1, and TGFBI were significantly higher in tumor tissues compared with normal tissues on the HPA database (Figure 7).
Table 1
Pathway ID | Pathway description | Count | P value |
---|---|---|---|
GO:0030198 | Extracellular matrix organization | 37 | 2.1E-20 |
GO:0051301 | Cell division | 38 | 6.8E-13 |
GO:0005829 | Cytosol | 171 | 8.2E-24 |
GO:0005654 | Nucleoplasm | 132 | 1.9E-14 |
GO:0070062 | Extracellular exosome | 152 | 2.6E-20 |
GO:0005518 | Collagen binding | 13 | 4.2E-8 |
GO:0005515 | Protein binding | 324 | 7.9E-20 |
GO:0019901 | Protein kinase binding | 37 | 2.2E-11 |
hsa04512 | ECM-receptor interaction | 24 | 3.0E-16 |
hsa04510 | Focal adhesion | 34 | 8.5E-16 |
hsa04151 | PI3K-Akt signaling pathway | 38 | 5.5E-12 |
DEGs, differentially expressed genes.
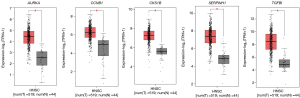
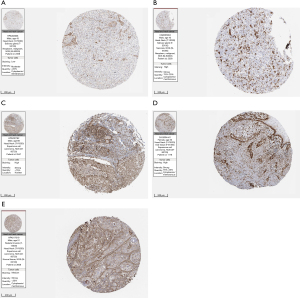
Survival analysis and ROC curve analyses of hub genes
To determine the survival of AURKA, CCNB1, CKS1B, SERPINH1, and TGFBI in HNSCC, OS and DFS were analyzed using the GEPIA2 database. As shown in Figure 8, the high expression level of AURKA, CCNB1, CKS1B, SERPINH1, and TGFBI in HNSCC patients showed worse OS. In addition, high expression of AURKA, CCNB1, CKS1B, SERPINH1, and TGFBI were associated with shorter DFS (Figure 9). These results indicated that AURKA, CCNB1, CKS1B, SERPINH1, and TGFBI might become potential biomarkers of recurrence and prognosis in HNSCC. Furthermore, ROC curves were performed to assess the diagnostic role in HNSCC. As shown in Figure 10 and Figure 11, AURKA [area under the curve (AUC) =92.769%/88.889%], CCNB1 (AUC =77.479%/86.667%), CKS1B (AUC =91.116%/89.333%), SERPINH1 (AUC =92.975%/90.667%), and TGFBI (AUC =86.157%/79.111%) were of diagnostic value (P<0.001) in GSE 6631and GSE 58911.
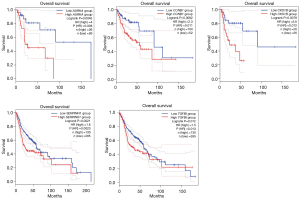
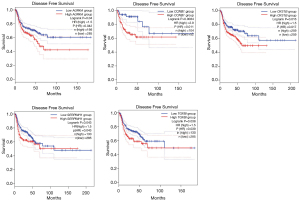
Correlation of hub genes’ expression with tumor purity and immune infiltration
Considering to the complex tumor microenvironment which included diverse types of tumor and immune cells, the Tumor Immune Estimation Resource (TIMER) was used to analyze the correlation of hub genes’ expression with tumor purity and immune infiltration. As a result, AURKA, CCNB1, CKS1B were positively associated with tumor purity while SERPINH1, and TGFBI were observed to be positively associated with CD4+ T cells, macrophages, neutrophils, and dendritic cells (Figure 12).
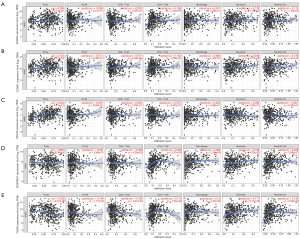
Discussion
The HNSCCs are the most common tumors worldwide. Although numerous advances have been made in the treatment of HNSCC, the prognosis has remained poor and it is therefore understood that lymph node metastasis indicated poor prognosis for HNSCC patients. Therefore, it is crucial to elucidate the molecular mechanism of lymph node metastasis for understanding of the disease progression to develop novel therapeutic targets. Due to the rapid development of high-throughput sequencing technology, bioinformatic analysis might contribute to identifying the DEGs and functional pathways involved in the lymph node metastasis of HNSCC.
In this study, 2 datasets were selected to identify the DEGs between HNSCC tissues and normal tissues. As a result, 913 DEGs including 476 upregulated and 437 downregulated genes were identified. Subsequently, GO/KEGG pathway enrichment analysis and PPI network construction were conducted to demonstrate interactions of the DEGs. In functional enrichment analysis conducted based on DEGs, we found that DEGs were significantly involved in ECM organization and enzyme activity inhibition. The ECM was a complex network of extracellular-secreted macromolecules which dealed with structural scaffolding and biochemical support of cells and tissues and was related with epithelial-to-mesenchymal transition (EMT) during tumor progression to promote tumor growth and metastasis (14). Alterations of ECM such as cancer associated fibroblasts (CAF) and tumor associated macrophages (TAM), which were involved with ECM receptors and markers, were shown to promote the development of lymphangiogenesis and lymph node metastasis. Enzyme activity inhibition revealed that dysregulation of the cell cycle progression and uncontrolled cell proliferation were the hallmarks of cancer and would promote lymph node metastasis of HNSCC (15).
Among the hub genes identified by bioinformatics analysis, AURKA, CCNB1, CKS1B, SERPINH1, and TGFBI were chosen for further analysis due to their relationship with lymph node metastasis, which had rarely been reported in HNSCC. The AURKA gene, also known as Aurora kinase A, was a member of the Aurora/Ipl1p family of cell cycle-regulating serine/threonine kinases which shared a highly conserved auto-phosphorylating sites. It played important roles in regulating cell division during mitosis and possesses oncogenic activity when it was overexpressed. Tong et al. showed that the expression of AURKA was considerably higher in esophageal squamous cell carcinoma (ESCC) than other normal tissues and the result was similar between ESCC samples and normal adjacent tissue, while the cancer cell migrating ability was suppressed when the expression of AURKA was knocked down using a small interfering RNA (siRNA) technique (16). Our results showed that AURKA was significantly high expressed in HNSCC samples compared to normal tissues, and there was a negative correlation between the expression of AURKA and prognosis. As a potential target for tumorigenesis and development, further work was necessary to demonstrate the molecular mechanism of AURKA.
Known as CyclinB1, CCNB1 was a vital member of the highly conserved cyclin family, which was expressed in almost all normal tissues to control cell mitosis rigorously. It can regulate and combine with cyclin-dependent kinase 1 (CDK1) to promote the transition of cell cycle from G2 phase to mitosis by phosphorylating related substances (17). The abnormal expression of CCNB1 in different cancers including breast cancer, lung cancer, and melanoma indicted its potential roles in cancer transformation and progression (18-20). Fang et al. showed that CCNB1 was activated by Chk1 and overexpressed in human colorectal cancer tissues, where CCNB1 low expression caused a strong G2/M phase arrest and impaired colorectal cancer proliferation in vitro and tumor growth in vivo (21). Liu et al. showed that CCNB1 could be activated by GTSE1 overexpression via changing the distribution of p53 in cytoplasm, which occurred with disease recurrence history, lymph node invasion, and progression in bladder cancer (22). Based on our GO terms and pathways analysis, CCNB1 appeared to participate in pathways of the growth of tumor cells. Thus, CCNB1 might be a new potential marker and prognostic factor of HNSCC, and its pathways might be potential treatment targets.
Also known as CDK regulatory subunit 1B, CKS1B belonged to the cyclin kinase subunit 1 (CKS1) protein family, which was conserved and played a key role in cell cycle regulation via tightly binding with CDK. It promoted cell growth, invasion, metastasis, and chemical resistance and was crucial for normal cell division and growth. High expression of CKS1B had been shown in many cancers, such as colon cancer, hepatocellular carcinoma, breast cancer, and lung cancer (23-26). Recently, studies had shown that CKS1B was closely associated with lymph node metastasis. Xu et al. found that lymph node metastasis and survival status were significantly associated with high expression of CKS1 and revealed that CKS1B was an independent poor prognostic biomarker in nasopharyngeal carcinoma (27). In our study, we showed that CKS1B was positively correlated in patients with HNSCC, indicating that CKS1B played an important role in progression and prognosis of HNSCC. However, the underlying molecular mechanisms still required clarification.
Serpin family H member 1 (SERPINH1) was required for the correct folding and secretion of collagen and could promote invasion and metastasis of cancer cells by regulating the expression of several ECM proteins (28). Tian et al. showed that SERPINH1 regulated EMT and cell metastasis via the Wnt/β-catenin signaling pathway in gastric cancer (29). Wang et al. found that silencing the expression of SERPINH1 inhibited the proliferation and migration of oral squamous cell carcinoma (OSCC) cells and caused cell cycle arrest at S phase (30). However, the role of SERPINH1 in HNSCC was not fully elucidated and further study is required.
Transforming growth factor-beta-induced protein (TGFBI) played an important role in tumorigenesis, cell proliferation, adhesion, migration, differentiation, and inflammation as an ECM protein composed of 683-amino acid with 4 conserved fasciclin-1 domains and a C-terminal Arg-Gly-Asp motif (31). It could improve tumor metastasis via promoting extravasation of colon cancer cells in the ECM (32), while it had been revealed as a tumor suppressor in mesothelioma and breast cancer (33). The high expression of TGFBI in HNSCC in our study demonstrated that TGFBI might have a different function of lymph node metastasis in HSNCC compared with other cancer and the underlying molecular mechanisms should be clarified.
After survival analysis, we confirmed that AURKA, CCNB1, CKS1B, SERPINH1, and TGFBI had good diagnostic and prognostic value of recurrence in HNSCC. The AUC of ROC curves in these 5 hub genes were all mostly above 75%, indicating that they had potential as biomarkers in prediction of HNSCC. Furthermore, the relationship between hub genes and immune infiltration in HNSCC proved that AURKA, CCNB1, CKS1B was positively associated with tumor purity in HNSCC, which identified that they were mainly expressed in HNSCC tumor cells, while SERPINH1 and TGFBI were positively related to the levels of CD4+ T cells, macrophages, neutrophils, and dendritic cells, which demonstrated that the high expression of SERPINH1 and TGFBI might have an advantage for cancer immunotherapy. Lots of work had proved that AURKA, CCNB1, CKS1B, SERPINH1, and TGFBI were valuable as therapeutic targets in different cancers. MLN8237, one of AURKA inhibitors, was proved to have synergistic effect to treat aggressive B-cell NHL with vincristine and rituximab and used in clinical trials (34). As a new and selective ubiquitin-like inhibitor, MLN4924 reduced the proliferation of CKS1B-overexpression cells which were resistant to bortezomib by inhibiting ubiquitin-like enzyme activating (35). Also, there were many drug targeting hub gens on the development, which would be potentially applied to clinical treatment in the future.
In conclusion, we successfully screened 5 hub genes related to lymph node metastasis of HNSCC using a series of bioinformatics analysis methods, which were shown to be potential biomarkers and therapeutic targets. However, further research was still necessary to demonstrate the function and mechanism of these hub genes.
Conclusions
In summary, our study identified 913 DEGs, consisting of 476 upregulated and 437 downregulated genes, and AURKA, CCNB1, CKS1B, SERPINH1, and TGFBI were selected as hub genes which were significantly enriched in signal pathways such as ECM or enzyme activity inhibition and might be closely related to lymph node metastasis in HNSCC. This study might help us better understand the development of HNSCC and provides potential diagnostic and/or prognostic biomarkers in HNSCC. However, more research would be required to verify the usefulness of these findings.
Acknowledgments
Funding: None.
Footnote
Reporting Checklist: The authors have completed the STREGA reporting checklist. Available at https://dx.doi.org/10.21037/atm-21-5704
Conflicts of Interest: All authors have completed the ICMJE uniform disclosure form (available at https://dx.doi.org/10.21037/atm-21-5704). The authors have no conflicts of interest to declare.
Ethical Statement: The authors are accountable for all aspects of the work in ensuring that questions related to the accuracy or integrity of any part of the work are appropriately investigated and resolved. The study was conducted in accordance with the Declaration of Helsinki (as revised in 2013).
Open Access Statement: This is an Open Access article distributed in accordance with the Creative Commons Attribution-NonCommercial-NoDerivs 4.0 International License (CC BY-NC-ND 4.0), which permits the non-commercial replication and distribution of the article with the strict proviso that no changes or edits are made and the original work is properly cited (including links to both the formal publication through the relevant DOI and the license). See: https://creativecommons.org/licenses/by-nc-nd/4.0/.
References
- Nakaminato S, Toriihara A, Makino T, et al. Prevalence of esophageal cancer during the pretreatment of hypopharyngeal cancer patients: routinely performed esophagogastroduodenoscopy and FDG-PET/CT findings. Acta Oncol 2012;51:645-52. [Crossref] [PubMed]
- Miller KD, Siegel RL, Lin CC, et al. Cancer treatment and survivorship statistics, 2016. CA Cancer J Clin 2016;66:271-89. [Crossref] [PubMed]
- Wang B, Wang T, Cao XL, et al. Critical genes in head and neck squamous cell carcinoma revealed by bioinformatic analysis of gene expression data. Genet Mol Res 2015;14:17406-15. [Crossref] [PubMed]
- Biau J, Lapeyre M, Troussier I, et al. Selection of lymph node target volumes for definitive head and neck radiation therapy: a 2019 Update. Radiother Oncol 2019;134:1-9. [Crossref] [PubMed]
- Vervoort Y, Linares AG, Roncoroni M, et al. High-throughput system-wide engineering and screening for microbial biotechnology. Curr Opin Biotechnol 2017;46:120-5. [Crossref] [PubMed]
- Davis S, Meltzer PS. GEOquery: a bridge between the Gene Expression Omnibus (GEO) and BioConductor. Bioinformatics 2007;23:1846-7. [Crossref] [PubMed]
- Ritchie ME, Phipson B, Wu D, et al. limma powers differential expression analyses for RNA-sequencing and microarray studies. Nucleic Acids Res 2015;43:e47 [Crossref] [PubMed]
- Yu G, Wang LG, Han Y, et al. clusterProfiler: an R package for comparing biological themes among gene clusters. OMICS 2012;16:284-7. [Crossref] [PubMed]
- von Mering C, Huynen M, Jaeggi D, et al. STRING: a database of predicted functional associations between proteins. Nucleic Acids Res 2003;31:258-61. [Crossref] [PubMed]
- Praneenararat T, Takagi T, Iwasaki W. Integration of interactive, multi-scale network navigation approach with Cytoscape for functional genomics in the big data era. BMC Genomics 2012;13:S24. [Crossref] [PubMed]
- Tang Z, Li C, Kang B, et al. GEPIA: a web server for cancer and normal gene expression profiling and interactive analyses. Nucleic Acids Res 2017;45:W98-W102. [Crossref] [PubMed]
- Robin X, Turck N, Hainard A, et al. pROC: an open-source package for R and S+ to analyze and compare ROC curves. BMC Bioinformatics 2011;12:77. [Crossref] [PubMed]
- Li B, Severson E, Pignon JC, et al. Comprehensive analyses of tumor immunity: implications for cancer immunotherapy. Genome Biol 2016;17:174. [Crossref] [PubMed]
- Paolillo M, Schinelli S. Extracellular Matrix Alterations in Metastatic Processes. Int J Mol Sci 2019;20:4947. [Crossref] [PubMed]
- Hanahan D, Weinberg RA. Hallmarks of cancer: the next generation. Cell 2011;144:646-74. [Crossref] [PubMed]
- Tong T, Zhong Y, Kong J, et al. Overexpression of Aurora-A contributes to malignant development of human esophageal squamous cell carcinoma. Clin Cancer Res 2004;10:7304-10. [Crossref] [PubMed]
- Krek W, Nigg EA. Differential phosphorylation of vertebrate p34cdc2 kinase at the G1/S and G2/M transitions of the cell cycle: identification of major phosphorylation sites. EMBO J 1991;10:305-16. [Crossref] [PubMed]
- Niméus-Malmström E, Koliadi A, Ahlin C, et al. Cyclin B1 is a prognostic proliferation marker with a high reproducibility in a population-based lymph node negative breast cancer cohort. Int J Cancer 2010;127:961-7. [Crossref] [PubMed]
- Yoshida T, Tanaka S, Mogi A, et al. The clinical significance of Cyclin B1 and Wee1 expression in non-small-cell lung cancer. Ann Oncol 2004;15:252-6. [Crossref] [PubMed]
- Kedinger V, Meulle A, Zounib O, et al. Sticky siRNAs targeting survivin and cyclin B1 exert an antitumoral effect on melanoma subcutaneous xenografts and lung metastases. BMC Cancer 2013;13:338. [Crossref] [PubMed]
- Fang Y, Yu H, Liang X, et al. Chk1-induced CCNB1 overexpression promotes cell proliferation and tumor growth in human colorectal cancer. Cancer Biol Ther 2014;15:1268-79. [Crossref] [PubMed]
- Liu A, Zeng S, Lu X, et al. Overexpression of G2 and S phase-expressed-1 contributes to cell proliferation, migration, and invasion via regulating p53/FoxM1/CCNB1 pathway and predicts poor prognosis in bladder cancer. Int J Biol Macromol 2019;123:322-34. [Crossref] [PubMed]
- Lee EK, Kim DG, Kim JS, et al. Cell-cycle regulator Cks1 promotes hepatocellular carcinoma by supporting NF-κB-dependent expression of interleukin-8. Cancer Res 2011;71:6827-35. [Crossref] [PubMed]
- Wang X, Xu J, Ju S, et al. Livin gene plays a role in drug resistance of colon cancer cells. Clin Biochem 2010;43:655-60. [Crossref] [PubMed]
- Zolota VG, Tzelepi VN, Leotsinidis M, et al. Histologic-type specific role of cell cycle regulators in non-small cell lung carcinoma. J Surg Res 2010;164:256-65. [Crossref] [PubMed]
- Slotky M, Shapira M, Ben-Izhak O, et al. The expression of the ubiquitin ligase subunit Cks1 in human breast cancer. Breast Cancer Res 2005;7:R737-44. [Crossref] [PubMed]
- Xu L, Fan S, Zhao J, et al. Increased expression of Cks1 protein is associated with lymph node metastasis and poor prognosis in nasopharyngeal carcinoma. Diagn Pathol 2017;12:2. [Crossref] [PubMed]
- Duarte BDP, Bonatto D. The heat shock protein 47 as a potential biomarker and a therapeutic agent in cancer research. J Cancer Res Clin Oncol 2018;144:2319-28. [Crossref] [PubMed]
- Tian S, Peng P, Li J, et al. SERPINH1 regulates EMT and gastric cancer metastasis via the Wnt/β-catenin signaling pathway. Aging (Albany NY) 2020;12:3574-93. [Crossref] [PubMed]
- Wang C, Wang Z, Zhang L, et al. MiR-29c inhibits the metastasis of oral squamous cell carcinoma and promotes its cell cycle arrest by targeting SERPINH1. Ann Transl Med 2021;9:1423. [Crossref] [PubMed]
- Kawamoto T, Noshiro M, Shen M, et al. Structural and phylogenetic analyses of RGD-CAP/beta ig-h3, a fasciclin-like adhesion protein expressed in chick chondrocytes. Biochim Biophys Acta 1998;1395:288-92. [Crossref] [PubMed]
- Ma C, Rong Y, Radiloff DR, et al. Extracellular matrix protein betaig-h3/TGFBI promotes metastasis of colon cancer by enhancing cell extravasation. Genes Dev 2008;22:308-21. [Crossref] [PubMed]
- Li B, Wen G, Zhao Y, et al. The role of TGFBI in mesothelioma and breast cancer: association with tumor suppression. BMC Cancer 2012;12:239. [Crossref] [PubMed]
- Mahadevan D, Stejskal A, Cooke LS, et al. Aurora A inhibitor (MLN8237) plus vincristine plus rituximab is synthetic lethal and a potential curative therapy in aggressive B-cell non-Hodgkin lymphoma. Clin Cancer Res 2012;18:2210-9. [Crossref] [PubMed]
- Huang J, Zhou Y, Thomas GS, et al. NEDD8 Inhibition Overcomes CKS1B-Induced Drug Resistance by Upregulation of p21 in Multiple Myeloma. Clin Cancer Res 2015;21:5532-42. [Crossref] [PubMed]
(English Language Editor: J. Jones)