Development of a novel miR-3648-related gene signature as a prognostic biomarker in esophageal adenocarcinoma
Introduction
Esophageal adenocarcinoma (EA) is an aggressive malignancy with high mortality (1). Patients with EA are often diagnosed at an advanced stage, which results in less than half of the patients being eligible for potentially curative treatment at diagnosis (2). Treatment is largely reliant upon minimally effective cytotoxic chemotherapy, with the 5-year overall survival (OS) rate for patients with advanced EA being lower than 20% (3). There are significant prognostic discrepancies in patients with the same TNM classification of malignant tumors (TNM stage) (4). To improve risk prediction, it is necessary to identify objective biomarkers to predict response to EA treatment and to predict survival.
Tumor cells can restrain anti-tumor immunity by forming an immunosuppressive microenvironment (5). Cells around the tumor cells called the tumor microenvironment (TME) are also involved in tumor progression and therapy response (6). Different populations of immune cells in TME can also affect the anti-tumor response, which becomes a major obstacle to the treatment of tumors (7). The tumor immune microenvironment of EA remains poorly understood and an improved understanding could be crucial to improving EA prognosis and treatment.
MicroRNAs (miRNAs) regulate many important cellular processes such as cell proliferation, migration, and apoptosis (8). Several cancer-associated miRNAs have now been identified, of which miRNA-3648 (miR-3648) is one of the most established and broad-acting (9-11). MiR-3648 is abnormally expressed in many cancers, including esophageal carcinoma (11). MiR-3648 could regulate cell growth by effecting APC2 levels, which is a tumor suppressor and a negative regulator of Wnt signaling (9). Dysregulation of miR-3648 can promote cell proliferation and induce the invasion and metastasis of cancer cells (10,12). However, the impact of miR-3648 on clinical characteristics and the immune microenvironment in EA patients is less well explored. A more in-depth understanding of the immune regulatory network regulated by miR-3648 may provide better prognostic information for EA.
In the present study, we utilized weighted gene co-expression network analysis (WGCNA) to construct a co-expression network of miR-3648-targeted genes. The infiltrated immune cells were evaluated with the CIBERSORT algorithm, and immune-related genes (IRGs) in the co-expression network were determined. A Cox analysis was then performed to select genes that were closely related to patients’ survival, and a multigene prognostic signature was constructed. Finally, we developed a nomogram to improve the ability to predict patient survival. We demonstrated that miR-3648 could play a critical role in the development of EA, and constructed a novel 4-gene signature that target by miR-3648 could serve as a prognosis biomarker in EA. We present the following article in accordance with the TRIPOD reporting checklist (available at https://dx.doi.org/10.21037/atm-21-6237).
Methods
Source of data
The patients used for this study included 12 women and 75 men aged 27 to 86 years old from The Cancer Genome Atlas (TCGA) database (https://cancergenome.nih.gov/). Clinical information and expression profiles of miRNAs/mRNAs associated with EA were retrieved from TCGA database (see available online: https://cdn.amegroups.cn/static/public/atm-21-6237-1.xlsx). The outcome was defined as any death recorded in TCGA clinical data. MiRNA data were extracted from 87 EA tumor tissues and 13 normal esophageal tissues. The mRNA-seq expression profiles of 54 normal esophageal tissues as controls were downloaded from the Genotype-Tissue Expression (GTEx) database (https://www.gtexportal.org/). Finally, the expression data of 19835 genes in 87 tumor samples and 67 normal samples were summarized as mRNA data. The study was conducted in accordance with the Declaration of Helsinki (as revised in 2013).
Differential expression analysis and target gene prediction
The R package limma was used to identify mRNAs that differed in expression between tumor and normal samples (13). For differentially expressed mRNA identification, we used a false discovery rate (FDR)-adjusted P value of <0.05 with a simultaneous absolute value of >1 for logFC.
To identify the target genes of miR-3648, 12 databases (Microt4, miRWalk, mir-bridge, miRanda, miRDB, miRMap, Pictar2, PITA, MiRNAMap, RNAhybrid, RNA22, and TargetScan) in miRWalk2.0 (http://zmf.umm.uni-heidelberg.de/apps/zmf/mirwalk2/) were employed. Genes that appeared in at least 3 databases were identified as the target genes of miR-3648.
A differentially expressed target gene (DETG) of miR-3648 was defined as any gene that was cross-linked to the target genes of miR-3648 or the differentially expressed genes (DEGs) in EA.
Evaluation of immune cell infiltration
The deconvolution algorithm CIBERSORT (https://cibersort.stanford.edu/) was applied with 100 permutations and leukocyte signature matrix 22 (LM22) to quantify the proportions of 22 infiltrating immune cell types in tumor and normal samples (14). CIBERSORT P values reflect the statistical significance of the results, and in this study unmatched samples that had P>0.05 were removed.
WGCNA
The R package “weighted gene co-expression network analysis” (15) was utilized to identify modules of highly correlated DETGs in EA tumor samples as previously described (16). Briefly, paired Pearson correlation was used to evaluate the weighted co-expression relationships among the subjects from all datasets in the adjacency matrix. A soft threshold of β=4 was selected, and the co-expression similarity matrix was changed to the adjacency matrix. Next, a topological overlap matrix (TOM) was built based on the adjacency matrix. Different modules were identified from TOM-based dissimilarity as densely interconnected gene clusters. Dynamic hybrid tree-cutting was then used to create a hierarchical clustering tree for dividing modules.
The infiltration fractions of immune cells in EA tumor tissues were employed as clinical traits. Pearson’s correlation test was then used to evaluate the module correlations with clinical traits. Among these clinical traits, the genes of the most significant module were determined to be IRGs for subsequent analysis.
Identification of candidate prognostic genes
To identify key prognostic genes, a Cox regression analysis was applied to estimate the correlation between the expression of the genes of the most significant module and the OS time of patients. Finally, four statistically significant genes for which P was <0.05 were identified as candidate prognostic genes.
Construction of the prognostic signature
A prognostic signature was constructed based on the expression levels of the candidate genes. The risk score (RS) for each tumor sample was calculated using the following formula:
In the above formula, Coefi represents the coefficient of Cox regression of gene i. Expi represents the expression level of gene i, and n represents the number of genes included in the prognostic signature. To determine whether this RS could independently predict the prognosis of EA patients, univariate and multivariate Cox regression analyses were conducted. The median RS was then used as a cut-off for deciding high- or low-risk patients.
Establishment of the nomogram
A nomogram was established by incorporating all significant prognostic characteristics and the RS to estimate the probability of the 1-, 3-, and 5-year OS of EA. The calibration curve was visualized to measure the prediction performance of the nomogram. We also calculated Harrell’s concordance index (C-index) to assess the survival prediction capability of the nomogram. R package rms v6.0-2 was employed to construct the nomogram.
Statistical analysis
The area under the curve (AUC) of the receiver operating characteristic (ROC) curve and Kaplan-Meier (K-M) curve analyses were performed to evaluate the prognostic performance and diagnostic capacity. The Wilcoxon test was applied to estimate the differences between groups. All R program packages for statistical analysis were implemented on the R program (v4.0.4). P<0.05 was considered statistically significant.
Results
Upregulation of miR-3648 and its target genes in EA
MiR-3648 was expectedly more highly expressed in tumor tissues than in normal tissues (P<0.001; Figure 1A) and showed excellent discrimination between tumor tissue and normal tissue (AUC =0.935; P=4.03e-15; Figure 1B). We also compared the expression of miR-3648 between different clinical feature groups, but no significant difference was observed (Figure 1C-1H). Based on the 12 databases of miRWalk2.0, 12,289 potential target genes of miR-3648 were identified. We identified 608 genes in at least 3 databases as the targets of miR-3648.
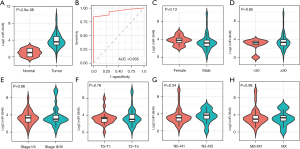
A total of 5,746 DEGs were identified, including 2,817 upregulated genes and 2,929 downregulated genes (Figure 2A). Furthermore, we cross-linked the 608 target genes of miR-3648 and DEGs, and identified 170 DETGs (Figure 2B).
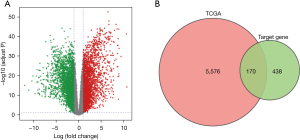
The landscape of infiltrating immune cells of EA
We applied the CIBERSORT algorithm to calculate the infiltration fraction of 22 immune cell types. Our results showed that M0 macrophages, M1 macrophages, activated dendritic cells, activated mast cells, activated memory CD4 T cells, follicular helper T cells, and regulatory T cells (Tregs) were the most common immune cell populations in EA tumor tissue. By contrast, resting dendritic cells, resting mast cells, monocytes, activated natural killer cells (NK cells), resting memory CD4 T cells, and naïve CD4 T cells were the most common immune cell populations in normal tissue (Figure 3A). We further evaluated the associations among the expression of miR-3648 and the infiltrating immune cells. The high expression group of miR-3648 was found to promote more infiltrating NK cells as well as memory CD4 T cells (Figure 3B).
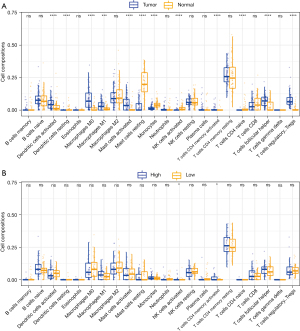
Identification of IRGs
The 170 DETGs were then considered for the WGCNA. We selected β=4 to satisfy the scale-free network law. In the network analysis, 2 gene modules (denoted “turqoise” and “blue”) and 37 oligogenes were identified under the parameter settings minModuleSize =20 and mergeCutHeight =0.25 (Figure 4A). The turquoise module contained 70 genes, and the blue module contained 63 genes. Subsequently, the infiltration fraction of the 13 immune cells in samples were examined as a clinical trait in order to assess the associations between them and gene modules (Figure 4B). Turquoise exhibited the most significant negative correlation with Treg infiltration (cor =−0.48; P=3e-6). Activated mast cells and activated NK cells appeared to be negatively correlated with the turquoise module. Turquoise was also related to resting dendritic cells. The 70 genes in the turquoise module were therefore identified as IRGs.
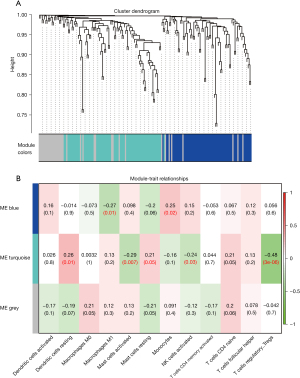
Construction of the 4-gene signature
Through a Cox regression analysis, the prediction performance of 70 IRGs on patients’ OS was evaluated. Four genes (C10orf55, DLL4, PANX2, and PANX2) that were closely related to OS were identified (Table 1). Then, the RS was calculated by multiplying these 4 expression values by the Cox coefficients. The formula for calculating the RS was: RS = 0.29 × expression of C10orf55 + 0.39 × expression of DLL4 + 0.15 × expression of PANX2 + 0.16 × expression of NKAIN1.
Table 1
Gene symbol | Gene full name | Coefficient | HR (95% CI) | P value |
---|---|---|---|---|
C10orf55 | Chromosome 10 putative open reading frame 55 | 0.29 | 1.3 (1.1–1.7) | 0.017 |
DLL4 | Delta-like canonical Notch ligand 4 | 0.39 | 1.5 (1.1–2.0) | 0.018 |
PANX2 | Pannexin 2 | 0.15 | 1.2 (1.0–1.3) | 0.030 |
NKAIN1 | Na+/K+ transporting ATPase interacting 1 | 0.16 | 1.2 (1.0–1.4) | 0.046 |
HR, hazard ratio; CI, confidence interval.
The median RS was 9.04. The 87 patients were separated into 43 high-risk patients and 44 low-risk patients. Low-risk patients had longer survival times than high-risk patients (P=0.0012; Figure 5A). The AUCs of the RS for 1-, 3-, and 5-year survival prediction were 0.740, 0.717, and 0.622, respectively (Figure 5B).
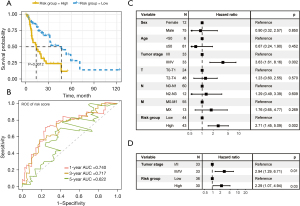
Independent prognostic analysis of the 4-gene signature
We used univariate Cox regression analysis to evaluate the prognostic performance of the RS and clinical characteristics (sex, age, TNM stage, and classification). The univariate Cox regression analysis results revealed that the RS [hazard ratio (HR) =2.71 in the high-risk group; P=0.002] and the TNM stage (stage III/IV; HR =3.63; P=0.002) were correlated with patients’ survival (Figure 5C). TNM stage was therefore considered for the multivariate Cox regression analysis to evaluate the prognostic performance of the RS. Multivariate Cox analysis suggested that the RS could be considered an independent prognostic marker [HR =2.29 in the high-risk group; 95% confidence interval (CI): 1.07–4.94; P=0.03] (Figure 5D).
Nomogram analysis
Based on the TNM stage and the RS, nomograms were constructed using the Cox regression model to predict the probability of death at 1, 3, and 5 years (Figure 6). Based on the RS (C-index =0.661) and TNM stage (C-index =0.630), the nomograms proved to be good predictors. Our optimal survival prediction nomogram was built by combining the RS and TNM stage. According to the nomogram, OS had a C-index of 0.698.
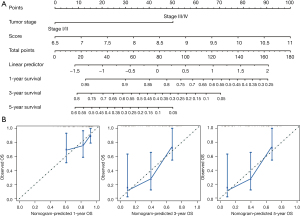
Discussion
EA is a typical immunogenic malignant tumor. Because the TME in EA is highly heterogeneous, it is not predictable which patient will respond best to treatment. Therefore, it is essential to screen prognostic markers that are related to EA’s immune microenvironment. In this study, we found that miR-3648 was significantly upregulated in EA tumor tissue compared with normal tissue and identified 4 miR-3648-targeted genes related to the TME. A multigene signature for EA was then developed. This signature may be used as a potential prognostic biomarker in EA patients.
MiR-3468 is abnormally expressed and has been implicated in many kinds of cancer (10,11). A previous study suggested that miR-3648 can induce the proliferation of prostate cancer cells (12). Upregulated miR-3648 was reported to induce the invasion and metastasis of human bladder cancer (10). EA tumor tissue expresses a higher level of miR-3648 than normal tissue, indicating a positive clinical effect, which is in line with previous studies. Furthermore, the high expression of miR-3648 was related to the infiltration of activated NK cells and activated CD4 cells. This finding suggests that miR-3648 may play a crucial role in the progression of EA. Despite we demonstrated that miR-3648 was related to the outcome of EA patients, the mechanisms of miR-3648 in EA should be further explored in vitro experiments.
We applied the CIBERSORT algorithm to predict immune cell infiltration levels to better understand the complexity of the EA tumor immune microenvironment. We found that M0 macrophages, M1 macrophages, activated dendritic cells, activated mast cells, activated memory CD4 T cells, follicular helper T cells, and Tregs were the most common immune cell populations in EA tumor tissues. The IRGs targeted by miR-3648 most related to Tregs were further identified. Tregs are an immunosuppressive subset of CD4+ T cells and can suppress anticancer immunity, thus promoting tumor development and progression (17). The correlation of miR-3648 with the tumor immune microenvironment may be a prominent mechanism in the pathological process of EA.
Based on the 170 DEGs targeted by miR-3648, we identified 70 IRGs using the WGCNA algorithm. Four genes were identified as being associated with patient survival based on a Cox regression analysis. Previous reports indicate that these genes are associated with different types of cancer in various ways. For example, C10orf55 has been found to be a potential biomarker to predict the relapse of acute myeloid leukemia (18). DLL4 plays a key role in tumor angiogenesis, functioning as a negative regulator of tumor angiogenesis and is upregulated in the tumor vasculature (19,20). A previous study indicated that DLL4 could be an independent prognostic factor for predicting the OS of patients with non-small cell lung cancer and was correlated with immunocyte infiltration (21). PANX2 is a channel-forming glycoprotein and is associated with many common diseases, including cancers (22). PANX2 was reported as a growth regulator in glioma cells (23) and was associated with the survival of clear cell renal cell carcinoma patients (24). NKAIN1 was overexpressed in urinary sediments from patients with prostate cancer and could be a promising biomarker for the early diagnosis of prostate cancer (25). In our study, all these genes were suggested as potentially useful biomarkers for predicting survival outcomes in EA. Future, in vitro and in vivo experiments are needed to determine their role in the proliferation, metastasis, and invasion of tumor EA cells.
We constructed an IRG-based 4-gene (C10orf55, DLL4, PANX2, and NKAIN1) prognostic signature. By combining this signature with the TNM stage, a nomogram was developed which was extremely accurate in predicting a patient’s prognosis. The novel immune-related prognostic signature may serve as a prognostic biomarker for EA and may reflect changes in immune cell infiltration in the TME. It may also help in individualized diagnosis and treatment.
Some limitations should be noted here. First, the study included only DETGs of miR-3648, so the prognostic signature does not reflect all EA transcription. Second, the associations between the 4 genes and the biological mechanisms of EA were not clarified in this study. Finally, as a retrospective study, our results should be confirmed in prospective multicenter clinical trials.
In summary, using WGCNA and CIBERSORT, we evaluated the tumor immune microenvironment of EA versus normal tissue and identified 70 miR-3648-targeted genes involved in immune cell infiltration in EA. Subsequently, a novel 4-gene signature was identified (C10orf55, DLL4, PANX2, and NKAIN1). A nomogram based on the signature and the TNM stage showed promising predictive performance for EA survival and may enable individualized treatment.
Acknowledgments
The authors appreciate the academic support from the AME Esophageal Cancer Collaborative Group.
Funding: This study was supported by the Project of Wu Jieping Medical Foundation (320.6750.19092.42).
Footnote
Reporting Checklist: The authors have completed the TRIPOD reporting checklist. Available at https://dx.doi.org/10.21037/atm-21-6237
Conflicts of Interest: All authors have completed the ICMJE uniform disclosure form (available at https://dx.doi.org/10.21037/atm-21-6237). The authors have no conflicts of interest to declare.
Ethical Statement: The authors are accountable for all aspects of the work in ensuring that questions related to the accuracy or integrity of any part of the work are appropriately investigated and resolved. The study was conducted in accordance with the Declaration of Helsinki (as revised in 2013).
Open Access Statement: This is an Open Access article distributed in accordance with the Creative Commons Attribution-NonCommercial-NoDerivs 4.0 International License (CC BY-NC-ND 4.0), which permits the non-commercial replication and distribution of the article with the strict proviso that no changes or edits are made and the original work is properly cited (including links to both the formal publication through the relevant DOI and the license). See: https://creativecommons.org/licenses/by-nc-nd/4.0/.
References
- Siegel RL, Miller KD, Fuchs HE, et al. Cancer Statistics, 2021. CA Cancer J Clin 2021;71:7-33. [Crossref] [PubMed]
- Smyth EC, Lagergren J, Fitzgerald RC, et al. Oesophageal cancer. Nat Rev Dis Primers 2017;3:17048. [Crossref] [PubMed]
- Cohen DJ, Leichman L. Controversies in the treatment of local and locally advanced gastric and esophageal cancers. J Clin Oncol 2015;33:1754-9. [Crossref] [PubMed]
- Markar SR, Gronnier C, Pasquer A, et al. Discrepancy Between Clinical and Pathologic Nodal Status of Esophageal Cancer and Impact on Prognosis and Therapeutic Strategy. Ann Surg Oncol 2017;24:3911-20. [Crossref] [PubMed]
- Hegde PS, Chen DS. Top 10 Challenges in Cancer Immunotherapy. Immunity 2020;52:17-35. [Crossref] [PubMed]
- McShane R, Arya S, Stewart AJ, et al. Prognostic features of the tumour microenvironment in oesophageal adenocarcinoma. Biochim Biophys Acta Rev Cancer 2021;1876:188598 [Crossref] [PubMed]
- Tamborero D, Rubio-Perez C, Muiños F, et al. A Pan-cancer Landscape of Interactions between Solid Tumors and Infiltrating Immune Cell Populations. Clin Cancer Res 2018;24:3717-28. [Crossref] [PubMed]
- Fabian MR, Sonenberg N. The mechanics of miRNA-mediated gene silencing: a look under the hood of miRISC. Nat Struct Mol Biol 2012;19:586-93. [Crossref] [PubMed]
- Rashid F, Awan HM, Shah A, et al. Induction of miR-3648 Upon ER Stress and Its Regulatory Role in Cell Proliferation. Int J Mol Sci 2017;18:1375. [Crossref] [PubMed]
- Sun W, Li S, Yu Y, et al. MicroRNA-3648 Is Upregulated to Suppress TCF21, Resulting in Promotion of Invasion and Metastasis of Human Bladder Cancer. Mol Ther Nucleic Acids 2019;16:519-30. [Crossref] [PubMed]
- Hu Y, Dingerdissen H, Gupta S, et al. Identification of key differentially expressed MicroRNAs in cancer patients through pan-cancer analysis. Comput Biol Med 2018;103:183-97. [Crossref] [PubMed]
- Xing R. miR-3648 Promotes Prostate Cancer Cell Proliferation by Inhibiting Adenomatous Polyposis Coli 2. J Nanosci Nanotechnol 2019;19:7526-31. [Crossref] [PubMed]
- Ritchie ME, Phipson B, Wu D, et al. limma powers differential expression analyses for RNA-sequencing and microarray studies. Nucleic Acids Res 2015;43:e47 [Crossref] [PubMed]
- Newman AM, Liu CL, Green MR, et al. Robust enumeration of cell subsets from tissue expression profiles. Nat Methods 2015;12:453-7. [Crossref] [PubMed]
- Langfelder P, Horvath S. WGCNA: an R package for weighted correlation network analysis. BMC Bioinformatics 2008;9:559. [Crossref] [PubMed]
- Yang Z, Zi Q, Xu K, et al. Development of a macrophages-related 4-gene signature and nomogram for the overall survival prediction of hepatocellular carcinoma based on WGCNA and LASSO algorithm. Int Immunopharmacol 2021;90:107238 [Crossref] [PubMed]
- Nishikawa H, Sakaguchi S. Regulatory T cells in tumor immunity. Int J Cancer 2010;127:759-67. [PubMed]
- Walker CJ, Mrózek K, Ozer HG, et al. Gene expression signature predicts relapse in adult patients with cytogenetically normal acute myeloid leukemia. Blood Adv 2021;5:1474-82. [Crossref] [PubMed]
- Liu Z, Fan F, Wang A, et al. Dll4-Notch signaling in regulation of tumor angiogenesis. J Cancer Res Clin Oncol 2014;140:525-36. [Crossref] [PubMed]
- Perez-Fidalgo JA, Ortega B, Simon S, et al. NOTCH signalling in ovarian cancer angiogenesis. Ann Transl Med 2020;8:1705. [Crossref] [PubMed]
- Li R, Liu X, Zhou XJ, et al. Identification and validation of the prognostic value of immune-related genes in non-small cell lung cancer. Am J Transl Res 2020;12:5844-65. [PubMed]
- Penuela S, Harland L, Simek J, et al. Pannexin channels and their links to human disease. Biochem J 2014;461:371-81. [Crossref] [PubMed]
- Lai CP, Bechberger JF, Naus CC. Pannexin2 as a novel growth regulator in C6 glioma cells. Oncogene 2009;28:4402-8. [Crossref] [PubMed]
- Kim KM, Hussein UK, Bae JS, et al. The Expression Patterns of FAM83H and PANX2 Are Associated With Shorter Survival of Clear Cell Renal Cell Carcinoma Patients. Front Oncol 2019;9:14. [Crossref] [PubMed]
- Leyten GH, Hessels D, Smit FP, et al. Identification of a Candidate Gene Panel for the Early Diagnosis of Prostate Cancer. Clin Cancer Res 2015;21:3061-70. [Crossref] [PubMed]