Identification of key genes associated with esophageal adenocarcinoma based on bioinformatics analysis
Introduction
Esophageal cancer (EC) is an aggressive malignancy and accounts for the majority of cancer-related deaths worldwide (1,2). The disease mainly includes 2 epidemiological and pathologically different subtypes: esophageal squamous cell carcinoma (ESCC) and esophageal adenocarcinoma (EAC) (3). ESCC is the most common and the dominant subtype in Asians, while EAC has a higher incidence in Western countries (4). Currently, the 5-year survival rate of EAC is less than 20% unless diagnosed and treated in the early stage (5). Additionally, surgical therapy, which can significantly improve prognosis, is not suitable for patients with advanced-stage cancer (6). Therefore, novel key genes and possible molecular mechanisms associated with the initiation, progression, and prognosis of EAC may provide a more effective approach to the early diagnosis and subsequent clinical decision making for personalized treatment and then improved survival.
The occurrence and development of EAC are closely related to alcohol and tobacco addiction, obesity, and gastroesophageal reflux, but the specific carcinogenic mechanism remains unclear (3). Barrett’s esophagus (BE), which involves the specialized small intestinal metaplastic epithelium of the esophagus, is a precursor to EAC (7,8). Previous studies have identified a series of significantly mutated genes in the progress of BE and EAC, such as TP53, CDKN2A, SMAD4, ARID1A, and PIK3CA (9,10). DNA hypermethylation in the promoter regions of genes has also been observed in BE and EAC (8,11). A study conducted by Wu et al. in 2013 identified several progressively altered-expressed microRNAs (miRNAs) of malignant progression in BE and EAC (12), and a recent study using high-throughput sequencing analysis revealed that genes and pathways involved in EAC were associated with DNA replication, cell cycle, and fatty acid degradation signaling pathways (13).
Although the research on the genes and molecular mechanisms of EAC has increased in recent years, a comprehensive picture of its genes and regulation is still lacking. With the development of genomic microarrays and high-throughput sequencing technologies, bioinformatics analysis is gradually becoming a prevailing tool for the exploration of cancer-related biomarkers and molecular mechanisms (13,14). Weighted gene co-expression network analysis (WGCNA) is a novel, systematic, bioinformatics method for selecting co-expression modules of related genes and the critical module associated with clinical traits, and may provide a novel means to exploring the potential biomarkers that could be used in the early diagnose and individual treatment of cancer (15). In this study, EAC microarray datasets from The Cancer Genome Atlas (TCGA) and the Gene Expression Omnibus (GEO) databases were analyzed to identify differentially expressed genes (DEGs) of EAC. Protein-protein interaction (PPI) and WGCNA were then applied in the identification of the key genes closely associated with the development of EAC. Moreover, patients’ clinical information and RNA-sequencing of DEGs from TCGA database were used for univariate cox regression analysis, lasso regression analysis, and multivariate cox regression analysis to establish a risk score prognostic model. We present the following article in accordance with the reporting recommendations for tumor MARKer (REMARK) reporting checklist (available at https://dx.doi.org/10.21037/atm-21-4015).
Methods
Data acquisition and preprocessing
The gene expression profiles based on RNA-sequencing and relevant clinical data of EAC patients were downloaded from TCGA (https://www.cancer.gov/tcga) data repository (16). The level of gene expression was measured and standardized by R package “DEseq2” (The R Foundation for Statistical Computing) (17).
To increase the robustness of our study, we searched for publicly available studies and samples in the GEO (https://www.ncbi.nlm.nih.gov/geo/) that met the following conditions for analysis: (I) the gene expression data series contained EAC and normal tissue samples; (II) the number of samples in every data series was more than 30; (III) the species was Homo sapiens. Finally, 2 gene expression profiles (GSE13898 and GSE26886) were identified for further analysis (18). The maximum value of expression of the genes was considered as the gene expression level if multiple probes corresponded to the same gene (19). The flow diagram of this study is shown in Figure 1. The study was conducted in accordance with the Declaration of Helsinki (as revised in 2013).
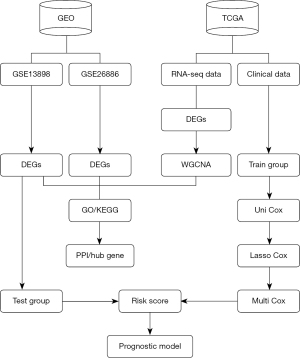
Identification of DEGs
R package “DEseq2” was used to identify DEGs in TCGA database (17), while R package “limma” was used for GEO datasets (20). Thresholds of |log2FC| >1.0 and an adjusted P value of <0.05 were selected.
Construction of a gene co-expression network (WGCNA)
The “WGCNA” package in R was used to construct a gene co-expression network of DEGs identified from TCGA data, and the analysis was performed according to the package instructions (15). The scale independence and average connectivity analysis of modules was performed by gradient test, and an appropriate power value was selected when the scale independence value was equal to 0.9. A WGCNA algorithm was then used to construct the co-expression network. A network with co-expression weight >2 was considered as candidate network, and genes with high absolute correlations were clustered into the same modules by cutting the clustering tree into branches. Only when the number of genes exceeded 30 was a module defined, and modules with higher correlation were merged (r<0.25). To visualize the results, different colors was assigned to each module.
Identification of cancer-related modules and genes
We used the module eigengene (ME) method to identify modules which were related with the clinical traits of EAC in TCGA data. The ME could represent the gene expression profiles of a module. The module-trait relationships (MTRs) were measured by linking the MEs to the clinical traits, and MTRs were then used to select significant clinical modules for in-depth analysis. Modules with an |MTRs| >0.5 were considered as cancer-related modules. Moreover, we took the intersection of the DEGs in the GSE13898 and GSE26886 datasets and those in the cancer-related modules and defined these as cancer-related genes.
Gene Ontology (GO) and pathway enrichment analysis
GO enrichment analysis identified which GO terms were over or underrepresented within a given set of genes, consisting of molecular function (MF), cellular components (CC), and biological processes (BP) (21); meanwhile, the KEGG database was used to identify functional and metabolic pathways (22). To explore the potential biological themes and pathways of cancer-related genes, we used the “clusterprofiler” package in R to annotate and visualize GO terms and KEGG pathways.
PPI network construction and module analysis
The Search Tool for The Retrieval of Interaction Genes (STRING, Zurich, Switzerland; https://string-db.org/) was used to construct the PPI network (23). Cancer-related genes were mapped to STRING to evaluate the PPI information with a confidence score >0.4 as the cutoff standard, which was then visualized using Cytoscape software (24). The key genes in the PPI were selected using five methods in CytoHubba plug-in, including edge percolated component (EPC), maximal clique centrality (MCC), maximal neighborhood component (MNC), degree (node connect degree) and closeness (node connect closeness). The intersection of top 15 genes identified by five methods was then taken to acquire the key genes in PPI analysis. In addition, Molecular Complex Detection (MCODE) was used to identify the finest clusters of PPI (25).
Survival analysis
The consensus survival analysis for EAC (OSeac) online survival analysis tool (http://bioinfo.henu.edu.cn/EAC/EACList.jsp) was applied to calculate Kaplan-Meier (K-M) survival curves with hazard ratio (HR) and log-rank tests of key genes in the PPI analysis (26).
Immune infiltration analysis
The Tumor Immune Estimation Resource (TIMER; http://timer.cistrome.org/) algorithm is a comprehensive online resource for the systematic analysis of immune infiltrates across various cancer types (27). In this study, we performed TIMER to determine the relationship between key gene expression in EAC and 6 immune infiltrates (B cells, CD4+ T cells, CD8+ T cells, neutrophils, macrophages, and dendritic cells).
Construction of a prognostic risk score model
A total of 154 patients with overall survival (OS) data were selected for further survival analysis. The clinical data of the GSE13898 dataset was provided by Professor Wang in Henan University. To give the established prognostic model better generalization ability, we identified the data from TCGA database as a training group (79 samples) and GSE13898 as a test group (75 samples). The training dataset was used to build the prognostic risk score model and validate it using the test dataset. To do this, we first took the intersection of the DEGs in the GSE13898 dataset and the genes in the cancer-related modules of TCGA analysis as candidate genes. Univariate Cox proportional hazards regression analysis was then used to identify key genes significantly associated with prognosis (P<0.05) (28). The collinearity between genes was eliminated through lasso regression analysis (29), and multivariate Cox proportional hazards regression analysis was performed to establish prognostic risk score model (30). The model used risk scores as predictors of prognostic status, with patients categorized into high- or low-risk groups according to the threshold of risk score. K-M survival curves were then plotted to evaluate the prediction effect of the model with log-rank test (P<0.05). The predictive performance of this model at different endpoints (1, 2, or 3 years) was assessed using a time-dependent receiver operating characteristic (ROC) curve (31), and the R packages “survival”, “glmnet”, “survminer”, and “survivalROC” were used in the construction of a prognostic risk score model.
Statistical analysis
R v4.1.1 was used to conduct data preprocessing, DEG screening, WGCNA analysis and functional annotation analysis. CytoHubba and MCODE in Cytoscape v3.7.0 was selected to mine key genes. The details of these bioinformatic analyses have been described in corresponding subsections. The potential diagnostic value of the prognostic risk score model was shown by ROC analysis using R v4.1.1. A P value <0.05 was considered statistically significant.
Results
Characteristics of selected datasets
The gene expression profiles based on RNA-sequencing were obtained from TCGA until April 2021. Studies from the GEO database up to April 2021 were also examined to increase the robustness of the study. Through layers of screening, we identified two datasets (GSE13898 and GSE 26886) in the GEO database that met the inclusion criteria, and the detailed characteristics of the selected datasets are summarized in Table 1. The data from TCGA were used to perform WGCNA analysis, and a risk score prognostic model was established using TCGA data and clinical information which was validated with the GSE13898 dataset.
Table 1
Datasets | Number (tumor) | Number (normal) | Total | Database |
---|---|---|---|---|
TCGA | 79 | 9 | 88 | TCGA |
GSE13898* | 75 | 28 | 103 | GEO |
GSE26886 | 21 | 19 | 40 | GEO |
*, clinical information of this dataset was provided by Qiang Wang, a professor from Henan university. TCGA, The Cancer Genome Atlas; GEO, Gene Expression Omnibus.
Identification of DEGs
After the quality of samples in each group was measured, there were 75 EAC samples and 28 normal samples in GSE13898, and 21 EAC samples and 19 normal samples in GSE26886. We then identified 807 and 3,128 significantly upregulated DEGs and 811 and 2,595 downregulated DEGs in GSE13898 and GSE26886, respectively. In TCGA dataset, which contained 79 EAC samples and 9 normal samples, 2,038 upregulated genes and 2,855 downregulated genes were identified. The volcano plots of GEO and TCGA samples are presented in Figure 2.
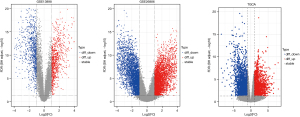
WGCNA of DEGs in TCGA
Clinical and RNA-sequencing data for 79 EAC and 9 normal samples were downloaded from TCGA database, and for module detection, a total of 4,893 DEGs were selected using R package “DEseq2” for further analysis. We first used the average linkage method and Pearson’s correlation coefficient to cluster a dendrogram of samples with clinical traits (Figure 3A), and co-expression analysis was then applied to construct the co-expression network. The connectivity between genes met a scale-free network distribution (scale-free R2=0.9) when the value of soft thresholding power β was set to 8 (Figure 3B). For cluster splitting, the minimum module size was set to 30, and the modules with a higher correlation were merged (r<0.25). Finally, 12 modules were identified through hierarchical clustering (Figure 3C), and a unique color was assigned to each module as an identifier (pink, blue, salmon, green-yellow, black, purple, green, brown, red, magenta, tan, and grey). The number of genes in modules ranged from 34 to 1,864.
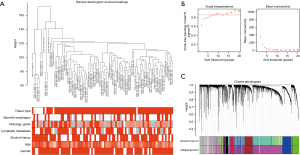
Identification of cancer-related modules and genes
To explain the gene expression variation, an ME was calculated that represented each module. We used the tissue type (EAC or normal) as the clinical phenotype to select the cancer-related modules for further analysis (Figure 4). Based on the criteria of |MTR| >0.5, we selected 5 modules as cancer-related modules for in-depth analysis: the blue module (606 genes), purple module (84 genes), brown module (524 genes), red module (178 genes), and magenta module (1,864 genes). In addition, we plotted a scatterplot of gene significance vs. module membership in each of the 5 modules (Figure S1).
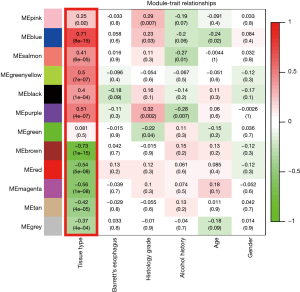
To identify the cancer-related genes, we took the intersection of the DEGs in the GSE13898 and GSE26886 datasets from the GEO database and the genes in 5 cancer-related modules from TCGA database. Finally, 190 cancer-related genes were identified (Figure 5).
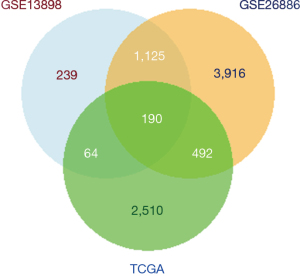
Enrichment analysis of cancer-related genes
GO and KEGG enrichment analysis were performed on the cancer-related genes identified in the above analysis. As illustrated in Figure 6, genes in the GO analysis were most relevant with the BP of extracellular matrix organization, the CC of complex of collagen-containing extracellular matrix, and the MF of extracellular matrix structural constituent. As shown in KEGG analysis, 15 pathways were significantly associated with cancer-related genes, including regulation of actin cytoskeleton, tight junction, protein digestion, and absorption, nuclear factor-kappa B (NF-κB) signaling pathway, and human papillomavirus infection (Figure 6).
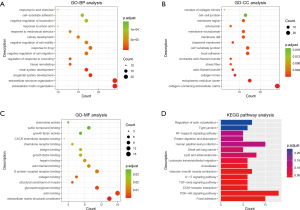
PPI network analysis of cancer-related genes
The PPI network was established by Cytoscape based on the STRING database and consisted of 152 nodes and 366 edges (Figure 7A), with MCODE in Cytoscape being used to perform module analysis. One module was found to be significant (MCODE =7.375) and consisted of 17 nodes and 59 edges (Figure 7B).
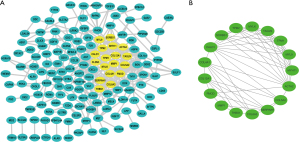
Identification of hub genes associated with EAC
The genes with a score in the top 15 according to all five methods in CytoHubba were identified as hub genes of EAC. Six genes that may play an important role in EAC progression were identified: actin alpha 2 (ACTA2), biglycan (BGN), caldesmon 1 (CALD1), collagen type I alpha 1 chain (COL1A1), collagen type IV alpha 1 chain (COL4A1), and decorin (DCN) (Table 2).
Table 2
Category | Rank methods in CytoHubba | ||||
---|---|---|---|---|---|
MNC | Closeness | Degree | MCC | EPC | |
1 | COL1A1* | COL1A1* | COL1A1* | ACTA2* | COL1A1* |
2 | TIMP1 | PTGS2 | TIMP1 | CALD1* | TIMP1 |
3 | ACTA2* | TIMP1 | PTGS2 | MYL9 | BGN* |
4 | BGN* | ACTA2* | ACTA2* | MYH11 | DCN* |
5 | THBS1 | THBS1 | DCN* | TPM2 | COL4A1* |
6 | MMP1 | DCN* | BGN* | MYLK | ACTA2* |
7 | PTGS2 | BGN* | THBS1 | TPM1 | THBS1 |
8 | DCN* | MMP1 | MMP1 | COL1A1* | MMP1 |
9 | COL4A1* | CALD1* | CALD1* | BGN* | COL5A2 |
10 | CALD1* | COL4A1* | COL4A1* | DCN* | COL12A1 |
11 | COL5A2 | GJA1 | COL5A2 | COL4A1* | CALD1* |
12 | COL12A1 | MET | MYLK | SERPINH1 | COL6A3 |
13 | MYL9 | MYH11 | COL12A1 | COL12A1 | FMOD |
14 | FMOD | FMOD | MET | COL6A3 | SERPINH1 |
15 | MYH11 | MYL9 | MYL9 | TAGLN | PTGS2 |
*, the overlap genes in top 15 by five ranked methods. EPC, edge percolated component; MCC, maximal clique centrality; MNC, maximal neighborhood component; Degree, node connect degree; Closeness, node connect closeness.
Survival analysis and immune infiltration analysis of hub genes
K-M plots made using OSeac demonstrated the prognostic impact of the 6 hub genes identified from PPI analysis, and the results demonstrated that high expression of ACTA2, CALD1, COLA1A, and COL4A1 was associated with poor OS in patients with EAC (P<0.05), as shown in Figure 8.
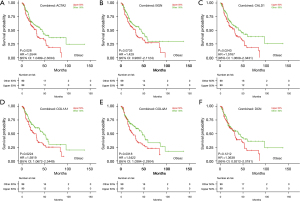
The TIMER database was used to assess the correlation between the expression of hub genes and immune infiltration (Figure 9), and a positive correlation between ACTA2 expression and the infiltration of B cells (Cor =0.179; P=1.63e-02), CD4+ T cells (Cor =0.293; P=6.74e-05), macrophage cells (Cor =0.582; P=1.05e-17), and dendritic cells (Cor =0.187; P=1.18e-02) was seen. The expression of BGN was positively related to the infiltration of CD4+ T cells (Cor =0.198; P=7.77e-03), macrophage cells (Cor =0.514; P=1.68e-13), and dendritic cells (Cor =0.238; P=1.27e-03), while CALD1 expression was positively associated with the infiltration of macrophage cells (Cor =0.605; P=2.44e-19) and dendritic cells (Cor =0.228; P=2.08e-03). COL1A1 expression was positively associated with the infiltration of macrophage cells (Cor =0.463, P=5.88e-11) and dendritic cells (Cor =0.152; P=4.23e-02), and COL4A1 expression was positively associated with the infiltration of macrophage cells (Cor =0.366; P=4.45e-07). The expression of DCN was positively associated with the infiltration of B cells (Cor =0.154; P=3.97e-02), CD4+ T cells (Cor =0.225; P=2.48e-03), macrophage cells (Cor =0.647; P=1.03e-22), and dendritic cells (Cor =0.159; P=3.31e-02).
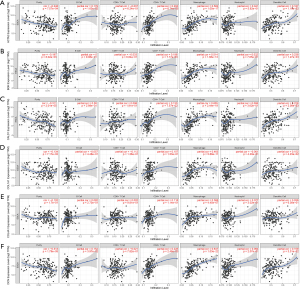
Construction of a prognostic risk score model
To establish an effective prognostic model for predicting the prognosis of EAC, univariate, multivariate Cox proportional hazards regression analysis and lasso regression analysis were employed to screen the genes. First, we identified 163 genes as candidate genes for this model by taking the intersection of the DEGs in the GSE13898 dataset and the genes in the cancer-related modules of the WGCNA (Figure S2). In the univariate Cox regression analysis, 14 genes significantly associated with prognosis were identified (P<0.05) (Table S1), while in lasso regression, when partial likelihood deviance was the smallest, 11 of the 14 genes had coefficients that were not 0 (Figure S3). Finally, a total of 6 genes were then obtained in multivariate Cox regression analysis to establish a prognostic risk score model: claudin-3 (CLDN3), erythrocyte membrane protein band 4.1 like 4A (EPB41L4A), endothelial cell specific molecule-1 (ESM1), metallothionein 1X (MT1X), progestin and adipoQ receptor family member 5 (PAQR5), and plasminogen activator urokinase (PLAU) (Figure 10A, Table S2). The risk score was calculated using the following formula: risk score = (0.5864 × CLDN3) + (0.5773 × ESM1) + (0.3891 × PLAU) + (−0.4981 × MT1X) + (−0.3769 × EPB41L4A) + (–0.2727 × PAQR5).
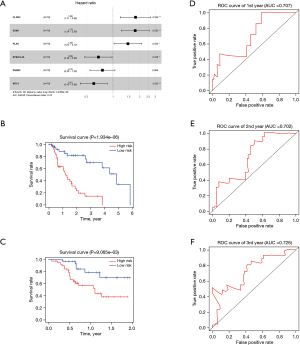
The K-M curves were grouped by defined risk scores (Figure S4), which indicated that the prognosis of the high-risk group was significantly poorer than that of the low-risk group in the training data (Figure 10B), as well as test data (Figure 10C). By predicting the survival of patients at 1, 2, and 3 years, the areas under the ROC curve (AUCs) obtained from the risk-based prediction model in the training data were 0.79, 0.888, and 0.889 (Figure S5), while in the test data, they were 0.707, 0.702, and 0.726 (Figure 10D-10F).
We also plotted scatter plots to illustrate the relationship between survival time and risk scores. As the risk scores increased, the duration of survival gradually decreased and the number of patient deaths gradually increased in the both training (Figure 11A) and test group (Figure 11B), which demonstrated the definition of “risk score” was effective. In the training data, the expression of CLDN3, ESM1, and PLAU genes were significantly higher in the high-risk group (P<0.05), while the EPB41L4A, MT1X, and PAQR5 genes were significantly highly expressed in the low-risk group (P<0.05), which were consistent with their coefficients in the risk score formula (Figure 11C). The nomogram of this risk score prognostic model is presented in Figure 11D.
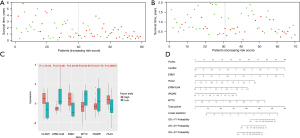
Discussion
EAC is a refractory type of cancer with high mortality due to its high metastasis rate, treatment resistance, and poor prognosis (3). Although many studies have been performed in recent years, the early diagnosis, effective treatment, and prognosis for EAC have not been well resolved, and it is essential to develop a better understanding of the molecular mechanisms involved in the occurrence and progression of the disease to explore potential targets for its diagnosis and treatment.
In this study, we identified 6 genes as hub genes which may play an important role in the initiation and development of EAC by integrating TCGA and GEO data and combining the WGCNA and PPI network analysis. WGCNA provides module construction and correlation analysis within gene expression data to determine the associations between genes (15), and the PPI network was based on protein networks reported in the known literature and used to explore the keys genes of specific diseases (23). This study has provided strong evidence for a novel method combining WGCNA and PPI for the identification of key genes. Abnormal expression levels of key genes have been found in various human malignant tumors and might become potential targets for the diagnosis and treatment of malignancy (32-35).
ACTA2 is a protein-coding gene generally expressed in smooth muscle cells and activated cancer-related fibroblasts; tumor cells may break away from a primary site and invade the surrounding tissue with the help of actin bundles (36). A study by Masszi et al. demonstrated that tumor growth factor beta (TGF-β)-elicited epithelial-mesenchymal transition (EMT) induced the expression of ACTA2, which then increased tumor invasion and worsened the prognosis of patients (37,38). It has recently been reported that the level of ACTA2 is considerably increased in ESCC tumors (39). BGN is a member of the family of small leucine-rich proteoglycans (SLRPs) which is strongly expressed in inflammatory and fibrotic tissue (40-42) and may act as an angiogenic factor by stimulating tumor endothelial cell migration in an autocrine manner through TLR2 and TLR4 (43). Caldesmon (CALD) is an actin- and myosin-binding protein family which is an essential component of the cytoskeleton in smooth muscle and non-muscle cells (44). As a member of this family, CALD1 is involved in the regulation of the endothelial cytoskeleton as well as migration, and p38 MAPK-mediated CALD phosphorylation may be involved in endothelial cytoskeletal remodeling (45). In humans, there are at least 28 different types of collagen proteins encoded by 44 collagen genes. The COL1A1 and COL4A1 are 2 of these genes and are essential in the extra cellular matrix (ECM), which is closely related to the biological behavior of tumor cells (46,47). A recent study has demonstrated that COL1A1 and COL4A1 are associated with several clinical parameters, including TNM staging, lymph node metastasis, and tumor invasion depth in gastric cancer patients (48). COL1A1 has been reported to be highly expressed in EC cells, and upregulation of COL1A1 has been associated with cell proliferation, invasion, and apoptosis (49). DCN, a small stromal proteoglycan, is a member of the SLRP gene family (50), and Bozoky et al. found that its expression is consistently decreased in the tumor microenvironment of various cancers (51). It has also been reported that the expression level of DCN is significantly decreased in EC tumor, suggesting it may serve as a key gene in the progression of EC (52). This information clarifies why the predicted genes, especially ACTA2, BGN, CALD1, and COL4A1 (not previously reported) are highly associated with the development of EAC, and these genes may act as potential biomarkers for its diagnosis and prognosis.
It is widely recognized that cyclooxygenase-2 (COX-2) and SRY-box transcription factor 2 (SOX2) are reliable biomarkers for EAC. COX-2 plays important roles in the induction of inflammation and tumorigenesis (53), and neoplastic progression of BE towards EAC is highly related to increased expression of COX-2 (54). Selective COX-2 inhibition downregulates COX-2 and MET proto-oncogene (MET) expression, which are both important molecules involved in EAC progression and dissemination (55). SOX2 is a transcription factor associated with cancer stem cells (CSCs) and embryonic stem cells, and is involved in the formation and differentiation of esophageal epithelium (56). SOX2 expression is lost during transition from BE to EAC, which is related to an increased risk of neoplastic progression (57). In addition, the pattern of p53 and particularly SOX2 protein expression in EAC predicts the response to neoadjuvant chemoradiotherapy (nCRT) (58). Compared with the above 2 biomarkers, those identified through our bioinformatic analysis seem less credible due to the lack of functional experiments. Therefore, further experimental studies to elucidate the expression, molecular mechanism, and prognostic role of the potential biomarkers are required.
A risk score prognostic model was established to predict the survival rate of patients with EAC and contained 6 key genes: CLDN3, EPB41L4A, ESM1, MT1X, PAQR5, and PLAU. While CLDN3, ESM1, and PLAU were found to be negative prognostic genes, EPB41L4A, MT1X, and PAQR5 was found to be positive.
CLDN3, as a member of the claudin (CLDN) gene family, is generally expressed on the epithelia of multiple tissue and is involved in the formation of intercellular tight junctions (59). Tight junctions, the most apical intercellular junctions, play vital roles in intercellular cell adhesion and the maintenance of tissue osmotic homeostasis. CLDN3 has additionally been revealed to serve as a receptor of Clostridium perfringens enterotoxin (CPE) (60). Moreover, the binding of CPE to CLDN3 has been shown to cause the proximal portion part of the CPE to interact with the cell membrane and form small cell membrane pores, resulting in increased cell membrane permeability, loss of cell osmotic balance, and ultimately cell death (61,62). It has also been reported that the expression level of CLDN3 is significantly increased in EAC tumor tissue, which is consistent with our results, and might be associated with the progression and poor prognosis of EAC (63). In addition, the CLDN3 gene had the largest coefficient (0.5864) in the risk score formula, indicating that it may serve as a very important prognostic biomarker in EAC.
ESM1, also called endocan, is an endothelial cell-related proteoglycan (64). Accumulated evidence has demonstrated that tESM1 plays an important role in the regulation of major process, such as cell adhesion, endothelial dysfunction, inflammatory disorders, and tumor progression (65). In addition, ESM1 is preferentially expressed in tumor endothelium, is dramatically overexpressed in many cancers, and has been shown to be directly involved in tumor progression (65,66). Cui et al. recently reported that ESM1 plays a tumor-driving role in EC and has the potential to become a biomarker for diagnosis and prognosis (67). However, the specific role and molecular mechanism of ESM1 in EAC requires further investigation.
PLAU is a member of plasminogen activator system and participates in various physiological and pathophysiological processes, such as cell proliferation, adhesion, migration, and other functions through the proteolytic system, intracellular signal transduction, and chemokine activation (68). Remarkably, a recent study performed by Fang et al. has revealed that PLAU could promote progression of ESCC tumor cell and that tumor cell-secreted PLAU could expedite the conversion of fibroblasts to inflammatory cancer-associated fibroblasts, accelerating the proliferation and migration of ESCC cells (69). Our study demonstrated that PLAU may serve as a prognostic biomarker of EAC, which warrants further exploration in future studies.
MT1X belongs to the metallothionein gene family (MTs), which encode a series of cysteine-rich proteins (70). MTs are involved in various BP, including metal homeostasis, DNA damage, oxidative stress, angiogenesis, apoptosis, cell differentiation, and carcinogenesis (71). It has been reported that abnormal overexpression of MT1X delayed the G1/S progression of cell cycle and promoted apoptosis by inactivating NF-κB signaling in hepatocellular carcinoma cells in vitro and suppressed tumor growth and lung metastasis in nude mice in vivo (72). We also found that MT1X had a relatively higher coefficient in the risk score formula. Taken together, this suggests MT1X may serve as a potential prognostic biomarker and may inhibit the progression and metastasis of EAC.
EPB41L4A belongs to the FERM band (four-point-one, ezrin, radixin, moesin) superfamily, members of which mainly form a group of membrane-associated proteins whose major biological functions are the regulation of cytoskeletal rearrangements, intracellular trafficking, and Wnt/β-catenin signaling (73,74). It has been revealed that the Wnt/β-catenin signaling pathway is prominently involved in intercellular adhesion and carcinogenesis (75). Recent cancer research suggests that a high expression of EPB41L4A is associated with better prognosis in multiple myeloma (MM), which has been hypothesized to result from the expression changes of genes involved in DNA replication (76). It is worth noting that EPB41L4A has not been reported in EAC at present, and further investigation is required to explore its important roles in the progression of this cancer.
PAQR5 is a member of the progestin and adipoQ receptor (PAQR) family, which encode functional receptors with a broad range of apparent ligand specificities (77). Until now, the role of in malignancy has not been extensively studied; one article in this area reported that high PAQR5 expression in endometrial cancer may be associated with good prognosis. The results of our study suggest that PAQR5 might have the potential to serve as a tumor suppressor gene of EAC (78).
Interestingly, we found that “points” can often show significant improvement as risk scores increase, as shown in the nomogram (Figure 11D), indicating that risk scores may have a greater impact on prognosis compared with clinical information. To the best of our knowledge, the 6-gene signature-related prognostic model and nomogram in our study have not been reported previously, and we believe this model has the potential to be a practical clinical tool for predicting the prognosis of patients with EAC.
However, this study has several limitations that should be noted. First, our study was mainly based on the data from the GEO and TCGA databases, in which most patients are White, African, or Latino, and caution must be taken when extending the findings to other ethnic groups. Second, due to the lack of basic experimental verification, the expression, molecular mechanism, and prognostic role of the genes at the protein level warrant further experimental studies. In addition, the mechanical analyses in our study were exclusively descriptive, and further functional experiments are needed to clarify the underlying mechanism of the genes. Finally, the amount of data included in our study is relatively small because of the low incidence of EAC, which may decrease the credibility and generalizability of the results.
Conclusions
This study identified 6 genes with the potential to become useful targets for the diagnosis and treatment of EAC, namely ACTA2, BGN, CALD1, COL1A1, COL4A1, and DCN. A risk score prognostic model based on the CLDN3, EPB41L4A, ESM1, MT1X, PAQR5, and PLAU genes was established to predict the survival rate of patients with EAC. The 6-gene-related risk score prognostic model and the nomogram based on it might be a reliable tool for predicting the prognosis of patients with EAC.
Acknowledgments
We are very grateful to Professor Qiang Wang (Henan University) for providing the original clinical data of GSE13898. We thank AME Editing Service (http://editing.amegroups.cn/#editing) for its linguistic assistance during the preparation of this manuscript.
Funding: This work was funded by the Key Research and Development Program of Shandong Province (2020CXGC011303), the National Major Scientific and Technological Projects for New Drug Discovery and Development (2012ZX09101103) and the Jinan Science and Technology Bureau (2019GXRC051).
Footnote
Reporting Checklist: The authors have completed the REMARK reporting checklist. Available at https://dx.doi.org/10.21037/atm-21-4015
Peer Review File: Available at https://dx.doi.org/10.21037/atm-21-4015
Conflicts of Interest: All authors have completed the ICMJE uniform disclosure form (available at https://dx.doi.org/10.21037/atm-21-4015). The authors have no conflicts of interest to declare.
Ethical Statement: The authors are accountable for all aspects of the work in ensuring that questions related to the accuracy and integrity of any part of the work are appropriately investigated and resolved. The study was conducted in accordance with the Declaration of Helsinki (as revised in 2013).
Open Access Statement: This is an Open Access article distributed in accordance with the Creative Commons Attribution-NonCommercial-NoDerivs 4.0 International License (CC BY-NC-ND 4.0), which permits the non-commercial replication and distribution of the article with the strict proviso that no changes or edits are made and the original work is properly cited (including links to both the formal publication through the relevant DOI and the license). See: https://creativecommons.org/licenses/by-nc-nd/4.0/.
References
- Chen W, Zheng R, Baade PD, et al. Cancer statistics in China, 2015. CA Cancer J Clin 2016;66:115-32. [Crossref] [PubMed]
- Siegel RL, Miller KD, Jemal A. Cancer statistics, 2020. CA Cancer J Clin 2020;70:7-30. [Crossref] [PubMed]
- Smyth EC, Lagergren J, Fitzgerald RC, et al. Oesophageal cancer. Nat Rev Dis Primers 2017;3:17048. [Crossref] [PubMed]
- Siewert JR, Ott K. Are squamous and adenocarcinomas of the esophagus the same disease? Semin Radiat Oncol 2007;17:38-44. [Crossref] [PubMed]
- Tramontano AC, Sheehan DF, Yeh JM, et al. The Impact of a Prior Diagnosis of Barrett's Esophagus on Esophageal Adenocarcinoma Survival. Am J Gastroenterol 2017;112:1256-64. [Crossref] [PubMed]
- Davies AR, Gossage JA, Zylstra J, et al. Tumor stage after neoadjuvant chemotherapy determines survival after surgery for adenocarcinoma of the esophagus and esophagogastric junction. J Clin Oncol 2014;32:2983-90. [Crossref] [PubMed]
- Coleman HG, Xie SH, Lagergren J. The Epidemiology of Esophageal Adenocarcinoma. Gastroenterology 2018;154:390-405. [Crossref] [PubMed]
- Kaz AM, Grady WM. Epigenetic biomarkers in esophageal cancer. Cancer Lett 2014;342:193-9. [Crossref] [PubMed]
- Dulak AM, Stojanov P, Peng S, et al. Exome and whole-genome sequencing of esophageal adenocarcinoma identifies recurrent driver events and mutational complexity. Nat Genet 2013;45:478-86. [Crossref] [PubMed]
- Fatehi Hassanabad A, Chehade R, Breadner D, et al. Esophageal carcinoma: Towards targeted therapies. Cell Oncol (Dordr) 2020;43:195-209. [Crossref] [PubMed]
- Yu M, Maden SK, Stachler M, et al. Subtypes of Barrett's oesophagus and oesophageal adenocarcinoma based on genome-wide methylation analysis. Gut 2019;68:389-99. [Crossref] [PubMed]
- Wu X, Ajani JA, Gu J, et al. MicroRNA expression signatures during malignant progression from Barrett's esophagus to esophageal adenocarcinoma. Cancer Prev Res (Phila) 2013;6:196-205. [Crossref] [PubMed]
- Nangraj AS, Selvaraj G, Kaliamurthi S, et al. Integrated PPI- and WGCNA-Retrieval of Hub Gene Signatures Shared Between Barrett's Esophagus and Esophageal Adenocarcinoma. Front Pharmacol 2020;11:881. [Crossref] [PubMed]
- Gao M, Kong W, Huang Z, et al. Identification of Key Genes Related to Lung Squamous Cell Carcinoma Using Bioinformatics Analysis. Int J Mol Sci 2020;21:2994. [Crossref] [PubMed]
- Langfelder P, Horvath S. WGCNA: an R package for weighted correlation network analysis. BMC Bioinformatics 2008;9:559. [Crossref] [PubMed]
- Zhu Y, Qiu P, Ji Y. TCGA-assembler: open-source software for retrieving and processing TCGA data. Nat Methods 2014;11:599-600. [Crossref] [PubMed]
- Love MI, Huber W, Anders S. Moderated estimation of fold change and dispersion for RNA-seq data with DESeq2. Genome Biol 2014;15:550. [Crossref] [PubMed]
- Edgar R, Domrachev M, Lash AE. Gene Expression Omnibus: NCBI gene expression and hybridization array data repository. Nucleic Acids Res 2002;30:207-10. [Crossref] [PubMed]
- Barrett T, Wilhite SE, Ledoux P, et al. NCBI GEO: archive for functional genomics data sets--update. Nucleic Acids Res 2013;41:D991-5. [Crossref] [PubMed]
- Ritchie ME, Phipson B, Wu D, et al. limma powers differential expression analyses for RNA-sequencing and microarray studies. Nucleic Acids Res 2015;43:e47. [Crossref] [PubMed]
- Ashburner M, Ball CA, Blake JA, et al. Gene ontology: tool for the unification of biology. The Gene Ontology Consortium. Nat Genet 2000;25:25-9. [Crossref] [PubMed]
- Kanehisa M, Goto S, Kawashima S, et al. The KEGG resource for deciphering the genome. Nucleic Acids Res 2004;32:D277-80. [Crossref] [PubMed]
- Szklarczyk D, Gable AL, Lyon D, et al. STRING v11: protein-protein association networks with increased coverage, supporting functional discovery in genome-wide experimental datasets. Nucleic Acids Res 2019;47:D607-13. [Crossref] [PubMed]
- Shannon P, Markiel A, Ozier O, et al. Cytoscape: a software environment for integrated models of biomolecular interaction networks. Genome Res 2003;13:2498-504. [Crossref] [PubMed]
- Bader GD, Hogue CW. An automated method for finding molecular complexes in large protein interaction networks. BMC Bioinformatics 2003;4:2. [Crossref] [PubMed]
- Wang Q, Yan Z, Ge L, et al. OSeac: An Online Survival Analysis Tool for Esophageal Adenocarcinoma. Front Oncol 2020;10:315. [Crossref] [PubMed]
- Li T, Fan J, Wang B, et al. TIMER: A Web Server for Comprehensive Analysis of Tumor-Infiltrating Immune Cells. Cancer Res 2017;77:e108-10. [Crossref] [PubMed]
- Lunn M, McNeil D. Applying Cox regression to competing risks. Biometrics 1995;51:524-32. [Crossref] [PubMed]
- Zhou D, Liu X, Wang X, et al. A prognostic nomogram based on LASSO Cox regression in patients with alpha-fetoprotein-negative hepatocellular carcinoma following non-surgical therapy. BMC Cancer 2021;21:246. [Crossref] [PubMed]
- Han S, Choi S, Nah S, et al. Cox regression model of prognostic factors for delayed neuropsychiatric sequelae in patients with acute carbon monoxide poisoning: A prospective observational study. Neurotoxicology 2021;82:63-8. [Crossref] [PubMed]
- Schemper M, Henderson R. Predictive accuracy and explained variation in Cox regression. Biometrics 2000;56:249-55. [Crossref] [PubMed]
- Cruceriu D, Baldasici O, Balacescu O, et al. The dual role of tumor necrosis factor-alpha (TNF-α) in breast cancer: molecular insights and therapeutic approaches. Cell Oncol (Dordr) 2020;43:1-18. [Crossref] [PubMed]
- Liang J, Li H, Han J, et al. Mex3a interacts with LAMA2 to promote lung adenocarcinoma metastasis via PI3K/AKT pathway. Cell Death Dis 2020;11:614. [Crossref] [PubMed]
- Song J, Zhao W, Lu C, et al. Spliced X-box binding protein 1 induces liver cancer cell death via activating the Mst1-JNK-mROS signalling pathway. J Cell Physiol 2020;235:9378-87. [Crossref] [PubMed]
- Zhang H, Qin G, Zhang C, et al. TRAIL promotes epithelial-to-mesenchymal transition by inducing PD-L1 expression in esophageal squamous cell carcinomas. J Exp Clin Cancer Res 2021;40:209. [Crossref] [PubMed]
- Lambrechts A, Van Troys M, Ampe C. The actin cytoskeleton in normal and pathological cell motility. Int J Biochem Cell Biol 2004;36:1890-909. [Crossref] [PubMed]
- Lee HW, Park YM, Lee SJ, et al. Alpha-smooth muscle actin (ACTA2) is required for metastatic potential of human lung adenocarcinoma. Clin Cancer Res 2013;19:5879-89. [Crossref] [PubMed]
- Masszi A, Di Ciano C, Sirokmány G, et al. Central role for Rho in TGF-beta1-induced alpha-smooth muscle actin expression during epithelial-mesenchymal transition. Am J Physiol Renal Physiol 2003;284:F911-24. [Crossref] [PubMed]
- Yazdian-Robati R, Ahmadi H, Riahi MM, et al. Comparative proteome analysis of human esophageal cancer and adjacent normal tissues. Iran J Basic Med Sci 2017;20:265-71. [PubMed]
- Babelova A, Moreth K, Tsalastra-Greul W, et al. Biglycan, a danger signal that activates the NLRP3 inflammasome via toll-like and P2X receptors. J Biol Chem 2009;284:24035-48. [Crossref] [PubMed]
- Fisher LW, Heegaard AM, Vetter U, et al. Human biglycan gene. Putative promoter, intron-exon junctions, and chromosomal localization. J Biol Chem 1991;266:14371-7. [Crossref] [PubMed]
- Mohan H, Krumbholz M, Sharma R, et al. Extracellular matrix in multiple sclerosis lesions: Fibrillar collagens, biglycan and decorin are upregulated and associated with infiltrating immune cells. Brain Pathol 2010;20:966-75. [Crossref] [PubMed]
- Yamamoto K, Ohga N, Hida Y, et al. Biglycan is a specific marker and an autocrine angiogenic factor of tumour endothelial cells. Br J Cancer 2012;106:1214-23. [Crossref] [PubMed]
- Huber PA. Caldesmon. Int J Biochem Cell Biol 1997;29:1047-51. [Crossref] [PubMed]
- Mirzapoiazova T, Kolosova IA, Romer L, et al. The role of caldesmon in the regulation of endothelial cytoskeleton and migration. J Cell Physiol 2005;203:520-8. [Crossref] [PubMed]
- Fang M, Yuan J, Peng C, et al. Collagen as a double-edged sword in tumor progression. Tumour Biol 2014;35:2871-82. [Crossref] [PubMed]
- Järveläinen H, Sainio A, Koulu M, et al. Extracellular matrix molecules: potential targets in pharmacotherapy. Pharmacol Rev 2009;61:198-223. [Crossref] [PubMed]
- Zhang QN, Zhu HL, Xia MT, et al. A panel of collagen genes are associated with prognosis of patients with gastric cancer and regulated by microRNA-29c-3p: an integrated bioinformatics analysis and experimental validation. Cancer Manag Res 2019;11:4757-72. [Crossref] [PubMed]
- Chen X, Sun H, Zhao Y, et al. CircRNA circ_0004370 promotes cell proliferation, migration, and invasion and inhibits cell apoptosis of esophageal cancer via miR-1301-3p/COL1A1 axis. Open Med (Wars) 2021;16:104-16. [Crossref] [PubMed]
- Järvinen TA, Prince S. Decorin: A Growth Factor Antagonist for Tumor Growth Inhibition. Biomed Res Int 2015;2015:654765. [Crossref] [PubMed]
- Bozoky B, Savchenko A, Guven H, et al. Decreased decorin expression in the tumor microenvironment. Cancer Med 2014;3:485-91. [Crossref] [PubMed]
- Augoff K, Grabowski K, Rabczynski J, et al. Expression of decorin in esophageal cancer in relation to the expression of three isoforms of transforming growth factor-beta (TGF-beta1, -beta2, and -beta3) and matrix metalloproteinase-2 activity. Cancer Invest 2009;27:443-52. [Crossref] [PubMed]
- Moon H, White AC, Borowsky AD. New insights into the functions of Cox-2 in skin and esophageal malignancies. Exp Mol Med 2020;52:538-47. [Crossref] [PubMed]
- Moons LM, Kuipers EJ, Rygiel AM, et al. COX-2 CA-haplotype is a risk factor for the development of esophageal adenocarcinoma. Am J Gastroenterol 2007;102:2373-9. [Crossref] [PubMed]
- Tuynman JB, Buskens CJ, Kemper K, et al. Neoadjuvant selective COX-2 inhibition down-regulates important oncogenic pathways in patients with esophageal adenocarcinoma. Ann Surg 2005;242:840-9, discussion 849-50. [Crossref] [PubMed]
- Honing J, Pavlov KV, Meijer C, et al. Loss of CD44 and SOX2 expression is correlated with a poor prognosis in esophageal adenocarcinoma patients. Ann Surg Oncol 2014;21:S657-64. [Crossref] [PubMed]
- van Olphen S, Biermann K, Spaander MC, et al. SOX2 as a novel marker to predict neoplastic progression in Barrett's esophagus. Am J Gastroenterol 2015;110:1420-8. [Crossref] [PubMed]
- van Olphen SH, Biermann K, Shapiro J, et al. P53 and SOX2 Protein Expression Predicts Esophageal Adenocarcinoma in Response to Neoadjuvant Chemoradiotherapy. Ann Surg 2017;265:347-55. [Crossref] [PubMed]
- Morita K, Furuse M, Fujimoto K, et al. Claudin multigene family encoding four-transmembrane domain protein components of tight junction strands. Proc Natl Acad Sci U S A 1999;96:511-6. [Crossref] [PubMed]
- Katahira J, Inoue N, Horiguchi Y, et al. Molecular cloning and functional characterization of the receptor for Clostridium perfringens enterotoxin. J Cell Biol 1997;136:1239-47. [Crossref] [PubMed]
- Kokai-Kun JF, McClane BA. Deletion analysis of the Clostridium perfringens enterotoxin. Infect Immun 1997;65:1014-22. [Crossref] [PubMed]
- Kokai-Kun JF, Benton K, Wieckowski EU, et al. Identification of a Clostridium perfringens enterotoxin region required for large complex formation and cytotoxicity by random mutagenesis. Infect Immun 1999;67:5634-41. [Crossref] [PubMed]
- Montgomery E, Mamelak AJ, Gibson M, et al. Overexpression of claudin proteins in esophageal adenocarcinoma and its precursor lesions. Appl Immunohistochem Mol Morphol 2006;14:24-30. [Crossref] [PubMed]
- Li C, Geng H, Ji L, et al. ESM-1: A Novel Tumor Biomaker and its Research Advances. Anticancer Agents Med Chem 2019;19:1687-94. [Crossref] [PubMed]
- Sarrazin S, Adam E, Lyon M, et al. Endocan or endothelial cell specific molecule-1 (ESM-1): a potential novel endothelial cell marker and a new target for cancer therapy. Biochim Biophys Acta 2006;1765:25-37. [PubMed]
- Abid MR, Yi X, Yano K, et al. Vascular endocan is preferentially expressed in tumor endothelium. Microvasc Res 2006;72:136-45. [Crossref] [PubMed]
- Cui Y, Guo W, Li Y, et al. Pan-cancer analysis identifies ESM1 as a novel oncogene for esophageal cancer. Esophagus 2021;18:326-38. [Crossref] [PubMed]
- Mahmood N, Mihalcioiu C, Rabbani SA. Multifaceted Role of the Urokinase-Type Plasminogen Activator (uPA) and Its Receptor (uPAR): Diagnostic, Prognostic, and Therapeutic Applications. Front Oncol 2018;8:24. [Crossref] [PubMed]
- Fang L, Che Y, Zhang C, et al. PLAU directs conversion of fibroblasts to inflammatory cancer-associated fibroblasts, promoting esophageal squamous cell carcinoma progression via uPAR/Akt/NF-κB/IL8 pathway. Cell Death Discov 2021;7:32. [Crossref] [PubMed]
- Babula P, Masarik M, Adam V, et al. Mammalian metallothioneins: properties and functions. Metallomics 2012;4:739-50. [Crossref] [PubMed]
- Si M, Lang J. The roles of metallothioneins in carcinogenesis. J Hematol Oncol 2018;11:107. [Crossref] [PubMed]
- Liu Z, Ye Q, Wu L, et al. Metallothionein 1 family profiling identifies MT1X as a tumor suppressor involved in the progression and metastastatic capacity of hepatocellular carcinoma. Mol Carcinog 2018;57:1435-44. [Crossref] [PubMed]
- Guo Y, Christine KS, Conlon F, et al. Expression analysis of epb41l4a during Xenopus laevis embryogenesis. Dev Genes Evol 2011;221:113-9. [Crossref] [PubMed]
- Ishiguro H, Furukawa Y, Daigo Y, et al. Isolation and characterization of human NBL4, a gene involved in the beta-catenin/tcf signaling pathway. Jpn J Cancer Res 2000;91:597-603. [Crossref] [PubMed]
- Zhang Y, Wang X. Targeting the Wnt/β-catenin signaling pathway in cancer. J Hematol Oncol 2020;13:165. [Crossref] [PubMed]
- Zhang W, Lai R, He X, et al. Clinical prognostic implications of EPB41L4A expression in multiple myeloma. J Cancer 2020;11:619-29. [Crossref] [PubMed]
- Tang YT, Hu T, Arterburn M, et al. PAQR proteins: a novel membrane receptor family defined by an ancient 7-transmembrane pass motif. J Mol Evol 2005;61:372-80. [Crossref] [PubMed]
- Sinreih M, Knific T, Thomas P, et al. Membrane progesterone receptors β and γ have potential as prognostic biomarkers of endometrial cancer. J Steroid Biochem Mol Biol 2018;178:303-11. [Crossref] [PubMed]