Development and validation of a nomogram prediction model for early mortality in patients with primary malignant cardiac tumors
Introduction
Although primary cardiac tumors (PCTs) are mainly benign, primary malignant cardiac tumors (PMCTs) account for 5.1–28.7% of PCTs (1). As PMCTs are rare, with an incidence of 34 to 46.6 cases per 100 million, the core knowledge about these tumors has been mostly based on single center studies, case reports, and small case series. Despite the continuous improvement in different treatment modalities, such as surgery, radiotherapy and chemotherapy, the prognosis of PMCT patients is still poor, with a 1-year survival rate of 46%, which is worse than extracardiac cancer of similar histopathology (2,3). In recent years, clinical researchers have paid more attention to PMCTs, and a predictive model was established to estimate 1- and 3-year survival rate using data from the SEER database (4). However, PMCT patients who died early after diagnosis have received little attention. The identification of PMCT patients with high risk of early mortality is conducive to early implementation of preventive interventions, supportive care, and individualized treatment to improve their life quality and survival rate.
Nomograms provide personalized disease-related risk predictions and estimate a specific endpoint via incorporating several variables (5,6). As graphical calculating devices, they provide better user friendliness, discriminatory degree, and predictive accuracy. Nomogram prediction models are helpful for clinical physicians to manage patients in different survival prediction and risk stratification (7,8), and have been successfully developed to estimate early mortality for many malignant tumors, such as gastric cancer, colorectal cancer, and glioma (9-11). However, to our knowledge, a nomogram model for predicting early mortality in PMCT patients has not been established and is worthy of further investigation.
Based on data from the SEER databases, the risk factors for early mortality for PMCT patients were old age, advanced tumor stages, and no surgical resection and non-chemotherapy. Based on the risk factors, we developed and validated an early mortality prediction nomogram model to assist physicians during the therapeutic decision-making process. We present the following article in accordance with the TRIPOD reporting checklist (available at https://dx.doi.org/10.21037/atm-21-5574).
Methods
Patient selection
We obtained data from the SEER database version Incidence-SEER 18 Regs Custom Data (with additional treatment fields), Nov 2018 Sub (1975–2016 varying). The SEER*Stat, 8.3.8, software program was applied to extract eligible patient information and the selection and exclusion criteria are shown in Figure 1. Finally, a total of 638 patients with a PMCT diagnosis between 1975 to 2016 were included. As public data from the SEER database which did not include human subject use or personal identifying information was used, and ethics committee approval was not required. This study was complied with the Helsinki Declaration (as revised in 2013).
Parameters and definition of early mortality
Patient demographic and clinical characteristics included age at diagnosis, gender, marital status, insurance state, tumor grade, tumor stage, tumor histopathology, surgery, radiotherapy, chemotherapy, and year of diagnosis. Age at diagnosis was classified (cut-off points 44 and 76) and year of diagnosis was categorized (cut-off points 2001 and 2008) via the X-tile 3.6.1 program. Race was classified into three groups: black, white, and others, and marital status was classified into married, single, others, and unknown. Insurance state was divided into insured, uninsured, and unknown. Histopathology was grouped into three subgroups: sarcoma, lymphoma, and others, while tumor stage was classified as local, regional, distant, and unknown. Tumor grade was classified into I level, II level, III level, IV level, and unknown, and cancer-directed surgery, radiotherapy, and chemotherapy were divided into yes and no evidence comprising no treatment and unknown. The variables mentioned above without specific information were listed as “unknown” and were also involved in the construction of final nomogram. In the present study, the outcome of each patient was recorded as alive or death. Based on previous studies, early mortality was defined as overall survival (OS) time ≤3 months after diagnosis (12-14).
Statistical analysis
SPSS version 21.0 (SPSS, Chicago, IL, USA), R software version 3.6.2, and X-tile program (Yale University) were used in the analysis. Categorical variables (age at diagnosis, gender, race, marital status, insurance, histopathology, tumor stage, tumor grade, tumor-directed surgery, chemotherapy, radiotherapy, and year of diagnosis) were compared using the Chi-square test or Fisher’s exact test. Variables (with P<0.05) in univariate analysis were further analyzed using a multivariate forward-conditional stepwise logistic regression model. We used multiple logistic regression analysis to identify the independent prognostic factors, which were further used in the construction of the predictive nomogram model for early mortality, and the performance of the model was evaluated by the calibration curve and ROC curve in the training and validation cohort. The calibration plots were established by bootstrapping with 1,000 re-samples in the training and validation cohorts to evaluate the calibration of the nomogram model, and the relationship between predicted probabilities and observed probabilities of early mortality was described graphically. In terms of the ROC curve, the larger the AUC, which was close to 1.0, the more perfect discrimination ability. All statistical tests were two-sided, and P value <0.05 was considered significant.
Results
Patient characteristics
A total of 448 eligible patients were assigned to the training cohort and 190 to the validation cohort. The baseline characteristics of both are shown in Table 1, which also shows there was no significant difference between the two (P>0.05).
Table 1
Variable | Full cohort (n=638) (%) | Training cohort (n=448) (%) | Validation cohort (n=190) (%) | P value |
---|---|---|---|---|
Total early mortality | 0.171 | |||
Yes | 418 (65.5) | 286 (63.8) | 132 (69.5) | |
No | 220 (34.5) | 162 (36.2) | 58 (30.5) | |
Age at diagnosis | 0.495 | |||
<44 | 216 (33.9) | 158 (35.3) | 58 (30.5) | |
44–76 | 339 (53.1) | 232 (51.8) | 107 (56.3) | |
>76 | 83 (13.0) | 58 (12.9) | 25 (13.2) | |
Gender | 0.632 | |||
Male | 335 (52.5) | 238 (53.1) | 97 (51.1) | |
Female | 303 (47.5) | 210 (46.9) | 93 (48.9) | |
Race | 0.826 | |||
White | 494 (77.4) | 344 (76.8) | 150 (78.9) | |
Black | 70 (11.0) | 51 (11.4) | 19 (10.0) | |
Others | 74 (11.6) | 53 (11.8) | 21 (11.1) | |
Marital status | 0.203 | |||
Married | 337 (52.8) | 231 (51.6) | 106 (55.8) | |
Single | 168 (26.3) | 119 (26.6) | 49 (25.8) | |
Others | 112 (17.6) | 79 (17.6) | 33 (17.3) | |
Unknown | 21 (3.3) | 19 (4.2) | 2 (1.1) | |
Insurance state | 0.686 | |||
Insured | 233 (36.5) | 165 (36.8) | 68 (35.8) | |
Uninsured | 18 (2.8) | 11 (2.5) | 7 (3.7) | |
Unknown | 387 (60.7) | 272 (60.7) | 115 (60.5) | |
Grade | 0.598 | |||
I | 3 (0.5) | 3 (0.7) | 0 (0.0) | |
II | 20 (3.1) | 15 (3.3) | 5 (2.6) | |
III | 79 (12.4) | 54 (12.1) | 25 (13.2) | |
IV | 141 (22.1) | 105 (23.4) | 36 (18.9) | |
Unknown | 395 (61.9) | 271 (60.5) | 124 (65.3) | |
Histopathology | 0.483 | |||
Sarcoma | 393 (61.6) | 272 (60.7) | 121 (63.7) | |
Lymphoma | 144 (22.6) | 100 (22.3) | 44 (23.1) | |
Others | 101 (15.8) | 76 (17.0) | 25 (13.2) | |
Stage | 0.25 | |||
Local | 126 (19.7) | 95 (21.2) | 31 (16.3) | |
Regional | 125 (19.6) | 90 (20.1) | 35 (18.4) | |
Distant | 171 (26.8) | 111 (24.8) | 60 (31.6) | |
Unknown | 216 (33.9) | 152 (33.9) | 64 (33.7) | |
Surgery | 0.82 | |||
Yes | 239 (37.4) | 170 (37.9) | 69 (36.3) | |
No | 250 (39.2) | 172 (38.4) | 78 (41.1) | |
Unknown | 149 (23.4) | 106 (23.7) | 43 (22.6) | |
Radiotherapy | 0.726 | |||
Yes | 68 (10.7) | 49 (10.9) | 19 (10.0) | |
No | 570 (89.3) | 399 (89.1) | 171 (90.0) | |
Chemotherapy | 0.188 | |||
Yes | 317 (49.7) | 215 (48.0) | 102 (53.7) | |
No/unknown | 321 (50.3) | 233 (52.0) | 88 (46.3) | |
Year of diagnosis | 0.319 | |||
1975–2000 | 183 (28.7) | 122 (27.2) | 61 (32.1) | |
2001–2008 | 190 (29.8) | 132 (29.5) | 58 (30.5) | |
2009–2016 | 265 (41.5) | 194 (43.3) | 71 (37.4) |
Risk factors for early mortality in the training cohort
Univariate analysis revealed that age (year, P<0.001), histopathology (P<0.001), tumor stage (P<0.001), surgery (P<0.001), radiotherapy (P=0.006), chemotherapy (P<0.001), and insurance state (P=0.021) were factors related with 3-month mortality for PMCT patients in the training cohort, as shown in Table 2.
Table 2
Variable | All patients (n=448) (%) | Not early mortality (n=286) (%) | Early mortality (n=162) (%) | P value |
---|---|---|---|---|
Age at diagnosis | <0.001 | |||
<44 | 158 (35.3) | 118 (41.3) | 40 (24.7) | |
44–76 | 232 (51.8) | 141 (49.3) | 91 (56.2) | |
>76 | 58 (12.9) | 27 (9.4) | 31 (19.1) | |
Gender | 0.118 | |||
Male | 238 (53.1) | 144 (50.3) | 94 (58.0) | |
Female | 210 (46.9) | 142 (49.7) | 68 (42.0) | |
Race | 0.888 | |||
White | 344 (76.8) | 219 (76.6) | 125 (77.2) | |
Black | 51 (11.4) | 34 (11.9) | 17 (10.5) | |
Others | 53 (11.8) | 33 (11.5) | 20 (12.3) | |
Marital status | 0.516 | |||
Married | 231 (51.6) | 147 (51.4) | 84 (51.8) | |
Single | 119 (26.6) | 81 (28.3) | 38 (23.5) | |
Others | 79 (17.6) | 48 (16.8) | 31 (19.1) | |
Unknown | 19 (4.2) | 10 (3.5) | 9 (5.6) | |
Insurance state | 0.021 | |||
Insured | 165 (36.8) | 118 (41.3) | 47 (29.0) | |
Uninsured | 11 (2.5) | 5 (1.7) | 6 (3.7) | |
Unknown | 272 (60.7) | 163 (57.0) | 109 (67.3) | |
Grade | 0.395 | |||
I | 3 (0.7) | 3 (1.0) | 0 (0.0) | |
II | 15 (3.3) | 9 (3.2) | 6 (3.7) | |
III | 54 (12.1) | 38 (13.3) | 16 (9.9) | |
IV | 105 (23.4) | 71 (24.8) | 34 (21.0) | |
Unknown | 271 (60.5) | 165 (57.7) | 106 (65.4) | |
Histopathology | <0.001 | |||
Sarcoma | 272 (60.7) | 178 (62.2) | 94 (58.0) | |
Lymphoma | 100 (22.3) | 74 (25.9) | 26 (16.1) | |
Others | 76 (17.0) | 34 (11.9) | 42 (25.9) | |
Tumor stage | <0.001 | |||
Local | 95 (21.2) | 74 (25.9) | 21 (13.0) | |
Regional | 90 (20.1) | 60 (21.0) | 30 (18.5) | |
Distant | 111 (24.8) | 55 (19.2) | 56 (34.5) | |
Unknown | 152 (33.9) | 97 (33.9) | 55 (34.0) | |
Surgery | <0.001 | |||
Yes | 170 (37.9) | 130 (45.5) | 40 (24.7) | |
No | 172 (38.4) | 93 (32.5) | 79 (48.8) | |
Unknown | 106 (23.7) | 63 (22.0) | 43 (26.5) | |
Radiotherapy | 0.006 | |||
Yes | 49 (10.9) | 40 (14.0) | 9 (5.6) | |
No | 399 (89.1) | 246 (86.0) | 153 (94.4) | |
Chemotherapy | <0.001 | |||
Yes | 215 (48.0) | 181 (63.3) | 34 (21.0) | |
No | 233 (52.0) | 105 (36.7) | 128 (79.0) | |
Year of diagnosis | 0.053 | |||
1975–2000 | 122 (27.2) | 73 (25.5) | 49 (30.2) | |
2001–2008 | 132 (29.5) | 77 (26.9) | 55 (34.0) | |
2009–2016 | 194 (43.3) | 136 (47.6) | 58 (35.8) |
Based on multivariate logistic analysis, old age (P=0.05), advanced tumor stage (P<0.001), no surgery (P=0.001), and no chemotherapy (P<0.001) were identified as independent risk factors for early mortality in PMCT patients, as shown in Table 3.
Table 3
Variable | HR | 95% CI | P value | |
---|---|---|---|---|
Low | High | |||
Age at diagnosis | 0.05 | |||
<44 | Reference | Reference | ||
44–76 | 1.827 | 1.072 | 3.144 | |
>76 | 2.307 | 1.018 | 5.231 | |
Tumor stage | <0.001 | |||
Local | Reference | Reference | ||
Regional | 1.956 | 0.934 | 4.095 | |
Distant | 7.728 | 3.592 | 16.625 | |
Unknown | 1.976 | 0.949 | 4.113 | |
Surgery | 0.001 | |||
Yes | Reference | Reference | ||
No | 2.696 | 1.515 | 4.797 | |
Unknown | 2.425 | 1.313 | 4.477 | |
Chemotherapy | <0.001 | |||
Yes | Reference | Reference | ||
No | 10.453 | 6.048 | 18.066 |
HR, hazard ratio; CI, confidence interval.
Performance of the nomogram for predicting early mortality
Based on the results of the multivariate logistic regression analysis, the four variables, including age, tumor stage, surgery, and chemotherapy, were selected to construct the predictive nomogram model for early mortality, as shown in Figure 2. By summing up the scores in the top scale assigned to the variable, the total points could be calculated, which could be easily converted to the probability of early mortality. The nomogram scoring system is shown in Table 4 for a more precise calculation.
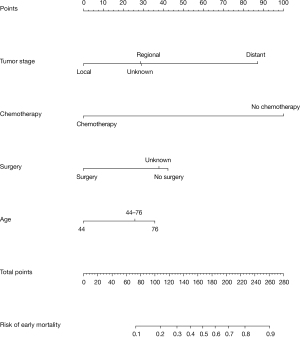
Table 4
Variable | Point |
---|---|
Age at diagnosis | |
<44 | 0 |
44–76 | 26 |
>76 | 36 |
Chemotherapy | |
Yes | 0 |
No | 100 |
Surgery | |
Yes | 0 |
No | 42 |
Unknown | 38 |
Tumor stage | |
Local | 0 |
Regional | 29 |
Distant | 87 |
Unknown | 29 |
In the calibration curve, the mortality estimated by the nomogram was labeled on the x-axis and the actual mortality was labeled on the y-axis. An ideal model indicated as dash lines predicted the same mortality as the outcome of that observed clinically. In the training cohort, the calibration curve showed proper agreement between the predicted and observed probability, with the former close to the 45-degree line (Figure 3A). The AUC of the nomogram model for early mortality prediction was 0.816 in the training cohort, which revealed a satisfactory strength of discrimination (Figure 3B). The calibration curve of the validation cohort revealed that the early mortality predicted by the nomogram was in compliance with actual observation at a high level (Figure 3C), and the AUC of the nomogram model for early mortality prediction was 0.805 in the validation cohort (Figure 3D).
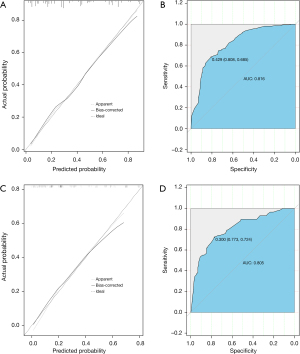
Discussion
PMCTs are extremely rare and fatal malignancies, with 65.5% of patients dying within 3 months of diagnosis in our study. The high percentage of early mortality demonstrates it is crucial to offer attention to patients at high risk of early death. Apart from the aggressive biological behaviour, the lack of a prediction model contributes to the poor survival of PMCT patients. We conducted a retrospective analysis to develop a credible nomogram model to predict early mortality in PMCTs patients using a large population in the SEER database.
As reported in previous studies, old age has been proven to be related with poor survival in patients with breast cancer (15), prostate cancer (16), and osteosarcoma (17). However, the precise cut-off value of age in PMCT remains controversial. Based on the maximum χ2 and minimum P value in the X-tile software (18), the optimal cut-off value for age in PMCTs patients was objectively identified as 44 and 76 in the current study, which is considered to be more credible and accurate. In previous studies on PMCTs patients, age at diagnosis showed a strong prognostic association with OS (4,19). In our study, older age was found to be associated with higher odds of early mortality, with patients over 76-year old having the highest odds of early mortality, followed by the 44–76 age group and 44, which was similar to the optical age cut-off value for OS for PMCT patients (4). Accordingly, patients older than 76 years are considered as a high risk group and should be provided with specific detailed treatment plans and strengthened care.
Advanced tumor stages (regional and distant stage) were also associated with higher odds of early mortality. In previous cancer related study, advanced tumor stages were identified as independent risk factors for early mortality (20) in colorectal cancer (21) and hepatocellular carcinoma (22). Blood vessel tumors constitute most PMCTs, and manifest nonspecific clinical symptoms, such as fever, weakness, and weight (23-25), and many patients are already in an advanced clinical stage at presentation. Hence, a complete resection of the tumor and timely treatment cannot be performed, which contributes to the high odds of early mortality.
Although PMCT patients have a dismal prognosis, our study confirms surgical resection and chemotherapy are associated with a favourable early prognosis. In one study, clinical experience suggested cancer-directed surgical excision was associated with better survival in PMCTs patients (26-28). Surgery intervention is mostly performed in those at an advanced tumor stage, as without it PMCTs can cause hemodynamics disorder, heart failure, hemorrhagic pericardial effusion, and supra-ventricular arrhythmia in a short time. Surgery should be encouraged for eligible patients to decrease the odds of early mortality. Lymphoma is the main pathological type of malignant cardiac tumor and is sensitivity to chemotherapy making this the primary treatment modality (29,30). Sarcoma is also believed to benefit from chemotherapy treatment (4,31,32). In our study, chemotherapy was found to be significantly associated with a decreased incidence of early mortality. Analyze data from China cases showed that the PMCTs accounted 16.03% of the all primary cardiac tumor, and the 1-, 3-, and 5-year survival rate of primary cardiac patient was 83.20%, 78.62% and 66.41% respectively. The prognostic data of Chinese PMCT patients was dismal, and further researches on cardiac tumor were urgently needed in the future (33). According to our research conclusion, it is suggested that active surgical treatment and chemotherapy should be given if the PMCTs patient’s condition permits. For the elderly and patients with advanced tumor stage, early mortality risk should be paid attention to.
The present study has some limitations. Due to the nature of a retrospective study spanning over four decades, there are unavoidable confounding factors despite adjustment. Secondly, data in the SEER database does not include molecular factors, which may influence prognosis and limit our conclusion. The histopathology type of PMCTs has been reclassified many times since the 1970’s, making inference about the early mortality of its sub-types less reliable, so the risk factors for early mortality analysis were not thoroughly performed in each histopathology type.. In addition, we did not analyze cancer specific death separately. Despite these limitations, no other study has provided such a high number of PMCT patients, covering all age range and all kinds of histopathology. The wide range of data, retrieved from SEER, consisting of 18 cancer registries and covering approximately 34.6% of the US population, allows the nomogram to be applied widely. More importantly, we constructed a reliable prognostic nomogram for early mortality in PMCT patients for the first time, the predictive accuracy of which has been validated in several ways. While as an auxiliary screening tool, the nomogram model can be potentially used by physicians, further studies are required validate and refine the nomogram model.
Conclusions
In conclusion, the results of this study showed old age (44–76 and >76 years) and advantaged tumor stage (regional and distant stage) were related with a higher probability of early mortality in PMCT patients, whereas surgery and chemotherapy could reduce this. A nomogram model based on the independent risk factors can be utilized to predict the probability of early mortality in PMCT patients, following which, individual treatment or supportive care can be scheduled in patients with high risk.
Acknowledgments
We sincerely thank Dong Xu for valuable editorial assistance.
Funding: None.
Footnote
Reporting Checklist: The authors have completed the TRIPOD reporting checklist. Available at https://dx.doi.org/10.21037/atm-21-5574
Conflicts of Interest: All authors have completed the ICMJE uniform disclosure form (available at https://dx.doi.org/10.21037/atm-21-5574). The authors have no conflicts of interest to declare.
Ethical Statement: The authors are accountable for all aspects of the work in ensuring that questions related to the accuracy or integrity of any part of the work are appropriately investigated and resolved. The study was conducted in accordance with the Declaration of Helsinki (as revised in 2013). Participant consent was not required as the study involved the use of a previously published de-identified database according to the SEER database.
Open Access Statement: This is an Open Access article distributed in accordance with the Creative Commons Attribution-NonCommercial-NoDerivs 4.0 International License (CC BY-NC-ND 4.0), which permits the non-commercial replication and distribution of the article with the strict proviso that no changes or edits are made and the original work is properly cited (including links to both the formal publication through the relevant DOI and the license). See: https://creativecommons.org/licenses/by-nc-nd/4.0/.
References
- Endo Y, Nakamura Y, Kuroda M, et al. Treatment of malignant primary cardiac lymphoma with tumor resection using minimally invasive cardiac surgery. J Cardiothorac Surg 2018;13:97. [Crossref] [PubMed]
- He S, Chen H, Cao Y, et al. Risk factors for early death in primary malignant cardiac tumors: An analysis of over 40 years and 500 patients. Int J Cardiol 2018;270:287-92. [Crossref] [PubMed]
- Oliveira GH, Al-Kindi SG, Hoimes C, et al. Characteristics and Survival of Malignant Cardiac Tumors: A 40-Year Analysis of >500 Patients. Circulation 2015;132:2395-402. [Crossref] [PubMed]
- Guan T, Li Y, Qiu Z, et al. Nomograms and risk classification systems predicting overall and cancer-specific survival in primary malignant cardiac tumor. J Card Surg 2019;34:1540-9. [Crossref] [PubMed]
- Iasonos A, Schrag D, Raj GV, et al. How to build and interpret a nomogram for cancer prognosis. J Clin Oncol 2008;26:1364-70. [Crossref] [PubMed]
- Zuo Z, Zhang G, Song P, et al. Survival Nomogram for Stage IB Non-Small-Cell Lung Cancer Patients, Based on the SEER Database and an External Validation Cohort. Ann Surg Oncol 2021;28:3941-50. [Crossref] [PubMed]
- Balachandran VP, Gonen M, Smith JJ, et al. Nomograms in oncology: more than meets the eye. Lancet Oncol 2015;16:e173-80. [Crossref] [PubMed]
- Liang W, Zhang L, Jiang G, et al. Development and validation of a nomogram for predicting survival in patients with resected non-small-cell lung cancer. J Clin Oncol 2015;33:861-9. [Crossref] [PubMed]
- Wang X, Mao M, Xu G, et al. The incidence, associated factors, and predictive nomogram for early death in stage IV colorectal cancer. Int J Colorectal Dis 2019;34:1189-201. [Crossref] [PubMed]
- Yang Y, Chen ZJ, Yan S. The incidence, risk factors and predictive nomograms for early death among patients with stage IV gastric cancer: a population-based study. J Gastrointest Oncol 2020;11:964-82. [Crossref] [PubMed]
- Zhou X, Zhang S, Niu X, et al. Risk Factors for Early Mortality Among Patients with Glioma: A Population-Based Study. World Neurosurg 2020;136:e496-503. [Crossref] [PubMed]
- Burroughs AK, Sabin CA, Rolles K, et al. 3-month and 12-month mortality after first liver transplant in adults in Europe: predictive models for outcome. Lancet 2006;367:225-32. [Crossref] [PubMed]
- Zhou X, Niu X, Li J, et al. Risk Factors for Early Mortality in Patients with Primary Central Nervous System Lymphoma: A Large-Cohort Retrospective Study. World Neurosurg 2020;138:e905-12. [Crossref] [PubMed]
- Schechtman KB, Capone RJ, Kleiger RE, et al. Differential risk patterns associated with 3 month as compared with 3 to 12 month mortality and reinfarction after non-Q wave myocardial infarction. The Diltiazem Reinfarction Study Group. J Am Coll Cardiol 1990;15:940-7. [Crossref] [PubMed]
- Pace LE, Keating NL. A systematic assessment of benefits and risks to guide breast cancer screening decisions. JAMA 2014;311:1327-35. [Crossref] [PubMed]
- Drazer MW, Huo D, Eggener SE. National Prostate Cancer Screening Rates After the 2012 US Preventive Services Task Force Recommendation Discouraging Prostate-Specific Antigen-Based Screening. J Clin Oncol 2015;33:2416-23. [Crossref] [PubMed]
- Yang QK, Lai QY, Wang Y, et al. Establishment and validation of prognostic nomograms to predict overall survival and cancer-specific survival for patients with osteosarcoma. Neoplasma 2021;68:434-46. [Crossref] [PubMed]
- Camp RL, Dolled-Filhart M, Rimm DL. X-tile: a new bio-informatics tool for biomarker assessment and outcome-based cut-point optimization. Clin Cancer Res 2004;10:7252-9. [Crossref] [PubMed]
- Xiao M, Lin J, Xiao T, et al. The incidence and survival outcomes of patients with primary cardiac lymphoma: A SEER-based analysis. Hematol Oncol 2020;38:334-43. [Crossref] [PubMed]
- Muller P, Walters S, Coleman MP, et al. Which indicators of early cancer diagnosis from population-based data sources are associated with short-term mortality and survival? Cancer Epidemiol 2018;56:161-70. [Crossref] [PubMed]
- Downing A, Aravani A, Macleod U, et al. Early mortality from colorectal cancer in England: a retrospective observational study of the factors associated with death in the first year after diagnosis. Br J Cancer 2013;108:681-5. [Crossref] [PubMed]
- Chen ZH, Hong YF, Lin J, et al. Validation and ranking of seven staging systems of hepatocellular carcinoma. Oncol Lett 2017;14:705-14. [Crossref] [PubMed]
- Hamidi M, Moody JS, Weigel TL, et al. Primary cardiac sarcoma. Ann Thorac Surg 2010;90:176-81. [Crossref] [PubMed]
- Ramlawi B, Leja MJ, Abu Saleh WK, et al. Surgical Treatment of Primary Cardiac Sarcomas: Review of a Single-Institution Experience. Ann Thorac Surg 2016;101:698-702. [Crossref] [PubMed]
- Shanmugam G. Primary cardiac sarcoma. Eur J Cardiothorac Surg 2006;29:925-32. [Crossref] [PubMed]
- Boyacıoğlu K, Ak A, Dönmez AA, et al. Outcomes After Surgical Resection of Primary Non-Myxoma Cardiac Tumors. Braz J Cardiovasc Surg 2018;33:162-8. [Crossref] [PubMed]
- Elbardissi AW, Dearani JA, Daly RC, et al. Survival after resection of primary cardiac tumors: a 48-year experience. Circulation 2008;118:S7-15. [Crossref] [PubMed]
- Wu Y, Million L, Moding EJ, et al. The impact of postoperative therapy on primary cardiac sarcoma. J Thorac Cardiovasc Surg 2018;156:2194-203. [Crossref] [PubMed]
- Sultan I, Aranda-Michel E, Habertheuer A, et al. Long-Term Outcomes of Primary Cardiac Lymphoma. Circulation 2020;142:2194-5. [Crossref] [PubMed]
- Burazor I, Aviel-Ronen S, Imazio M, et al. Primary malignancies of the heart and pericardium. Clin Cardiol 2014;37:582-8. [Crossref] [PubMed]
- Aboud A, Farha K, Hsieh WC, et al. Prognostic Factors for Long-Term Survival after Surgical Resection of Primary Cardiac Sarcoma. Thorac Cardiovasc Surg 2019;67:665-71. [Crossref] [PubMed]
- Randhawa JS, Budd GT, Randhawa M, et al. Primary Cardiac Sarcoma: 25-Year Cleveland Clinic Experience. Am J Clin Oncol 2016;39:593-9. [Crossref] [PubMed]
- Yin L, He D, Shen H, et al. Surgical treatment of cardiac tumors: a 5-year experience from a single cardiac center. J Thorac Dis 2016;8:911-9. [Crossref] [PubMed]
(English Language Editor: B. Draper)