Autophagy-related long non-coding RNA signature for potential prognostic biomarkers of patients with cervical cancer: a study based on public databases
Introduction
As the most common gynecological cancer, cervical cancer (CC) accounts for 10–15% of cancer-related deaths in women worldwide (1). Research has shown that metastasis and recurrence are the main causes of death from cervical cancer. Comprehensive evidence has demonstrated that the survival rate and cure rate of early-stage CC (I–II) are 80–90% and 60%, respectively. When the cancer develops to the late stage or in the case of relapse, the prognosis is poor (2,3). For patients with advanced stage CC, the 5-year survival rate is not more than 40%. It is urgently important to place greater focus on the pathogenesis of CC to identify biomarkers that have high sensitivity and specificity for prognosis for CC.
Autophagy is an important molecular pathway for cell homeostasis and participates in various pathological and physiological processes (4). With the development of research, autophagy is generally considered to play a dual role in the development of tumors (5). In the early stage, autophagy plays an inhibitory role, while in the late stage, autophagy can provide nutrition for tumor cells via the digestion and decomposition of normal cells and can thus promote the development of tumors (6). A study showed that autophagy is closely related to the occurrence and development of CC (7). Under the influence of autophagy on the formation, growth, and treatment of CC, the specific mechanism of autophagy and related genes in cervical cancer is gradually becoming clear. Long non-coding RNAs (lncRNAs) are noncoding RNAs longer than 200 nucleotides. The expression of lncRNAs is commonly related to the malignant phenotype, metastasis, invasion, and prognosis of CC (8). Although progress has been made on the relationship between lncRNAs and CC (9), the molecular mechanism by which lncRNAs participate in the development of CC has not been fully elucidated. In recent years, studies have shown that lncRNA, through regulating autophagy in tumor occurrence, inhibition, and chemotherapeutic drug sensitivity, has become a heavily researched subject in the cancer field (10). However, the specific mechanism governing this effect has not been fully explained. A previous study provided evidence that functional suppression of lncRNA HOX transcript antisense RNA (HOTAIR) could enhance sensitivity to radiotherapy by reducing autophagy in HeLa cells (11). Another study determined that after overexpression of lncRNA RP11-381N20.2 in SiHa cells, the killing effect of paclitaxel on tumor cells is enhanced because the autophagy induced by paclitaxel is inhibited. The results of these studies suggested that autophagy-related lncRNAs may play significant roles in the prognosis of CC and may be potential targets of CC treatment (12). However, there are few reports describing screening autophagy-related lncRNAs in CC. The existing studies on lncRNA and cervical cancer prognosis model mainly focus on the prognosis, metastasis and recurrence of cervical cancer (13,14), and some studies are devoted to the impact of immune related cells and HPV related lncRNA on the prognosis of cervical cancer (15,16). No prediction model should be used in practice until the prediction accuracy of new patients is formally verified. Our study first proposed and studied the predictive value of autophagy-related lncRNA in the prognosis of cervical cancer for the first time. It not only predicted the high and low risk of cervical cancer, but also hoped to find some valuable predictive and diagnostic indicators. In this study, The Cancer Genome Atlas database (TCGA) was used to screen autophagy-related lncRNAs in CC, we then attempted to establish a risk score model, and perform validation to identify effective and sensitive molecular biomarkers to improve the prognosis of CC. We present the following article in accordance with the TRIPOD reporting checklist (available at https://dx.doi.org/10.21037/atm-21-5156).
Methods
Information extraction from TCGA database
The RNA sequencing data of 309 cervical squamous cell carcinoma and endocervical adenocarcinoma (CESC; 306 CC patients and 3 adjacent normal tissue samples) were downloaded from TCGA (https://cancergenome.nih.gov/) database. These data also included the clinical information of the patients. The patients were primarily diagnosed as CESC, and the lncRNA expression profile was required to be available for analysis. Patients with other malignant tumors or without primary CC were excluded. The study was conducted in accordance with the Declaration of Helsinki (as revised in 2013).
Identification of lncRNA and autophagy gene screening in CC
The data of lncRNA genes were downloaded from TCGA messenger RNA (mRNA) sequence dataset, and the expression levels of autophagy genes in CC were extracted from TCGA database. At the same time, a list of autophagy genes were screened from the Human Autophagy Database (HADb, http://autophagy.lu/clustering/index.html). Blinded to predictor variables and patient outcome of the other 2 databases, 3 certified physicians extracted and analyzed their relevant variables in the standardized language development format. The correlation expression between the lncRNA genes and the autophagy-related genes was calculated by Pearson correlation test. The results showed the co-expression of lncRNA genes and autophagy genes. In addition, if the correlation coefficient |R2| >0.3 and P<0.05 at the same time, the lncRNA was considered an autophagy-related lncRNA.
Potential prognostic signature development
To determine the autophagy-related lncRNAs (prognostic genes) related to the overall survival (OS) of CC, the Kaplan-Meier (K-M) method and univariate Cox regression analysis were performed. If an outcome was missing, the patient data were excluded from the analysis. The expression levels of lncRNAs screened out previously were divided into high and low groups according to the median value. The difference was detected by the K-M method. In addition, univariate Cox regression was also implemented to determine the lncRNA expression related to the survival time and state of participants. Then, autophagy-related lncRNAs with a value of P<0.05 by both the K-M method and univariate Cox analysis were selected for the multivariate stepwise regression Cox analysis to set up the risk score of the participants. A risk score signature was established by the expression and coefficients of prognostic lncRNAs in the multivariate Cox regression model: risk score = βlncRNA1 × ExplncRNA1 + βlncRNA2 × ExplncRNA2 + … + βlncRNAn × ExplncRNAn. The βlncRNA was the regression coefficient derived by the multivariate Cox regression model. The ExplncRNA was the expression level of lncRNAs. Next, the risk score of each participant could be calculated according to the risk model. According to the median value, all patients with CC could be divided into high- and low-risk score groups. Both K-M curves of survival status and time-dependent receiver operating characteristic (survival ROC) curves were used to verify the prognostic ability of this risk score model. To maximize the generalizability and power of the results, all eligible data in these databases were extracted and used.
Nomogram construction
Based on the OS information and clinical data of the patients with CC, univariate and multivariate Cox regression analyses were performed to investigate the relationship between survival status, clinical characteristics, and risk score. Finally, a nomogram was established to predict the progression of CC (1, 3, and 5 years). The prediction value of the nomogram was verified by calibration curves.
Gene set enrichment analysis
To investigate the gene expression status, gene set enrichment analysis (GSEA) was implemented, and gene data were downloaded from the Kyoto Encyclopedia of Genes and Genomes (KEGG). This method was employed to judge whether gene sets showed significant differences in different biological aspects. Then, we examined whether the differentially expressed genes (DEGs) of the 2 groups were enriched during tumor pathogenesis and autophagy.
Statistical analysis
The data were expressed as the mean ± SD, and data analysis was processed by R language (R version 3.6.3; https://www.R-project.org/), SPSS 17.0 (IBM Corp., Armonk, NY, USA), and GraphPad Prism 8 (GraphPad Software Inc., La Jolla, CA, USA). The co-expression network of autophagy genes and lncRNAs was structured by Cytoscape software (version 3.7.2; the Cytoscape Consortium, San Diego, CA, USA). All graphs were drawn by R software, and P<0.05 was considered significant.
Results
Basic information of the CC patients
In total, 307 CC samples were extracted from TCGA database, all which had information on survival time and status. The 307 CC patients were female. The average age of the patients was 48.27±13.79 years, and among them, 71 (23.1%) were dead, and 236 (76.9%) were still alive. The average survival time was 1,026.33±1,142.21 days.
Construction of a prognosis model for CC with autophagy-related lncRNAs
In total, 14,142 lncRNAs were extracted from TCGA database. By calculating whether lncRNAs were co-expressed with autophagy genes, which were downloaded from the HADb, 1,245 autophagy-related lncRNAs were extracted. Then, after screening by the K-M method and univariate Cox regression analysis (|R2| >0.3 and P≤0.05), 25 autophagy-related lncRNAs were related to prognosis and could meet the needs of both of the above data analyses (Table 1). Cox regression analysis showed that 10 autophagy-related lncRNAs (AC012306.2, AL109976.1, ATP2A1-AS1, ILF3-DT, Z83851.2, STARD7-AS1, AC099343.2, AC008771.1, DBH-AS1, and AC097468.3) were independent prognostic indicators for CC patients. A net of lncRNAs with co-expressed autophagy genes for the prognosis of CC was created with Cytoscape (Figure 1A). A 10-lncRNA prognostic signature was constructed by weighting β values: Risk score = ExpAC012306.2 × (0.767) + ExpAL109976.1 × (−1.208) + ExpATP2A1-AS1 × (−0.076) + ExpILF3-DT × (−0.094) + ExpZ83851.2 × (−0.275) + ExpSTARD7-AS1 × (−0.396) + ExpAC099343.2 × (0.502) + ExpAC008771.1 × (−0.121) + ExpDBH-AS1 × (−0.581) + ExpAC097468.3 × (−0.302). As shown in the model, the coefficients of AL109976.1, ATP2A1-AS1, ILF3-DT, Z83851.2, STARD7-AS1, AC008771.1, DBH-AS1, and AC097468.3 were negative, suggesting that they were protective factors for the survival of CC patients, while the coefficients of AC012306.2 and AC099343.2 were positive, indicating that both lncRNAs may be risk factors. This information is presented in the Sankey diagram (Figure 1B). The survival curves of these 10 prognostic autophagy-related lncRNAs for CC in TCGA database were established by the K-M method (Figure 2).
Table 1
LncRNA | K-M | β | SE | HR | 95% CI of HR | P |
---|---|---|---|---|---|---|
AC005332.4 | 0.001 | −0.571 | 0.167 | 0.565 | 0.407–0.784 | 0.001 |
AC012306.2 | 0.011 | 0.348 | 0.121 | 1.416 | 1.117–1.794 | 0.004 |
CNNM3-DT | 0.006 | −0.613 | 0.234 | 0.542 | 0.343–0.856 | 0.009 |
AL109976.1 | 0.011 | −0.854 | 0.322 | 0.426 | 0.226–0.801 | 0.008 |
ATP2A1-AS1 | 0.009 | −0.141 | 0.057 | 0.868 | 0.776–0.971 | 0.013 |
ARRDC1-AS1 | 0.004 | −0.173 | 0.061 | 0.841 | 0.746–0.949 | 0.005 |
PCED1B-AS1 | 0.005 | −0.260 | 0.091 | 0.771 | 0.644–0.922 | 0.004 |
AC026979.2 | 0.014 | −0.320 | 0.114 | 0.726 | 0.580–0.908 | 0.005 |
MAPKAPK5-AS1 | 0.003 | −0.134 | 0.049 | 0.875 | 0.795–0.962 | 0.006 |
AC090948.3 | 0.015 | −0.832 | 0.277 | 0.435 | 0.253–0.748 | 0.003 |
ILF3-DT | 0.001 | −0.087 | 0.027 | 0.917 | 0.869–0.968 | 0.002 |
AP005482.3 | 0.000 | −0.466 | 0.151 | 0.628 | 0.467–0.845 | 0.002 |
Z83851.2 | 0.011 | −0.416 | 0.148 | 0.660 | 0.493–0.882 | 0.005 |
STARD7-AS1 | 0.008 | −0.617 | 0.247 | 0.539 | 0.333–0.875 | 0.012 |
AC139530.1 | 0.000 | −0.365 | 0.140 | 0.694 | 0.528–0.913 | 0.009 |
AL031673.1 | 0.001 | −0.315 | 0.129 | 0.729 | 0.566–0.940 | 0.015 |
AL109811.3 | 0.013 | −0.229 | 0.089 | 0.796 | 0.668–0.947 | 0.010 |
AL021707.6 | 0.000 | −0.143 | 0.047 | 0.867 | 0.791–0.950 | 0.002 |
AC108134.4 | 0.008 | −0.864 | 0.330 | 0.421 | 0.220–0.805 | 0.009 |
AC099343.2 | 0.014 | −0.760 | 0.290 | 0.468 | 0.265–0.825 | 0.009 |
AC008771.1 | 0.001 | −0.133 | 0.041 | 0.876 | 0.809–0.948 | 0.001 |
DBH-AS1 | 0.009 | −0.466 | 0.169 | 0.627 | 0.450–0.874 | 0.006 |
AC097468.3 | 0.000 | −0.325 | 0.120 | 0.723 | 0.572–0.913 | 0.007 |
LIPE-AS1 | 0.001 | −0.622 | 0.234 | 0.537 | 0.339–0.850 | 0.008 |
AC079414.3 | 0.006 | -0.478 | 0.155 | 0.620 | 0.458–0.840 | 0.002 |
Both the K-M value <0.05 and P<0.05 was statically significant.
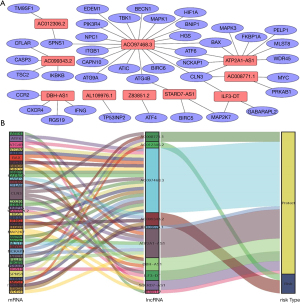
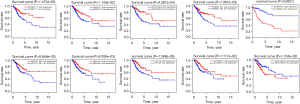
Impact of the autophagy-related lncRNA signature on the prognosis of CC
By using a risk score method, CC patients were divided into low- and high-risk groups by median risk score to establish an autophagy-related lncRNA signature (Figure 3A). The survival status indicated that the survival time became shorter, and the number of deceased patients gradually increased from a low-risk score to a high-risk score (Figure 3B). Through heatmaps, it was determined that the expression of lncRNAs, which should be protective factors, was lower in the high-risk group than in the low-risk group, and the expression of lncRNAs, which should be risk factors, was higher in the high-risk group than in the low-risk group (Figure 3C). The risk score could also significantly predict the OS of CC. The OS status in the low-risk group was considerably longer than that in the high-risk group (P<0.01, Figure 4A). In addition, survival-ROC analysis revealed that the 10-lncRNA signature could predict the survival rate of CC, and the area under the survival-ROC curve (AUC) was 0.810 (Figure 4B). Both univariate and multivariate Cox regression analyses were used to analyze the relationship between the 10-lncRNA signature established in this study and the clinical characteristics of CC patients. UniCox regression analysis indicated a significant prognostic impact of the risk score on CC [hazard ratio (HR) =1.665; 95% confidence interval (CI): 1.331–2.082; P<0.001; Figure 4C]. In addition, multivariate Cox regression analysis was performed. It was confirmed that this risk score signature was an effective prognostic predictor of CC. Compared with other factors, such as age, grade, and tumor (T) and node (N) stages, the HR of 1.738 (95% CI: 1.359–2.223; P<0.001; Figure 4D) indicated that the risk score could significantly predict the survival of CC patients (Tables 2,3). Unfortunately, the cases in some groups of metastasis (M) stage were not enough to be analyzed.
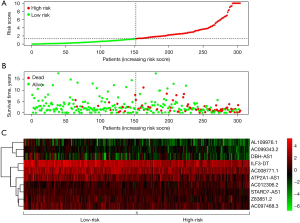
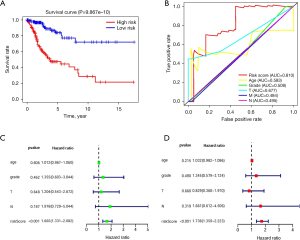
Table 2
Variable | β | SE | Z | HR | 95% CI of HR | P |
---|---|---|---|---|---|---|
Age | 0.012 | 0.023 | 0.516 | 1.012 | 0.967–1.060 | 0.606 |
Grade | 0.205 | 0.374 | 0.549 | 1.228 | 0.590–2.553 | 0.583 |
AJCC T | 0.449 | 0.350 | 1.283 | 1.567 | 0.789–3.111 | 0.200 |
AJCC N | 0.651 | 0.493 | 1.320 | 1.918 | 0.729–5.044 | 0.187 |
Risk score | 0.510 | 0.114 | 4.467 | 1.665 | 1.331–2.082 | 0.000 |
P<0.05 was statically significant. CC, cervical cancer.
Table 3
Variable | β | SE | z | HR | 95% CI of HR | P |
---|---|---|---|---|---|---|
Age | 0.021 | 0.026 | 0.802 | 1.021 | 0.970–1.075 | 0.423 |
Grade | 0.230 | 0.414 | 0.557 | 1.259 | 0.560–2.833 | 0.578 |
AJCC T | 0.131 | 0.359 | 0.364 | 1.140 | 0.564–2.301 | 0.716 |
AJCC N | 0.517 | 0.516 | 1.003 | 1.678 | 0.610–4.613 | 0.316 |
Risk score | 0.582 | 0.135 | 4.305 | 1.789 | 1.373–2.332 | 0.000 |
P<0.05 was statically significant. CC, cervical cancer.
Nomogram construction
To build and validate a more accurate prediction model, a nomogram was constructed using the clinical features, including age, clinical stage, TNM staging, and risk score. The prognosis of CC patients for 1, 3, and 5 years was predicted (Figure 5A). The results showed that the risk score plays a major role in the prediction model and the prognosis of CC, and the concordance index (C-index) predicted by the nomogram was 0.909±0.942. In addition, a calibration curve was plotted to evaluate the consistency between the actual survival and the predicted survival (P<0.001), and the high consistency suggested that the model could have a better prediction for the survival of CC patients (Figure 5B).
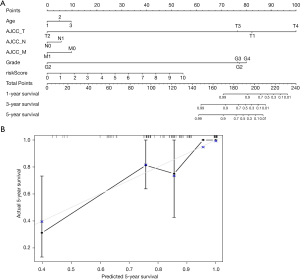
GSEA
Functional annotation associated with autophagy-related lncRNAs was performed through GSEA. There were 9 gene sets between the 2 biological groups mainly enriched in autophagy-related pathways at a nominal P value <0.05% (Table 4), and several pathways were well conformed in tumor performance and autophagy, including adherens junction, focal adhesion, extracellular matrix (ECM) receptor interaction, DNA replication, oxidative phosphorylation, and glutathione metabolism signaling pathways (Figure 6). In addition, several gene sets were also established involving special diseases closely related to autophagy, such as Alzheimer’s disease, Parkinson’s disease, and Huntington’s disease. In summary, the defined lncRNAs contribute to tumor pathogenesis and autophagy signature pathways. Moreover, the lncRNAs may supply important biomarkers and targets for the early diagnosis and treatment of CC through further research of the genes and pathways.
Table 4
Name | Size | ES | NES | NOM, P value | FDR, q value | FWER, P value | Rank at max | Leading edge |
---|---|---|---|---|---|---|---|---|
KEGG-Adherens-Junction | 73 | 0.580383 | 2.000085 | 0 | 0.009945 | 0.02 | 8,177 | Tags =53%, list =15%, signal =63% |
KEGG-Focal-Adhesion | 199 | 0.579221 | 2.053815 | 0 | 0.004661 | 0.007 | 5,975 | Tags =44%, list =11%, signal =49% |
KEGG-ECM-Receptor-Interaction | 84 | 0.680222 | 2.084129 | 0 | 0.004629 | 0.004 | 8,976 | Tags =58%, list =16%, signal =70% |
KEGG-DNA-Replication | 36 | −0.75591 | −1.94416 | 0.001901 | 0.014582 | 0.037 | 7,890 | Tags =67%, list =14%, signal =78% |
KEGG-Glutathione-Metabolism | 49 | −0.61798 | −1.90916 | 0.005814 | 0.017095 | 0.058 | 10,702 | Tags =55%, list =19%, signal =68% |
KEGG-Oxidative-Phosphorylation | 131 | −0.7549 | −2.22452 | 0 | 0.001667 | 0.001 | 4,901 | Tags =54%, list =9%, signal =59% |
KEGG-Alzheimer’s-Disease | 165 | −0.58917 | −2.12353 | 0.001961 | 0.001289 | 0.003 | 5,427 | Tags =42%, list =10%, signal =46% |
KEGG-Huntington’s-Disease | 180 | −0.62113 | −2.16203 | 0.001887 | 0.001962 | 0.002 | 5,510 | Tags =45%, list =10%, signal =50% |
KEGG-Parkinson’s-Disease | 127 | −0.71309 | −2.13856 | 0.001988 | 0.001718 | 0.003 | 4,901 | Tags =50%, list =9%, signal =55% |
P<0.05 was statically significant. KEGG, Kyoto Encyclopedia of Genes and Genomes; lncRNAs, long noncoding RNAs; ES, enrichment score; FDR, false discovery rate; FWER, familywise-error rate; NES, normalized enrichment score; NOM P value, nominal P value.
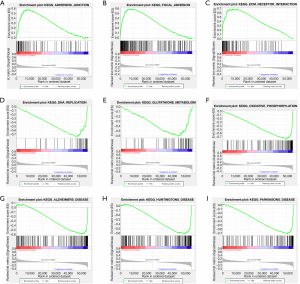
Discussion
Among women worldwide, and especially in developing countries, CC is still the major cause of cancer-related mortality. The prognosis and the 5-year OS rate of CC worsen from the early- to advanced-stage. Recurrence and distant metastasis are still dominant reasons for death from CC. Postoperative adjuvant chemoradiotherapy may be important in reducing metastasis and prolonging OS in advanced CC. Hence, valuable prognostic markers predicting the survival of CC patients may be helpful for the development of individualized treatment.
The role of lncRNAs in CC development has been well investigated, and many studies have confirmed that lncRNAs have important impacts on the pathogenesis of CC. compared with normal tissues, the expression of some lncRNAs in cervical cancer tissues increased or decreased, manifested as oncogenes or tumor suppressor genes. For example, HOTAIR, MALAT1, ANRIL and H19 play the role of oncogenes (17), while MEG3, GAS5 and LET play the role of tumor suppressor genes (18). Some studies have found that HOTAIR starts Wnt/β-Catenin signaling pathway in HeLa cells, through methylation of TET1 promoter and down-regulation of its expression, so as to inhibit the negative regulators of this signaling pathway PCDH10, SOX17, AJAP1 and MAGI2 to achieve oncogene action (19). HOTAIR up-regulates the expression of VEGF and MMP-9, which are important factors to promote the metastasis and invasion of cervical cancer cells, and up-regulates the expression of β-catenin, vimentin, snail and twist. It makes HOTAIR possible to be a marker and therapeutic target for prognosis of cervical cancer. In recent years. Many studies have found that lncRNA polymorphism is related to the susceptibility to cervical cancer, such as HOTAIR, THRIL, GAS5 and so on. Some studies indicated HOTAIR SNPs (rs920778, rs1899663 and Rs4759314) was associated with cervical cancer. It was found that the risk of cervical cancer increased to 2.88 and 1.34 in patients with rs920778 TT and CT genotypes, respectively (20). Some others studies found through case-control study that carriers of A allele and AA + AG genotype in linc00673 rs11655237 had a significantly higher risk of cervical cancer (21). It was also found that the T allele of rs3787016 located in the intron region of POLR2E is a cervical cancer susceptibility gene. Compared with CC and TC genotypes, TT genotype carriers have a higher risk of cervical cancer (22). These studies show that lncRNA polymorphism is closely related to the risk of cervical cancer in women. However, due to the different sample size and population genetic background, some research results are different, which needs to be further confirmed by multi-center large sample population research.
However, autophagy in CC has not been fully elucidated to date, with its role currently remaining controversial. Recent studies have confirmed that lncRNAs can regulate the performance of autophagy, which indicates a new way for advanced research on autophagy-related diseases, especially tumors. Deng et al. indicated that the high expression of loc146880 can upregulate the autophagy factor lc3b, thereby promoting the migration, invasion, and epithelial-mesenchymal transition (EMT) of lung cancer (23). Yuan et al. illustrated that downregulated miR-216 can lead to autophagy in hepatocellular carcinoma cells, and the enhancement of autophagy has been demonstrated to be an important reason for drug resistance (24). Wang et al. supported that lncRNA CTA can inhibit autophagy by competitively binding mir-210, thereby significantly enhancing the role of doxorubicin (DOX), and that it can also be reactivated by DOX. Therefore, lncRNA CTA is not only an essential regulator of DOX but also enhances the sensitivity of osteosarcoma to DOX by downregulating autophagy (25). In summary, lncRNAs can regulate autophagy and affect the development of tumors. They can activate or enhance autophagy directly or through binding of microRNA (miRNA). Additionally, by inhibiting autophagy, lncRNAs can enhance the sensitivity of tumors to chemotherapy drugs. Therefore, using lncRNAs to regulate autophagy to provide important targets for the treatment and prognosis prediction of tumors may have important clinical value. Although there are a number of reports on the establishment of gene signatures in CC, including lncRNA-related signatures in CC (13,16), effective molecular biomarkers, especially autophagy-related biomarkers, are still needed to predict the progression and prognosis of CC. Therefore, it is necessary to establish an autophagy-related lncRNA signature for the prognosis of CC.
In this study, data from TCGA were screened to establish the prognostic signature of autophagy-related lncRNAs for CC. First, target lncRNAs were distinguished from the lncRNA–autophagy co-expression network. The obtained lncRNAs can be considered molecular biomarkers of CC related to autophagy, which is of strong significance for regulating autophagy and improving CC prognosis. Furthermore, 10 autophagy-associated lncRNA signatures were identified, which could divide CC patients into high- and low-risk groups according to the median risk score. Our study confirmed that the OS was shorter in the high-risk group than in the low-risk group. Additionally, the 10-lncRNA signature could significantly predict the 5-year survival rate of CC (AUC =0.810). Through univariate and multivariate Cox regression analyses, it was shown that the risk score (P<0.001) is an independent prognostic indicator that is significantly related to OS. In addition, a nomogram was also drawn to build a prediction model of CC, which indicated that the risk score is the most important factor in the prognosis of CC, which is consistent with the previous findings in this study. The calibration curve of the nomogram verified the predicted survival of the model, which is in keeping with the actual survival. Thus, this finding notably reflects that the predictive model followed by the risk score model can properly predict the prognosis of CC.
Our model relies on demographic data and autophagy-related lncRNA to predict the prognosis of cervical cancer from TGCA database. We found that a high-risk score showed shorter survival time and the OS status compared with other factors, such as age, grade, and T and N stages. Moreover, the expression of lncRNAs was higher in the high-risk group. This indicated that lncRNAs were also a risk factor and the 10 autophagy-related lncRNA signatures established in this study can effectively predict the prognosis of CC. Although these indicators have previously been associated with the progression of CC, our work integrated them into a single risk model. In addition, by adding variables of age, grade, and T and N stages, we showed that the performance of the model did not improve. Although these other variables are obviously important for diagnosis and treatment of CC, it is unknown whether they are related to the lack of some grading indicators in the sample. It is hoped that the sample data can be added in the later stage to further confirm.
To the best of our knowledge, these prognostic lncRNAs have rarely been reported, except lncRNA DBH-AS1. A series of rescue experiments supported the role of DBH-AS1 in diffuse large B cell lymphoma. Tumor progression is regulated by the RNA-binding protein BUD13 and fibronectin 1. It was concluded that DBH-AS1 may be the target of lymphoma treatment (26). Another study showed that knocking out the DBH-AS1 gene can inhibit the proliferation, migration, and invasion of osteosarcoma cells by inhibiting activation of the PI3K/Akt pathway (27). Other studies have shown that DBH-AS1 is a carcinogenic gene of hepatocellular carcinoma (28,29). A GSEA analysis was conducted to forecast the potential roles of these lncRNAs in CC. These lncRNAs may play their roles in several KEGG pathways associated with autophagy and tumor formation and development. The role of autophagy in cellular processes, such as cell homeostasis, epithelial cell junctions, focal adhesion, and ECM receptor interaction, has been increasingly recognized. E-cadherin, which represents adherens junctions, is associated with the invasion and metastasis of CC (30). Other studies have also indicated that ECM-integrin receptors can predict metastatic propensities in CC, and the expression of biomarkers on tumor cells was studied to select more suitable patients for radiotherapy (31). These genes are also significantly enriched in DNA replication, oxidative phosphorylation, and the glutathione metabolism signaling pathway. Studies have found that autophagy captures damaged DNA and organelles with implications for cancer. Autophagy has been demonstrated to be regulated by oxidative phosphorylation and glutathione metabolism (31,32), and the latter is related to the development of CC. Thus, these genes may be new targets for the study of CC metastasis and treatment. In addition, as a new drug screening method, network pharmacology has been widely used in tumor therapy in recent years, including targeted therapy of cervical cancer. In the next study, we can focus on autophagy-related lncRNA related drug screening and use network pharmacology to study its feasibility in the treatment of cervical cancer.
Clearly, there were several limitations to this study. There were sufficiently few cases of metastasis that some grades only had 1 or 2 cases. Thus, the metastasis status of CC could not be used to predict survival. In addition, TCGA data are based on RNA sequencing technology. Whether more methods are needed for validation remains to be discussed. Finally, the mechanisms of autophagy-related lncRNAs in CC warrant further study. More detailed experiments should be implemented to explore the exact mechanisms in the near future.
Conclusions
In this study, a 10 autophagy-related lncRNA signature was found, which was demonstrated to be an important prognostic method of CC. These lncRNAs have a strong relationship with autophagy and tumorigenesis, participate in some important signaling pathways, and interact with CC. These autophagy-related lncRNA signatures may contribute to the prognosis and pathogenesis of CC and might provide new targets for the prevention and treatment of CC.
Acknowledgments
Funding: This work was supported by the National Natural Science Foundation of China (81702579 to HW).
Footnote
Reporting Checklist: The authors have completed the TRIPOD reporting checklist. Available at https://dx.doi.org/10.21037/atm-21-5156
Conflicts of Interest: All authors have completed the ICMJE uniform disclosure form (available at https://dx.doi.org/10.21037/atm-21-5156). HW reported that this work was supported by the National Natural Science Foundation of China (81702579 to HW). The other authors have no conflicts of interest to declare.
Ethical Statement: The authors are accountable for all aspects of the work in ensuring that questions related to the accuracy or integrity of any part of the work are appropriately investigated and resolved. The study was conducted in accordance with the Declaration of Helsinki (as revised in 2013).
Open Access Statement: This is an Open Access article distributed in accordance with the Creative Commons Attribution-NonCommercial-NoDerivs 4.0 International License (CC BY-NC-ND 4.0), which permits the non-commercial replication and distribution of the article with the strict proviso that no changes or edits are made and the original work is properly cited (including links to both the formal publication through the relevant DOI and the license). See: https://creativecommons.org/licenses/by-nc-nd/4.0/.
References
- Ojesina AI, Lichtenstein L, Freeman SS, et al. Landscape of genomic alterations in cervical carcinomas. Nature 2014;506:371-5. [Crossref] [PubMed]
- Siegel RL, Miller KD, Jemal A. Cancer statistics, 2018. CA Cancer J Clin 2018;68:7-30. [Crossref] [PubMed]
- Zhou H, Li Q, Xu C, et al. Prognosis of stage III cervical cancer: a two-way outcome study. Transl Cancer Res 2020;9:2565-75. [Crossref]
- Ohsumi Y. Historical landmarks of autophagy research. Cell Res 2014;24:9-23. [Crossref] [PubMed]
- Orfanelli T, Jeong JM, Doulaveris G, et al. Involvement of autophagy in cervical, endometrial and ovarian cancer. Int J Cancer 2014;135:519-28. [Crossref] [PubMed]
- Choi KS. Autophagy and cancer. Exp Mol Med 2012;44:109-20. [Crossref] [PubMed]
- Xu L, Liu JH, Zhang J, et al. Blockade of autophagy aggravates endoplasmic reticulum stress and improves Paclitaxel cytotoxicity in human cervical cancer cells. Cancer Res Treat 2015;47:313-21. [Crossref] [PubMed]
- Du Z, Fei T, Verhaak RG, et al. Integrative genomic analyses reveal clinically relevant long noncoding RNAs in human cancer. Nat Struct Mol Biol 2013;20:908-13. [Crossref] [PubMed]
- Yang W, Hong L, Xu X, et al. LncRNA GAS5 suppresses the tumorigenesis of cervical cancer by downregulating miR-196a and miR-205. Tumour Biol 2017;39:1010428317711315 [Crossref] [PubMed]
- Shan D, Shang Y, Hu T. Long noncoding RNA BLACAT1 promotes cell proliferation and invasion in human cervical cancer. Oncol Lett 2018;15:3490-5. [Crossref] [PubMed]
- Guo X, Xiao H, Guo S, et al. Long noncoding RNA HOTAIR knockdown inhibits autophagy and epithelial-mesenchymal transition through the Wnt signaling pathway in radioresistant human cervical cancer HeLa cells. J Cell Physiol 2019;234:3478-89. [Crossref] [PubMed]
- Zou SH, Du X, Lin H, et al. Paclitaxel inhibits the progression of cervical cancer by inhibiting autophagy via lncRNARP11-381N20.2. Eur Rev Med Pharmacol Sci 2018;22:3010-7. [PubMed]
- Shen L, Yu H, Liu M, et al. A ten-long non-coding RNA signature for predicting prognosis of patients with cervical cancer. Onco Targets Ther 2018;11:6317-26. [Crossref] [PubMed]
- Wang J, Zhang C. Identification and validation of potential mRNA- microRNA- long-noncoding RNA (mRNA-miRNA-lncRNA) prognostic signature for cervical cancer. Bioengineered 2021;12:898-913. [Crossref] [PubMed]
- Ye J, Chen X, Lu W. Identification and Experimental Validation of Immune-Associate lncRNAs for Predicting Prognosis in Cervical Cancer. Onco Targets Ther 2021;14:4721-34. [Crossref] [PubMed]
- Cheng Y, Yang S, Shen Y, et al. The Role of High-Risk Human Papillomavirus-Related Long Non-Coding RNAs in the Prognosis of Cervical Squamous Cell Carcinoma. DNA Cell Biol 2020;39:645-53. [Crossref] [PubMed]
- Wang N, Hou MS, Zhan Y, et al. MALAT1 promotes cisplatin resistance in cervical cancer by activating the PI3K/AKT pathway. Eur Rev Med Pharmacol Sci 2018;22:7653-9. [PubMed]
- Zhang J, Yao T, Lin Z, et al. Aberrant Methylation of MEG3 Functions as a Potential Plasma-Based Biomarker for Cervical Cancer. Sci Rep 2017;7:6271. [Crossref] [PubMed]
- Salmerón-Bárcenas EG, Illades-Aguiar B, Del Moral-Hernández O, et al. HOTAIR Knockdown Decreased the Activity Wnt/β-Catenin Signaling Pathway and Increased the mRNA Levels of Its Negative Regulators in Hela Cells. Cell Physiol Biochem 2019;53:948-60. [Crossref] [PubMed]
- Guo L, Lu X, Zheng L, et al. Association of Long Non-Coding RNA HOTAIR Polymorphisms with Cervical Cancer Risk in a Chinese Population. PLoS One 2016;11:e0160039 [Crossref] [PubMed]
- Wang Y, Luo T. LINC00673 rs11655237 Polymorphism Is Associated With Increased Risk of Cervical Cancer in a Chinese Population. Cancer Control 2018;25:1073274818803942 [Crossref] [PubMed]
- Chen B, Jiao Y, Yaolong F, et al. The POLR2E rs3787016 polymorphism is strongly associated with the risk of female breast and cervical cancer. Pathol Res Pract 2019;215:1061-5. [Crossref] [PubMed]
- Deng X, Feng N, Zheng M, et al. PM2.5 exposure-induced autophagy is mediated by lncRNA loc146880 which also promotes the migration and invasion of lung cancer cells. Biochim Biophys Acta Gen Subj 2017;1861:112-25. [Crossref] [PubMed]
- Yuan P, Cao W, Zang Q, et al. The HIF-2α-MALAT1-miR-216b axis regulates multi-drug resistance of hepatocellular carcinoma cells via modulating autophagy. Biochem Biophys Res Commun 2016;478:1067-73. [Crossref] [PubMed]
- Wang Z, Liu Z, Wu S. Long non-coding RNA CTA sensitizes osteosarcoma cells to doxorubicin through inhibition of autophagy. Oncotarget 2017;8:31465-77. [Crossref] [PubMed]
- Song Y, Gao F, Peng Y, et al. Long non-coding RNA DBH-AS1 promotes cancer progression in diffuse large B-cell lymphoma by targeting FN1 via RNA-binding protein BUD13. Cell Biol Int 2020;44:1331-40. [Crossref] [PubMed]
- Liu ZB, Wang JA, Lv RQ. Downregulation of long non-coding RNA DBH-AS1 inhibits osteosarcoma progression by PI3K-AKT signaling pathways and indicates good prognosis. Eur Rev Med Pharmacol Sci 2019;23:1418-27. [PubMed]
- Bao J, Chen X, Hou Y, et al. LncRNA DBH-AS1 facilitates the tumorigenesis of hepatocellular carcinoma by targeting miR-138 via FAK/Src/ERK pathway. Biomed Pharmacother 2018;107:824-33. [Crossref] [PubMed]
- Huang JL, Ren TY, Cao SW, et al. HBx-related long non-coding RNA DBH-AS1 promotes cell proliferation and survival by activating MAPK signaling in hepatocellular carcinoma. Oncotarget 2015;6:33791-804. [Crossref] [PubMed]
- Cunniffe C, Ryan F, Lambkin H, et al. Expression of tight and adherens junction proteins in cervical neoplasia. Br J Biomed Sci 2012;69:147-53. [Crossref] [PubMed]
- Wu K, Yi Y, Liu F, et al. Identification of key pathways and genes in the progression of cervical cancer using bioinformatics analysis. Oncol Lett 2018;16:1003-9. [Crossref] [PubMed]
- Kageyama S, Ii H, Taniguchi K, et al. Mechanisms of Tumor Growth Inhibition by Depletion of γ-Glutamylcyclotransferase (GGCT): A Novel Molecular Target for Anticancer Therapy. Int J Mol Sci 2018;19:2054. [Crossref] [PubMed]
(English Language Editor: J. Jones)