Identification of biomarkers and ceRNA network in glioblastoma through bioinformatic analysis and evaluation of potential prognostic values
Introduction
Glioblastoma (GBM) remains one of the most malignant, primary brain tumors in adults (1). In the United States, the incidence rate of GBM is 5–8 per 100,000 people, accounting for 54% of all glioma patients (2). According to the World Health Organization (WHO) classification of central nervous system tumors, GBM is classified into the highest grade of IV. At present, the standard treatment for GBM is surgical resection, postoperative radiotherapy, and chemotherapy with temozolomide. However, the efficacy of these methods is limited in patients with GBM. The median survival of GBM patients after standard treatment is only 15 months (3). Improving the treatment of GBM patients, extending survival periods, and enhancing quality of life are vital issues for neurosurgeons today. GBM is the most common primary brain tumor in adults (4). Considering the high degree of malignancy and incidence rate of GBM, the development of more effective therapeutic strategies is urgently needed.
lncRNA is a class of non-coding RNAs, sequences of which have no ability to encode proteins. However, emerging evidence indicate that lncRNAs are involved in diverse biological processes and exert important functions. They can be transcribed into antisense RNA to interfere coding genes or can act as a competitive endogenous RNA by binding microRNAs (miRNAs). Competing endogenous RNA (ceRNA) hypothesis that lncRNA serving as miRNA sponges to regulate the level of genes which harbor the common miRNA binding sites has been widely demonstrated in cancer (5,6). Substantial studies revealed that lncRNA can regulate tumorigenesis and pathogenesis of glioma (7). In recent years, tumor molecular biology has made remarkable progress. An increasing number of genes and molecular pathways have been found to be related to GBM and each discovery has the potential of becoming a therapeutic target (8). Datasets of genes and molecular pathways are abundantly available and can be processed quickly and accurately through bioinformatic analyses. These analyses are now widely applied in oncology research and specifically in glioma research (9), from the initial differential gene analysis to the complex ceRNA network analysis (10). Bioinformatics can also be used to predict prognostic factors and potential molecular mechanisms of glioma (11,12).
In this study, we performed bioinformatic analyses on datasets downloaded from the National Center for Biotechnology Information (NCBI) Gene Expression Omnibus (GEO) and the Chinese Glioma Genome Atlas (CGGA). A miRNA regulation network was constructed using miRWalk2.0 (http://zmf.umm.uni-heidelberg.de/apps/zmf/mirwalk2/). A ceRNA network of lncRNA-miRNA target genes was constructed by integrating the relationships between long non-coding RNAs (lncRNA) and miRNAs, miRNAs and target genes, and the co-expression between differential messenger RNAs (mRNA) and differential lncRNAs (positive co-expression relationship). The results of our study provide potential prognostic biomarkers and therapeutic targets for GBM. The flow chart of this study protocol is shown in Figure 1. Finally, we identified a novel lncRNA IL10RB-AS1, which is up-regulated in GBM and associated with poor prognosis of patients. The potential ceRNA networks of IL10RB-AS1 in GBM were also identified. Our findings provide a potential target for developing therapeutic strategies. We present the following article in accordance with the REMARK reporting checklist (available at https://dx.doi.org/10.21037/atm-21-4925).
Methods
Patients and samples
Patient samples were collected at the Changzheng Hospital, Naval Medical University, from February 2019 to June 2020. Fourteen glioblastoma tissues (Grade IV) and six non-tumor brain tissues obtained from patients underwent cranial injury internal decompression as for negative control were collected and enrolled. The tissues were collected by surgery and stored at −80 °C. All patients were officially informed and signed consent forms. The diagnoses of the above samples were provided by two individual pathologists under the guidance of the WHO glioma classification criteria. The study was conducted in accordance with the Declaration of Helsinki (as revised in 2013). This study has been approved by the ethics committee of the Naval Medical University (No. 112019053).
Microarray data
Expression profiles were downloaded from the NCBI GEO database (https://www.ncbi.nlm.nih.gov/geo/). Nine GBM samples and three normal samples were extracted from accession GSE104267. The mRNA data were generated using the GPL22448 Phalanx Human lncRNA OneArray v1_mRNA platform (https://www.ncbi.nlm.nih.gov/geo/query/acc.cgi?acc=GPL22448), while the lncRNA data were generated using the GPL22449 Phalanx Human lncRNA OneArray v1_lncRNA platform (https://www.ncbi.nlm.nih.gov/geo/query/acc.cgi?acc=GPL22449).
Data preprocessing and Differential expressed genes (DEGs) screening of mRNA and lncRNA
Raw data were downloaded from the GEO database, and R statistical software (version 3.5.3, https://www.r-project.org/) and Bioconductor analysis tools (http://www.bioconductor.org/) were utilized to analyze the raw data. The “affy” (Gautier et al., 2004) (version 1.56.0, http://bioconductor.org/packages/release/bioc/html/oligo.html) package in R was utilized to preprocess the raw data and normalize via background adjustment, quantile normalization, and finally, summarization and log base 2 scale. The platform annotation file was used to perform one-to-one matching of probe numbers and mRNA/lncRNA gene symbols. Probes that were not matched to a gene symbol were removed. For multiple probes mapped to the same gene, we took the mean value of the probes as the final expression value of the mRNA/lncRNA. The classical Bayesian method provided by the R software package “limma” (version 3.10.3, http://www.bioconductor.org/packages/2.9/bioc/html/limma.html) was used to screen DEGs between tumor and normal tissues (13). MRNAs/lncRNAs with a P value <0.05 and |logFC|>2 were considered to be differentially expressed.
Functional and pathway enrichment analysis
The clusterProfiler (version 3.2.11, http://www.bioconductor.org/packages/release/bioc/html/clusterprofiler.html) in R was used to perform a Kyoto Encyclopedia of Genes and Genomes (KEGG) pathway enrichment analysis and biological process (BP) enrichment analysis of gene ontology (GO) (13,14). These analyses were carried out separately in the upregulated and downregulated groups. Results satisfying the condition of count ≥2 with a P value <0.05 were regarded as a significant enrichment results.
Co-expression analysis and prediction of lncRNA function
The Pearson correlation coefficient (r) of each lncRNA and mRNA were calculated, and the correlation tests were performed. Differential lncRNA and mRNA relationship pairs were screened; r>0.85 and the adjusted P<0.05 following correction of the false discovery rate (FDR) was regarded as differential. The function of each lncRNA was analyzed by the relationship pairs in the regulatory lncRNA-mRNA network. The clusterProfiler package in R was used to analyze the pathways and BPs in which the target genes of these lncRNAs were involved. Pathways and BPs with the number of parameter enrichment genes count ≥2 and P<0.05 were regarded as significant enrichment results.
Prediction of miRNA target regulation
The DEGs of Homo sapiens were inputted into the target gene analysis module of miRWalk 2.0 (http://zmf.umm.uni-heidelberg.de/apps/zmf/mirwalk2/miRretsys-self.html) (15). Additional databases used for analysis included MicroT v4.0(http://snf-515788.vm.okeanos.grnet.gr/index.php?r=microtv4/index), miRanda(http://www.microrna.org/microrna/home.do), miRDB(http://www.mirdb.org/miRDB/policy.html), miRMap(http://miRNAMap.mbc.nctu.edu.tw/), PITA(http://genie.weizmann.ac.il/pubs/mir07/mir07_data.html/), RNA22(http://cbcsrv.watson.ibm.com/rna22.html/), and Targetscan (http://www.targetscan.org/). The regulation list was acquired by running pairs through each database; regulation pairs appearing in more than six databases were used to construct the miRNA regulation network.
Construction of the ceRNA network
We used the DIANA-LncBase v.2 database (http://carolina.imis.athena-innovation.gr/diana_tools/web/index.php?r=lncbasev2%2Findex) to predict lncRNAs related to the regulatory network (16), with miRNA as the background of the lncRNA-miRNA relationship, and to screen differential lncRNA-miRNA regulatory relationships. The ceRNA lncRNA-miRNA target gene network was constructed by integrating the relationships between lncRNAs and miRNAs, miRNAs and target genes, and the co-expression between differential mRNAs and lncRNAs (positive co-expression relationship).
Survival analysis
We analyzed the prognosis-related clinical information of GBM in TCGA, including overall survival (OS) and survival status. According to the expression of lncRNA, samples were divided into two groups: high expression and low expression, and a log-rank statistical test was carried out (P<0.05 was set as the statistical significance threshold).
Also, Kaplan-Meier (K-M) survival curves were constructed according to the K-M single-factor analysis. Additional clinical TCGA-GBM information relevant to prognosis was integrated with lncRNA expression, including gender, age, and treatment type. Samples with missing clinical information were excluded (Table 1). Cox univariate and multivariate survival analyses of possible prognostic factors were performed using SPSS version 19.0 software (SPSS Inc., Chicago, IL, USA) and are shown in Table 1.
Table 1
Sample information | Number |
---|---|
Clinical factors | |
Gender | |
Female | 50 |
Male | 100 |
Age | |
≤45 | 21 |
>45 | 129 |
Treatment (pharmaceutical therapy or radiation therapy) | |
Yes | 130 |
No | 20 |
RNA expression | |
IL10RB-AS1 | |
High | 61 |
Low | 89 |
qRT-PCR
Total RNA was extracted from 100 µg plaques using Trizol reagent (QIAGEN, Germany) and was reverse-transcribed into complementary DNA (cDNA) with a miScript Reverse Transcription Kit (QIAGEN). Real-time quantitative reverse transcription polymerase chain reaction (qRT-PCR) was performed with an miScript SYBR Green PCR kit (QIAGEN). Gene expression was measured with qRT-PCR on an ABI 7300 Real-Time PCR System (Applied Biosystems, USA) at 95 °C for 10 min, followed by 40 cycles of 95 °C for 15 s, 60 °C for 15 s, and 75 °C for 20 s. Gene expression was normalized to the reference gene, Glyceraldehyde-3-Phosphate Dehydrogenase (GADPH). The relative quantification of gene expression was determined using the 2−∆∆Ct method (17). The primer sequences are listed in Table 2.
Table 2
Primer | Sequence (5'-3') |
---|---|
Human-GAPDH-Forward | GGAAGCTTGTCATCAATGGAAATC |
Human-GAPDH-Reverse | TGATGACCCTTTTGGCTCCC |
Human-IL10RB-AS1-Forward | ATGCCCTACAACACCAACCC |
Human-IL10RB-AS1-Reverse | ACTTCCCTCTGCTTGACTTTACG |
Human-DPYD-Forward | AGTTTCTCCATAGTGGTGCTTCC |
Human-DPYD-Reverse | CTCTGTCCATCCCAGTCTTGTAG |
Human-GBP1-Forward | TGAGAACACTAATGGGCGACT |
Human-GBP1-Reverse | CACACACCACATCCAGATTCCTT |
Statistical analysis
Statistical analyses were performed using R software (https://mirrors.tuna.tsinghua.edu.cn/CRAN/) and GraphPad Prism version 7.0 (GraphPad Software, Inc., San Diego, California, USA). Kaplan-Meier survival analysis was conducted together with the log-rank test to compare the prognostic risk of GBM patients with different expression levels. Student’s t-test was used to compare two different groups. Cox hazard regression model was used for univariate and multivariate survival analyses, and factors with P<0.05 in univariate analysis were included in the multivariate analysis. Data were presented as means ± standard deviation (SD) and P<0.05 was considered as statistically significant.
Results
Differential expression analysis
According to the screening criteria, 253 DEGs were obtained, including 152 upregulated and 101 downregulated genes. Moreover, 54 differentially-expressed lncRNAs were also obtained, including 10 upregulated and 44 downregulated genes (Figure 2A,2B).
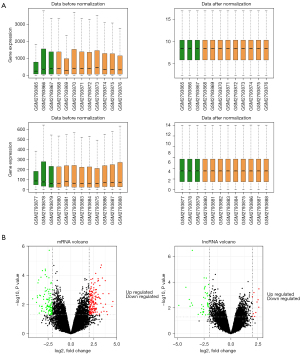
Functional enrichment analysis of DEGs
The DEGs were divided into two groups, which were significantly upregulated and downregulated, based on the log-fold change (logFC) values. According to the BP and KEGG enrichment annotation analyses, 280 BP and 16 KEGG pathways were significantly enriched in the upregulated group, while 242 BP and 12 KEGG pathways were markedly enriched in the downregulated group. In the downregulated group, the most enriched terms were GO:0048665, neuron fate specification (BP, P value 6.49E-12), and hsa:04080, neuroactive ligand-receptor interaction (KEGG, P value 0.000913857). In the upregulated group, the most significant terms were GO:0002446, neutrophil mediated immunity (BP, P value 4.99E-07), and hsa:04657, interleukin 17 (IL-17) signaling pathway (KEGG, P value 0.000240137) (Figure 3A,3B).
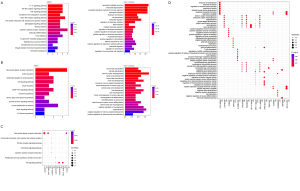
lncRNA-mRNA co-expression and functional prediction analysis of lncRNA
A total of 237 co-expressed differential lncRNA-mRNA pairs (all positive correlations) were obtained, including 49 lncRNAs and 104 mRNAs. Based on the co-expression of lncRNA and mRNA, the functions of differential lncRNAs were predicted, and 11 related pathways were obtained. The top five pathways with significant P values of each lncRNA were selected for demonstration (Figure 3C). LncRNA GO-BP enrichment was also demonstrated (Figure 3D).
Construction of the ceRNA network
A total of 251 miRNAs, 12 target genes, and 302 miRNA target regulatory pairs were identified in the miRNA-target network. Fourteen lncRNAs and 720 miRNA-lncRNA pairs were identified by analysis, while 228 miRNAs, 12 mRNAs, and 826 ceRNA regulatory pairs were obtained by integrating the miRNA network with the gene network. The ceRNA network was constructed via Cytoscape (18) (version 3.2.0, http://www.cytoscape.org/) (Figure 4A). Glioma-related miRNAs were identified by searching the miRWalk database. Six of these miRNAs also existed in the ceRNA network. According to these six miRNAs, the ceRNA subnetwork, including 21 nodes and 29 regulatory pairs, was constructed (Figure 4B).
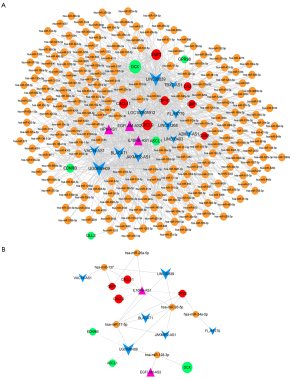
Survival analysis
Based on the clinical data of GBM in TCGA, a K-M single-factor analysis of lncRNAs in the ceRNA subnetwork was conducted. One survival-related differentially-expressed lncRNA, IL10RB-AS1, was identified through the log-rank statistical test, and a K-M survival curve was constructed (Figure 5A). Two clinical prognostic factors, age and treatment, were identified through Cox univariate survival analyses (Table 3). Cox multivariate survival analyses of IL10RB-AS1 expression, age, and treatment was also performed (Table 4, Figure 5B).
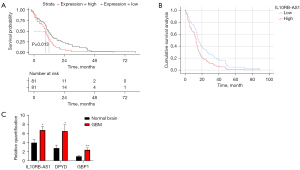
Table 3
Variables | β | Standard error (SE) | Wald χ2 | P | Hazard ratio (HR) (95% CI) |
---|---|---|---|---|---|
IL10RB-AS1 (high vs. low) | 0.52 | 0.187 | 7.758 | 0.005 | 1.683 (1.167–2.426) |
Age (≤45 vs. >45) | 0.939 | 0.308 | 9.257 | 0.002 | 2.556 (1.397–4.680) |
Treatment (yes vs. no) | 0.703 | 0.259 | 7.337 | 0.007 | 2.019 (1.214–3.357) |
Gender (female vs. male) | – | – | – | 0.313 | – |
Table 4
Variables | β | SE | Wald χ2 | P | HR (95% CI) |
---|---|---|---|---|---|
IL10RB-AS1 (high vs. low) | 0.478 | 0.189 | 6.382 | 0.012 | 1.613 (1.113–2.339) |
Age (≤45 vs. >45) | 0.778 | 0.312 | 6.209 | 0.013 | 2.177 (1.181–4.015) |
Treatment (yes vs. no) | 0.618 | 0.263 | 5.511 | 0.019 | 1.854 (1.107–3.106) |
Expression of IL10RB-AS1, DPYD, and GBP1 between GBM and normal brain tissues
IL10RB-AS1, Dihydropyrimidine Dehydrogenase (DPYD), and Guanylate Binding Protein 1 (GBP1) were markedly more upregulated in the GBM tissues than in the normal brain tissues, as determined by qRT-PCR (Figure 5C).
Discussion
In this study, we identified 253 DEGs and 54 differentially-expressed lncRNAs from GBM samples, compared with normal tissue samples. Based on BP and KEGG enrichment annotation analyses, 280 BP and 16 KEGG pathways were significantly enriched in the upregulated group, while 242 BP and 12 KEGG pathways were significantly enriched in the downregulated group. Eleven lncRNA-related pathways were obtained via functional prediction analysis of lncRNA. The ceRNA network, and small chemical molecule-target network were constructed via Cytoscape. Based on the clinical data of GBM in TCGA, a K-M single-factor analysis of lncRNAs in the ceRNA network was performed.
One survival-related differentially-expressed lncRNA, IL10RB-AS1, was identified via K-M single-factor analysis. According to our screening of DEGs, IL10RB-AS1, a lncRNA located at 21q22.11, was significantly upregulated in GBM compared with normal brain tissue. Currently, there is no available research on IL10RB-AS1. IL10RB-AS1, also known as IL10RB Divergent Transcript (IL10RB-DT), is a divergent transcript of IL10RB, which is a subunit of the IL-10 receptor and has already been identified to be a prognostic factor with a strong predictive value in GBM (19). It is possible that IL10RB-AS1 may retain the GBM-associated characteristic of IL10RB. According to the K-M survival curve of IL10RB-AS1, the OS of patients with GBM was significantly reduced in the IL10RB-AS1 high-expression group compared to the IL10RB-AS1 low-expression group. Additionally, IL10RB-AS1 was identified via Cox multivariate survival analysis to be a prognostic factor independent of clinical prognostic factors. In summary, IL10RB-AS1 was revealed as a new prognostic factor of GBM.
According to the KEGG analysis, four pathways, including viral protein interaction with cytokines and cytokine receptors, Toll-like receptor signaling pathways, chemokine signaling pathways, and cytokine-cytokine receptor interaction were identified to be related to IL10RB-AS1. Toll-like receptor 4 (TLR4) has already been identified to be related to the prognosis of GBM (20). The C-X-C Motif Chemokine Ligand 12 (CXCL12)/C-X-C Motif Chemokine Receptor 4 (CXCR4) system, a chemokine signaling pathway, has been found to contribute to the proliferation and invasion of glioma-initiating cells (21). Thus, IL10RB-AS1 might influence the prognosis of GBM via Toll-like receptor or chemokine signaling pathways.
According to the GO-BP analysis, IL10RB-AS1 may be involved in six BPs, including T cell chemotaxis, regulation of cyclic adenosine monophosphate (cAMP)-mediated signaling, positive regulation of the release of sequestered calcium ions into cytosol, T cell migration, second-messenger-mediated signaling, and divalent inorganic cation homeostasis. CAMP-mediated signaling has been identified and can be regulated to suppress GBM cell migration and invasion by Mps One Binder Kinase Activator-Like 2 (MOB2) (22). IL10RB-AS1 might promote GBM cell migration and invasion through reverse regulation cAMP-mediated signaling.
A total of two ceRNA systems related to IL10RB-AS1, including the IL10RB-AS1/hsa-miR-26a-5p/GBP1 system and the IL10RB-AS1/hsa-miR-93-5p/DPYD system, were identified in the ceRNA subnetwork. GBP1 has previously been confirmed to be a prognostic factor and promote tumor growth in GBM (23). Thus, IL10RB-AS1 might promote tumor growth by competitively binding hsa-miR-26a-5p with GBP1 in GBM.
The expression levels of IL10RB-AS1, DPYD, and GBP1 were shown to be higher in GBM tissue than that in normal brain tissue in our qRT-PCR validation analysis (Figure 5).
To summarize, our research demonstrated that IL10RB-AS1 is a new prognostic factor of GBM. We also predicted several signaling pathways and ceRNA systems related to IL10RB-AS1 that might be regulated by IL10RB-AS1 to influence the prognosis of GBM.
Conclusions
Through a combination of bioinformatic analyses, we identified one survival-related differentially-expressed lncRNA, IL10RB-AS1, and several related signaling pathways and ceRNA systems with potential prognostic value in GBM. This insight has the potential to improve the treatment of GBM by providing more targets for therapy.
Acknowledgments
Funding: This study was supported by National Natural Science Foundation of China (Grant No.81902538) and the Wuxi Taihu Talent Program (BJ2020072).
Footnote
Reporting Checklist: The authors have completed the REMARK reporting checklist. Available at https://dx.doi.org/10.21037/atm-21-4925
Data Sharing Statement: Available at https://dx.doi.org/10.21037/atm-21-4925
Conflicts of Interest: All authors have completed the ICMJE uniform disclosure form (available at https://dx.doi.org/10.21037/atm-21-4925). The authors have no conflicts of interest to declare.
Ethical Statement: The authors are accountable for all aspects of the work in ensuring that questions related to the accuracy or integrity of any part of the work are appropriately investigated and resolved. The study was conducted in accordance with the Declaration of Helsinki (as revised in 2013). This study has been approved by the ethics committee of the Naval Medical University (No. 112019053). Informed consent to participate in this study was obtained from the research subjects prior to study commencement.
Open Access Statement: This is an Open Access article distributed in accordance with the Creative Commons Attribution-NonCommercial-NoDerivs 4.0 International License (CC BY-NC-ND 4.0), which permits the non-commercial replication and distribution of the article with the strict proviso that no changes or edits are made and the original work is properly cited (including links to both the formal publication through the relevant DOI and the license). See: https://creativecommons.org/licenses/by-nc-nd/4.0/.
References
- Moretti E, Favero G, Rodella LF, et al. Melatonin's Antineoplastic Potential Against Glioblastoma. Cells 2020;9:599. [Crossref] [PubMed]
- Ostrom QT, Gittleman H, Liao P, et al. CBTRUS Statistical Report: Primary brain and other central nervous system tumors diagnosed in the United States in 2010-2014. Neuro Oncol 2017;19:v1-v88. [Crossref] [PubMed]
- Alphandéry E. Nano-Therapies for Glioblastoma Treatment. Cancers (Basel) 2020;12:242. [Crossref] [PubMed]
- Dobes M, Khurana VG, Shadbolt B, et al. Increasing incidence of glioblastoma multiforme and meningioma, and decreasing incidence of Schwannoma (2000-2008): Findings of a multicenter Australian study. Surg Neurol Int 2011;2:176. [Crossref] [PubMed]
- Abdollahzadeh R, Daraei A, Mansoori Y, et al. Competing endogenous RNA (ceRNA) cross talk and language in ceRNA regulatory networks: A new look at hallmarks of breast cancer. J Cell Physiol. 2019;234:10080-10100. [Crossref] [PubMed]
- Wang L, Cho KB, Li Y, et al. Long Noncoding RNA (lncRNA)-Mediated Competing Endogenous RNA Networks Provide Novel Potential Biomarkers and Therapeutic Targets for Colorectal Cancer. Int J Mol Sci. 2019;20:5758. [Crossref] [PubMed]
- Cen L, Liu R, Liu W, et al. Competing Endogenous RNA Networks in Glioma. Front Genet. 2021;12:675498 [Crossref] [PubMed]
- Le Rhun E, Preusser M, Roth P, et al. Molecular targeted therapy of glioblastoma. Cancer Treat Rev 2019;80:101896 [Crossref] [PubMed]
- Tao Z, Shi A, Li R, et al. Microarray bioinformatics in cancer- a review. J BUON 2017;22:838-43. [PubMed]
- Wang H, Zhang H, Zeng J, et al. ceRNA network analysis reveals prognostic markers for glioblastoma. Oncol Lett 2019;17:5545-57. [Crossref] [PubMed]
- Kiran M, Chatrath A, Tang X, et al. A Prognostic Signature for Lower Grade Gliomas Based on Expression of Long Non-Coding RNAs. Mol Neurobiol 2019;56:4786-98. [Crossref] [PubMed]
- Long S, Li G. Comprehensive analysis of a long non-coding RNA-mediated competitive endogenous RNA network in glioblastoma multiforme. Exp Ther Med 2019;18:1081-90. [Crossref] [PubMed]
- Kanehisa M, Goto S. KEGG: kyoto encyclopedia of genes and genomes. Nucleic Acids Res 2000;28:27-30. [Crossref] [PubMed]
- Yu G, Wang LG, Han Y, et al. clusterProfiler: an R package for comparing biological themes among gene clusters. OMICS 2012;16:284-7. [Crossref] [PubMed]
- Dweep H, Gretz N. miRWalk2.0: a comprehensive atlas of microRNA-target interactions. Nat Methods 2015;12:697. [Crossref] [PubMed]
- Paraskevopoulou MD, Vlachos IS, Karagkouni D, et al. DIANA-LncBase v2: indexing microRNA targets on non-coding transcripts. Nucleic Acids Res 2016;44:D231-8. [Crossref] [PubMed]
- Lovett JK, Gallagher PJ, Hands LJ, et al. Histological correlates of carotid plaque surface morphology on lumen contrast imaging. Circulation 2004;110:2190-7. [Crossref] [PubMed]
- Shannon P, Markiel A, Ozier O, et al. Cytoscape: a software environment for integrated models of biomolecular interaction networks. Genome Res 2003;13:2498-504. [Crossref] [PubMed]
- Cai J, Zhang W, Yang P, et al. Identification of a 6-cytokine prognostic signature in patients with primary glioblastoma harboring M2 microglia/macrophage phenotype relevance. PLoS One 2015;10:e0126022 [Crossref] [PubMed]
- Alvarado AG, Thiagarajan PS, Mulkearns-Hubert EE, et al. Glioblastoma Cancer Stem Cells Evade Innate Immune Suppression of Self-Renewal through Reduced TLR4 Expression. Cell Stem Cell 2017;20:450-461.e4. [Crossref] [PubMed]
- Yi L, Zhou X, Li T, et al. Not ch1 signaling pathway promotes invasion, self-renewal and growth of glioma initiating cells via modulating chemokine system CXCL12/CXCR4. J Exp Clin Cancer Res 2019;38:339. [Crossref] [PubMed]
- Jiang K, Yao G, Hu L, et al. MOB2 suppresses GBM cell migration and invasion via regulation of FAK/Akt and cAMP/PKA signaling. Cell Death Dis 2020;11:230. [Crossref] [PubMed]
- Ji X, Zhu H, Dai X, et al. Overexpression of GBP1 predicts poor prognosis and promotes tumor growth in human glioblastoma multiforme. Cancer Biomark 2019;25:275-90. [Crossref] [PubMed]
(English Language Editor: A. Kassem)