Key immune-related gene ITGB2 as a prognostic signature for acute myeloid leukemia
Introduction
Acute myeloid leukemia (AML) is a hematological malignancy characterized by clonal expansion, differentiation arrest, and evasion of apoptosis, leading to inhibition of normal hematopoiesis. Since leukemia stem cells display diversity in expression, immunophenotype, and differentiation, it is considered a heterogeneous hematological malignancy (1). In 2020, there were an estimated 19,940 new diagnoses and 11,180 deaths from AML in the United States (2). The primary therapeutic protocol for AML is chemotherapy. However, many elderly AML patients are unable to tolerate intensive chemotherapeutic treatment (3). Further, despite advances in treatment approaches, relapse remains a substantial challenge for AML patients (4). Effective and personalized therapy regimens for AML patients are urgently needed.
In the 1970s, conventional chemotherapeutic regimens for AML patients consisted of cytarabine and daunorubicin. Subsequently, homoharringtonine (HHT) was combined with cytarabine and anthracycline to treat AML patients (5,6). Currently, there are no therapeutic regimens for AML patients that prevent relapse and achieve sustained remission. Many cancers, including AML, typically arise from genetic aberrations and immune system deficiency, and strategies to regulate immune function have been introduced in the treatment of cancer. Hematopoietic stem cell transplantation (HSCT) has shown that donor T cells and natural killer (NK) cells can inhibit and eliminate leukemia cells (7), providing a theoretical foundation for cellular immunotherapy in AML. Several studies have found that the tumor microenvironment (TME) of AML plays a key role in disease progression and therapeutic resistance (8,9). However, the TME of AML remains insufficiently understood.
In the present study, we aimed to explore the key irgs involved in AML. Our study involved a discovery phase and 2 validation phases. In the discovery phase, bioinformatics screening was used to identify irgs that had a significant association with AML prognosis. In the prognostics validation phase, the relationship between the screened genes and AML prognosis was evaluated in a cohort from the Cancer Genome Atlas (TCGA) program. In the immunity validation phase, the integrin lymphocyte function-associated antigen 1 (CD11A/CD18; ITGAL/ITGB2) was selected for further bioinformatics analysis to assess associations between ITGB2 and expression levels, immune cell infiltrates, and prognostic values for AML. Finally, we confirmed the protein expression levels of ITGB2 via bone marrow immunohistochemistry. Compared to the Wang et al. study (34148032), we further explored the mRNA expression level of ITGB2 via immunohistochemistry, further drug sensitivity analysis was performed based on the expression of ITGB2.
We present the following article in accordance with the REMARK reporting checklist (available at https://dx.doi.org/10.21037/atm-21-3641).
Methods
Raw data
Transcriptome RNA-Seq data for 151 AML samples were downloaded from TCGA database (https://portal.gdc.cancer.gov/).
Analysis of immune, stromal, and estimate scores
The ESTIMATE algorithm in R version 4.0.3 (https://www.r-project.org/) was used to estimate the ratio of immune-stromal component in the TME, including immune, stromal, and estimate scores.
Survival analysis and Cox risk regression
The survminer R package was used to perform survival analysis. Univariate Cox regression analysis and Kaplan-Meier were used to assess the prognostic role of differential expression genes (DEGs) in AML patients. Kaplan-Meier was used to estimate a survival curve, with log-rank P<0.05 considered significant. Cox regression analysis combined with stepwise regression was performed to establish a Cox risk model.
Analysis of DEGs in high- and low-score groups via immune and stromal scores
The 151 AML samples were divided into high- and low-risk score groups based on median immune and stromal scores. DEGs in the high- and low-score groups were analyzed using the R LIMMA package. A fold change >1 and false discovery rate (FDR) <0.05 were considered significant.
Enrichment function analysis
The biological functions and pathways of the DEGs were investigated using the R packages clusterProfiler, enrichplot, and ggplot2. A P value and Q-value of <0.05 were considered significant.
Heat map construction
A heat map of DEGs was generated using the pheatmap R package.
Constructing the protein-protein interaction (PPI) network
We used STRING datasets to generate a PPI network, which was reconstructed via Cytoscape (Version 3.8.1) with a confidence interval of more than 0.95.
Tumor-infiltrating immune cell (TIC) profile
The TIC abundance profile for each AML sample was analyzed using CIBERSORT analysis, with the 134 AML samples with P<0.05 chosen for further analysis.
Analysis of ITGB2 expression levels in normal and AML bone marrow via immunohistochemistry
We collected bone marrow samples from 3 normal and 3 newly diagnosed AML patients in our department to conduct immunohistochemistry.
Analysis of drug sensitivity
Drug sensitivity analysis was performed via the R pRRophetic package.
Ethical statement
The study was conducted in accordance with the Declaration of Helsinki (as revised in 2013).
Statistical analysis
Difference expressed genes analysis is carried out by limma package and with a log2 fold change |logFC| >1 and false discovery rate (FDR) <0.05 were deemed as significant. The Kaplan-Meier method was used to perform the survival curves. The cluterProfiler packages and enrichplot packages were used for enrichment functions analysis. The R package CIBERSORT was used to estimate the tumor infiltrating immune cells. P value <0.05 was seem as the significant difference.
Results
The association between immune scores, stromal scores, and AML patients’ prognosis
The immune scores, stromal scores, and clinical features of the TCGA cohort of AML patients were extracted. The results of immune and stromal scores indicated that higher estimates (P=0.066) and immune scores (P=0.087) led to poor prognosis in AML patients, while stromal scores did not appear to have an influence on prognosis (Figure 1A-1C).
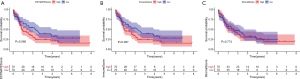
Screening DEGs associated with immune scores and stromal scores in AML
Based on immune and stromal scores, we divided the TCGA cohort of AML patients into either a high- or low-score group. We then analyzed the differential gene expression of the 2 groups by generating a heat map of DEGs (Figure 2A,2B). The results revealed that there were 222 downregulated genes and 655 upregulated genes based on immune scores (high vs. low), and 567 upregulated genes and 218 downregulated genes based on high and low stromal scores (Figure 3A,3B). Genes that were significantly associated with immune and stromal cells were screened via Venn analysis, with the results showing that there were 502 upregulated and 122 downregulated genes in the intersection (Figure 3A,3B). The intersectional genes were further analyzed to determine their potential biological function and prognostics value in AML patients.
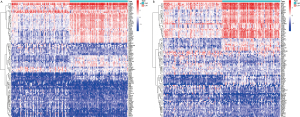
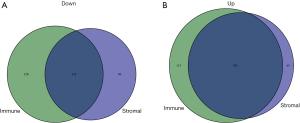
Enrichment functions of DEGs in the intersection
Gene Ontology (GO) and Kyoto Encyclopedia of Genes and Genomes (KEGG) pathway analysis were used to investigate the biological functions of DEGs. The results of enrichment function analysis revealed that the top 10 biological processes (BP) included neutrophil activation, neutrophil activation involved in immune response, neutrophil degranulation, neutrophil-mediated immunity, positive regulation of cytokine production, cytokine secretion, regulation of cytokine secretion, regulation of tumor necrosis factor (TNF) superfamily cytokine production, TNF superfamily cytokine production, and TNF regulation of tumor necrosis factor. The top 10 cellular components (CC) included secretory granule lumen, specific granule membrane, tertiary granule lumen, tertiary granule membrane, collagen-containing extracellular matrix, specific granule, external side of plasma membrane, secretory granule membrane, cytoplasmic vesicle lumen, and tertiary granule. The molecular functions (MF) included lipopeptide binding, carbohydrate binding, immunoglobulin G (IgG) binding, cytokine receptor activity, proteoglycan binding, cytokine binding, immunoglobulin binding, immune receptor activity, cargo receptor activity, and amide binding (Figure 4A,4B). The results of KEGG pathway analysis indicated that the DEGs were involved in cytokine−cytokine receptor interaction, tuberculosis, the NOD-like receptor signaling pathway, the chemokine signaling pathway, hematopoietic cell lineage, the B cell receptor-signaling pathway, and the Toll-like receptor signaling pathway (Figure 4C,4D). On the basis of these results, we concluded that the screened DEGs were an essential part of the immune system.
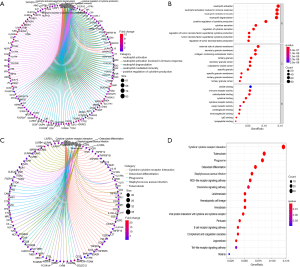
PPI network of DEGs
We generated a PPI network of the DEGs to explore the interaction between the selected genes The top 30 interacting genes were selected for further analysis. The results showed that ITGB2 was included in the top 30 interacting genes (Figure 5).
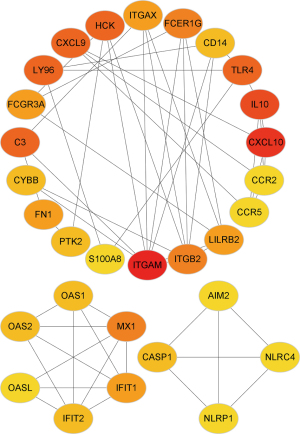
The prognostic value of DEGs in AML patients
Univariate Cox regression and multivariate Cox regression were used to explore the relationship between the selected DEGs in the intersection and prognosis in AML patients. Eleven genes were included in the forest analysis: KCNE1B (P=0.007), CD4 (P=0.063), ZNF385A (P=0.058), GNGT2 (P=0.052), KIR2DL4 (P=0.008), and UGT3A2 (P=0.077) appeared to be adverse biomarkers for AML, while ZSCAN23 (P=0.036), ABI3 (P=0.076), and ITGB2 (P=0.083) appeared to serve a protective role (Figure 6A). The calibration curve of the risk model (Figure 6B-6D). The genes expression levels in AML patients that significantly influence on the prognosis of AML patients based on the risk model (Figure 6E). The AML patients were divided into low and high-risk scores groups based on the risk models scores (Figure 6F). And the high-risk scores indicated the poor outcome of AML patients (Figure 6G,6H). The risk model showed accuracy for predicting prognosis in AML patients, with area under curve (AUC) at 1 year, 0.816; AUC at 3 years, 0.82; and AUC at 5 years, 0.875 (Figure 6I). This indicated that this model can predict the prognosis of AML patients.
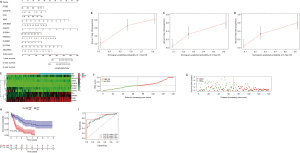
Construction of predicted models for assessing prognosis of AML via 11 genes
Risk modeling indicated that a high-risk score led to poor outcomes (P=3.076e−08) in AML patients. The risk model showed accuracy for predicting prognosis in AML patients, with area under curve (AUC) at 1 year, 0.816; AUC at 3 years, 0.82; and AUC at 5 years, 0.875 (Figure 7).
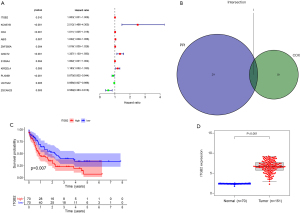
Immune cell infiltration in AML patients
We further analyzed the relationships between different immune cell infiltrates in the individual sample and the TCGA AML cohort (Figure 8A,8B). The results showed that non-activated (M0) macrophages were the most important negative regulator for resting dendritic cells, activated (M1) macrophages, activated CD4 memory T cells, resting CD4 memory T cells, CD8 T cells, monocytes, naive B cells, eosinophils, resting mast cells, and plasma cells, while CD8 T cells may be a positive regulator for memory B cells, resting dendritic cells, M1 macrophages, activated CD4 memory T cells, resting CD4 memory T cells, and monocytes. We then divided the AML patients into 2 groups based on mean ITGB2 expression and explored the relationship between ITGB2 expression levels and immune infiltrates. The association between ITGB2 and the different immune cell infiltrates showed that higher ITGB2 expression levels indicated elevated monocytes (P<0.001) and M2 macrophages (P=0.004) infiltrates, while lower expression levels led to higher infiltration of resting mast cells (P<0.001), eosinophils (P=0.001), resting CD4 memory cells (P<0.001), plasma cells (P<0.001), naive B cells (P<0.001), CD8 T cells (P=0.002), and resting NK cells (P=0.001) (Figure 9). We then investigated the prognostic role of different immune cell infiltrates in AML. The results showed that elevated resting mast cells led to favorable prognosis (P<0.001), while other immune cell infiltrate levels did not have a significant influence on prognosis (Figure 10).
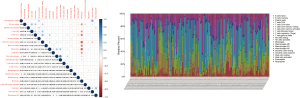
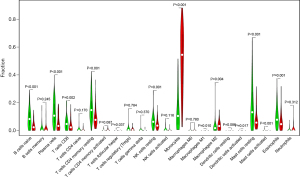
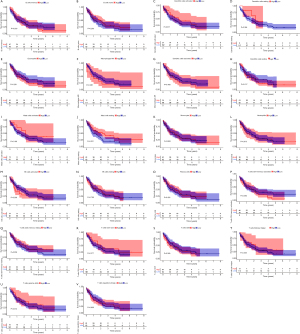
Immunohistochemistry
We collected samples from newly diagnosed AML patients in our department and compared ITGB2 expression levels between AML and normal samples. The results showed that AML patients had significantly higher ITGB2 expression levels than normal samples (Figure 11).
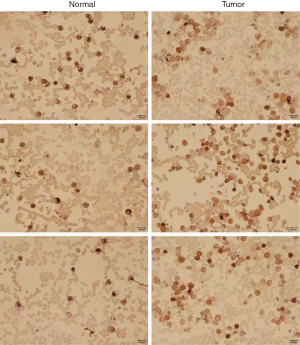
Drug sensitivity analysis
The results of drug sensitivity analysis for ITGB2 genes showed that patients with high-risk scores may be sensitive to cytarabine (P=0.0088), axitinib (P=6.5e-05), bosutinib (P=0.00011), docetaxel (P=0.011), bryostatin 1 (P=0.02), etoposide (P=0.027), imatinib (P=0.0043), lenalidomide (P=0.0027), vinblastine (P=0.013), tipifarnib (P=0.038), nilotinib (P=0.0015), midostaurin (P=7e-06), and vorinostat (P=0.0025) but resistant to cisplatin (P=0.0014), bortezomib (P=0.037), dasatinib (P=0.024), and lapatinib (P=0.036) (Figure 12). These results indicated the ITGB2 has a significant correlation with chemotherapy and target therapy regimens for AML.
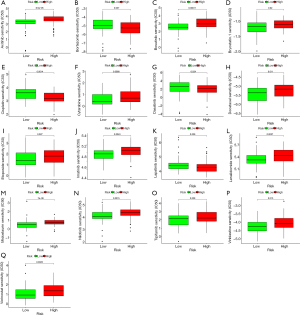
Discussion
TME plays a critical role in tumorigenesis, progression, and therapy resistance in various cancers. The main contents of TME include tumor cells, immune cells, and stromal cells. Among these, immune cells may be the most significant regulator of tumor progression (10), which has been well documented in multiple cancer types (11-13). Immune cell infiltration is influenced by several factors. irgs may be the main regulator of immune cell infiltrates into TME. Many studies have found that irgs act as a significant prognostic biomarker for cancer by regulating TME (14-17). There is considerable research on TME for solid tumors but few studies have investigated the correlation between TME and prognosis, therapy response, and disease progression in AML patients. This study aimed to investigate the function and prognostic role of significant irgs in AML patients.
In the present study, we divided the analysis process into a discovery phase and 2 validation phases. First, ITGB2 was screened as the key immune-related gene for AML patients. In the validation phases, overexpression of ITGB2 was related to poor prognosis in AML patients. The integrin lymphocyte function-associated antigen 1 (CD11A/CD18; ITGAL/ITGB2) is an essential regulator for lymphocyte trafficking and activation, and increasing lymphocyte residence in the lymph nodes (18). ITGB2 expresses in various cells, including neutrophils, T-lymphocytes, B-lymphocytes, monocytes, and macrophages (19-23). With various blood cells involved in its expression, ITGB2 may be an important regulator for immune cell infiltration and a therapeutic target for hematological malignancies.
Zhang et al. found that ITGB2 was significantly overexpressed in cancer-associated fibroblasts and that the higher the expression level the more advanced the status of oral squamous cell carcinoma (OSCC), with ITGB2 acting as a promotor in OSCC (24). Puerkaiti et al. observed overexpression of ITGB2 in triple negative breast cancer (TNBC), and also that the expression level of ITGB2 was significantly correlated to cancer stage, local metastasis, and prognosis (25). In hematological malignancies, ITGB2 has been correlated with chronic lymphocytic leukemia (CLL) and myeloma (26-28), with investigation into the role of ITGB2 in CLL currently at the preliminary stage. A study by Hutterer et al. revealed that ITGB2 expression in CLL was regulated via DNA methylation-dependent or -independent mechanisms, and upregulation of ITGB2 promoted CLL cell growth (26). Blackburn et al. further explored ITGB2 expression levels in different hematological malignancy subtypes (29). However, no studies have investigated the underlying mechanism of ITGB2 in AML. The potential function and role of ITGB2 in cancer remains unclear and further research on the relationship between ITGB2 and cancer is needed.
This study found that ITGB2 acted as a key immune-related gene and had an adverse signature in AML patients. We investigated the relationship between ITGB2 and immune cell infiltrates. The results showed that the higher the expression of ITGB2, the lower the infiltration of resting mast cells in AML, while the higher infiltration of resting mast cells acted as a protector in AML patients. Further, results of Gene Expression Profiling Interactive Analysis (GEPIA) also showed that overexpression of ITGB2 indicated poor outcomes in AML patients. These results indicated that ITGB2 expression levels could influence the prognosis of AML via regulation of immune cell infiltration. To date, no study has investigated the association between resting mast cells and cancer. Research by Frossi et al. has revealed mast cells as a significant regulator for innate and adaptive immune responses (30). Further research exploring the relationship between mast cells and AML is still needed. To confirm the difference in protein expression levels of ITGB2 between AML and normal samples, we collected AML and normal bone marrow for immunohistochemistry. The results showed that ITGB2 had higher expression levels in AML bone marrow than normal samples.
The final objective of this study was to provide useful information for clinicians in choosing reasonable therapy regimens for cancer patients. Therapy response in AML patients is influenced by multiple factors, such as subtypes, molecular biology, and cytogenetics, with molecular biology mainly involving genetic aberrations. With advances in next generation sequencing, genetic aberrations in AML patients have been identified, with FMS-related tyrosine kinase 3 (FLT3), nucleophosmin (NPM1), and DNA methyltransferase 3A (DNMT3A) mutations found in over 25% of AML patients (31). Several genetic aberrations have been observed to have significant correlations with drug resistance (32,33). Our study found that ITGB2 displayed significant differential expression in AML compared to normal samples, and acted as a significant influencing factor on prognosis in AML patients. We also found correlations between ITGB2 and drug sensitivity in AML patients. Patients with high-risk scores were found to be sensitive to cytarabine, axitinib, bosutinib, docetaxel, bryostatin 1, etoposide, imatinib, lenalidomide, vinblastine, tipifarnib, nilotinib, midostaurin, and vorinostat but resistant to cisplatin, bortezomib, dasatinib, and lapatinib. These results are interesting, with prognosis analysis revealing that overexpression of ITGB2 led to a reduced lifespan in AML patients. However, drug sensitivity analysis showed that upregulation of ITGB2 may promote sensitivity to cytarabine, the cornerstone chemotherapy regimen. These results indicated that while ITGB2 affects prognosis in AML patients, through regulation of drug resistance, AML patients with an ITGB2 aberration may still be able to use traditional chemotherapy regimens. The results of drug sensitivity analysis can provide useful guidance when choosing therapy regimens for AML patients with an ITGB2 gene mutation.
Conclusions
Our study found that AML patients had significantly higher expression of ITGB2 than normal samples, and that overexpression of ITGB2 can lead to poor prognosis in AML patients. ITGB2 also has a significant correlation with immune cell infiltration in AML. Drug sensitivity analysis indicated that upregulation of ITGB2 was correlated with sensitivity to cytarabine, etoposide, midostaurin, and vorinostat, and resistance to cisplatin, bortezomib, dasatinib, and lapatinib, indicating the usefulness of ITGB2 as a drug sensitivity biomarker.
Acknowledgments
Funding: None.
Footnote
Reporting Checklist: The authors have completed the REMARK reporting checklist. Available at https://dx.doi.org/10.21037/atm-21-3641
Conflicts of Interest: All authors have completed the ICMJE uniform disclosure form (available at https://dx.doi.org/10.21037/atm-21-3641). The authors have no conflicts of interest to declare.
Ethical Statement: The authors are accountable for all aspects of the work in ensuring that questions related to the accuracy or integrity of any part of the work are appropriately investigated and resolved. The study was conducted in accordance with the Declaration of Helsinki (as revised in 2013).
Open Access Statement: This is an Open Access article distributed in accordance with the Creative Commons Attribution-NonCommercial-NoDerivs 4.0 International License (CC BY-NC-ND 4.0), which permits the non-commercial replication and distribution of the article with the strict proviso that no changes or edits are made and the original work is properly cited (including links to both the formal publication through the relevant DOI and the license). See: https://creativecommons.org/licenses/by-nc-nd/4.0/.
References
- Wiseman DH, Greystoke BF, Somervaille TC. The variety of leukemic stem cells in myeloid malignancy. Oncogene 2014;33:3091-8. [Crossref] [PubMed]
- Siegel RL, Miller KD, Jemal A. Cancer statistics, 2020. CA Cancer J Clin 2020;70:7-30. [Crossref] [PubMed]
- Pleyer L, Stauder R, Burgstaller S, et al. Azacitidine in patients with WHO-defined AML - results of 155 patients from the Austrian Azacitidine Registry of the AGMT-Study Group. J Hematol Oncol 2013;6:32. [Crossref] [PubMed]
- Aldoss I, Marcucci G. More options for older patients with acute myeloid leukemia: venetoclax in combination with low dose cytarabine. Chin Clin Oncol 2019;8:S25. [Crossref] [PubMed]
- Li X, Yin X, Wang H, et al. The combination effect of homoharringtonine and ibrutinib on FLT3-ITD mutant acute myeloid leukemia. Oncotarget 2017;8:12764-74. [Crossref] [PubMed]
- Cao J, Feng H, Ding NN, et al. Homoharringtonine combined with aclarubicin and cytarabine synergistically induces apoptosis in t(8;21) leukemia cells and triggers caspase-3-mediated cleavage of the AML1-ETO oncoprotein. Cancer Med 2016;5:3205-13. [Crossref] [PubMed]
- Barrett AJ. Understanding and harnessing the graft-versus-leukaemia effect. Br J Haematol 2008;142:877-88. [Crossref] [PubMed]
- van Galen P, Hovestadt V, Wadsworth Ii MH, et al. Single-Cell RNA-Seq Reveals AML Hierarchies Relevant to Disease Progression and Immunity. Cell 2019;176:1265-1281.e24. [Crossref] [PubMed]
- Carter BZ, Mak PY, Wang X, et al. An ARC-Regulated IL1β/Cox-2/PGE2/β-Catenin/ARC Circuit Controls Leukemia-Microenvironment Interactions and Confers Drug Resistance in AML. Cancer Res 2019;79:1165-77. [Crossref] [PubMed]
- Gajewski TF, Schreiber H, Fu YX. Innate and adaptive immune cells in the tumor microenvironment. Nat Immunol 2013;14:1014-22. [Crossref] [PubMed]
- Blanc C, Hans S, Tran T, et al. Targeting Resident Memory T Cells for Cancer Immunotherapy. Front Immunol 2018;9:1722. [Crossref] [PubMed]
- Constantino J, Gomes C, Falcão A, et al. Dendritic cell-based immunotherapy: a basic review and recent advances. Immunol Res 2017;65:798-810. [Crossref] [PubMed]
- Fujii SI, Shimizu K. Immune Networks and Therapeutic Targeting of iNKT Cells in Cancer. Trends Immunol 2019;40:984-97. [Crossref] [PubMed]
- Shi L, Huang Y, Huang X, et al. Analyzing the key gene expression and prognostics values for acute myeloid leukemia. Transl Cancer Res 2020;9:7284-98. [Crossref]
- Shen S, Wang G, Zhang R, et al. Development and validation of an immune gene-set based Prognostic signature in ovarian cancer. EBioMedicine 2019;40:318-26. [Crossref] [PubMed]
- Wang J, Yu S, Chen G, et al. A novel prognostic signature of immune-related genes for patients with colorectal cancer. J Cell Mol Med 2020;24:8491-504. [Crossref] [PubMed]
- Zhu B, Tse LA, Wang D, et al. Immune gene expression profiling reveals heterogeneity in luminal breast tumors. Breast Cancer Res 2019;21:147. [Crossref] [PubMed]
- Reichardt P, Patzak I, Jones K, et al. A role for LFA-1 in delaying T-lymphocyte egress from lymph nodes. EMBO J 2013;32:829-43. [Crossref] [PubMed]
- Kuwano Y, Spelten O, Zhang H, et al. Rolling on E- or P-selectin induces the extended but not high-affinity conformation of LFA-1 in neutrophils. Blood 2010;116:617-24. [Crossref] [PubMed]
- Hogg N, Laschinger M, Giles K, et al. T-cell integrins: more than just sticking points. J Cell Sci 2003;116:4695-705. [Crossref] [PubMed]
- Arana E, Harwood NE, Batista FD. Regulation of integrin activation through the B-cell receptor. J Cell Sci 2008;121:2279-86. [Crossref] [PubMed]
- Sumagin R, Prizant H, Lomakina E, et al. LFA-1 and Mac-1 define characteristically different intralumenal crawling and emigration patterns for monocytes and neutrophils in situ. J Immunol 2010;185:7057-66. [Crossref] [PubMed]
- Shrivastava A, Shishodia S, Sodhi A. Expression of LFA-1 adhesion molecules on cisplatin-treated macrophages. Biochim Biophys Acta 1998;1402:269-76. [Crossref] [PubMed]
- Zhang X, Dong Y, Zhao M, et al. ITGB2-mediated metabolic switch in CAFs promotes OSCC proliferation by oxidation of NADH in mitochondrial oxidative phosphorylation system. Theranostics 2020;10:12044-59. [Crossref] [PubMed]
- Puerkaiti P, Fan JJ, Ma BL. Effect of overexpression of integrin β2 on clinical prognosis in triple negative breast cancer. Zhonghua Yi Xue Za Zhi 2020;100:2358-62. [PubMed]
- Hutterer E, Asslaber D, Caldana C, et al. CD18 (ITGB2) expression in chronic lymphocytic leukaemia is regulated by DNA methylation-dependent and -independent mechanisms. Br J Haematol 2015;169:286-9. [Crossref] [PubMed]
- Tiao G, Improgo MR, Tausch E, et al. Analysis of ITGB2 rare germ line variants in chronic lymphocytic leukemia. Blood 2017;130:2443-4. [Crossref] [PubMed]
- Yang Q, Li K, Li X, et al. Identification of Key Genes and Pathways in Myeloma side population cells by Bioinformatics Analysis. Int J Med Sci 2020;17:2063-76. [Crossref] [PubMed]
- Blackburn NB, Marthick JR, Banks A, et al. Evaluating a CLL susceptibility variant in ITGB2 in families with multiple subtypes of hematological malignancies. Blood 2017;130:86-8. [Crossref] [PubMed]
- Frossi B, Mion F, Tripodo C, et al. Rheostatic Functions of Mast Cells in the Control of Innate and Adaptive Immune Responses. Trends Immunol 2017;38:648-56. [Crossref] [PubMed]
- Cancer Genome Atlas Research Network. Genomic and epigenomic landscapes of adult de novo acute myeloid leukemia. N Engl J Med 2013;368:2059-74. [Crossref] [PubMed]
- Hou P, Wu C, Wang Y, et al. A Genome-Wide CRISPR Screen Identifies Genes Critical for Resistance to FLT3 Inhibitor AC220. Cancer Res 2017;77:4402-13. [Crossref] [PubMed]
- Nechiporuk T, Kurtz SE, Nikolova O, et al. The TP53 Apoptotic Network Is a Primary Mediator of Resistance to BCL2 Inhibition in AML Cells. Cancer Discov 2019;9:910-25. [Crossref] [PubMed]
(English Language Editor: A. Muijlwijk)