Identification of diagnostic and prognostic signatures derived from preoperative blood parameters for oral squamous cell carcinoma
Introduction
Oral squamous cell carcinoma (OSCC) is the predominant type of oral malignancy that develops from the tongue, buccal, palate, and floor of the mouth, and poses a considerable clinical challenge as evidenced by its high mortality and morbidity (1,2). The past few decades have witnessed tremendous progress in therapeutic strategies against this malignancy, including in ablative surgery, radiotherapy, chemotherapy, and immunotherapy. However, the long-term survival of patients with OSCC has not been improved substantially, especially for those with advanced lesions at initial diagnosis (3). Currently, patient stratification, treatment selection, and prognostic prediction largely depend on tumor-node-metastasis (TNM) stage, histopathological characteristics of primary lesions, and cervical node metastases as determined by postoperative pathological examinations (4). However, the prognosis for patients within the same TNM stages varies remarkably, which might be in part explained by the imperfect specificity, sensitivity, and performance of these routine biomarkers. These facts highlight the urgent need to identify novel prognostic biomarkers with adequate performance and clinical feasibility. Moreover, preoperative biomarkers might be superior to those obtained after surgery due to their advantages in patient stratification and treatment planning before surgery.
Cancer-associated immunity, inflammation and nutrition have been recognized as emerging hallmarks underlying tumorigenesis and increasingly been exploited as diagnostic, prognostic and therapeutic targets with translational promise (5). Indeed, immunity, inflammation, and nutrition have been revealed to be intricately interrelated with tumorigenesis. The immune and inflammatory cells such as lymphocytes, macrophages, and neutrophils have been demonstrated to have potent protumorigenic or tumor-suppressive roles that critically involved in cancer initiation and progression (5,6). In particular, recent breakthroughs in cancer immunotherapy have revolutionized the current paradigm in cancer therapeutics: it has been shown that reinvigorating the infiltrating lymphocytes in situ via blocking immune checkpoints can confer remarkable benefits across multiple types of cancers (7). From the clinical perspective, immune, inflammatory, and nutritional status has also been identified as source for novel biomarkers in patient diagnosis and prognostic prediction. For example, lymphocyte counts (L), lymphocyte-monocyte ratio (LMR), neutrophil-lymphocyte ratio (NLR), platelet-lymphocyte ratio (PLR), systemic immune-inflammation index (SII), prognostic nutritional index (PNI), and C-reactive protein (CRP) have been developed and verified with prognostic values in a broad spectrum of cancers (8-12). In our previous studies, high SII and platelet-neutrophil-lymphocyte score (PNL), low PNI, high counts of platelets and neutrophils, and low lymphocyte counts were significantly associated with reduced survival in patients with resectable OSCC (12-14). In addition, most cancer-induced profound metabolic and physiological alterations that undermine patients’ nutritional status and nutrient intake have been found to ultimately result in severe malnutrition and cachexia (15). Other studies have indicated that malnutrition manifests as low albumin in peripheral blood and is associated with immune deficiency and unfavorable prognosis in patients with various cancers (16,17). However, these studies generally focused on single parameters in the blood. We hypothesized that a prognostic signature that integrates multiple immune, inflammatory, and nutritional parameters in the blood might capture cancer-related status more comprehensively and offer thus superior performance in diagnostic differentiation and prognostic prediction.
Previous studies have typically used classical statistical approaches such as Cox regression to identify cancer biomarkers. Recent introduction of machine learning into the biomedical field has shown superiority in biomarker screening, disease diagnosis, treatment planning, and prognostic estimation in oncology (18). Machine learning is a branch of artificial intelligence that uses a series of statistical, probabilistic, and optimization techniques that enable computers to “learn” from past examples and discover difficult-to-recognize patterns (19). Several pioneering reports have revealed that machine learning algorithms use recognized patterns to optimize the complex combination of multiple biomarkers, thereby improving the accuracy and sensitivity of prediction (20,21). We previously reported that prediction of cervical node metastasis in early oral cancer by machine learning had better performance than did conventional methods (22). These previous findings point to the utility of machine learning algorithms in the diagnosis and prognosis of cancer, including OSCC.
In the present study, we retrospectively collected 13 preoperative inflammatory, immunological, and nutritional parameters in blood (PIINPBs) reflecting the immune, inflammation, and nutritional status of patients with OSCC. We aimed to develop novel signatures using machine learning algorithms for accurate diagnosis and prognostic prediction for OSCC. We present the following article in accordance with the Transparent Reporting of a Multivariable Prediction Model for Individual Prognosis or Diagnosis (TRIPOD) reporting checklist (available at https://dx.doi.org/10.21037/atm-21-631).
Methods
OSCC patients and non-OSCC controls
A total of 486 patients with primary OSCC and 200 age- and gender-matched non-OSCC patients were screened and enrolled from the disease registry at the Department of Oral and Maxillofacial Surgery, Affiliated Stomatological Hospital of Nanjing Medical University between January 2010 and November 2019. Detailed criteria for patient inclusion were as follows: (I) patients with primary OSCC without prior history of treatment and who received radical resection of lesions and neck dissection when indicated; (II) patients without other tumors, infectious diseases, hematological diseases, autoimmune diseases, or severe liver/renal dysfunctions; and (III) detailed epidemiological, clinicopathological, and follow-up information available for these enrolled patients. Additionally, the criteria for patient exclusion were as follows: (I) patients with simultaneous steroid or other drugs which might affect the total amount of white blood cells, (II) patients with a history of any other malignancies, and (III) patients with inflammation or infectious diseases within 1 month prior to preoperative blood collection.
Histopathological grading of tumor and clinical staging of patients were assessed according to the World Health Organization (WHO) grading system and the American Joint Committee on Cancer (AJCC) 7th staging system, respectively. After undergoing ablative surgical treatment, the patients were followed up once every 3 months in the first 2 years, once every 6 months within 5 years, and then once every year thereafter. The overall survival (OS) and disease-free survival (DFS) were defined as the length of time between death, local recurrence, metastasis, or the last follow-up and initial ablative surgery. The age- and gender-matched nontumor patients served as controls who were diagnosed as noninfectious, nontumor diseases, such as supernumerary teeth, cysts in jaws, sialolithiasis, and sublingual gland cysts treated at the same period. In addition, the percentages of those who used alcohol and/or tobacco were similar between OSCC patients and non-OSCC controls. The study was conducted in accordance with the Declaration of Helsinki (as revised in 2013). The study was reviewed and approved by the Ethics and Research Committee of Nanjing Medical University (No. 2020-230), and individual consent for this retrospective analysis was waived.
We randomly divided the whole OSCC data set into the training cohort and validation cohort (training: validation =7:3) by using the createDataPartition function in caret package (6.0–86) until there was no significant difference (P>0.1) between these 2 cohorts in terms of clinicopathological variables. The non-OSCC data set was also split into 2 cohorts by a similar approach. Thus, there were 341 OSCC patients and 140 non-OSCC patients assigned to the training cohort, while 145 OSCC patients and 60 nontumor patients were allocated to the validation cohort (Table 1).
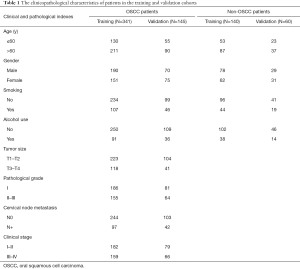
Full table
Data acquisition and preprocessing of PIINPBs
Routine blood was collected within 3 days of surgery, with results including white blood cell count (WBC), neutrophil count (N), monocyte count (M), lymphocyte count (L), platelet count (P), albumin (ALB), albumin–globulin ratio (A/G), and hemoglobin concentration (Hb). According to our own and other authors’ studies (13,14,23), the NLR, PLR, LMR, SII, and PNI were calculated as follows: NLR = N/L, PLR = P/L, LMR = L/M, SII = P × N/L, and PNI = ALB (g/L) + 5 × L (per mm3). The inflammatory and immunological parameters included WBC, N, M, L, P, NLR, PLR, LMR, and SII, while the nutritional parameters included ALB, A/G, Hb, and PNI. The clinical and pathological parameters of patients, including age, gender, tumor size, clinical stage, pathological grade, and cervical node metastasis were collected from medical charts.
Diagnostic model constructed by machine learning classifiers
In this study, 6 commonly used types of supervised machine learning classifiers including random forest (RF), support vector machine (SVM), extreme gradient boosting (XGBoost), naive Bayes (NBS), neural network (NN), and logistic regression (LR) were initially used for diagnostic model development. These machine learning approaches were developed by using R package “Random Forest” (4.6-12; The R Foundational for Statistical Computing) for RF, “e1071” package (1.7-3) for SVM and NB, “xgboost” package (1.1.1.1) for Xgboost, and “nnet” package (7.3-14) for NN. Classifiers were trained using repeated 10-fold cross-validations of the training cohort, and then their predictive performances were further evaluated in the validation cohort. In addition, the receiver operating characteristic (ROC) curve was plotted to evaluate the sensitivity and specificity of these classifiers via the “ROCR” package (1.0-9) in R software. All algorithms were implemented in R software (version 3.6.3).
Prognostic model construction by least absolute shrinkage and selection operator (LASSO)
Univariate Cox regression analyses were performed on 13 parameters of interest to identify survival-related variables with a P value <0.05 in the training cohort with “Survival” package (3.2-3). Then, LASSO analyses were employed to screen significant parameters and construct prognostic signatures as previously reported (24). During the LASSO procedure, the absolute value of the regression coefficients of the assessed variables was continuously reduced through the use of a penalty. With this penalty, which was the sum of the absolute size of the regression coefficients multiplied by a tuning parameter (lambda, λ), some coefficients were reduced to zero. The corresponding variables hold little predictive value and can be neglected during the fitting of the model. Variables with nonzero coefficients were extracted to construct the prognostic model. The risk score for each patient was calculated using the following formula: , where coefi is the coefficient of LASSO regression and xi is the value of each blood parameter.
Statistical analyses
All analyses were performed using the R software (version 3.6.3). Correlations between blood markers were evaluated using the Spearman rank coefficient, and the principal component analysis (PCA) was performed with the “pca3d package” (0.10.2). The LASSO analysis was performed with the “glmnet” package (4.0-2). Using the median risk score in the training cohort, we stratified patients into high-risk and low-risk subgroups. The Kaplan-Meier method was used to estimate the OS and DFS, and differences were compared using the logrank test. A time-dependent ROC curve with 3 and 5 years as the defining points was drawn with the “survivalROC” package to evaluate the predictive value of prognostic risk scores (1.0.3). Nomograms and calibration plots were drawn with the “rms” package (5.1-4), while decision curve analysis was conducted with the “stdca” package (1.2.1). Univariate and multivariate Cox regression analyses were employed to determine the prognostic factors associated with OS and DFS. All statistical tests were 2-sided and considered significant when the P value was less than 0.05.
Results
Patient characteristics
A total of 486 patients with primary OSCC who met our inclusion criteria were enrolled, with 341 in the training cohort and 145 in the validation cohort. The baseline characteristics of patients from the 2 cohorts were compared and are listed in detail in Table 1. Based on follow-up information, 128 and 51 deaths occurred in the training and validation cohorts, respectively. The number of patients alive but with evidence of local recurrence or metastases, were 29 and 12 in these 2 cohorts, respectively. Hence, the OS ratios were 62.4% and 64.8%, while the DFS ratios were 53.9% and 56.5% in the training and validation cohorts, respectively.
Diagnostic models developed by machine learning algorithms based on PIINPB
The workflow of our study and the data analytic pipeline are illustrated in Figure 1. Initially, we sought to determine whether differences in PIINPBs existed between OSCC and non-OSCC controls, and if they existed, whether these differences were able to differentiate OSCC from non-OSCC. We used multiple machine learning algorithms to build the diagnostic classifiers based on 13 PIINPBs including WBC, N, M, L, P, ALB A/G, Hb, NLR, PLR, LMR, SII, and PNI. The results showed that the predictive accuracy of 6 machine learning algorithms were 87.3% (SVM), 84.6% (Xgboost), 80.5% (NBs), 83.8% (NN), 82.5% (LR), and 80.5% (RF) in the training cohort, respectively. As shown in Figure 2A, the highest AUC value was 0.846 with SVM, followed by 0.823 with Xgboost, 0.819 with NBs, 0.799 with NN, 0.775 with LR, and 0.744 with RF. Moreover, these findings were validated using data from the validation cohort. SVM, Xgboost, and NBs classifiers exhibited better performance in segregating OSCC from non-OSCC than did the other classifiers (Figure 2B). Next, the relative importance of variables of interest in differentiating OSCC from non-OSCC was calculated within these 3 predictive approaches. As shown in Figure S1, PNI, ALB, LMR, lymphocyte, and PLR were identified as the top important factors in differential diagnosis classifiers. To complement this, violin plots were used to show the distributions of each variable between OSCC and non-OSCC patients: most of these markers except WBC, were significantly different between these 2 cohorts. In particular, PNI was a critical variable in all analytical approaches, while the importance of other variables substantially varied among different models. Moreover, results from PCA indicated obvious differences between OSCC and non-OSCC (Figure 2C). In addition, as shown in Figure 2D, there were positive correlations between ALB and PNI, PLR and SII, and SII and NLR, and negative correlations between PLR and NLR, and PNI, lymphocyte count and LMR. However, several supervised machine learning algorithms, including RF, SVM, and Xgboost were resistant to multicollinearity interference and showed excellent predictive performance (21).
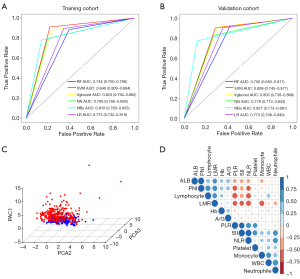
Next, we attempted to determine whether the classifiers derived from these preoperative parameters were able to predict clinical stages of OSCC. Our results showed that the predictive accuracy of the 6 machine learning algorithms was 83.6% (Xgboost), 81.1% (SVM), 80.6% (NN), 74.5% (LR), 74.2% (NBs), and 70.4% (RF) in the training cohort. As shown in Figure 3A, the highest AUC value was 0.833 with Xgboost, followed by 0.812 with SVM, 0.807 with NN, 0.725 with LR, 0.724 with NBs, and 0.680 with RF. Noticeably, these classifiers also showed robustness using data from the validation cohort (Figure 3B). As expected, these parameters substantially differed between patients with stage I/II and III/IV diseases (Figure S2). We further aimed to determine whether these classifiers were able to predict pathological grades of OSCC. The results indicated that the predictive accuracy of 6 algorithms was 85.9% (NN), 84.7% (SVM), 85.1% (Xgboost), 82.7% (LR), 82.1% (NBs), and 80.1% (RF) in the training cohort. As shown in Figure 3C, the highest AUC value was 0.854 with NN, followed by 0.842 with SVM, 0.838 with Xgboost, 0.833 with LR, 0.818 with NBs, and 0.791 with RF. These findings were also supported from results in the validation cohort (Figure 3D). As expected, these variables, except for Hb and P, significantly differed between patients with grade I and grade II/III diseases (Figure S3).
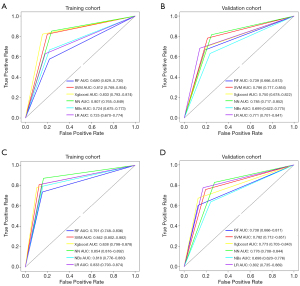
Construction of a prognostic signature for OSCC based on PIINPB
We initially performed the univariate Cox regression analyses in these 13 parameters and identified 10 survival-related parameters including L, P, N, A/G, ALB, PLR, NLR, LMR, PNI, and SII (P value <0.05) using data from the training cohort. Next, the LASSO-Cox regression analyses were undertaken to identify 6 key parameters affecting patient prognosis (Figure 4A,4B). The coefficients of these 6 parameters were as follows: L, –0.0881252384; P, 0.0026305767; PNI, –0.1241620762; LMR, 0.0372959535; SII, 0.0003013864; and A/G, –0.0703668172. Subsequently, a prognostic risk score for each patient was developed based on the following formula: risk score= (–0.0881252384) × lymphocyte count + (0.0026305767) × platelet + (–0.1241620762) × PNI + (0.0372959535) × LMR + (0.0003013864) × SII + (–0.0703668172) × A/G. Patients in the training cohort were stratified into subgroups with high-risk or low-risk scores. The Kaplan-Meier analyses indicated that patients in the high-risk subgroup had significantly shorter OS than did those in the low-risk subgroup in both the training and validation cohorts (Figure 4C,4D). The time-dependent ROC curve revealed that this prognostic model was robust in predicting patient OS, with a 3- and 5-year AUC of 0.806 and 0.822 in the training cohort and 0.791 and 0.767 in the validation cohort (Figure 4E,4F). Consistently, similar findings were observed in predicting patient DFS in both cohorts (Figure 5). To further verify the values of this prognostic risk score, we carried out univariate and multivariate Cox regression analyses. Not surprisingly, our data identified the risk score as an important factor affecting patient survival in both cohorts (Figure 6). Moreover, as illustrated in Figure 7, results from the multivariate Cox regression analyses revealed that the proposed risk score was an independent factor affecting patient survival in both cohorts after adjustment were made for well-established prognostic factors, like clinical stage, pathological grade, tumor size, and cervical node metastasis. Together, these findings support the clinical value of a risk score established from routine presurgical blood parameters in OSCC prognostication.
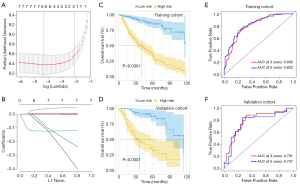
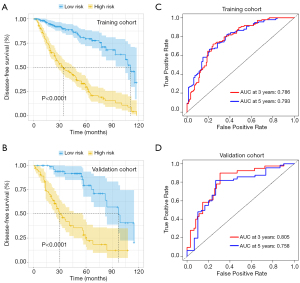
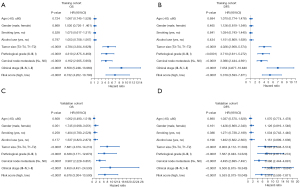
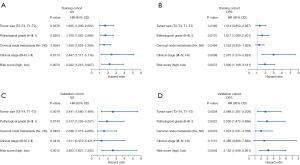
To develop a more robust prognostic nomogram, we set out to integrate the abovementioned prognostic signature with additional routine clinicopathological parameters (Figure 8A). As shown in Figure 8B, the nomogram worked well in predicting patient survival as evidenced by the AUC of 3- and 5-year time-dependent ROC of 0.825 and 0.845, respectively. Moreover, the calibration curve of this nomogram showed good agreement between prediction and clinical observation in both cohorts (Figure 8C,8D). In addition, decision curve analyses were performed for this nomogram, pathological grade, and clinical stage. Results revealed that the nomogram established here showed a higher net benefit and better predictive accuracy than did pathological grade and clinical stage (Figure 8E,8F).
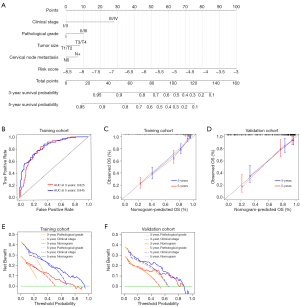
Discussion
Unfavorable long-term prognosis in OSCC highlights the urgent need to identify more simple, accurate, and convenient biomarkers to facilitate early diagnosis, patient stratification, treatment guidelines, and prognostic prediction. Mounting evidence has demonstrated that inflammatory, immunological, and nutritional factors are critically involved in tumor initiation, progression, recurrence, and metastatic spread, which affect not only in tumor sites, but also blood circulation (5,25). Importantly, these parameters in pretreatment blood circulation hold great translational potential as biomarkers for patient diagnosis, prognostic estimation, and therapeutic response (26-28). Here, we developed both diagnostic and prognostic models for OSCC by integrating 13 preoperative parameters from routine blood examinations via machine learning algorithms and LASSO, respectively. Our results strongly suggest that these novel optimized diagnostic and prognostic signatures had superior performance, thus warranting further validation and clinical translation.
Under current clinical settings, OSCC diagnosis largely depends on histopathological examinations in samples obtained from pretreatment biopsy or postoperative resected lesions. The advent and increasing popularization of liquid biopsy allows presurgical measurements of cells, proteins, DNA and RNA molecules, and exosomes for early diagnosis of cancer, representing a viable alternative and complement to routine examinations for disease diagnosis and differential diagnosis (29). For example, circulating tumor cells were found to be an independent prognostic marker predicting relapse with higher sensitivity than routine staging procedures in OSCC (30). RNA sequencing of tumor-educated blood platelets can distinguish cancer patients from healthy individuals and differentiate between 6 primary tumor types of patients with high accuracy (31). Furthermore, machine learning, a branch of artificial intelligence, has played an increasingly important role in cancer diagnosis and prognosis prediction. For example, several models and classifiers with considerable translational potential have been developed by machine learning to predict the lymph node metastasis, survival, and hypoxia-immune microenvironment in OSCC (22,32,33). Here, we retrospectively enrolled OSCC patients and age and gender- matched non-OSCC patients and constructed a diagnostic signature based on PIINPBs via multiple machine learning algorithms. Our results revealed that most models developed from diverse machine learning algorithms had potent ability to discriminate between OSCC and non-OSCC in both the training and validation cohorts. Notably, models derived from SVM, Xgboost, and NBs seemed more stable and had better performance than did other algorithms as evidenced from the AUCs. In addition, we compared the abilities of 6 algorithms to discriminate between patients in terms of pathological grade and clinical stage. Our data indicated that these algorithms robustly stratified patients into subgroups with a different pathological grade and clinical stage. These findings suggest that important information regarding OSCC might be more easily obtained from pretreatment blood parameters followed by machine learning as compared to postoperative data. This might be helpful for clinicians to stratify patients and select individualized treatment options. Of course, much work is needed to optimize and standardize this diagnostic pipeline for OSCC diagnosis and further verify its feasibility and efficiency in the routine clinical practice.
Although tremendous progress has been made in OSCC therapy during the past decades, long-term prognosis in patients with OSCC remains dismal. The prognostic prediction mainly relies on conventional TNM staging and select clinicopathological parameters. However, these prognostic factors are far from optimal in offering accurate information, since survival substantially varies among patients with the same TNM stage. Previous studies have usually focused on a single or a few parameters in blood to identify prognostic predictors for cancer (9,17,34). We previously reported that high counts of circulating P, N, and low P were significantly associated with reduced survival in patients with OSCC (13). Moreover, systemic inflammatory indexes such as NLR, PLR, LMR, and SII calculated by N, M, P, and L, have been demonstrated to be associated with cancer prognosis (12,23,35). However, this prognostic model derived from a single parameter might be limited in its accuracy and sensitivity. To address this, we constructed a novel prognostic signature derived from routine blood parameters and found that this signature robustly stratified patients into subgroups with high or low survival. Moreover, to further improve the accuracy of our prognostic signature, we integrated it with other well-established clinicopathological parameters into a nomogram. Our results indicate that this nomogram outperformed all other parameters, thus supporting its value and translational potential as a novel prognostic biomarker. It is reasonable to assume that this analytic approach can enable simultaneous integration of multiple parameters and may impact patient prognosis, which together enhances accuracy and sensitivity of prognostic prediction.
The diagnostic and prognostic significance of these blood parameter-derived biomarkers highlights the key roles of inflammatory, immunological, and nutritional parameters in the blood on tumorigenesis. Moreover, these parameters might, at least in part, reflect the status of host response malignancies and systemic effects induced by tumor. For example, one study found that peripheral blood monocytes from bone marrow are recruited locally and then differentiate into tumor-associated macrophages in response to chemokines produced by tumor cells. These tumor-associated macrophages promote tumor initiation and metastasis, inhibit antitumor immune responses mediated by T cells, and stimulate tumor angiogenesis and subsequently tumor progression in diverse cancer contexts (36). Moreover, platelet-derived transforming growth factor beta (TGF-β) and direct platelet–tumor cell contacts synergistically activate the TGF-β/Smad and necrosis factor kappa-B (NF-κB) pathways, resulting in their transition to an invasive mesenchymal-like phenotype and enhanced metastasis in vivo (31,37). Given the high complexity and heterogeneity of blood parameters, our classifiers based on routine blood parameters may not provide enough information to identify OSCC-specific inflammatory or immune status. Large data sets from high-throughput multiomics analyses, such as genomewide RNA sequencing and metabolomics in preoperative peripheral blood, may facilitate the identification of the OSCC-specific inflammatory/immune state.
The advantages of both diagnostic and prognostic models established here lie in the integrative analyses of multiple parameters rather than in individual ones, as reported in previous studies (8,38). This type of integrative analysis simultaneously covers multiple parameters related to diverse aspects of the disease and host, which might contribute to its effectiveness and performance. Moreover, the powerful machine learning algorithms based on artificial intelligence further enhanced the accuracy and efficiency of models developed here. Among 6 machine learning algorithms used for model development, results from SVM, Xgboost, and NN were relatively stable and consistent. However, which machine learning algorithm is best suited for model construction still needs to be determined by further study.
Nonetheless, there are several limitations in our study. First, the number of patients with OSCC is relatively small, and these diagnostic and prognostic models need to be independently validated in multiple cohorts. Second, this study was a retrospective analysis and inevitably had selection bias. However, our research design of training-validation cohorts might have partially compensated for these disadvantages. Third, some key prognostic factors such as margin status, depth of invasion (DOI), presence of extracapsular extension, or perineural invasion were not included in the univariate and multivariate survival analyses. Furthermore, the aim of our present study was to integrate preoperative blood parameters to develop a novel and potent prognostic signature for individualized treatment planning and prognostication. Therapeutic options which might significantly affect prognosis among patients were not included. Finally, although machine learning algorithms have inherent advantages in data processing and analyses, they cannot provide detailed information on decision-making processes, reflecting their black box nature. Collectively, these weaknesses necessitate further optimization and independent validation of these signatures before they can be applied in routine clinical practice.
Conclusions
We developed novel diagnostic and prognostic signatures for OSCC based on preoperative routine blood parameters with satisfactory accuracy and sensitivity, and further confirmed that inflammatory, immunological, and nutritional parameters in blood have clinical significance and potential biological functions in driving tumorigenesis. Our findings indicate that machine learning algorithms can be successfully leveraged for biomarker discovery and data integration and analyses. More research is warranted to further validate our findings in multiple, independent OSCC cohorts.
Acknowledgments
Funding: This work was financially supported by the National Natural Science Foundation of China (No. 82072991), the Key Research Program in Jiangsu Province-Social Developmental Project (No. BE2020706), the Priority Academic Program Development of Jiangsu Higher Education Institutions (No. 2018-87), and Qing-Lan Project and Jiangsu Young Medical Talent Project (No. QNRC2016851).
Footnote
Reporting Checklist: The authors have completed the TRIPOD reporting checklist. Available at https://dx.doi.org/10.21037/atm-21-631
Data Sharing Statement: Available at https://dx.doi.org/10.21037/atm-21-631
Peer Review File: Available at https://dx.doi.org/10.21037/atm-21-631
Conflicts of Interest: All authors have completed the ICMJE uniform disclosure form (available at https://dx.doi.org/10.21037/atm-21-631). The authors have no conflicts of interest to declare.
Ethical Statement: The authors are accountable for all aspects of the work in ensuring that questions related to the accuracy or integrity of any part of the work are appropriately investigated and resolved. The study was conducted in accordance with the Declaration of Helsinki (revised in 2013). The study was reviewed and approved by the Ethics and Research Committee of Nanjing Medical University (No. 2020-230), and individual consent for this retrospective analysis was waived.
Open Access Statement: This is an Open Access article distributed in accordance with the Creative Commons Attribution-NonCommercial-NoDerivs 4.0 International License (CC BY-NC-ND 4.0), which permits the non-commercial replication and distribution of the article with the strict proviso that no changes or edits are made and the original work is properly cited (including links to both the formal publication through the relevant DOI and the license). See: https://creativecommons.org/licenses/by-nc-nd/4.0/.
References
- Chinn SB, Myers JN. Oral Cavity Carcinoma: Current Management, Controversies, and Future Directions. J Clin Oncol 2015;33:3269-76. [Crossref] [PubMed]
- Siegel RL, Miller KD, Jemal A. Cancer statistics, 2019. CA Cancer J Clin 2019;69:7-34. [Crossref] [PubMed]
- Chi AC, Day TA, Neville BW. Oral cavity and oropharyngeal squamous cell carcinoma--an update. CA Cancer J Clin 2015;65:401-21. [Crossref] [PubMed]
- Okuyemi OT, Piccirillo JF, Spitznagel E. TNM staging compared with a new clinicopathological model in predicting oral tongue squamous cell carcinoma survival. Head Neck 2014;36:1481-9. [Crossref] [PubMed]
- Shalapour S, Karin M. Immunity, inflammation, and cancer: an eternal fight between good and evil. J Clin Invest 2015;125:3347-55. [Crossref] [PubMed]
- Diakos CI, Charles KA, McMillan DC, et al. Cancer-related inflammation and treatment effectiveness. Lancet Oncol 2014;15:e493-503. [Crossref] [PubMed]
- Fritz JM, Lenardo MJ. Development of immune checkpoint therapy for cancer. J Exp Med 2019;216:1244-54. [Crossref] [PubMed]
- Vähämurto P, Pollari M, Clausen MR, et al. Low Absolute Lymphocyte Counts in the Peripheral Blood Predict Inferior Survival and Improve the International Prognostic Index in Testicular Diffuse Large B-Cell Lymphoma. Cancers (Basel) 2020;12:1967. [Crossref] [PubMed]
- Xu W, Wu X, Wang X, et al. Prognostic Significance of the Preoperative Lymphocyte to Monocyte Ratio in Patients with Gallbladder Carcinoma. Cancer Manag Res 2020;12:3271-83. [Crossref] [PubMed]
- Valero C, Zanoni DK, McGill MR, et al. Pretreatment peripheral blood leukocytes are independent predictors of survival in oral cavity cancer. Cancer 2020;126:994-1003. [Crossref] [PubMed]
- Okadome K, Baba Y, Yagi T, et al. Prognostic Nutritional Index, Tumor-infiltrating Lymphocytes, and Prognosis in Patients with Esophageal Cancer. Ann Surg 2020;271:693-700. [Crossref] [PubMed]
- Diao P, Wu Y, Li J, et al. Preoperative systemic immune-inflammation index predicts prognosis of patients with oral squamous cell carcinoma after curative resection. J Transl Med 2018;16:365. [Crossref] [PubMed]
- Diao P, Wu Y, Ge H, et al. Preoperative circulating platelet, neutrophil, and lymphocyte counts predict survival in oral cancer. Oral Dis 2019;25:1057-66. [Crossref] [PubMed]
- Wu X, Jiang Y, Ge H, et al. Predictive value of prognostic nutritional index in patients with oral squamous cell carcinoma. Oral Dis 2020;26:903-11. [Crossref] [PubMed]
- Vandebroek AJ, Schrijvers D. Nutritional issues in anti-cancer treatment. Ann Oncol 2008;19:v52-5. [Crossref] [PubMed]
- Djaladat H, Bruins HM, Miranda G, et al. The association of preoperative serum albumin level and American Society of Anesthesiologists (ASA) score on early complications and survival of patients undergoing radical cystectomy for urothelial bladder cancer. BJU Int 2014;113:887-93. [Crossref] [PubMed]
- Gupta D, Lis CG. Pretreatment serum albumin as a predictor of cancer survival: a systematic review of the epidemiological literature. Nutr J 2010;9:69. [Crossref] [PubMed]
- Bera K, Schalper KA, Rimm DL, et al. Artificial intelligence in digital pathology - new tools for diagnosis and precision oncology. Nat Rev Clin Oncol 2019;16:703-15. [Crossref] [PubMed]
- Huang S, Yang J, Fong S, et al. Artificial intelligence in cancer diagnosis and prognosis: Opportunities and challenges. Cancer Lett 2020;471:61-71. [Crossref] [PubMed]
- Kourou K, Exarchos TP, Exarchos KP, et al. Machine learning applications in cancer prognosis and prediction. Comput Struct Biotechnol J 2015;13:8-17. [Crossref] [PubMed]
- Kawakami E, Tabata J, Yanaihara N, et al. Application of Artificial Intelligence for Preoperative Diagnostic and Prognostic Prediction in Epithelial Ovarian Cancer Based on Blood Biomarkers. Clin Cancer Res 2019;25:3006-15. [Crossref] [PubMed]
- Shan J, Jiang R, Chen X, et al. Machine Learning Predicts Lymph Node Metastasis in Early-Stage Oral Tongue Squamous Cell Carcinoma. J Oral Maxillofac Surg 2020;78:2208-18. [Crossref] [PubMed]
- Szkandera J, Gerger A, Liegl-Atzwanger B, et al. The lymphocyte/monocyte ratio predicts poor clinical outcome and improves the predictive accuracy in patients with soft tissue sarcomas. Int J Cancer 2014;135:362-70. [Crossref] [PubMed]
- Tibshirani R. Regression shrinkage and selection via the lasso: a retrospective. J R Statist Soc B 2011;73:273-82. [Crossref]
- Yoshida N, Harada K, Baba Y, et al. Preoperative controlling nutritional status (CONUT) is useful to estimate the prognosis after esophagectomy for esophageal cancer. Langenbecks Arch Surg 2017;402:333-41. [Crossref] [PubMed]
- Okugawa Y, Toiyama Y, Yamamoto A, et al. Lymphocyte-C-reactive Protein Ratio as Promising New Marker for Predicting Surgical and Oncological Outcomes in Colorectal Cancer. Ann Surg 2020;272:342-51. [Crossref] [PubMed]
- Karimi S, Vyas MV, Gonen L, et al. Prognostic significance of preoperative neutrophilia on recurrence-free survival in meningioma. Neuro Oncol 2017;19:1503-10. [Crossref] [PubMed]
- Lee JS, Park S, Park JM, et al. Elevated levels of preoperative CA 15-3 and CEA serum levels have independently poor prognostic significance in breast cancer. Ann Oncol 2013;24:1225-31. [Crossref] [PubMed]
- Crowley E, Di Nicolantonio F, Loupakis F, et al. Liquid biopsy: monitoring cancer-genetics in the blood. Nat Rev Clin Oncol 2013;10:472-84. [Crossref] [PubMed]
- Gröbe A, Blessmann M, Hanken H, et al. Prognostic relevance of circulating tumor cells in blood and disseminated tumor cells in bone marrow of patients with squamous cell carcinoma of the oral cavity. Clin Cancer Res 2014;20:425-33. [Crossref] [PubMed]
- Best MG, Sol N, Kooi I, et al. RNA-Seq of Tumor-Educated Platelets Enables Blood-Based Pan-Cancer, Multiclass, and Molecular Pathway Cancer Diagnostics. Cancer Cell 2015;28:666-76. [Crossref] [PubMed]
- Alhazmi A, Alhazmi Y, Makrami A, et al. Application of artificial intelligence and machine learning for prediction of oral cancer risk. J Oral Pathol Med 2021;50:444-50. [Crossref] [PubMed]
- Zeng H, Luo M, Chen L, et al. Machine learning analysis of DNA methylation in a hypoxia-immune model of oral squamous cell carcinoma. Int Immunopharmacol 2020;89:107098 [Crossref] [PubMed]
- Ray-Coquard I, Cropet C, Van Glabbeke M, et al. Lymphopenia as a prognostic factor for overall survival in advanced carcinomas, sarcomas, and lymphomas. Cancer Res 2009;69:5383-91. [Crossref] [PubMed]
- Shi M, Zhao W, Zhou F, et al. Neutrophil or platelet-to-lymphocyte ratios in blood are associated with poor prognosis of pulmonary large cell neuroendocrine carcinoma. Transl Lung Cancer Res 2020;9:45-54. [Crossref] [PubMed]
- Yang L, Zhang Y. Tumor-associated macrophages: from basic research to clinical application. J Hematol Oncol 2017;10:58. [Crossref] [PubMed]
- Labelle M, Begum S, Hynes RO. Direct signaling between platelets and cancer cells induces an epithelial-mesenchymal-like transition and promotes metastasis. Cancer Cell 2011;20:576-90. [Crossref] [PubMed]
- Yuk HD, Kang M, Hwang EC, et al. The platelet-to-lymphocyte ratio as a significant prognostic factor to predict survival outcomes in patients with synchronous metastatic renal cell carcinoma. Investig Clin Urol 2020;61:475-81. [Crossref] [PubMed]