An excellent nomogram predicts microvascular invasion that cannot independently stratify outcomes of small hepatocellular carcinoma
Introduction
Hepatocellular carcinoma (HCC) is the sixth most prevalent neoplasm and the third leading cause of cancer death (1). The 5-year overall survival (OS) of HCC is approximately 10–20% (2-4), with 5-year recurrence rate after liver resection reaching 50–70% (5). Increasing evidence shows that microvascular invasion (MVI) impairs the surgical outcome (6,7), and tumor size is closely correlated to the incidence of MVI (7) and poor prognosis (8). This implies tumor size may be a potential confounder—a major threat to the retrospective study’s validity—for MVI in predicting prognosis. Meanwhile, early diagnosis of MVI in patients with HCC, especially small HCC (sHCC), will enable clinicians to better understand its pathobiological behaviour for optimizing therapeutic regimens and improving long-term survival.
The definition of sHCC varies greatly owing to its confusing criteria of the maximum tumor diameter from 2 to 5 cm (9-13). Recent studies have indicated that tumor growth over 3 cm is a critical turning point towards more aggressive behaviour (10,12,13). MVI presents in 15–57.1% surgical specimens of HCC and is only visible under microscopy (14). But a wide resection margin is recommended for MVI-positive patients (15). Hence, the effective prediction of histopathological MVI and the identification of key prognostic indicators before surgery can tailor the initial treatment plan to achieve the maximum benefit in sHCC patients.
Recently, some preoperative imaging traits (e.g., peritumoral enhancement) and radiomics features (e.g., texture signatures) have shown great discrimination in the diagnosis of MVI (6,14-16). But few studies focus on the tumor size within 3 cm. Compared with extracellular agents, gadoxetate disodium (Gd-EOB-DTPA) with hepatocyte-specific properties can not only reflect the vascularity of HCC lesions, but also can effectively identify small/early HCC (17-19). Additionally, few studies (20,21) have noticed the application of the Liver Imaging Reporting and Data System (LI-RADS) in MVI or outcome, especially for sHCC patients underwent Gd-EOB-DTPA MRI. Meanwhile, previous studies concerning MVI in HCC patients are retrospective analysis, which cannot avoid selective bias.
Therefore, this study aimed to explore the preoperative value of Gd-EOB-DTPA MRI based on LI-RARS for predicting MVI in patients with sHCC ≤3 cm, and to investigate the correlation between MVI and prognosis by propensity score matching (PSM). We present the following article in accordance with the TRIPOD reporting checklist (available at http://dx.doi.org/10.21037/atm-20-7952).
Methods
Study population
This retrospective, single-center, cohort study was conducted in accordance with the Declaration of Helsinki (as revised in 2013). The study was approved and informed consent was waived by the Ethical Review Committee of Zhongshan Hospital, Fudan University, Shanghai, China (approval No. B2018-236). Between March 2012 and September 2020, 455 consecutive patients with pathologically confirmed sHCC ≤3 cm underwent liver resection and preoperative Gd-EOB-DTPA MRI, who met the following inclusion criteria (Figure 1A): (I) without gross vascular invasion, bile duct tumor thrombosis or extrahepatic metastasis upon preoperative imaging; (II) without previous history of HCC-related treatments: radiotherapy, chemotherapy, transarterial chemoembolization (TACE), radiofrequency ablation (RFA), immunosuppressive therapy, liver resection or transplantation; (III) complete clinicoradiolgic data (i.e., the pathologic report and laboratory indexes); (IV) MRI with sufficient image quality scanned within 1 month before hepatectomy. Besides, the frequency of MVI stratified by tumor size is plotted in Figure 1B.
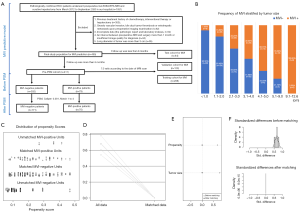
Laboratory examinations and histopathology
Preoperative laboratory indexes (Table 1) comprised serum alpha-fetoprotein (AFP), viral hepatitis type B (HBV), anti-hepatitis C virus, HBV-DNA loads, alanine aminotransferase, aspartate aminotransferase, total bilirubin, direct bilirubin, alkaline phosphatase, γ-glutamyl transpeptidase, albumin, prealbumin, and prothrombin time.
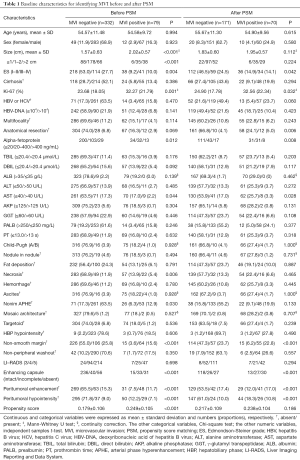
Full table
Histopathological characteristics included tumor size, tumor number, Edmondson-Steiner grade, MVI status, Ki-67 protein expression, liver fibrosis grade based on the Scheuer scoring system. MVI is defined as the cancerous thrombosis of portal vein, hepatic vein, or large capsular vessels lined with endothelium in the contiguous hepatic parenchyma (13-16). MVI grades are stratified as M0 (no MVI), M1 (invasion of micro-vessels up to five at peritumoral parenchyma within 1 cm from tumor surface) and M2 (MVI >5 sites or at >1 cm away from tumor surface) (13,16,22). These indexes were independently assessed by two experienced abdominal pathologists. In case of disagreement, a consensus was reached after discussion.
Meanwhile, the Barcelona Clinic Liver Cancer (BCLC) staging classification, the tumor-node-metastasis (TNM) system of the American Joint Committee on Cancer (8th Edition), the preoperative and postoperative Early Recurrence After Surgery for Liver tumor (ERASL-pre, ERASL-post) models (23) were enrolled into the prognostic study.
Gd-EOB-DTPA MRI
A 1.5T MRI scanner (Magnetom Aera, Siemens Healthcare, Erlangen, Germany) equipped with phased-array coils was performed on all eligible patients. Gd-EOB-DTPA (Primovist, Bayer Pharma, Berlin, Germany) was injected intravenously at a dosage of 0.025 mmol/kg at a rate of 1.5 mL/s, and immediately flushed by 20-mL saline. The conventional MRI sequences of upper abdomen were as below: free-breath diffusion-weighted imaging with b values of 0 and 500 s/mm2, axial T2-weighted imaging with fat suppression, in-phase and opposed-phase T1-weighted imaging (T1WI), pre- and post-contrast dynamic three-dimensional volumetric-interpolated breath-hold examination. When Gd-EOB-DTPA reached the ascending aorta (at 20–30 s after injection), the acquisition of arterial phase (AP) was automatically triggered. The subsequent portal venous phase (PVP), transitional phase (TP), and hepatobiliary phase (HBP) were collected at 60–70 s, 180 s and 20 min after injection, respectively. Detailed MRI parameters are shown in Table S1.
MRI hallmarks
Two radiologists with 8 and 15 years of abdominal MRI experience, who were blinded to MVI status, independently reviewed MRI morphologic hallmarks. Inconsistent diagnoses were discussed to make a final decision.
The major features of LI-RADS (version 2018) (24,25) were as follows: (I) tumor size, the longest axis diameter measured on HBP images; (II) enhancing capsule on PVP or TP images; (III) non-peripheral washout; (IV) non-rim arterial phase hyperenhancement (APHE). LI-RADS categories were calculated by these major features. The ancillary features of LI-RADS (24) were as below: (I) necrosis; (II) hemorrhage; (III) fat deposition; (IV) targetoid architecture; (V) nodule-in-nodule architecture; (VI) mosaic architecture; (VII) hypointensity of tumor on HBP images. Non-LI-RADS morphologic hallmarks comprised (I) non-smooth tumor margin, (II) peritumoral enhancement on AP images (26), (III) peritumoral hypointensity on HBP images (26), (VI) ascites. The definition of MRI hallmarks is shown in Supplementary methods.
Follow-up and data-grouping
Consistent follow-up with laboratory and imaging examinations was performed at intervals of 3 to 6 months after curative resection. Pathologic reports of recurrent/metastatic lesions were also included in the follow-up items, if applicable. The imaging follow-up was conducted by two radiologists, mainly based on the modified Response Evaluation Criteria in Solid Tumors (27) and the reliable diagnosis of HCC by “washin and washout” imaging pattern (1). Specifically, image-guided recurrent/metastatic lesion was determined by metabolic (i.e., maximum standardized uptake value beyond 2.5) or anatomic (e.g., altered lesion size, morphology, diffusivity, signal intensity, and contrast enhancement) pattern recognition of the de novo or original lesion. Notably, the abnormally elevated metabolism was regarded as an important auxiliary determinant of progression.
Patients were censored on September 26, 2020. The date of operation, recurrence, metastasis, death, and the last follow-up were recorded to calculate OS and recurrence-free survival (RFS). Among the 455 enrolled patients, 411 consecutive patients completed at least 6 months of follow-up, named as Pre-PSM cohort, and were used for MVI, PSM and prognosis analyses. In terms of MVI prediction, the 411 patients were divided into training and validation sets, according to the date of MRI examination at a ratio of 7:3. The remaining 44/455 population was classified as the test set to further verify the predictive performance of MVI model. Furthermore, the dataset of the eligible patients after PSM was denoted by post-PSM cohort.
Statistical analyses
Analyses were performed with IBM SPSS Statistics software (version 25.0; IBM Corporation, Armonk, NY, USA) or R (version 3.6.1, https://www.r-project.org) software. Continuous and categorical variables were expressed as mean ± standard deviation and numbers (proportions), respectively. Independent sample t-test, Mann Whitney U test, Chi-square test, Fisher’s exact test and logistic regression was applied to the univariate analysis, as appropriate. The intra-observer consistency of radiologic features was evaluated by McNemar’s and Kappa tests (κ statistics <0.40, poor; 0.40–0.59, fair; 0.60–0.80, good; and 0.80–1.00, excellent). P<0.05 was considered statistically significant.
Multivariate logistic regression (backward stepwise: ward) was used to identify the independent predictors of MVI. The discrimination of prediction model was quantified by the area under receiver operating characteristic curve (AUC) as well as Harrell’s C-index. The consistency between the predicted MVI model and the actual MVI state was evaluated by calibration curve analysis. With Kaplan-Meier analysis of two-sided log-rank test, survival curves were stratified by MVI status. The sample size was estimated by the reported median RFS of MVI and non-MVI cohorts (6). The detailed calculation is illustrated in Supplementary methods, and our sample sizes meets the statistical requirements. Multivariate Cox regression (forward stepwise: maximum like-hood ratio) determined the independent predictors of recurrence/mortality.
Multivariate logistic and Cox regressions were combined to identify confounding factors (28), and PSM was implemented to reduce confounders (28-30). Meanwhile, three R packages “PS MATCHING 3.03”, “SPSS Statistics R Essentials 22.0” and “R-2.15.3-win” were installed in SPSS for PSM analysis (29). The caliper and the optimal match ratio were set at 0.001 and 1:4, respectively. After PSM, the propensity score ensured that no significant differences were found in the baseline contexts of the matched cases, which was visualized in Figure 1C,B,C,D,E,F.
Results
455 eligible sHCC patients’ clinicoradiologic hallmarks for identifying MVI
Baseline characteristics of the training (n=288), validation (n=123) and test (n=44) cohorts for preoperative MVI prediction are shown in Table S2. The representative images of non-MVI and MVI cases are displayed in Figure 2. Multivariate logistic regression indicated that AFP >20 ng/mL, larger tumor size, incomplete or absent capsule enhancement, peritumoral enhancement and non-smooth margin were significantly susceptible to MVI (P<0.05), both in training and Pre-PSM cohorts. These five independent predictors were visualized as a nomogram (Figure 3A) that was further evaluated by calibration curves of training, validation and test cohorts (Figure 3B,C,D). The sensitivity, specificity, cut-off, AUC and C-index of the nomogram were as follows: 0.810, 0.848, 0.237, 0.884 (95% CI: 0.838–0.931; P<0.001; Figure 3E) and 0.874 (95% CI: 0.826–0.921) in the training cohort; 0.762, 0.873, 0.255, 0.845 (95% CI: 0.734–0.956; P<0.001; Figure 3F) and 0.828 (95% CI: 0.703–0.953) in the validation cohort; 1.000, 0.757, 0.182, 0.903 (95% CI: 0.813–0.994; P=0.001; Figure 3G) and 0.954 (95% CI: 0.897–1.010) in the test cohort. The formula of the predictive MVI nomogram were illustrated as below:
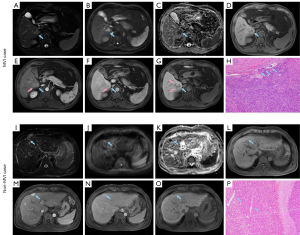
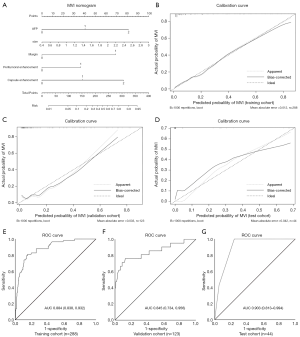
Meanwhile, the AUC and C-index yielded 0.875 (95% CI: 0.828–0.921; P<0.001) and 0.863 (95% CI: 0.815–0.911) in Pre-PSM cohort, and reached 0.824 (95% CI: 0.761–0.887; P<0.001) and 0.826 (95% CI: 0.764–0.887) in post-PSM cohort. Additionally, AFP >20 ng/mL, non-smooth margin, incomplete or absent capsule were robust independent risk factors for MVI after PSM.
411 sHCC patients’ clinicoradiologic hallmarks before PSM
Pathologically confirmed 79 (19.2%) MVI-positive and 332 (80.8%) MVI-negative patients in our hospital. Baseline characteristics of the two groups in Pre-PSM cohort are shown in Table 1. Owing to the conspicuous bias, the clinicoradiologic data for 11 of the 37 variables differed significantly (P<0.05), with an unmatched propensity score between MVI and non-MVI cases (0.249±0.105 vs. 0.179±0.106, P<0.001).
Confounders between MVI and prognosis
To investigate whether the existing confounders could lead to any bias, univariate logistic and Cox regressions were first conducted on the Pre-PSM dataset to filter out the variables related to MVI and recurrence/mortality (Table 2), respectively. Multivariate logistic regression showed that larger tumor size, AFP >20 ng/mL, non-smooth margin, peritumoral enhancement, incomplete or absent capsule enhancement were significantly positive associated with MVI (P<0.05, Table 3). Meanwhile, larger tumor size, MVI and prealbumin <250 mg/L markedly suffered from postoperative recurrence by the multivariate cox analysis (P<0.05). Moreover, the older, HBV-DNA loads >104, alkaline phosphatase >125 U/L, peritumoral enhancement, and peritumoral hypointensity were independent risk factors for mortality (P<0.05).
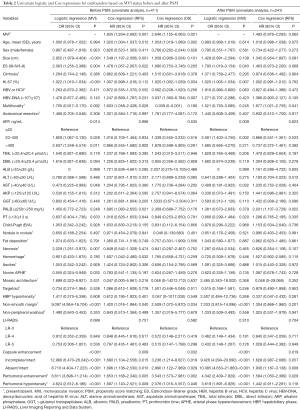
Full table
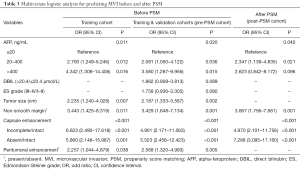
Full table
Therefore, tumor size was selected out to be the only confounder of MVI for predicting recurrence in our study population. Additionally, the frequency of MVI dramatically increased as tumor size growing (Figure 1B, P<0.001): ≤1 cm, 7.5% (8/107); 1.1–2 cm, 16.6% (38/229); 2.1–3 cm, 34.2% (39/114); 3.1–4 cm, 49.0% (25/51); 4.1–5 cm, 66.7% (12/18); 5.1–9 cm, 78.3% (18/23); 9.1–12.6 cm, 100% (8/8).
241 matched sHCC patients’ clinicoradiologic hallmarks after PSM
Though reducing the confounder—tumor size, 70 of 79 MVI-positive cases were matched with 171 of 332 MVI-negative subjects. The distribution of propensity scores in MVI-negative and MVI-positive groups before and after PSM is shown in Figure 1C. After PSM, the standard difference of each covariate decreased significantly (Figure 1D), the standard difference was concentrated around 0 (Figure 1E), and the standardized mean difference of tumor size and propensity score decreased to 0 (Figure 1F), all of which indicated an excellent matching effect. Consequently, no bias was found for 37 clinicoradiologic characteristics in the matched MVI and non-MVI groups (propensity score: 0.238±0.104 vs. 0.217±0.109, P=0.186; Table 1).
Prognostic value of MVI
Univariate Cox analysis demonstrated that MVI exerted an adverse impact on RFS of Pre-PSM (HR: 1.835, 95% CI: 1.264–2.662, P=0.001) and Post-PSM (HR: 1.493, 95% CI: 0.976–2.283, P=0.065) cohorts. Meanwhile, the Kaplan-Meier curve (Figure 4) signified that non-MVI patients benefited in a longer median RFS than that of MVI patients, whether before PSM (85.4 vs. 43.0 months, P=0.001) or after PSM (74.3 vs. 43.0 months, P=0.063). Baseline characteristics of recurrent prediction models before and after PSM are presented in Table S3.
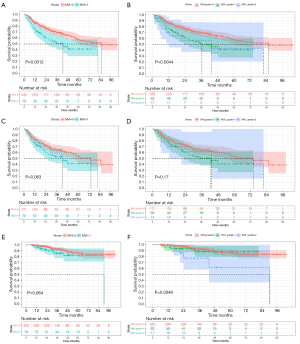
Before PSM, MVI (HR 1.555, 95% CI: 1.055–2.293, P=0.026), prealbumin <250 mg/L (HR 1.625, 95% CI: 1.037–2.548, P=0.034) and tumor size (HR 1.412, 95% CI: 1.078–1.849, P=0.012) were independently adverse to RFS. The formulas are as below, with AUC of 0.662 (95% CI: 0.604–0.719, P<0.001). Notably, the clinicoradiogic model outperformed the BCLC, TNM, ERASL-pre and ERASL-post models in predicting RFS (Table 4).
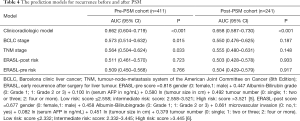
Full table
After PSM, prealbumin <250 mg/L (HR 1.980, 95% CI: 1.082–3.660, P=0.027), γ-glutamyl transpeptidase >60 U/L (HR 1.588, 95% CI: 1.036–2.434, P=0.034), incomplete or absent capsule enhancement (HR 1.804, 95% CI: 1.190–2.736, P=0.005) were independently induced to recurrence. Similarly, this clinicoradiogic algorithm (AUC 0.658, 95% CI: 0.587–0.730, P<0.001, Table 4) was also superior to the BCLC, TNM, ERASL-pre and ERASL-post models for RFS prediction. However, MVI did not independently impair RFS in sHCC ≤3 cm after balancing the confounder—tumor size.
In the multivariable Cox regression of OS, the older (HR 1.045, 95% CI: 1.010–1.081, P=0.012), alkaline phosphatase >125 U/L (HR 3.143, 95% CI: 1.144–8.636, P=0.026), HBV–DNA load >104 (HR 2.045, 95% CI: 1.044–4.009, P=0.037), peritumoral enhancement (HR 2.319, 95% CI: 1.126–4.778, P=0.022), and peritumoral hypointensity (HR 2.377, 95% CI: 1.126–5.018, P=0.023) were independently associated with mortality before PSM:
The median OS of MVI-positive and MVI-negative patients were 90.533 and >103.067 months (P=0.064), respectively. Despite MVI had a significant effect on mortality in univariate Cox regression (HR: 2.646, 95% CI: 1.155–6.063, P=0.021, Table 2), it failed to be an independent risk factor for mortality. Additionally, we further stratified MVI status into M0, M1, and M2, with median OS of >103.1, >81.7 and 90.5 months (P=0.005), respectively. However, MVI grade only significantly impaired OS in univariate Cox regression (P=0.011; M1, HR: 1.317, 95% CI: 0.539–3.220, P=0.546; M2, HR: 4.383, 95% CI: 1.671–11.493, P=0.003), it was also not independently prone to mortality. Accordingly, this MVI-related paper did not involve the Post-PSM analysis of overall survival. In addition, the comparison of the nomogram for predicting MVI between the published studies (6,14,15,31-36) and our research is summarized in Table 5.
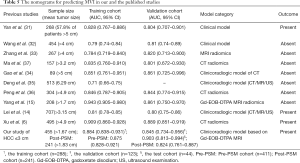
Full table
Discussion
Predictive and preventable strategies of MVI based on precision medicine will bring profound benefits (28). Compared with large HCC, sHCC within 3 cm has unique genetic changes (13,38), relatively homogeneous DNA stemline ploidy (39), benign biological behavior and favorable prognosis (10,12,13,39). Thus, this study aimed to investigate whether Gd-EOB-DTPA MRI is an efficient preoperative biomarker for MVI and whether MVI independently impairs prognosis in patients with sHCC ≤3 cm using a RCT-like method—PSM. We found that AFP >20 ng/mL, non-smooth margin, incomplete or absent capsule, peritumoral enhancement and larger tumor size were independent risk factors for histologic MVI. The frequency of MVI significantly elevated as tumor size growing (from 0 to 12.6 cm). Despite MVI independently impaired RFS before balancing the confounder—tumor size, it was ultimately identified as a potential but not an independent risk factor for recrudescence of sHCC ≤3 cm after PSM. Similarly, MVI was not independently detrimental to OS.
Elevated AFP level (14,15), non-smooth margin (6,7,15), incomplete/absent capsule enhancement (6,14), increased tumor size (14,40) and peritumoral enhancement (6,7,15) have been reported as independent risk factors for MVI, which also highly suitable for our patients with sHCC ≤3 cm. Furthermore, the first three predictors remained reliability and robustness in MVI prediction after PSM. Notably, peritumoral enhancement, peritumoral hypointensity, capsule and margin statuses imply peritumoral biological behaviour, reflecting an aggressive tendency to invade the tumor capsule and infiltrate into the peritumoral non-neoplastic parenchyma (41). Histologically, HCC’s capsule is composed of a fibrous-rich inner layer, and an outer layer containing portal venules (or sinusoids) and newly formed bile ducts (19). Thus, an intact capsule may serve as a border defenced against HCC’s dissemination and progression. Once cancerous cells break through the capsule, imaging signs of the incomplete/absent capsule enhancement and infiltrative border (non-smooth margin) will appear (42). These may interpret the excellent performance of capsule status in predicting MVI and even recurrence after PSM.
Pathologically, peritumoral parenchyma is the representative of tumor heterogeneity, rich in highly invasive cells (13), and is the first and most common susceptible region of MVI (26,41). Peritumoral enhancement potentiates the formation of MVI, which may stem from (I) compensatory arterial hyperperfusion, which often occurs in areas of decreased portal flow (e.g., minute portal venule occlusion caused by tumor thrombi) (15,42); (II) high expression of placental growth factor and vascular endothelial growth factor receptor (VEGFR) in peritumoral zones (43). Moreover, the tumor-host interaction between VEGFR and MVI has been reported to promote the progression of renal cell carcinoma (44). Hence, these may explain that peritumoral enhancement independently induce MVI and mortality in sHCC ≤3 cm. Analogically, peritumoral hypointensity independently shortened OS and is a potential risk indicator for MVI in our study. This imaging trait reflects the peritumoral reduction of Gd-EOB-DTPA uptake on HBP images, that is, a decreased expression of organic anionic transporting polypeptide (45). It may be induced by impaired hepatocyte function (45,46) or Kupffer cells injury in tumorous arterioportal shunts (46) because of portal branches obstruction by cancer embolus (i.e., MVI). Controversially, this radiologic feature is paucity of robustness for identifying MVI, RFS or OS in different studies (6,7,15,45,47-49). Thus, this equivocal finding needs further study.
According to the LI-RADS category, most patients are classified into the high-risk HCC of LR-5. Among the major features of LI-RADS, only tumor size and enhancing capsule were closely correlated with MVI and RFS. Similarly, in the majority of LI-RADS auxiliary features after univariate analysis, only non-rim APHE and necrosis were remarkably vulnerable to MVI, and only haemorrhage was markedly susceptible to recurrence. Generally, LI-RADS category and most LI-RADS features cannot independently predict MVI and prognosis. Our findings are basically agreement with the relationship between LI-RADS category/features and MVI in combined hepatocellular-cholangiocarcinoma (50), and postoperative recurrence in early HCC (21) or in LR-5 HCC (20,47). Previous study indicated (20) that mosaic architecture independently identified MVI and fat deposition independently predicted recurrence in LR-5 HCC after hepatectomy. The controversial results may originate from different grades of LI-RADS and thresholds of tumor size. Therefore, more homogeneous studies are needed to further explore these hallmarks for expanding the applicability of LI-RADS.
Compared with the published nomograms of MVI (6,14,15,31-36), only our study focused on sHCC ≤3 cm and the confounding factors of MVI for predicting prognosis. More precisely, two of ten published studies involved the prognostic analysis with the findings given below: the CT radiomics-related index of Xu et al. (6) and the clinicoradiologic indicators (HBV-DNA load, tumor size and number) of Lei et al. (14) were independent risk factors of MVI and recurrence/mortality. However, the two studies ignored the above-mentioned confounders between MVI and outcomes, and thus failed to recognize the actual role of MVI in prognosis to a certain extent. Besides, the study of Yan et al. (31) paid more attention to HCC lesions with tumor size >5 cm (155/268) and lymph node metastasis (41/268), and ignored the importance of imaging hallmarks in predicting MVI. Above all, while the Kaplan-Meier curves of Yan et al. (31) signified significance differences between MVI and non-MVI patients in RFS and OS, this study did not involve the multivariable COX analysis. Hence, this published paper (31) cannot interpret whether MVI independently predicts prognosis, and whether there are confounders between MVI and outcomes.
Controversially, MVI exerts an ambiguous effect on sHCC’s outcome. A non-PSM study of Du et al. (9) presented that MVI independently shortened OS and progression-free survival, and increasing tumor size facilitated higher incidences of MVI in solitary sHCC ≤3 cm. The results were highly consistent with our findings before PSM. However, the conclusion of Du et al. (9) neglected the bias of respective study and the potential confounder (e.g., tumor size) of MVI in prognostic analysis. Intriguingly, we identified that tumor size was the only confounder for MVI to predict RFS in our sHCC cases, confirming the hypothesis mentioned in the introduction. Furthermore, tumor size was significantly positive relevant to MVI incidence from 0 to 12.6 cm. This may interpret that unlike previous studies of larger tumor size (6,51), histologic MVI status or even MVI grades cannot independently induce to postoperative recurrence and mortality in our study. Contrary to our findings, the PSM study of Wang et al. (22) demonstrated that MVI engendered a negative influence on the RFS of solitary sHCC ≤2 cm. Nevertheless, this study only balanced the bias variables (P<0.1) between MVI and non-MVI groups, but neglected to reduce the confounder (alanine transaminase) which independently associated with both MVI and RFS. Meanwhile, this study displayed a higher percentage of MVI (pre-PSM cohort: 33.1%; post-PSM cohort: 50%) than that of our population with maximal size ≤2 cm (pre-PSM cohort: 13.7%; post-PSM cohort: 13.4%). In brief, our study had a lower but more robust incidence of MVI before and after PSM, and balanced the actual confounder between MVI and RFS, leading to an opposite conclusion of Wang et al. (22). Furthermore, Shindoh et al. (52) presented that the presence of MVI (26.5%) did not affect the long-term outcome of sHCC ≤2 cm, which was analogous with our findings. The prognostic discrepancy of MVI among sHCC studies may attribute to the mixture of different tumor sizes or stages, MVI incidences, underlying liver diseases, geographic heterogeneity, surgical procedure (e.g., wide or narrow resection margin, anatomical or non-anatomical resection, curative resection or liver transplantation) or postoperative treatment (e.g., preventive TACE, immunosuppression therapy). Consequently, the impact of MVI on sHCC’s prognosis remains a challenge, and more homogeneous studies need to be further verified.
At present, the radical treatment ratio of patients with sHCC ≤3 cm is markedly increased, posing an urgent and practical issue in hepatic surgery (12). Hence, we speculate that this random controlled trial (RCT)-like PSM study allows clinicians more time to optimize therapeutic schedule (I) with a reasonable surgical margin, because MVI status is independently irrespective of recurrence/mortality in sHCC ≤3 cm; (II) with a reasonable residual liver volume, for reducing the frequency of hepatic dysfunction/infection and improving the safety of liver resection (53). Furthermore, solitary HCC ≤3 cm can be ablated almost completely after RFA (54). Nowadays, the disparity of HCC patients’ survival between curative RFA and surgery is narrowing, especially for sHCC within 3 cm (55). Hence, this PSM study also provides additional evidence for clinicians to apply RFA for sHCC ≤3 cm, regardless of the histologic MVI status.
Several limitations should be noted. Firstly, this is a retrospective single-center study. Thus, we implemented PSM analysis to simulate a random controlled trial for minimizing selection bias. Meanwhile, the robustness of our results needs to be further verified by external and prospective dataset. Secondly, while BCLC, TNM, ERASL-pre, ERASL-post risk and the clinicoradiogic models have been used to predict prognosis, the AUCs of these algorithms were unsatisfactory. The results may stem from (I) the paucity of immunohistochemical biomarkers and postoperative characteristics; (II) the exclusion of well-established key risk factors for dismal outcomes (e.g., tumor size >3 cm, tumor thrombus in gross vessels) in our population; (III) the absence of radiomics that can high-throughput extract quantitative imaging signatures to improve diagnostic or prognostic accuracy (16,56). Hence, the above three points can be implemented to further investigate the relationship between MVI and prognosis of sHCC.
Conclusions
Mainly based on the peritumoral hallmarks rather than LI-RADS features of Gd-EOB-DTPA MRI, our preoperative nomogram can excellently distinguish the histopathological MVI status. However, MVI is a potential but not an independent risk factor for prognosis in patients with sHCC ≤3 cm.
Acknowledgments
Funding: The study was supported by National Natural Science Foundation of China (No. 91859107), Shanghai Science and Technology Committee (No. 18DZ1930102 & No. 19411965500), Zhongshan Hospital, Fudan University (No. 2018ZSLC22 & No. 2020ZSLC61), Shanghai Municipal Key Clinical Specialty (No. W2019-018), Clinical Research Plan of SHDC (No. SHDC2020CR1029B).
Footnote
Reporting Checklist: The authors have completed the TRIPOD reporting checklist. Available at http://dx.doi.org/10.21037/atm-20-7952
Data Sharing Statement: Available at http://dx.doi.org/10.21037/atm-20-7952
Conflicts of Interest: All authors have completed the ICMJE uniform disclosure form (available at http://dx.doi.org/10.21037/atm-20-7952). The authors have no conflicts of interest to declare.
Ethical Statement: The authors are accountable for all aspects of the work in ensuring that questions related to the accuracy or integrity of any part of the work are appropriately investigated and resolved. This study was conducted in accordance with the Declaration of Helsinki (as revised in 2013). The study was approved and informed consent was waived by the Ethical Review Committee of Zhongshan Hospital, Fudan University, Shanghai, China (approval No. B2018-236).
Open Access Statement: This is an Open Access article distributed in accordance with the Creative Commons Attribution-NonCommercial-NoDerivs 4.0 International License (CC BY-NC-ND 4.0), which permits the non-commercial replication and distribution of the article with the strict proviso that no changes or edits are made and the original work is properly cited (including links to both the formal publication through the relevant DOI and the license). See: https://creativecommons.org/licenses/by-nc-nd/4.0/.
References
- Forner A, Reig M, Bruix J. Hepatocellular carcinoma. Lancet 2018;391:1301-14. [Crossref] [PubMed]
- De Angelis R, Sant M, Coleman MP, et al. Cancer survival in Europe 1999-2007 by country and age: results of EUROCARE--5-a population-based study. Lancet Oncol 2014;15:23-34. [Crossref] [PubMed]
- Fujiwara N, Friedman SL, Goossens N, et al. Risk factors and prevention of hepatocellular carcinoma in the era of precision medicine. J Hepatol 2018;68:526-49. [Crossref] [PubMed]
- Hwang S, Lee YJ, Kim KH, et al. The Impact of Tumor Size on Long-Term Survival Outcomes After Resection of Solitary Hepatocellular Carcinoma: Single-Institution Experience with 2558 Patients. J Gastrointest Surg 2015;19:1281-90. [Crossref] [PubMed]
- Vogel A, Cervantes A, Chau I, et al. Hepatocellular carcinoma: ESMO Clinical Practice Guidelines for diagnosis, treatment and follow-up†. Ann Oncol 2018;29:238-55. [Crossref] [PubMed]
- Xu X, Zhang HL, Liu QP, et al. Radiomic analysis of contrast-enhanced CT predicts microvascular invasion and outcome in hepatocellular carcinoma. J Hepatol 2019;70:1133-44. [Crossref] [PubMed]
- Lee S, Kim SH, Lee JE, et al. Preoperative gadoxetic acid-enhanced MRI for predicting microvascular invasion in patients with single hepatocellular carcinoma. J Hepatol 2017;67:526-34. [Crossref] [PubMed]
- Takuma Y, Shota I, Miyatake H, et al. Nomograms to Predict the Disease-free Survival and Overall Survival after Radiofrequency Ablation for Hepatocellular Carcinoma. Intern Med 2018;57:457-68. [Crossref] [PubMed]
- Du M, Chen L, Zhao J, et al. Microvascular invasion (MVI) is a poorer prognostic predictor for small hepatocellular carcinoma. BMC Cancer 2014;14:38. [Crossref] [PubMed]
- Lu XY, Xi T, Lau WY, et al. Pathobiological features of small hepatocellular carcinoma: correlation between tumor size and biological behavior. J Cancer Res Clin Oncol 2011;137:567-75. [Crossref] [PubMed]
- Lim C, Mise Y, Sakamoto Y, et al. Above 5 cm, size does not matter anymore in patients with hepatocellular carcinoma. World J Surg 2014;38:2910-8. [Crossref] [PubMed]
- Cong WM, Wu MC. Small hepatocellular carcinoma: current and future approaches. Hepatol Int 2013;7:805-12. [Crossref] [PubMed]
- Cong WM, Bu H, Chen J, et al. Practice guidelines for the pathological diagnosis of primary liver cancer: 2015 update. World J Gastroenterol 2016;22:9279-87. [Crossref] [PubMed]
- Lei Z, Li J, Wu D, et al. Nomogram for Preoperative Estimation of Microvascular Invasion Risk in Hepatitis B Virus-Related Hepatocellular Carcinoma Within the Milan Criteria. JAMA Surg 2016;151:356-63. [Crossref] [PubMed]
- Yang L, Gu D, Wei J, et al. A Radiomics Nomogram for Preoperative Prediction of Microvascular Invasion in Hepatocellular Carcinoma. Liver Cancer 2019;8:373-86. [Crossref] [PubMed]
- Chong HH, Yang L, Sheng RF, et al. Multi-scale and multi-parametric radiomics of gadoxetate disodium–enhanced MRI predicts microvascular invasion and outcome in patients with solitary hepatocellular carcinoma ≤ 5 cm. Eur Radiol 2021; [Epub ahead of print]. [Crossref] [PubMed]
- Renzulli M, Biselli M, Brocchi S, et al. New hallmark of hepatocellular carcinoma, early hepatocellular carcinoma and high-grade dysplastic nodules on Gd-EOB-DTPA MRI in patients with cirrhosis: a new diagnostic algorithm. Gut 2018;67:1674-82. [Crossref] [PubMed]
- Kitao A, Matsui O, Yoneda N, et al. Gadoxetic acid-enhanced MR imaging for hepatocellular carcinoma: molecular and genetic background. Eur Radiol 2020;30:3438-47. [Crossref] [PubMed]
- Cho ES, Choi JY. MRI features of hepatocellular carcinoma related to biologic behavior. Korean J Radiol 2015;16:449-64. [Crossref] [PubMed]
- Chen J, Zhou J, Kuang S, et al. Liver Imaging Reporting and Data System Category 5: MRI Predictors of Microvascular Invasion and Recurrence After Hepatectomy for Hepatocellular Carcinoma. AJR Am J Roentgenol 2019;213:821-30. [Crossref] [PubMed]
- Zhang L, Kuang S, Chen J, et al. The Role of Preoperative Dynamic Contrast-enhanced 3.0-T MR Imaging in Predicting Early Recurrence in Patients With Early-Stage Hepatocellular Carcinomas After Curative Resection. Front Oncol 2019;9:1336. [Crossref] [PubMed]
- Wang H, Wu MC, Cong WM. Microvascular invasion predicts a poor prognosis of solitary hepatocellular carcinoma up to 2 cm based on propensity score matching analysis. Hepatol Res 2019;49:344-54. [Crossref] [PubMed]
- Chan AWH, Zhong J, Berhane S, et al. Development of pre and post-operative models to predict early recurrence of hepatocellular carcinoma after surgical resection. J Hepatol 2018;69:1284-93. [Crossref] [PubMed]
- American College of Radiology (2018). CT/MRI LI-RADS® v2018 core. Available online: https://www.acr.org/Clinical-Resources/Reporting-and-Data-Systems/LI-RADS/CT-MRI-LI-RADS-v2018
- Chernyak V, Fowler KJ, Kamaya A, et al. Liver Imaging Reporting and Data System (LI-RADS) Version 2018: Imaging of Hepatocellular Carcinoma in At-Risk Patients. Radiology 2018;289:816-30. [Crossref] [PubMed]
- Hu HT, Shen SL, Wang Z, et al. Peritumoral tissue on preoperative imaging reveals microvascular invasion in hepatocellular carcinoma: a systematic review and meta-analysis. Abdom Radiol (NY) 2018;43:3324-30. [Crossref] [PubMed]
- Eisenhauer EA, Therasse P, Bogaerts J, et al. New response evaluation criteria in solid tumours: revised RECIST guideline (version 1.1). Eur J Cancer 2009;45:228-47. [Crossref] [PubMed]
- Tang Z, Liu WR, Zhou PY, et al. Prognostic Value and Predication Model of Microvascular Invasion in Patients with Intrahepatic Cholangiocarcinoma. J Cancer 2019;10:5575-84. [Crossref] [PubMed]
- Zhou PY, Tang Z, Liu WR, et al. Perioperative blood transfusion does not affect recurrence-free and overall survivals after curative resection for intrahepatic cholangiocarcinoma: a propensity score matching analysis. BMC Cancer 2017;17:762. [Crossref] [PubMed]
- Lim C, Bhangui P, Salloum C, et al. Impact of time to surgery in the outcome of patients with liver resection for BCLC 0-A stage hepatocellular carcinoma. J Hepatol 2017; [PubMed]
- Yan Y, Zhou Q, Zhang M, et al. Integrated Nomograms for Preoperative Prediction of Microvascular Invasion and Lymph Node Metastasis Risk in Hepatocellular Carcinoma Patients. Ann Surg Oncol 2020;27:1361-71. [Crossref] [PubMed]
- Wang L, Jin YX, Ji YZ, et al. Development and validation of a prediction model for microvascular invasion in hepatocellular carcinoma. World J Gastroenterol 2020;26:1647-59. [Crossref] [PubMed]
- Zhang R, Xu L, Wen X, et al. A nomogram based on bi-regional radiomics features from multimodal magnetic resonance imaging for preoperative prediction of microvascular invasion in hepatocellular carcinoma. Quant Imaging Med Surg 2019;9:1503-15. [Crossref] [PubMed]
- Gao SX, Liao R, Wang HQ, et al. A Nomogram Predicting Microvascular Invasion Risk in BCLC 0/A Hepatocellular Carcinoma after Curative Resection. Biomed Res Int 2019;2019:9264137 [Crossref] [PubMed]
- Deng G, Yao L, Zeng F, et al. Nomogram For Preoperative Prediction Of Microvascular Invasion Risk In Hepatocellular Carcinoma. Cancer Manag Res 2019;11:9037-45. [Crossref] [PubMed]
- Peng J, Zhang J, Zhang Q, et al. A radiomics nomogram for preoperative prediction of microvascular invasion risk in hepatitis B virus-related hepatocellular carcinoma. Diagn Interv Radiol 2018;24:121-7. [Crossref] [PubMed]
- Ma X, Wei J, Gu D, et al. Preoperative radiomics nomogram for microvascular invasion prediction in hepatocellular carcinoma using contrast-enhanced CT. Eur Radiol 2019;29:3595-605. [Crossref] [PubMed]
- Moribe T, Iizuka N, Miura T, et al. Methylation of multiple genes as molecular markers for diagnosis of a small, well-differentiated hepatocellular carcinoma. Int J Cancer 2009;125:388-97. [Crossref] [PubMed]
- Cong WM, Wu MC. The biopathologic characteristics of DNA content of hepatocellular carcinomas. Cancer 1990;66:498-501. [Crossref] [PubMed]
- Banerjee S, Wang DS, Kim HJ, et al. A computed tomography radiogenomic biomarker predicts microvascular invasion and clinical outcomes in hepatocellular carcinoma. Hepatology 2015;62:792-800. [Crossref] [PubMed]
- Hu H, Zheng Q, Huang Y, et al. A non-smooth tumor margin on preoperative imaging assesses microvascular invasion of hepatocellular carcinoma: A systematic review and meta-analysis. Sci Rep 2017;7:15375. [Crossref] [PubMed]
- Wei Y, Huang Z, Tang H, et al. IVIM improves preoperative assessment of microvascular invasion in HCC. Eur Radiol 2019;29:5403-14. [Crossref] [PubMed]
- Kusano H, Han J, Bulthuis M, et al. Microvascular invasion in hepatocellular carcinoma is defined by tumor characteristics and aberrant angiogenesis in peritumoral tissue. Hepatology 2013;58:1214A.
- Yildiz E, Gokce G, Kilicarslan H, et al. Prognostic value of the expression of Ki-67, CD44 and vascular endothelial growth factor, and microvessel invasion, in renal cell carcinoma. BJU Int 2004;93:1087-93. [Crossref] [PubMed]
- Shin SK, Kim YS, Shim YS, et al. Peritumoral decreased uptake area of gadoxetic acid enhanced magnetic resonance imaging and tumor recurrence after surgical resection in hepatocellular carcinoma: A STROBE-compliant article. Medicine (Baltimore) 2017;96:e7761 [Crossref] [PubMed]
- Nishie A, Asayama Y, Ishigami K, et al. Clinicopathological significance of the peritumoral decreased uptake area of gadolinium ethoxybenzyl diethylenetriamine pentaacetic acid in hepatocellular carcinoma. J Gastroenterol Hepatol 2014;29:561-7. [Crossref] [PubMed]
- Wei H, Jiang H, Zheng T, et al. LI-RADS category 5 hepatocellular carcinoma: preoperative gadoxetic acid-enhanced MRI for early recurrence risk stratification after curative resection. Eur Radiol 2021;31:2289-302. [Crossref] [PubMed]
- Lee S, Kim KW, Jeong WK, et al. Gadoxetic acid-enhanced MRI as a predictor of recurrence of HCC after liver transplantation. Eur Radiol 2020;30:987-95. [Crossref] [PubMed]
- Bae JS, Kim JH, Lee DH, et al. Hepatobiliary phase of gadoxetic acid-enhanced MRI in patients with HCC: prognostic features before resection, ablation, or TACE. Eur Radiol 2020; [Epub ahead of print]. [Crossref] [PubMed]
- Wang X, Wang W, Ma X, et al. Combined hepatocellular-cholangiocarcinoma: which preoperative clinical data and conventional MRI characteristics have value for the prediction of microvascular invasion and clinical significance? Eur Radiol 2020;30:5337-47. [Crossref] [PubMed]
- Feng LH, Dong H, Lau WY, et al. Novel microvascular invasion-based prognostic nomograms to predict survival outcomes in patients after R0 resection for hepatocellular carcinoma. J Cancer Res Clin Oncol 2017;143:293-303. [Crossref] [PubMed]
- Shindoh J, Andreou A, Aloia TA, et al. Microvascular invasion does not predict long-term survival in hepatocellular carcinoma up to 2 cm: reappraisal of the staging system for solitary tumors. Ann Surg Oncol 2013;20:1223-9. [Crossref] [PubMed]
- Schindl MJ, Redhead DN, Fearon KC, et al. The value of residual liver volume as a predictor of hepatic dysfunction and infection after major liver resection. Gut 2005;54:289-96. [Crossref] [PubMed]
- Gao J, Wang SH, Ding XM, et al. Radiofrequency ablation for single hepatocellular carcinoma 3 cm or less as first-line treatment. World J Gastroenterol 2015;21:5287-94. [Crossref] [PubMed]
- Tiong L, Maddern GJ. Systematic review and meta-analysis of survival and disease recurrence after radiofrequency ablation for hepatocellular carcinoma. Br J Surg 2011;98:1210-24. [Crossref] [PubMed]
- Lambin P, Leijenaar RTH, Deist TM, et al. Radiomics: the bridge between medical imaging and personalized medicine. Nat Rev Clin Oncol 2017;14:749-62. [Crossref] [PubMed]