A model-based validation study of postoperative complications with considerations on operative timing
Introduction
Sleep deprivation (SD) is prevalent in the general population and may affect human attention and working memory (1). During prolonged wakefulness, the homeostatic drive for sleep competes with an effort to remain awake, resulting in impaired cognitive functions (2), errors, and accidents, especially during the circadian night (3,4). Circadian variation in performance is most evident when SD is present and chronic insufficient sleep (fewer than 5.6 hours of sleep per day) could negatively impact neurobehavioural performance, self-assessment, and alertness, even without extended wakefulness (5).
In medical circumstances, acute and/or chronic SD were found to affect the performance, learning, and personal well-being of medical trainees (6-8). A nationwide survey of 2,737 American residents in different specialties showed that extended working hours raised the risk of self-reported medical errors, patient fatalities, and attention failures (9). Deterioration of fine motor skills with SD was also shown in surgical residences (10,11), while anesthesia residents demonstrated sleepiness like narcolepsies even with no call duty over the preceding 48 hours (12). Since surgery is a highly technical procedure and is potentially vulnerable to fatigue and circadian rhythms, it is of great importance to investigate the relationship between operative start time as well as the duration and outcomes of surgery, for the purpose of improving healthcare.
In this study, we took postoperative complications from hospital stay as the outcome of interest, as previous research on general and vascular surgical procedures indicated that morbidity, instead of mortality could be strongly associated with the start time of surgery after appropriate adjustment (13). However, the results of possible associations between the two were found to be inconsistent and dependent on surgical disciplines. For example, operative timing in emergency general surgery was associated with increased postoperative complications (14) and the operative start time of cardiac surgery affected intraoperative transfusion rates (15). However, postoperative complications and overall survival rates did not vary with start time in the performance of radical gastrectomy (16), hip fracture fixation (17), renal transplantation (18), and liver resection (19). The outcome of minimal invasive endometrial cancer surgery and laparoscopic sacrocolpopexy (20) also had no association with operative start time (21) and although afternoon surgeries were found to be associated with increased levels of cortisol and inflammatory cytokines [interleukin (IL)-6, IL-8] (22), no conclusion of correlation between operation time and postoperative infectious complications (23).
This inconsistency in the results of studies investigating the association between operative timing and postoperative complications led us to consider the following alternative but closely related question: How does operative start time and duration rank in importance with other factors signifying a case of complication? To answer this question, we used an interpretable machine learning approach (24) by training various interpretable models and performing cross-validation on the training data, which in this case were retrospective surgical records collected, and chose the best-performing model for the classification task. We checked whether different time-domain features were important to classifying a case of complication from this model via the feature importance scores. In our study, we provide details of the dataset for this study and the pipeline of modeling and obtaining feature importance. We also discussed the factors that might lead to our observations and the implications of the results. We present the following article in accordance with the TRIPOD reporting checklist (available at http://dx.doi.org/10.21037/atm-21-669).
Methods
Study design
This single-center, retrospective validation study was approved by the Review Board of the Ethics Committee of Zhongshan Hospital affiliated with Fudan University. All procedures performed in this study involving human participants were in accordance with the Declaration of Helsinki (as revised in 2013). Individual consent for this retrospective analysis was waived. Zhongshan is a 2,005-bed major tertiary teaching hospital serving over 100,000 inpatients and 4,000,000 outpatients as well as emergencies annually. The records of 167,001 patients were collected from the hospital database, which involved all surgical procedures performed between January 1, 2018, and November 2, 2020. The following attributes were extracted: date and time the surgery was commenced and completed; duration of surgery; length of stay; surgical discipline; patient age and gender; admission and discharge consultation summaries; preoperative comorbidity (if any); and postoperative complications (if any). We excluded surgeries/patients under specific conditions, such as interventional procedures without anesthesia or sedation, anesthesiology surgeries, and transplants, resulting in 107,481 records being obtained for the study. Details of exclusion are shown in Figure 1.
We explored the associations of attributes (or features) with postoperative complications to achieve a better understanding of what features had the strongest correlation with the presence of complications. We also observed the hourly rate of complications trending with operative start times which we reason could result from multiple factors. The machine learning models used for generating cross-validation scores for the classification task included Logistic Regression (LR), Naive Bayes, CART, Random Forest (RF) (25), GBDT (26), AdaBoost (27), and XGBoost (28), LightGBM (LGB) (29), and CatBoost (30), which extracted information hidden in the surgical record data and detected those features having the strongest correlation with the binary target.
Statistical analysis
Prior to modeling, categorical features were one-hot encoded and all feature values were standardized. This resulted in 70% of the (shuffled) records used to train the models, leaving 30% as the validation set for model evaluation. To address the issue of class imbalance, we used the SMOTE oversampling algorithm (31) which generates synthetic data of the minority class to balance the classes and Recursive Feature Elimination (RFE) feature selection for extracting relevant features. Parameter tuning was performed via grid search 5-fold cross-validation for optimizing the F1 score [2 * precision * recall /(precision + recall)].
For model evaluation, accuracy, precision, recall, and the F1 score were calculated. We generated a Receiver Operating Characteristic (ROC) curve for each model and calculated the area under the curve (AUC). The F1 score and AUC were used as the main performance metrics for model comparison.
We also performed a time series analysis of the monthly rate towards complications, where the series was decomposed into additive components of trend, (periodic) seasonality, and residual. The trend component indicates a general tendency of complications that occurred during the past 3 years, which could be used for forecast and further planning.
Results
Table 1 summarizes the basic statistics of several attributes of the 107,481 surgical records for this study, among which 7,187 (6.69%) postoperative complications were detected. Table 2 describes complications categorized by the surgical discipline and grouped start time of the surgery {morning: [7 am, 12 pm); afternoon: [12 pm, 5 pm); evening: [5 pm, 7 am)}. The results show that the percentage of complications is highest in cardiac surgeries (26.32%), followed by neurological (10.73%) and plastic surgeries (7.82%). The percentage of complications is highest in surgeries which commence in the morning (7.73%) while lower in the afternoon group (6.18%) and lowest in the evening group (4.44%).
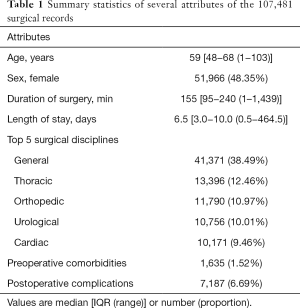
Full table
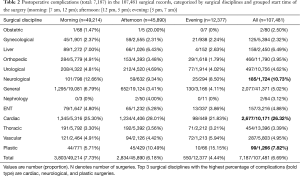
Full table
Figure 2 shows the hourly rate of complications against the histogram of surgeries, revealing that surgeries starting between 8–9 am lead to the highest percentage of complications (1,431/10,405, 13.75%), and that a linear trend drawn on the hourly rate of complications leans slightly upwards as the day progresses. Surgeries that start after 9 pm were observed to result in local peaks in complication rates, including 10–11 pm (23/320, 7.19%), 1–2 am (5/50, 10%), 4–5 am (4/12, 33.33%), and 6–7 am (10/72, 13.89%).
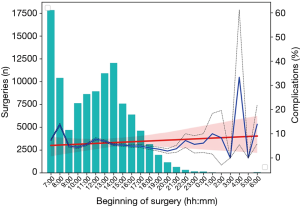
We performed RFE feature selection and then applied different machine learning models with cross validation, and the resulting performance metrics (accuracy, precision, recall, F1 score, and AUC) are listed in Table 3. It is clearly seen that XGBoost (F1: 0.95, AUC: 0.98) achieves the best evaluation performance among these models in all categories of performance metrics. Figure 3 compares the ROC curves of these models and shows XGBoost has the largest AUC and best optimal cutoff point. We calculated the relative feature importance for the XGBoost model and Figure 4 displays the most important eight features with duration of surgery, patient age, and cardiac surgical discipline as the three most important features for classifying a case of complication. Surgical disciplines such as plastic, liver, and general are also relatively important for classification, while patient sex—female [0] or male [1], ranks after these surgical disciplines in feature importance, as there is a higher proportion of male patient complication cases (4,520/55,515, 8.14%) than female cases (2,667/51,966, 5.13%) in the relatively balanced patient samples (male/female sample size ratio: 1.0682). The manually constructed feature ’Night’ (1: start time of surgery between 9 pm–7 am; 0 otherwise) also appears to be important, indicating it could be useful in classifying a case of complication.
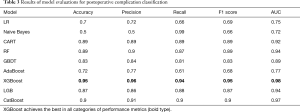
Full table
The monthly variation of complication rates is shown in Figure 5. For the past 3 years, the month of February was associated with the highest percentage of complications (405/4,416, 9.17%) while the month of July with the lowest (664/11,371, 5.84%), with a general trend decreasing during the year. The traditional Spring Festival is the biggest family holiday period in China normally beginning in February, which could explain why the number of surgeries is the lowest in that month while the percentage of complications tends to be the highest as only patients with severe conditions might undergo surgery during that period. Figure 6 shows the monthly variation of complication rates over the 3-year span. A general decreasing trend in the percentage of complications can be seen across each year (generally between 7.5% and 10.0% for 2018; between 5.0% and 7.5% for 2019; and approximately 5.0% for 2020). An additive decomposition of the monthly complication rates into trend, seasonal, and residual components is shown in Figure 7 and shows a decreasing trend over the past 3 years, as well as a roughly 12-month seasonal periodicity with highest and lowest peaks occurring in the months of February and July, respectively.
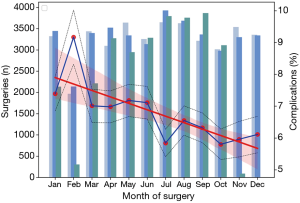
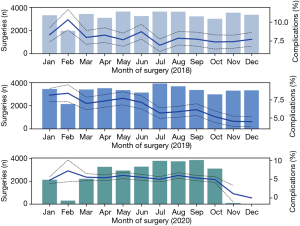
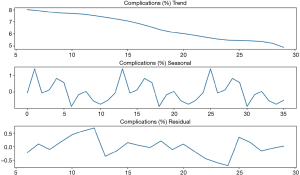
Discussion
We performed cross validation on the collected records of patients undergoing surgery at the Zhongshan Hospital between January 1, 2018 and November 2, 2020. The resulting best-performing model was XGboost, for which the feature importance scale indicated that duration of surgery most strongly signified postoperative complications. In addition, operative timing features showed less correlation with complications, with only one feature of operative start time, i.e., surgeries that started during the night (between 9 pm and 7 am), ranking 10th in feature importance. We observed that the rate of complications had some peaks during the night (see Figure 2), although there was insufficient evidence that surgeries started during that time period were correlated with a more frequent occurrence of complications. Some of these surgeries might be emergencies and with limited samples, there is not enough evidence supporting a relatively worse performance during the night in terms of the frequency of complications, and more data needs to be collected for analyzing surgeries commencing after midnight that led to postoperative complications. On the other hand, patient age and sex, and specific surgical disciplines, were more strongly correlated with classifying a case of complication. More data needs to be collected to strengthen the support of these observed characteristics, especially regarding operative timing during the night.
According to previous research, several factors might lead to a higher incidence of postoperative complications after night surgeries. The homeostatic drive for sleep competes with efforts to remain awake after a long wakefulness, resulting in worse performance in professional activities including complicated surgical procedures (32-34), but not simple ones (35). SD and circadian hormonal rhythm disturbance could also impair patient recovery from surgery (36-39). The influence of circadian rhythms on both medical care providers and patients might simultaneously contribute to a higher rate of complications after surgeries commencing late in the night.
Long-lasting work leads to fatigue which is associated with decline in technical skills (11,40), slower reaction, and inappropriate decision making (41,42). Studies have shown that the number of hours already worked has a significant impact on increasing the risk of certain adverse outcomes during unscheduled deliveries (43). In the present study we found that surgeries starting during the night (between 9 pm and 7 am) were more likely to be associated with complications, although the increase was only small. In our hospital, most midnight surgeries are emergencies which are performed by chief residents during 24-hour shifts. Fatigue is a prominent issue for these practitioners and may contribute to this observation.
We also observed a higher rate of complications for surgeries starting between 8–9 am, which could be due to the hospital arranging a large portion of major and prolonged surgeries during that time period. Figure 8 shows the box plot of duration of surgery in terms of its start time. Surgeries commencing between 8–9 am have the largest medium duration is 255.0 (IQR, 135.0–345.0) min during the day, and variation of the mediums for duration of surgery closely match that of complication rates, which is another way to appreciate the strong correlation between these two variables.
In the time series analysis, the monthly rate of complications demonstrated periodic seasonality with a general decreasing trend during the year as well as across the 3-year span. The relatively high rate of complications in the month of February could be explained by the timing of the traditional Spring Festival which is the most important holiday of the year in China. During that long vacation, elective surgeries are rare, and most surgeries are emergencies. It was curious that the month of July saw the least frequent occurrence of complications. If a seasonal rhythm exists, this occurrence pattern could be partly explained by previous research on mortality which showed a peak occurrence of postoperative complications in winter (44). The general decreasing trend of postoperative complications in the 3-year span indicates a constantly improving medical performance and working system in our hospital.
There are limitations of our study associated with data collection. Firstly, our data were collected from a single-center thus might not simply generalize to other settings. It is known that there are variations in working systems as well as patient characteristics between hospitals and locations. Secondly, the electronic medical records did not reveal detailed information about postoperative complications, such as their specific type and severity, and both surgical and non-surgical complications were listed together in the data. The extraction of such details would benefit future studies. Thirdly, the sample size of surgeries commencing during the night (9 pm to 7 am) was around 1,500, which may be too small to rule out false positives. Finally, we excluded transplant surgeries during data preparation because these patients are likely to be more complicated thus subject to an inherently higher rate of complication than in other surgeries. In addition, transplants are mostly scheduled to commence at night due to donor-related factors, which would further affect the complication rate for night surgeries.
The results of this retrospective study suggest operative timing could influence postoperative complications. However, more research is required to determine higher-order correlations.
Acknowledgments
Funding: This work was supported by WITMED Project of Zhongshan Hospital Affiliated to Fudan University (2020ZHZS23).
Footnote
Reporting Checklist: The authors have completed the TRIPOD reporting checklist. Available at http://dx.doi.org/10.21037/atm-21-669
Data Sharing Statement: Available at http://dx.doi.org/10.21037/atm-21-669
Conflicts of Interest: All authors have completed the ICMJE uniform disclosure form (available at http://dx.doi.org/10.21037/atm-21-669). The authors have no conflicts of interest to declare.
Ethical Statement: The authors are accountable for all aspects of the work in ensuring that questions related to the accuracy or integrity of any part of the work are appropriately investigated and resolved. All procedures performed in this study involving human participants were in accordance with the Declaration of Helsinki (as revised in 2013). This single-center, retrospective validation study was approved by the Review Board of the Ethics Committee of Zhongshan Hospital affiliated with Fudan University. Individual consent for this retrospective analysis was waived.
Open Access Statement: This is an Open Access article distributed in accordance with the Creative Commons Attribution-NonCommercial-NoDerivs 4.0 International License (CC BY-NC-ND 4.0), which permits the non-commercial replication and distribution of the article with the strict proviso that no changes or edits are made and the original work is properly cited (including links to both the formal publication through the relevant DOI and the license). See: https://creativecommons.org/licenses/by-nc-nd/4.0/.
References
- Hinz A, Glaesmer H, Brähler E, et al. Sleep quality in the general population: psychometric properties of the Pittsburgh Sleep Quality Index, derived from a German community sample of 9284 people. Sleep Med 2017;30:57-63. [Crossref] [PubMed]
- Doran SM, Van Dongen HP, Dinges DF. Sustained attention performance during sleep deprivation: evidence of state instability. Arch Ital Biol 2001;139:253-67. [PubMed]
- Spiegel K, Leproult R, Van Cauter E. Impact of sleep debt on metabolic and endocrine function. Lancet 1999;354:1435-9. [Crossref] [PubMed]
- Wyatt JK, Ritz-De Cecco A, Czeisler CA, et al. Circadian temperature and melatonin rhythms, sleep, and neurobehavioral function in humans living on a 20-h day. Am J Physiol 1999;277:R1152-63. [PubMed]
- Lim J, Dinges DF. A meta-analysis of the impact of short-term sleep deprivation on cognitive variables. Psychol Bull 2010;136:375-89. [Crossref] [PubMed]
- Owens JA. Sleep loss and fatigue in medical training. Curr Opin Pulm Med 2001;7:411-8. [Crossref] [PubMed]
- Weinger MB, Ancoli-Israel S. Sleep deprivation and clinical performance. JAMA 2002;287:955-7. [Crossref] [PubMed]
- Veasey S, Rosen R, Barzansky B, et al. Sleep loss and fatigue in residency training: a reappraisal. JAMA 2002;288:1116-24. [Crossref] [PubMed]
- Barger LK, Ayas NT, Cade BE, et al. Impact of extended-duration shifts on medical errors, adverse events, and attentional failures. PLoS Med 2006;3:e487 [Crossref] [PubMed]
- Grantcharov TP, Bardram L, Funch-Jensen P, et al. Laparoscopic performance after one night on call in a surgical department: prospective study. BMJ 2001;323:1222-3. [Crossref] [PubMed]
- Eastridge BJ, Hamilton EC, O'Keefe GE, et al. Effect of sleep deprivation on the performance of simulated laparoscopic surgical skill. Am J Surg 2003;186:169-74. [Crossref] [PubMed]
- Howard SK, Gaba DM, Rosekind MR, et al. The risks and implications of excessive daytime sleepiness in resident physicians. Acad Med 2002;77:1019-25. [Crossref] [PubMed]
- Kelz RR, Freeman KM, Hosokawa PW, et al. Time of day is associated with postoperative morbidity: an analysis of the national surgical quality improvement program data. Ann Surg 2008;247:544-52. [Crossref] [PubMed]
- Meschino MT, Giles AE, Rice TJ, et al. Operative timing is associated with increased morbidity and mortality in patients undergoing emergency general surgery: a multisite study of emergency general services in a single academic network. Can J Surg 2020;63:E321-8. [Crossref] [PubMed]
- Addis DR, Moore BA, Garner CR, et al. Case Start Time Affects Intraoperative Transfusion Rates in Adult Cardiac Surgery: A Single-Center Retrospective Analysis. J Cardiothorac Vasc Anesth 2020;34:632-9. [Crossref] [PubMed]
- Wang B, Yao Y, Wang X, et al. The start of gastrectomy at different time-of-day influences postoperative outcomes. Medicine (Baltimore) 2020;99:e20325 [Crossref] [PubMed]
- Switzer JA, Bennett RE, Wright DM, et al. Surgical time of day does not affect outcome following hip fracture fixation. Geriatr Orthop Surg Rehabil 2013;4:109-16. [Crossref] [PubMed]
- Sugünes N, Bichmann A, Biernath N, et al. Analysis of the Effects of Day-Time vs. Night-Time Surgery on Renal Transplant Patient Outcomes. J Clin Med 2019;8:1051. [Crossref] [PubMed]
- Lu Q, Shen Y, Zhang J, et al. Operation Start Times and Postoperative Morbidity from Liver Resection: A Propensity Score Matching Analysis. World J Surg 2017;41:1100-9. [Crossref] [PubMed]
- Jallad K, Barber MD, Ridgeway B, et al. The effect of surgical start time in patients undergoing minimally invasive sacrocolpopexy. Int Urogynecol J 2016;27:1535-9. [Crossref] [PubMed]
- Slaughter KN, Frumovitz M, Schmeler KM, et al. Minimally invasive surgery for endometrial cancer: does operative start time impact surgical and oncologic outcomes? Gynecol Oncol 2014;134:248-52. [Crossref] [PubMed]
- Kwon YS, Jang JS, Hwang SM, et al. Effects of surgery start time on postoperative cortisol, inflammatory cytokines, and postoperative hospital day in hip surgery: Randomized controlled trial. Medicine (Baltimore) 2019;98:e15820 [Crossref] [PubMed]
- Guidry CA, Davies SW, Willis RN, et al. Operative Start Time Does Not Affect Post-Operative Infection Risk. Surg Infect (Larchmt) 2016;17:547-51. [Crossref] [PubMed]
- Molnar, C. (2018). Interpretable Machine Learning: A Guide for Making Black Box Models Explainable (Leanpub)
- Breiman L. Random forests. Machine Learning 2001;45:5-32. [Crossref]
- Friedman JH. Greedy function approximation: A gradient boosting machines. The Annals of Statistics 2001;29:1189-232. [Crossref]
- Schapire RE. A brief introduction to boosting. Proceedings of the 16th International Joint Conference on Artificial Intelligence 1999;2:1401-6.
- Chen T, Carlos G. Xgboost: A scalable tree boosting system. Proceedings of the 22nd ACM SIGKDD International Conference on Knowledge Discovery and Data Mining, Association for Computing Machinery, 2016;785-94.
- Ke G, Meng Q, Finley T, et al. Lightgbm: a highly efficient gradient boosting decision tree. Proceedings of the 31st International Conference on Neural Information Processing Systems, 2017;3149-57.
- Prokhorenkova L, Gusev G, Vorobev A, et al. Catboost: unbiased boosting with categorical features. Proceedings of the 32nd International Conference on Neural Information Processing Systems 2018, 6639-49.
- Chawla NV, Bowyer KW, Hall LO, et al. Smote: Synthetic minority over-sampling technique. Journal of Artificial Intelligence Research 2002;16:321-57. [Crossref]
- Sanaka MR, Deepinder F, Thota PN, et al. Adenomas are detected more often in morning than in afternoon colonoscopy. Am J Gastroenterol 2009;104:1659-65. [Crossref] [PubMed]
- Teng TY, Khor SN, Kailasam M, et al. Morning colonoscopies are associated with improved adenoma detection rates. Surg Endosc 2016;30:1796-803. [Crossref] [PubMed]
- Hsu JC, Varosy PD, Parzynski CS, et al. Procedure timing as a predictor of inhospital adverse outcomes from implantable cardioverter-defibrillator implantation: insights from the National Cardiovascular Data Registry. Am Heart J 2015;169:45-52.e3. [Crossref] [PubMed]
- Jørgensen AB, Amirian I, Watt SK, et al. No Circadian Variation in Surgeons' Ability to Diagnose Acute Appendicitis. J Surg Educ 2016;73:275-80. [Crossref] [PubMed]
- Scheiermann C, Kunisaki Y, Frenette PS. Circadian control of the immune system. Nat Rev Immunol 2013;13:190-8. [Crossref] [PubMed]
- Cuesta M, Boudreau P, Dubeau-Laramée G, et al. Simulated Night Shift Disrupts Circadian Rhythms of Immune Functions in Humans. J Immunol 2016;196:2466-75. [Crossref] [PubMed]
- Geiger SS, Fagundes CT, Siegel RM. Chrono-immunology: progress and challenges in understanding links between the circadian and immune systems. Immunology 2015;146:349-58. [Crossref] [PubMed]
- Roenneberg T, Merrow M. The Circadian Clock and Human Health. Curr Biol 2016;26:R432-43. [Crossref] [PubMed]
- Vinden C, Nash DM, Rangrej J, et al. Complications of daytime elective laparoscopic cholecystectomies performed by surgeons who operated the night before. JAMA 2013;310:1837-41. [Crossref] [PubMed]
- Sugden C, Athanasiou T, Darzi A. What are the effects of sleep deprivation and fatigue in surgical practice? Semin Thorac Cardiovasc Surg 2012;24:166-75. [Crossref] [PubMed]
- Venkatraman V, Chuah YM, Huettel SA, et al. Sleep deprivation elevates expectation of gains and attenuates response to losses following risky decisions. Sleep 2007;30:603-9. [Crossref] [PubMed]
- Aiken CE, Aiken AR, Scott JG, et al. The influence of hours worked prior to delivery on maternal and neonatal outcomes: a retrospective cohort study. Am J Obstet Gynecol 2016;215:634.e1-7. [Crossref] [PubMed]
- Kork F, Spies C, Conrad T, et al. Associations of postoperative mortality with the time of day, week and year. Anaesthesia 2018;73:711-8. [Crossref] [PubMed]