A metabolism-related gene signature for predicting the prognosis and therapeutic responses in patients with hepatocellular carcinoma
Introduction
Hepatocellular carcinoma (HCC) accounts for 75–85% of all primary liver cancers. It is one of the most common malignant solid tumors in the world and the fourth leading cause of cancer-related deaths, with a 5-year survival rate of less than 20% (1,2). Although a small number of early-stage liver cancers can be treated by liver resection or liver transplantation, most liver cancer patients are in the middle to advanced stages of the disease at the time of diagnosis and often cannot be treated despite careful monitoring (3). Therefore, it is crucial to develop reliable prognostic tools that can predict clinical outcomes and assist in the decision-making process regarding observations, surgery, medications, and conservative treatments.
Reprogramming of cell metabolism and changes in bioenergetics have become characteristic signs of cancer (4). Cancer cells increase aerobic glycolysis, glutamine decomposition, and fat synthesis to meet their abnormal needs for proliferation and survival (5). It is now believed that the internal metabolism of cancer cells helps support the growth and metastasis of malignant tumors and affects the phenotype of malignant tumors. Cell energy reprogramming in the tumor microenvironment (TME) usually inevitably affects cells of the immune system (6). Consumption of glucose by tumors metabolically limits T cells, thereby promoting tumor progression (7). Lipid metabolism components constitute the main part of the daily diet and have a proven role in immune cell induction (8). Increasing evidence have shown that immunity and metabolism are key factors that are closely intertwined.
With the increasing application of bioinformatics analysis in the diagnosis and prognosis of malignancies, some researchers have linked metabolomics with genomics to analyze related metabolites (9). Although some liver cancer prognostic models based on metabolism-related genes have been established (10), it remains to be established whether the relevant indicators can also simultaneously predict the tumor immune microenvironment and the effects of immunotherapy in patients with liver cancer. The existing models have some limitations. Too many genes are required to construct a model, and its clinical practicality is not high (11). To this end, based on cancer genomics and bioinformatics, this study established a prognostic risk score based on metabolism-related genes. The prognostic value of the model was shown through Kaplan-Meier survival analysis, time-dependent receiver operating characteristic (ROC) curves, and nomograms. CIBERSORT was used to evaluate the relationship between the prognostic model and the tumor immune microenvironment. In addition, the study also analyzed the potential predictive value of the model in terms of tumor mutation burden (TMB), immunotherapy efficacy, and chemotherapeutic sensitivity. We present the following article in accordance with the TRIPOD reporting checklist (available at http://dx.doi.org/10.21037/atm-21-927).
Methods
Data collection and processing
The transcriptomic and clinical data of 352 HCC patients were obtained from The Cancer Genome Atlas (TCGA) Liver Hepatocellular Carcinoma (LIHC) database (https://portal.gdc.cancer.gov/). All data were background corrected prior to integration. The original data were normalized to quartiles, and then log2 conversion was performed to obtain the expression value of the normal distribution.
In this study, the clinical variables were age, gender, T stage, histological grade, survival status, and survival time. Cases of intrahepatic cholangiocarcinoma, normal tissue, and data sets with incomplete survival information were excluded from this study. Subsequently, patients were divided into a training cohort (n=212) and a testing cohort (n=140) at a ratio of 6:4. The study was conducted in accordance with the Declaration of Helsinki (as revised in 2013) (12).
Extraction of metabolism-related genes
All genes related to metabolism in this study were obtained from the “Kyoto Encyclopedia of Genes and Genomes” (KEGG), accessed on November 10, 2020. After analyzing the entire sample genome and the intersection with the metabolism genome, 1,466 genes related to metabolism were identified in the transcriptome data. The expression levels of these genes were extracted from each case for further analysis.
Construction of the metabolism-related signature
Patients who were followed up for more than 1 month were included in the survival analysis. The “survival” package was used to perform univariate Cox regression analysis to screen for survival-related metabolic genes in the training cohort. The LASSO-Cox regression model was then performed for variable screening and complexity adjustment to screen potential genes to establish the metabolic gene signature (13). The penalty value parameter was determined after a 100-fold cross-validation using the “glmnet” package (14).
Finally, a formula was developed using the gene signature constructed above. Risk score = expression of a gene [1] × corresponding coefficient [1] + expression of a gene [2] × corresponding coefficient [2] + expression of gene [n] ×corresponding coefficient [n].
The best critical value was determined using the R packages (“survival” and “survminer”) and a double-sided log-rank test. According to the threshold, patients were divided into high-risk and low-risk cohorts. Kaplan-Meier survival curves and log-rank tests were used to compare the differences in survival between high-risk and low-risk groups. The R package “survivalROC” was used to plot the time-dependent ROC curve to determine the prognostic value of the signature with OSin HCC patients (15). The predictive value of the prognostic gene signature was further studied in the testing cohort and the entire cohort. The nomogram (“rms” package), calibration curve (“rms” package) (16), and decision curve analysis (DCA) were used to assess the clinical utility and accuracy of the prognostic model.
Comprehensive analysis of molecular characteristics
To assess the potential differences in biological functions between the high- and low-risk groups, GSEA software (https://www.gsea-msigdb.org/gsea/login.jsp) was used on the basis of Hallmarks gene set (“h.all.v7.0.symbols.gmt”). Gene mutation information was downloaded from the cBioPortal database (HTTP://www.cbioportal.org/).
The tumor mutational burden (TMB) is an emerging therapeutic measure of sensitivity to immunotherapy and the TMB scores of the HCC patients in the TCGA cohort were calculated as previously described (17).
Estimation of tumor-infiltrating immune cells
The tumor immune microenvironment in the high- and low-risk groups were compared using two independent tools, CIBERSORT (HTTPS://cibersort.stanford.edu/) (18) and ImmuCellAI2 (http://bioinfo.life.hust.edu.cn/web/ImmuCellAI/) (19). Differences in the relative proportions of 22 types of immune cells and the clinicopathologic features between the high- and low-risk groups were compared in a landscape map.
Chemotherapeutic and Immunotherapeutic response prediction
The chemotherapeutic response of each sample was assessed by determining the half-maximal inhibitory concentration (IC50) via the R package “pRRophetic” based on the GDSC (Genomics of Drug Sensitivity in Cancer) database (https://www.cancerrxgene.org/) (20). Four chemotherapeutic drugs, cisplatin, gemcitabine, sorafenib, and docetaxel, were selected in this study. The TIDE algorithm (http://tide.dfci.harvard.edu/) and subclass mapping (SubMap; https://cloud.genepattern.org/gp/) were used to predict the clinical responses to two immune checkpoint inhibitors, including anti-PD1 and anti-CTLA4, as previously described (21). A Bonferroni-corrected P value <0.05 was considered statistically significant.
Statistical analysis
All statistical analyses were performed using the Software R version 3.6.0 (http://www.r-project.org). Wilcoxon tests were used to compare continuous variables, and chi-square tests were used to compare categorical variables. Differences in risk scores between various clinicopathological parameters were analyzed by the Student’s t-test. OS was defined as the time from the date of diagnosis to death from any cause. Significant differences in Kaplan-Meier curves were examined using the log-rank test. All statistical tests were two-sided, and P<0.05 was considered statistically significant.
Results
Construction of the five-gene risk score model
After excluding cases of intrahepatic cholangiocarcinoma, cases of normal tissue, and cases without survival information, a total of 352 patients were included in this study. Subsequently, the patients were randomly divided into a training cohort (n=212) and a testing cohort (n=140) at a ratio of 6:4. The univariate Cox regression analysis was adopted in the training cohort for screening survival-related metabolic genes. A total of 19 metabolic genes (P<0.05) were analyzed (Figure 1A).
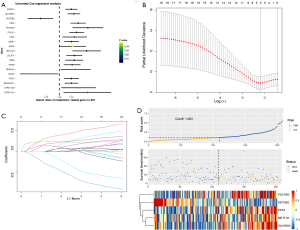
The LASSO algorithm was then used to decrease overfitting and the metabolism-related genes were narrowed down to 5 genes, namely, METTL6, POLR3G, PPAT, SETDB2 and SUV39H2. These 5 genes were used for constructing the following risk score model (Figure 1B,C): risk score = METTL6 × (0.336) + POLR3G × (0.614) + PPAT × (0.252) + SETDB2 × (−0.91) + SUV39H2× (0.338).
Each patient was then scored on the basis of this model. The best cut-off value that differentiated HCC patients into high- and low-risk groups was determined to be −1.063. The distribution of risk scores, the survival status, and the heatmap of the 5 prognostic genes are displayed in Figure 1D.
The association of the overall survival with the risk scores
As shown in the Kaplan-Meier survival curves in Figure 2A, high-risk patients showed significantly poorer OS compared to low-risk patients in the training cohort (P<0.0001). Similar results were observed in both the testing cohort and the entire cohort (Figure 2B). Time-dependent ROC curves showed that area under the curve (AUC) for the 1-, 3-, and 5-year OS of the training cohort, the testing cohort, and the entire cohort were 0.72, 0.761, and 0.764 (Figure 2C); 0.817, 0.703, and 0.677 (Figure 2D); and 0.75, 0.737, and 0.705 (Figure 2E), respectively. In conclusion, the five-gene signature model performed well in terms of OS prediction in patients with HCC.
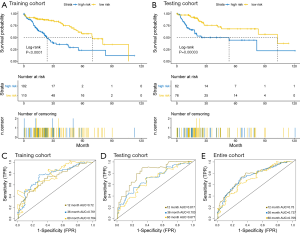
The risk score model is significantly correlated with disease progression
The potential relationships between the risk score model and the different clinicopathological characteristics were investigated. Considering the entire cohort, the risk score was significantly higher in HCC patients with advanced histological grade and advanced T stage (all P<0.001, Figure 3A). However, gender and age groups did not affect the risk scores (Figure 3A) . Subgroup survival analyses revealed that patients in the high-risk group have a poorer prognosis, irrespective of whether they had stage I/II (P<0.05; Figure 3B) or stage III/IV tumors (P<0.05; Figure 3C). These results demonstrated that the risk score model was statistically related to clinicopathological characteristics, and the higher the score, the poorer the clinical-pathological status.
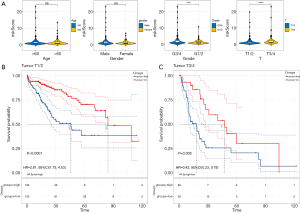
Development and evaluation of the nomogram
To further confirm the model’s predictive power and quantify its accuracy, the risk score and four clinical variables, including age, gender, T stage, and grade, were further integrated into the nomogram. This was then used to predict the 1-, 3-, and 5-year OS (Figure 4A). The calibration curves of the 5-gene prognostic nomogram demonstrated excellent consistency between actual observations and predicted values (Figure 4B). The decision curve analysis (DCA) was adopted for comparing the net clinical benefit between the nomogram and the conventional staging system. As shown in Figure 4C, the prognostic nomogram demonstrated a higher net benefit for predicting 2-, 3-, and 5-year OS compared to the T staging method.
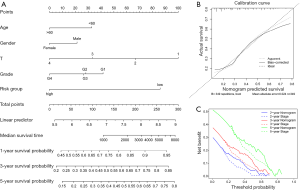
Molecular characteristics of patients in the different subgroups
GSEA software was used to find the differences between the high- and low-risk groups in the Hallmark pathway. The results showed that in the high-risk group, the top five pathways were all associated with cell proliferation, including G2M checkpoints, E2F targets, DNA repair, MYC targets V1, and MYC targets V2. While the top five pathways in the low-risk group were DNA repair, MYC targets V1, MYC targets V2, interferon alpha response, and mTOR complex 1 (MTORC1) signaling.
Somatic mutation and clonal selection can lead to cancer development (22). Therefore, gene mutations in the high- and low-risk groups were analyzed and the top ten genes with the highest mutation rates were identified (Figure 5). The mutation rates of TP53, titin (TTN), catenin beta 1 (CTNNB1), and mucin 16 (MUC16) were greater than or equal to 15% in HCC patients in both the high- and low-risk groups. Additionally, TP53 mutations were the most enriched in the high-risk group and significantly more prevalent compared to that in the low-risk group (41% vs. 19%).
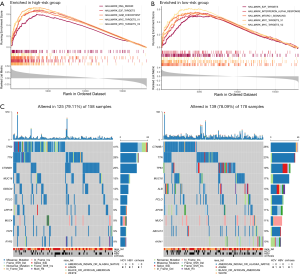
Recent studies have described certain associations between the genomic landscape and antitumor immunity. Since the tumor mutational burden (TMB) has been regarded as a promising biomarker for immune checkpoint inhibitors (23), the relationship between risk scores and the TMB was analzyed. As shown in Figure S1, patients in the high-risk group displayed higher tumor mutational load compared to patients in the low-risk group (P=0.013).
Immune characteristics of patients in the high- and low-risk groups
To further investigate HCC tumor heterogeneity, the tumor-infiltrating immune cells in the high- and low-risk groups were studied using two independent tools. The immune characteristics of 352 HCC samples were identified according to their gene expression data by using the CIBERSORT algorithm. The clinicopathologic features and the relative proportions of 22 different types of immune cells expressed in the high- and low-risk groups were then compared using a landscape map (Figure 6A). It can be seen from Figure 6A that M0 macrophages were more enriched in the high-risk group, while CD8 T cells and CD4 memory resting T cells were more abundant in the low-risk group. To more robustly and accurately evaluate the abundance of immune cells in the TME between the different subgroups, further analysis was performed using ImmuCellAI (19). As shown in Figure 6B, patients in the high-risk group were significantly correlated with a higher proportion of B cells, dendritic cells (DCs), neutrophils, natural regulatory T cells (nTreg), effector memory T (Tem) cells, and T regulatory type 1 (Tr1) cells. Conversely, patients in the low-risk group showed higher levels of CD4 T cells, naïve CD4 T cells, mucosal associated invariant T (MAIT) cells, natural killer (NK) cells, cytotoxic T (TC) cells, central memory T (Tcm) cells, and T follicular helper (Tfh) cells. These results indicated that our risk score model was significantly related to immune scores and may help to predict the immune microenvironment.
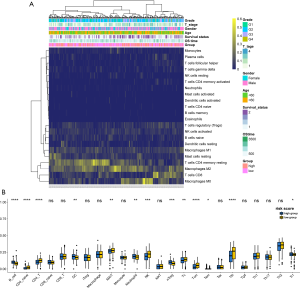
Chemotherapeutic and immunotherapeutic sensitivities of patients in the high- and low-risk groups
For patients with advanced unresectable HCC, systemic therapy may reduce the tumor burden and prolong their life. This study evaluated the response of patients in the high- and low-risk groups to four chemotherapy drugs, including cisplatin, gemcitabine, sorafenib, and docetaxel. With the exception of sorafenib, the other three chemotherapy drugs all showed significant differences in IC50 values between liver cancer patients in the high- and low-risk groups, with high-risk patients showing increased sensitivity to all three chemotherapy agents (Figure 7A).
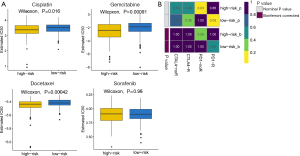
Recently, it has been reported that some immune checkpoint inhibitors, such as the anti-programmed cell death protein 1 (PD-1)/programmed death ligand-1 (PD-L1) antibody (24) and the anti-cytotoxic T-lymphocyte-associated protein 4 (CTLA-4) antibody (25), are promising therapeutic strategies for advanced HCC patients. Therefore, the clinical response of HCC patients to the PD-1 inhibitor and the CTLA-4 inhibitor was assessed. Although not statistically significant, patients in the low-risk group showed higher sensitivity to anti-PD-1 therapy (nominal P value =0.05, Bonferroni-corrected P value =0.46; Figure 7B).
Discussion
The incidence of liver cancer has increased significantly in the past two decades, and the mortality rate is not optimal (2). Therefore, identification of effective biomarkers for constructing good prognostic models to predict HCC patient survival is urgently needed. With the in-depth studies of metabolomics, the role of metabolism in cancer progression has received increasing attention (26).
This study identified a robust risk score model comprised of five genes, METTL6, POLR3G, PPAT, SETDB2, and SUV39H2, based on the TCGA-LIHC dataset. All these genes have been shown to be related to tumor growth and metastasis in previous studies (27-31). METTL6 belongs to the methyltransferase superfamily and can regulate pluripotency and promote tumor cell growth (27). Up-regulation of METTL6 on the cell surface can increase cisplatin sensitivity in the lung cancer cells (32). POLR3G is highly expressed in transitional cell carcinoma tissues compared with normal bladder tissues. Higher POLR3G expression is linked to lower OS (28). PPAT expression is one of the strongest markers for poor prognosis in small cell lung cancer (29). SUV39H2 promotes colorectal cancer cell proliferation and metastasis through the SLIT1 promoter tri-methylation (31). SETDB2 may contribute to gastric cancer progression (30). Consistent with above analysis, our study demonstrated that high-risk patients had significantly poorer OS The model developed in this study was statistically related to clinicopathological characteristics, and the higher the score, the poorer the clinical-pathological status. The risk scores, age, gender, and TNM stage were incorporated into a nomogram and a score was obtained for each patient. The results of the ROC and DCA confirmed the superiority of the nomogram for clinical judgment and prediction compared with the conventional staging system (P<0.05).
GSEA analysis was performed to investigate the biological processes in the two groups of patients. It revealed that the top upregulated HALLMARK gene sets in patients in the high-risk group were primarily associated with cell cycle-related pathways, which form the canonical signaling pathways involved in the initiation, invasion, and metastasis of tumor cells. In addition to the three common signal pathways, “DNA-Repair”, “Myc-Targets-V1”, and “Myc-Targets-V2”, two other significantly enriched immune-related pathways were identified in patients in the low-risk group. The mechanistic target of rapamycin (mTOR) signaling pathway is closely related to immune and inflammatory effects and plays an important role in regulating T cell development, activation and differentiation. Activation of the mTOR signaling pathway is crucial for maintaining the function of T cells (33). Interferon alpha can stimulate IFN-γ production by NK cells (34), and it contributes to developing a CD4+ and CD8+ dependent adaptive immune response (35) and modulates the Th1/Th2 balance towards Th1 (36). These results suggested that the difference in survival between the high- and low-risk groups may be closely related to the tumor immune microenvironment.
Therefore, the differences in the tumor-infiltrating immune cells between the two groups were examined. It was reported that the increase of T cells and NK cells in the tumor was related to the good prognosis of HCC, while the increase of Treg in the tumor was linked to the poor clinical outcome (37). The results demonstrated that neutrophils, nTreg cells, and Tr1 cells were markedly enriched in the high-risk group. Tr1 and nTreg cells participate in the immunoevasion of certain tumors and exerts immunosuppressive effects (38). Tumors stimulate neutrophils to promote tumor migration, invasion, and metastasis (39). In contrast, several antitumor cells, such as MAIT, NK, TC, and Tcm cells that are critical to killing cancer cells and eradicating tumors (40), showed higher infiltration in the tumor tissues of the low-risk group. Although the TMB values of high-risk patients were significantly higher than that of low-risk patients, no advantage of immunotherapy was observed in the high-risk groups. The lack of tumor T cell infiltration is one of the main causes of the resistance to immunotherapy (41). The immune cell infiltration patterns above may help to explain this phenomenon. Chemotherapy is one of the important treatments for advanced liver cancer. Interestingly, high-risk patients with HCC were more sensitive to cisplatin, gemcitabine, and docetaxel compared to low-risk patients.
Since our model reduces the need for whole-genome sequencing, it may be more cost-effective for routine use. However, several limitations should be considered. First, our research only focused on the mRNA sequencing data from TCGA. The sample size was small, and the study merely observed a trend that patients in the low-risk group were likely to be more sensitive to anti-PD-1 therapy. Future work will need to further explore other public databases to externally verify the prognostic value of the model in a more independent cohort. Second, as this is a retrospective study, there may be potential bias associated with the imbalance of clinicopathological characteristics. Further multicenter prospective studies are urgently needed. Third, this model was constructed through data mining and functional experiments are necessary to confirm its clinical value in patients with HCC.
In summary, for the first time, this study identified a 5-gene risk signature related to metabolism which can be used independently to predict the prognosis of patients with HCC. This signature may provide guidance for targeted therapies and may act as a potential biomarker in patients with HCC.
Acknowledgments
Funding: This work was supported by grants from the National Natural Scientific Funding (81702608) to NSJ and the Science and Technology Project of Nantong (MSZ20207) to YNH.
Footnote
Reporting Checklist: The authors have completed the TRIPOD reporting checklist. Available at http://dx.doi.org/10.21037/atm-21-927
Conflicts of Interest: All authors have completed the ICMJE uniform disclosure form (available at http://dx.doi.org/10.21037/atm-21-927). The authors have no conflicts of interest to declare
Ethical Statement: The authors are accountable for all aspects of the work in ensuring that questions related to the accuracy or integrity of any part of the work are appropriately investigated and resolved. The study was conducted in accordance with the Declaration of Helsinki (as revised in 2013).
Open Access Statement: This is an Open Access article distributed in accordance with the Creative Commons Attribution-NonCommercial-NoDerivs 4.0 International License (CC BY-NC-ND 4.0), which permits the non-commercial replication and distribution of the article with the strict proviso that no changes or edits are made and the original work is properly cited (including links to both the formal publication through the relevant DOI and the license). See: https://creativecommons.org/licenses/by-nc-nd/4.0/.
References
- Bray F, Ferlay J, Soerjomataram I, et al. Global cancer statistics 2018: GLOBOCAN estimates of incidence and mortality worldwide for 36 cancers in 185 countries. CA Cancer J Clin 2018;68:394-424. [Crossref] [PubMed]
- Siegel RL, Miller KD, Jemal A. Cancer statistics, 2020. CA Cancer J Clin 2020;70:7-30. [Crossref] [PubMed]
- Yang JD, Hainaut P, Gores GJ, et al. A global view of hepatocellular carcinoma: trends, risk, prevention and management. Nat Rev Gastroenterol Hepatol 2019;16:589-604. [Crossref] [PubMed]
- Chen X, Qian Y, Wu S. The Warburg effect: evolving interpretations of an established concept. Free Radic Biol Med 2015;79:253-63. [Crossref] [PubMed]
- Jin T, Wang C, Tian Y, et al. Mitochondrial metabolic reprogramming: An important player in liver cancer progression. Cancer Lett 2020;470:197-203. [Crossref] [PubMed]
- Wang X, Ping F, Bakht S, et al. Immunometabolism features of metabolic deregulation and cancer. J Cell Mol Med 2019;23:694-701. [Crossref] [PubMed]
- Chang CH, Qiu J, O'Sullivan D, et al. Metabolic Competition in the Tumor Microenvironment Is a Driver of Cancer Progression. Cell 2015;162:1229-41. [Crossref] [PubMed]
- Ringel A, Drijvers J, Baker G, et al. Obesity Shapes Metabolism in the Tumor Microenvironment to Suppress Anti-Tumor Immunity. Cell 2020;183:1848-66.e26. [Crossref] [PubMed]
- Luo T, Li Y, Nie R, et al. Development and validation of metabolism-related gene signature in prognostic prediction of gastric cancer. Comput Struct Biotechnol J 2020;18:3217-29. [Crossref] [PubMed]
- Zhu Z, Li L, Xu J, et al. Comprehensive analysis reveals a metabolic ten-gene signature in hepatocellular carcinoma. PeerJ 2020;8:e9201 [Crossref] [PubMed]
- Yang C, Huang X. Metabolism-associated molecular classification of hepatocellular carcinoma. Mol Oncol 2020;14:896-913.
- World Medical Association Declaration of Helsinki. ethical principles for medical research involving human subjects. JAMA 2013;310:2191-4. [Crossref] [PubMed]
- Shahraki H, Salehi A, Zare N. Survival Prognostic Factors of Male Breast Cancer in Southern Iran: a LASSO-Cox Regression Approach. Asian Pacific journal of cancer prevention APJCP 2015;16:6773-7. [PubMed]
- Friedman J, Hastie T, Tibshirani R. Regularization Paths for Generalized Linear Models via Coordinate Descent. J Stat Softw 2010;33:1-22. [Crossref] [PubMed]
- Obuchowski N, Bullen J. Receiver operating characteristic (ROC) curves: review of methods with applications in diagnostic medicine. Phys Med Biol 2018;63:07TR01 [Crossref] [PubMed]
- Núñez E, Steyerberg EW, Núñez J. Regression modeling strategies. Rev Esp Cardiol 2011;64:501-7. [PubMed]
- Chalmers ZR, Connelly CF, Fabrizio D, et al. Analysis of 100,000 human cancer genomes reveals the landscape of tumor mutational burden. Genome Med 2017;9:34. [Crossref] [PubMed]
- Chen B, Khodadoust MS, Liu CL, et al. Profiling Tumor Infiltrating Immune Cells with CIBERSORT. Methods Mol Biol 2018;1711:243-59. [Crossref] [PubMed]
- Miao YR, Zhang Q, Lei Q, et al. ImmuCellAI: A Unique Method for Comprehensive T-Cell Subsets Abundance Prediction and its Application in Cancer Immunotherapy. Adv Sci (Weinh) 2020;7:1902880 [Crossref] [PubMed]
- Geeleher P, Cox NJ, Huang RS. Clinical drug response can be predicted using baseline gene expression levels and in vitro drug sensitivity in cell lines. Genome Biol 2014;15:R47. [Crossref] [PubMed]
- Wang Z, Guo X, Gao L, et al. Glioblastoma cell differentiation trajectory predicts the immunotherapy response and overall survival of patients. Aging (Albany NY) 2020;12:18297-321. [Crossref] [PubMed]
- Martincorena I, Raine KM, Gerstung M, et al. Universal Patterns of Selection in Cancer and Somatic Tissues. Cell 2017;171:1029-41.e21. [Crossref] [PubMed]
- Rooney MS, Shukla SA, Wu CJ, et al. Molecular and genetic properties of tumors associated with local immune cytolytic activity. Cell 2015;160:48-61. [Crossref] [PubMed]
- El-Khoueiry AB, Sangro B, Yau T, et al. Nivolumab in patients with advanced hepatocellular carcinoma (CheckMate 040): an open-label, non-comparative, phase 1/2 dose escalation and expansion trial. Lancet 2017;389:2492-502. [Crossref] [PubMed]
- Duffy AG, Ulahannan SV, Makorova-Rusher O, et al. Tremelimumab in combination with ablation in patients with advanced hepatocellular carcinoma. J Hepatol 2017;66:545-51. [Crossref] [PubMed]
- Lin X, Wu Z, Hu H, et al. Non-coding RNAs rewire cancer metabolism networks. Semin Cancer Biol 2021; Epub ahead of print. [Crossref] [PubMed]
- Ignatova VV, Kaiser S, Ho JSY. METTL6 is a tRNA m(3)C methyltransferase that regulates pluripotency and tumor cell growth. Sci Adv 2020;6:eaaz4551 [Crossref] [PubMed]
- Liu X, Zhang W, Wang H, et al. Increased expression of POLR3G predicts poor prognosis in transitional cell carcinoma. PeerJ 2020;8:e10281 [Crossref] [PubMed]
- Kodama M, Oshikawa K, Shimizu H, et al. A shift in glutamine nitrogen metabolism contributes to the malignant progression of cancer. Nat Commun 2020;11:1320. [Crossref] [PubMed]
- Nishikawaji T, Akiyama Y, Shimada S, et al. Oncogenic roles of the SETDB2 histone methyltransferase in gastric cancer. Oncotarget 2016;7:67251-65. [Crossref] [PubMed]
- Shuai W, Wu J, Chen S, et al. SUV39H2 promotes colorectal cancer proliferation and metastasis via tri-methylation of the SLIT1 promoter. Cancer Lett 2018;422:56-69. [Crossref] [PubMed]
- Tan XL, Moyer AM, Fridley BL, et al. Genetic variation predicting cisplatin cytotoxicity associated with overall survival in lung cancer patients receiving platinum-based chemotherapy. Clin Cancer Res 2011;17:5801-11. [Crossref] [PubMed]
- Zeng H, Chi H. mTOR signaling in the differentiation and function of regulatory and effector T cells. Curr Opin Immunol 2017;46:103-11. [Crossref] [PubMed]
- Hunter CA, Gabriel KE, Radzanowski T, et al. Type I interferons enhance production of IFN-gamma by NK cells. Immunol Lett 1997;59:1-5. [Crossref] [PubMed]
- Earl PL, Americo JL, Moss B. Insufficient Innate Immunity Contributes to the Susceptibility of the Castaneous Mouse to Orthopoxvirus Infection. J Virol 2017;91:e01042-17. [Crossref] [PubMed]
- Boyoglu-Barnum S, Todd SO, Meng J, et al. Mutating the CX3C Motif in the G Protein Should Make a Live Respiratory Syncytial Virus Vaccine Safer and More Effective. J Virol 2017;91:e02059-16. [Crossref] [PubMed]
- Sachdeva M, Arora SK. Prognostic role of immune cells in hepatocellular carcinoma. EXCLI J 2020;19:718-33. [PubMed]
- Matsuda M, Doi K, Tsutsumi T, et al. Regulation of allergic airway inflammation by adoptive transfer of CD4(+) T cells preferentially producing IL-10. Eur J Pharmacol 2017;812:38-47. [Crossref] [PubMed]
- Rapoport BL, Steel HC. Role of the Neutrophil in the Pathogenesis of Advanced Cancer and Impaired Responsiveness to Therapy. Molecules 2020;25:1618. [Crossref] [PubMed]
- Martínez-Lostao L, Anel A, Pardo J. How Do Cytotoxic Lymphocytes Kill Cancer Cells? Clin Cancer Res 2015;21:5047-56. [Crossref] [PubMed]
- Durgeau A, Virk Y, Corgnac S, et al. Recent Advances in Targeting CD8 T-Cell Immunity for More Effective Cancer Immunotherapy. Front Immunol 2018;9:14. [Crossref] [PubMed]
(English Language Editor: J. Teoh)