A novel metabolic gene signature-based nomogram to predict overall survival in breast cancer
Introduction
Breast cancer is the most common cancer in females, accounting for 30% of new cancer diagnoses (1). It is the second leading cause of cancer death in females worldwide, responsible for nearly 15% of cancer-related fatalities. Despite the dramatic improvement in breast cancer prognosis due to advances in early diagnosis and treatment over the past decades, the high incidence and mortality of breast cancer still poses a significant threat to human health (2).
Growing evidence has shown that breast cancer is a heterogeneous disease. Patients with similar clinicopathological characteristics may differ in clinical outcomes because of different gene expression patterns (3). In recent years, the advent of high-throughput platforms has created great opportunities for researchers to explore the distinct molecular aberrations among tumors (4). On the basis of conventional clinicopathological features, individual gene signatures provide complementary information to predict disease prognosis (5,6). Among these gene signatures, a 50-gene classifier called prediction analysis of microarray 50 (PAM50) helps classify breast cancers into five intrinsic subtypes with distinct clinical outcomes: luminal A, luminal B, human epidermal growth factor receptor 2 (HER2)-overexpressed, basal-like, and normal-like tumors (7). However, the PAM50 signature alone cannot explain the discrepancies between the prognoses in patients with the same subtype, possibly because PAM50 primarily includes proliferation-associated genes (8). As a result, gene signatures involving diverse biological processes are needed to more precisely predict patient survival.
The relationship between metabolism and cancer is multifaceted and bidirectional. Despite the biological significance of metabolism in cancer, metabolic gene signature (MGS) has not been implemented to predict survival in breast cancer patients. For one, aberrant metabolism is a hallmark of cancer, and is involved in diverse aspects of cancer including tumorigenesis, proliferation, and metastasis (9,10). Energy and biomass (nucleotides, amino acids, and lipids) produced via metabolic processes are necessary to satisfy the excessive proliferation of cancer cells and to adapt to environmental stress (11). Metabolites are essential in coordinating gene expression and nutrient utilization (12). Under these circumstances, metabolic pathways are rewritten to adapt to changes in cancer progression. For another, dysregulation of oncogenes and tumor suppressors could result in impaired regulation of metabolic pathways (13-15), and metabolic phenotype is now used as a treatment target and can provide clues regarding cancer treatment (10).
Many metabolic pathways support the survival of cancer (16-19). Based on the complexity and diversity of tumor metabolism, genes from various metabolic pathways were selected to develop an MGS to predict breast cancer prognosis. In this study, metabolic genes with statistical significance in univariate Cox hazard analysis were incorporated into the least absolute shrinkage and selection operator (LASSO) model to calculate the risk score for each patient. Time-dependent receiver operating characteristic (tROC) curve analysis was applied to compare the prediction accuracy of the risk scores and clinicopathological parameters. A nomogram was established based on the risk score and the American Joint Committee on Cancer (AJCC) staging, and calibration plots were constructed in both the discovery and validation datasets.
Our study aimed to elucidate the metabolic pathways contributing to cancer and explore the value of MGS in breast cancer risk stratification. We found that MGS could be used as an effective prognostic predictor and provides a rationale for the individual management of breast cancer patients. We present the following article in accordance with the TRIPOD reporting checklist (available at http://dx.doi.org/10.21037/atm-20-4813).
Methods
Datasets and preprocessing
Datasets from the Gene Expression Omnibus (GEO) database and The Cancer Genome Atlas (TCGA) dataset between 2011 and 2017 were reviewed. Datasets were included if they reported overall survival (OS) and clinicopathological parameters. GSE20685 (platform GPL570), comprising 327 patients, was selected as the discovery dataset, from which messenger RNA (mRNA) expression data and related clinical data for breast cancer were downloaded from the GEO database (http://www.ncbi.nlm.nih.gov/geo/). Probes were transformed to corresponding Entrez gene names referring to the annotation files.
For the validation dataset, the GSE86166 dataset and related clinical data were downloaded from the GEO dataset. A total of 366 patients were included in this dataset. Another validation dataset was obtained from TCGA database comprising 1,039 patients. Normalized fragments per kilobase of exon model per million reads mapped (FPKM) data and related clinical data were downloaded from TCGA (https://portal.gdc.cancer.gov/). The metabolic signature was downloaded from the Gene Set Enrichment Analysis (GSEA) dataset. Pathways including glucose and lipid metabolism, amino acid metabolism, and nucleotide metabolism were examined in our analysis, and metabolic genes in both datasets were extracted for further analysis. Batches were removed with the R package “sva”. The study was conducted in accordance with the Declaration of Helsinki (as revised in 2013).
Construction of the risk score
Univariate Cox regression was conducted to assess the association between gene expression levels and the OS of patients. Genes with a P value <0.05 were included in further analyses. Genes with a hazard ratio (HR) >1 indicated a poor prognosis, while genes with an HR <1 denoted a good prognosis. The LASSO model, which uses a L1-norm to penalize the weight of the model parameters, was then applied to remove genes of high correlation (20,21). A risk score formula was established by including individual normalized gene expression values weighted by their LASSO Cox coefficients as follows: ∑i1 Coefficient (mRNAi)*Expression (mRNAi). The R package “glmnet” in R 3.5.2 was used to conduct the LASSO analysis. The median value of the risk scores in the discovery dataset was used as the cutoff to divide patients into high- and low-risk groups in both the discovery and validation datasets. In the discovery dataset, the cutoff value from the tROC curve was also tested in different subtypes. Survival was compared between the high- and low-risk groups using the Kaplan-Meier (K-M) survival curve with log-rank tests.
Construction of the nomogram
To evaluate whether the risk score could serve as an independent prognostic indicator for breast cancer patients, univariate and multivariate Cox regression was used in both the discovery and validation datasets. There were 327, 716, and 341 patients with clinicopathological parameters in GSE20685, TCGA, and GSE86166 datasets, respectively. Parameters available in all datasets such as age, intrinsic subtype, and the AJCC tumor-node-metastasis (TNM) staging system (AJCC stage) were included in the analysis. The subtypes of patients were calculated using the “genefu” package in R. AJCC stage was statistically significant in univariate analysis and was assessed in further analysis. A nomogram was constructed to visualize the multivariate Cox regression. Variables with a P value <0.05 in the multivariate Cox regression were incorporated into the nomogram using the R package “rms” to predict 3-year OS rates.
Statistical analysis
The predictive accuracy of independent prognostic parameters including AJCC stage, subtypes, and risk score was calculated using the tROC curve. The discriminatory ability of different parameters was evaluated by the area under the ROC curve (AUC). The “survivalROC” package in R software was used to plot the tROC curve and calculate the AUC. The calibration plot was used to visualize the performance of the nomogram in all datasets. Functional annotation analysis, such as the Kyoto Encyclopedia of Genes and Genomes (KEGG) and Gene Ontology (GO) analysis, was performed on the Database for Annotation, Visualization and Integrated Discovery (DAVID) website (https://david.ncifcrf.gov/). Heatmaps of the LASSO analysis genes were plotted using the “heatmap” R package. A P value <0.05 was considered statistically significant.
Results
Screening of genes related to OS and construction of the MGS
The flowchart of the screening process for prognostic metabolic genes is presented in Figure 1. First, metabolic genes were extracted from the GSEA database and a total of 863 genes were included. The genes were further validated as metabolic genes via KEGG (Figure 2A) and GO (Figure 2B) analysis. In the KEGG analysis, the top five pathways were purine metabolism, pyrimidine metabolism, carbon metabolism, inositol phosphate metabolism, and glycerophospholipid metabolism (Figure 2A). In the GO analysis, the top five pathways were oxidation-reduction process, metabolic process, xenobiotic metabolic process, phosphatidylinositol biosynthetic process, and inositol phosphate metabolic process (Figure 2B). The GSE20685 dataset was used as the discovery cohort, while the GSE86166 and TCGA datasets were used as the validation cohorts. In the GSE20685 dataset, a total of 161 metabolic genes were statistically significant (P<0.001) in the univariate Cox regression analysis and were selected as candidate genes for further analysis (Table S1). To reduce the high correlation between the genes, LASSO regression analysis was conducted to identify robust markers (Figure 2C), and 55 genes were finally included to construct the MGS (Figure 2D). The coefficients of the genes are listed in Table S2. As shown in Figure 3, patients with a high-risk score exhibited significantly worse OS compared to those with a low-risk score in the discovery dataset (P<0.001, Figure 3A), which was confirmed in TCGA (P<0.001, Figure 3B) and the GSE86166 (P=0.032, Figure 3C) validation cohorts.
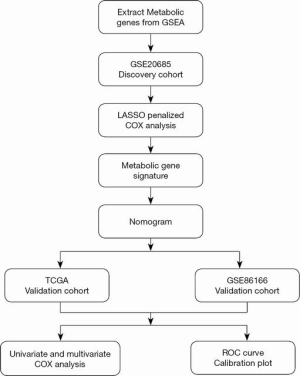
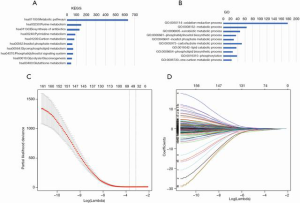
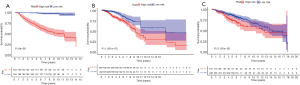
Assessment of the MGS
The OS of the low-risk group was significantly higher than that of the high-risk group in the discovery cohort (Figure 4A,B). The median OS was 9.48 years in the high-risk group, while it was still unreached in the low-risk group. Furthermore, in TCGA dataset, the OS of the high-risk group was also worse than that of the low-risk group (Figure 4C,D). The median survival time for the high- and low-risk groups was 11.4 and 17.69 years, respectively. In the GSE86166 validation dataset cohort, the median survival time for high- and low-risk groups was 12.6 and 14.4 years, respectively (Figure 4E,F).
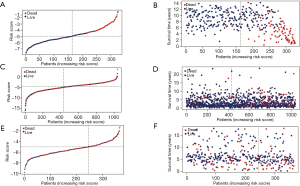
Detailed information about clinical characteristics is described in Table 1. In the discovery cohort, prognosis was correlated with risk score, AJCC stage, and subtype in the univariate analysis (Figure 5A); these factors were included in the multivariate analysis. Risk score [P<0.001, HR =2.275, 95% confidence interval (CI): 1.411, 3.668] and AJCC stage (P<0.01, HR =5.050, 95% CI: 3.910, 6.524) were identified as independent prognostic predictors in the multivariate analysis (Figure 5B). In TCGA cohort, age (P<0.001, HR =1.036, 95% CI: 1.019, 1.054), AJCC stage (P=0.010, HR =1.825, 95% CI: 1.157, 2.877), and risk score (P<0.001, HR =1.247, 95% CI: 1.115, 1.393) were identified as unfavorable prognostic factors and independent prognostic predictors in the multivariate analysis (Figure 5C,D). In the GSE86188 dataset, risk score (P=0.025, HR =1.229, 95% CI: 1.026, 1.473) and AJCC stage (P=0.001, HR =2.159, 95% CI: 1.363, 3.418) were independent prognostic predictors as identified through multivariate analysis (Figure 5E,F).
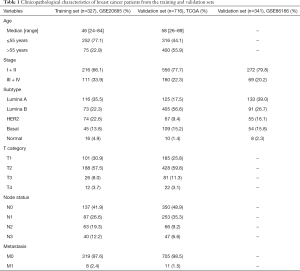
Full table
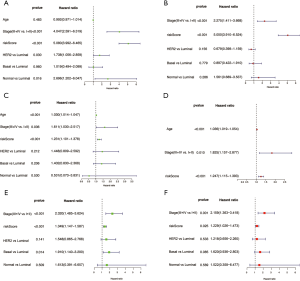
To evaluate whether MGS could predict survival regardless of intrinsic subtypes, K-M curves were plotted in different subtypes in the discovery dataset. The outcome of high-risk patients was significantly worse than that of low-risk patients (Figure 6A, P<0.001; Figure 6B, P<0.001; Figure 6C, P=0.007) regardless of their different cutoff values (Figure S1). The median survival time for the low-risk group was unreached in all three subtypes, which indicated that this MGS could stratify patients into different risk groups regardless of intrinsic subtypes.
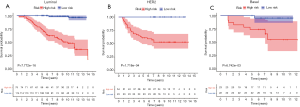
Indeed, the histological subtype of breast cancer has a notable effect on the prognosis. Thus, we performed subgroup analysis according to the histological subtype and found that there was no significant interaction between the MGS and the histological subtype (interaction P=0.36). In invasive ductal carcinoma (Figure S2A), a total of 462 patients (60.5%) were classified into the high-risk group, and 302 patients (39.5%) were classified into the low-risk group (P=0.002). In invasive lobular carcinoma (Figure S2B), a total of 86 patients (42.6%) were classified into the high-risk group and 116 patients (57.4%) were classified into the low-risk group (P=0.0004). In both invasive ductal carcinoma and invasive lobular carcinoma, patients with a high-risk score exhibited worse OS compared with patients with a low-risk score. This indicates that the MGS was a robust gene signature regardless of the pathological types in breast cancer. To assess whether the performance of the MGS was different in predicting the prognosis of males versus females, we applied our signature to lung cancer from TCGA database. A total of 938 patients with OS were included in this analysis. High-risk patients showed worse prognosis compared to low-risk patients in the entire dataset (P=0.026, Figure S3A). We then stratified patients according to sex and found that MGS could stratify female patients into high- and low-risk groups (P=0.045), while there was no difference between the high- and low-risk groups in males (P=0.23, Figure S3B,C). Thus, we cannot conclude that this method is more advantageous in predicting the prognosis of males compared to females; more studies are needed to evaluate whether there is a difference in the MGS for predicting the prognosis in different sexes.
The tROC curves were plotted to compare the prediction accuracy of the risk score, AJCC stage, and subtype on 3-year OS. In the discovery dataset, the AUC of the risk score was equal to 0.931. The AUC values of AJCC stage and subtype were 0.781 and 0.675, respectively (Figure 7A). In TCGA dataset, the AUC of risk score was 0.704, which was higher than that of subtype (AUC: 0.629) and AJCC stage (AUC: 0.601; Figure 7B). In the GSE86166 dataset, the AUC values of the risk score, AJCC stage, and subtype were 0.676, 0.667, and 0.615, respectively (Figure 7C).
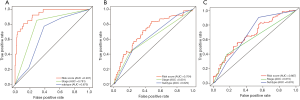
Construction of MGS-based model
In our analysis, statistically significant clinicopathological and genetic factors in both datasets (AJCC stage and risk score) were included to construct a nomogram (Figure 8A). The concordance index (C-index) of the nomogram was 0.91 (95% CI: 0.85–0.98), which indicated that this model had high prediction accuracy. The presentation of the calibration plot for patient survival prediction in the discovery and validation datasets demonstrated that the nomogram-predicted outcome had good agreement with the actual outcome (Figure 8B,C,D).
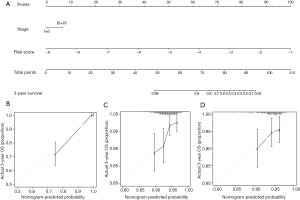
KEGG and GO pathway analysis of the MGS
KEGG and GO analyses were performed to further evaluate the function of the genes in the MGS (Figure 9).In the KEGG analysis, the top three pathways were histidine metabolism, arginine and proline metabolism, and glutathione metabolism (Figure 9A), indicating that our signature was associated with amino acid metabolism. In the GO analysis, the top three pathways were oxidation-reduction process pathway, xenobiotic metabolic process, and one-carbon metabolic process (Figure 9B).
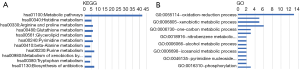
Discussion
The high incidence and mortality of breast cancer poses a significant threat to human health. Traditionally, clinicopathological factors have been common and important considerations for survival prediction of breast cancer patients (22). However, patients with the same clinical factors may differ in clinical outcomes due to diverse gene expression patterns (23,24). The conceptual changes in tumor heterogeneity have provided rationale for risk stratification and treatment decision-making based on gene expression profiles (25). In our study, an MGS was constructed and validated in different datasets. It was shown to be superior to the commonly used clinicopathological factors and could better predict the OS of breast cancer patients.
Cancer metabolism has experienced renewed interest in the past decade, with increasing evidence implying a vital role of metabolism in cancer tumorigenicity and malignancy (26). The application of a metabolic signature has been reported to predict prognosis in different cancers, such as thyroid and hepatocellular cancers; however, no metabolic signatures have been applied to predict outcomes in breast cancer. Metabolism pathways are complex, and one fundamental feature of cancer metabolism is the ability to obtain nutrients and biomass under nutritional stress (27).
To fully understand the impact of metabolism on prognosis, genes covering a variety of metabolic pathways including glucose and lipid metabolism, amino acid metabolism, and nucleotide metabolism were all involved in our analysis. After selection by LASSO analysis, amino acid metabolism pathways including histidine metabolism, arginine and proline metabolism, and glutathione metabolism were found to be largely enriched in our MGS, which indicated that amino acid metabolism played an important role in prognosis. Amino acids are essential nutrients for breast cancer proliferation and are involved in various cancer pathways (28). Histidine was reported to be associated with chemotherapy sensitivity in breast cancer (29), arginine plays an essential role in maintaining mammalian target of rapamycin (mTOR) pathway activation (30,31), while proline, which is catalyzed by two different enzymes (pyrroline-5-carboxylate reductase and proline dehydrogenase), can sustain intracellular nucleotide levels. The inhibition of proline biosynthesis in cancer cells was also found to impair tumorigenic potential and metastasis inhibition (32,33). Glutathione is the most abundant antioxidant in cells; it maintains cellular redox homeostasis and has a bidirectional effect on cancer progression. It is vital to the detoxification of carcinogens; however, excess glutathione promotes tumor progression (34). Incorporating these different metabolic pathways rather than selecting a particular metabolic pathway makes our prediction model more robust.
Our study highlighted the role of amino acid metabolism in breast cancer prognosis prediction. The application of an MGS in other types of tumors needs to be further explored. Moreover, it is also well established that there may be marked differences in metabolism between the sexes (35). Thus, the accuracy of an MGS in predicting the OS among patients of different sexes in other tumors needs to be further investigated.
In our study, OS was selected as the end point to evaluate clinical outcomes. LASSO regression analysis, an innovative shrinkage and selection method for regression analysis, converted a high-dimensional predictor into a low-dimensional predictor (36,37). The combination of univariate analysis and LASSO Cox regression, which has been used widely in numerous studies, was conducted to screen genes indicating either poor or good prognosis and to construct a robust gene signature (38,39). In our analysis, a 55-gene metabolic signature was developed to predict the OS of breast cancer patients. According to the tROC curves, the MGS showed a better prediction accuracy than did AJCC stage or PAM50.
Intrinsic subtypes are related to patient survival and can guide therapeutic strategies. Among patients with different PAM50 subtypes, our MGS could further classify these patients into different risk groups. Patients with low-risk scores had a better prognosis than patients with high-risk scores, indicating that the MGS can further guide individualized therapy regardless of molecular subtypes. Chemotherapy may be prescribed for patients with high-risk MGSs. Moreover, an appropriate target agent may be designed to improve the outcomes of these patients. Nomograms have been widely used to assess prognostic outcomes in cancer patients (40). A nomogram can incorporate statistical predictive models into a single numerical estimate of the probability of long-term OS for an individual patient. In our study, the metabolic signature and AJCC stage were identified as independent prognostic factors and were incorporated into the nomogram to predict the survival of patients. The high C-index of our nomogram indicated good accuracy in predicting the outcome of patients. Furthermore, calibration plots demonstrated that the nomogram had good discrimination in both the validation and discovery cohorts. However, more validation sets are needed to verify our signature.
Conclusions
Our results demonstrate that a risk score based on an MGS is superior to that based on AJCC stage or PAM50 subtypes in predicting the prognosis of breast cancer. This will provide evidence for further risk classification in guiding individualized management of breast cancer patients.
Acknowledgments
Funding: The authors appreciate the financial support provided by the National Natural Science Foundation of China (No. 81772797); the Shanghai Municipal Education Commission—Gaofeng Clinical Medicine Grant Support (No. 20172007); and the Ruijin Hospital, Shanghai Jiao Tong University School of Medicine “Guangci Excellent Youth Training Program” (No. GCQN-2017-A18). None of these financial sponsors had any role in the study design, collection, analysis, or interpretation of data.
Footnote
Reporting Checklist: The authors have completed the TRIPOD reporting checklist Available at http://dx.doi.org/10.21037/atm-20-4813
Conflicts of Interest: All authors have completed the ICMJE uniform disclosure form (available at http://dx.doi.org/10.21037/atm-20-4813). The authors have no conflicts of interest to declare.
Ethical Statement: The authors are accountable for all aspects of the work in ensuring that questions related to the accuracy or integrity of any part of the work are appropriately investigated and resolved. This study was conducted in accordance with the Declaration of Helsinki (as revised in 2013).
Open Access Statement: This is an Open Access article distributed in accordance with the Creative Commons Attribution-NonCommercial-NoDerivs 4.0 International License (CC BY-NC-ND 4.0), which permits the non-commercial replication and distribution of the article with the strict proviso that no changes or edits are made and the original work is properly cited (including links to both the formal publication through the relevant DOI and the license). See: https://creativecommons.org/licenses/by-nc-nd/4.0/.
References
- Siegel RL, Miller KD, Jemal A. Cancer statistics, 2020. CA Cancer J Clin 2020;70:7-30. [Crossref] [PubMed]
- Davies C, Godwin J, Gray R, et al. Relevance of breast cancer hormone receptors and other factors to the efficacy of adjuvant tamoxifen: patient-level meta-analysis of randomised trials. Lancet 2011;378:771-84. [Crossref] [PubMed]
- Walker RA, Jones JL, Chappell S, et al. Molecular pathology of breast cancer and its application to clinical management. Cancer Metastasis Rev 1997;16:5-27. [Crossref] [PubMed]
- Benjamin DI, Cravatt BF, Nomura DK. Global profiling strategies for mapping dysregulated metabolic pathways in cancer. Cell Metab 2012;16:565-77. [Crossref] [PubMed]
- Paik S, Shak S, Tang G, et al. A multigene assay to predict recurrence of tamoxifen-treated, node-negative breast cancer. N Engl J Med 2004;351:2817-26. [Crossref] [PubMed]
- Varga Z, Sinn P, Seidman AD. Summary of head-to-head comparisons of patient risk classifications by the 21-gene Recurrence Score(R) (RS) assay and other genomic assays for early breast cancer. Int J Cancer 2019;145:882-93. [Crossref] [PubMed]
- Parker JS, Mullins M, Cheang MC, et al. Supervised risk predictor of breast cancer based on intrinsic subtypes. J Clin Oncol 2009;27:1160-7. [Crossref] [PubMed]
- Dai X, Li T, Bai Z, et al. Breast cancer intrinsic subtype classification, clinical use and future trends. Am J Cancer Res 2015;5:2929-43. [PubMed]
- Hanahan D, Weinberg RA. Hallmarks of cancer: the next generation. Cell 2011;144:646-74. [Crossref] [PubMed]
- Vander Heiden MG, DeBerardinis RJ. Understanding the Intersections between Metabolism and Cancer Biology. Cell 2017;168:657-69. [Crossref] [PubMed]
- Sivanand S, Vander Heiden MG. Emerging Roles for Branched-Chain Amino Acid Metabolism in Cancer. Cancer Cell 2020;37:147-56. [Crossref] [PubMed]
- Dang CV. Links between metabolism and cancer. Genes Dev 2012;26:877-90. [Crossref] [PubMed]
- White E. Exploiting the bad eating habits of Ras-driven cancers. Genes Dev 2013;27:2065-71. [Crossref] [PubMed]
- Howell JJ, Ricoult SJ, Ben-Sahra I, et al. A growing role for mTOR in promoting anabolic metabolism. Biochem Soc Trans 2013;41:906-12. [Crossref] [PubMed]
- Kim IK, Rao G, Zhao X, et al. Mutant GTF2I induces cell transformation and metabolic alterations in thymic epithelial cells. Cell Death Differ 2020;27:2263-79. [Crossref] [PubMed]
- Fridman A, Saha A, Chan A, et al. Cell cycle regulation of purine synthesis by phosphoribosyl pyrophosphate and inorganic phosphate. Biochem J 2013;454:91-9. [Crossref] [PubMed]
- Bahreyni A, Samani SS, Rahmani F, et al. Role of adenosine signaling in the pathogenesis of breast cancer. J Cell Physiol 2018;233:1836-43. [Crossref] [PubMed]
- Wang X, Yang K, Wu Q, et al. Targeting pyrimidine synthesis accentuates molecular therapy response in glioblastoma stem cells. Sci Transl Med 2019;11:eaau4972. [Crossref] [PubMed]
- Vander Heiden MG, Cantley LC, Thompson CB. Understanding the Warburg effect: the metabolic requirements of cell proliferation. Science 2009;324:1029-33. [Crossref] [PubMed]
- Tibshirani R. The lasso method for variable selection in the Cox model. Stat Med 1997;16:385-95. [Crossref] [PubMed]
- Zhou ZR, Wang WW, Li Y, et al. In-depth mining of clinical data: the construction of clinical prediction model with R. Ann Transl Med 2019;7:796. [Crossref] [PubMed]
- Giuliano AE, Connolly JL, Edge SB, et al. Breast Cancer-Major changes in the American Joint Committee on Cancer eighth edition cancer staging manual. CA Cancer J Clin 2017;67:290-303.
- Sotiriou C, Pusztai L. Gene-expression signatures in breast cancer. N Engl J Med 2009;360:790-800. [Crossref] [PubMed]
- Beitsch PD, Whitworth PW, Hughes K, et al. Underdiagnosis of Hereditary Breast Cancer: Are Genetic Testing Guidelines a Tool or an Obstacle? J Clin Oncol 2019;37:453-60. [Crossref] [PubMed]
- Iwamoto T, Pusztai L. Predicting prognosis of breast cancer with gene signatures: are we lost in a sea of data? Genome Med 2010;2:81. [Crossref] [PubMed]
- Boroughs LK, DeBerardinis RJ. Metabolic pathways promoting cancer cell survival and growth. Nat Cell Biol 2015;17:351-9. [Crossref] [PubMed]
- Pavlova NN, Thompson CB. The Emerging Hallmarks of Cancer Metabolism. Cell Metab 2016;23:27-47. [Crossref] [PubMed]
- Bhutia YD, Babu E, Ramachandran S, et al. Amino Acid transporters in cancer and their relevance to "glutamine addiction": novel targets for the design of a new class of anticancer drugs. Cancer Res 2015;75:1782-8. [Crossref] [PubMed]
- Kanarek N, Keys HR, Cantor JR, et al. Histidine catabolism is a major determinant of methotrexate sensitivity. Nature 2018;559:632-6. [Crossref] [PubMed]
- Wise DR, Thompson CB. Glutamine addiction: a new therapeutic target in cancer. Trends Biochem Sci 2010;35:427-33. [Crossref] [PubMed]
- Locasale JW. Serine, glycine and one-carbon units: cancer metabolism in full circle. Nat Rev Cancer 2013;13:572-83. [Crossref] [PubMed]
- Elia I, Broekaert D, Christen S, et al. Proline metabolism supports metastasis formation and could be inhibited to selectively target metastasizing cancer cells. Nat Commun 2017;8:15267. [Crossref] [PubMed]
- Liu W, Hancock CN, Fischer JW, et al. Proline biosynthesis augments tumor cell growth and aerobic glycolysis: involvement of pyridine nucleotides. Sci Rep 2015;5:17206. [Crossref] [PubMed]
- Bansal A, Simon MC. Glutathione metabolism in cancer progression and treatment resistance. J Cell Biol 2018;217:2291-8. [Crossref] [PubMed]
- Tarnopolsky MA. Gender differences in metabolism; nutrition and supplements. J Sci Med Sport 2000;3:287-98. [Crossref] [PubMed]
- Ushkaryov YA, Lelianova V, Vysokov NV. Catching Latrophilin With Lasso: A Universal Mechanism for Axonal Attraction and Synapse Formation. Front Neurosci 2019;13:257. [Crossref] [PubMed]
- Ternes N, Rotolo F, Michiels S. Empirical extensions of the lasso penalty to reduce the false discovery rate in high-dimensional Cox regression models. Stat Med 2016;35:2561-73. [Crossref] [PubMed]
- Wu M, Li X, Zhang T, et al. Identification of a Nine-Gene Signature and Establishment of a Prognostic Nomogram Predicting Overall Survival of Pancreatic Cancer. Front Oncol 2019;9:996. [Crossref] [PubMed]
- Liu GM, Xie WX, Zhang CY, et al. Identification of a four-gene metabolic signature predicting overall survival for hepatocellular carcinoma. J Cell Physiol 2020;235:1624-36. [Crossref] [PubMed]
- Iasonos A, Schrag D, Raj GV, et al. How to build and interpret a nomogram for cancer prognosis. J Clin Oncol 2008;26:1364-70. [Crossref] [PubMed]
(English Language Editor: A. Kassem; Quality Control Editor: J. Gray)