The shortage of hospital beds for COVID-19 and non-COVID-19 patients during the lockdown of Wuhan, China
Introduction
An ongoing pandemic of coronavirus disease 2019 (COVID-19), caused by the severe acute respiratory syndrome coronavirus 2 (SARS-CoV-2), has spread throughout China and to over 200 countries since December 2019 (1). The rapid spread of COVID-19 in the early stage was largely facilitated by the traffic rush of the Lunar New Year holiday in China, when around 3 billion trips were expected from January 10 to February 18, 2020 (1). By January 24, 2020, 76 exported cases from Wuhan were found in 15 regions/cities of China and 6 overseas countries (2). In order to contain the COVID-19 outbreak, the Chinese government implemented a series of massive and unprecedented control measures (3).
The rapidly increased cases soon overburdened the healthcare system in Wuhan in the early stage of the outbreak. At the same time, suspension of non-emergency healthcare services and implementation of stringent traffic restrictions might have seriously delayed the hospitalization of non-COVID-19 patients, some of whom might have been in critical condition (4). Here non-COVID-19 patients refer to those who had acute or chronic diseases not directly caused by SARS-CoV-2 infection. Moreover, it was found that the lockdown could have increased anxiety and stress of local residents, thereby increasing their susceptibility to infection (5,6). In addition, many cases exported from Wuhan had been reported in other cities in China and overseas weeks before the lockdown, suggesting the need to evaluate the optimal timing of lockdown implementation. Some studies have evaluated the effects of the lockdown and social distancing measures on the COVID-19 outbreak in and outside of Wuhan (3,7,8), and one estimated the hospital bed shortage for COVID-19 patients in Wuhan (9). However, none have evaluated the healthcare demands of non-COVID-19 patients during the lockdown, when the healthcare system was overwhelmed by COVID-19 patients.
In this study, we used a mathematical model to simulate the epidemic curves of COVID-19 in Wuhan and 50 other cities in mainland China during the lockdown, after adjustment for temporal variation in reporting rates. We estimated the shortage of inpatient and ICU beds for patients with mild, severe and critical SARS-CoV-2 infection. In addition, we proposed a simple model based on the social media posts to estimate hospital bed shortages for non-COVID-19 patients. We present the study in accordance with the MDAR reporting checklist (available at http://dx.doi.org/10.21037/atm-20-5248).
Methods
Data sources
We retrieved daily numbers of laboratory-confirmed cases and deaths of SARS-CoV-2 infection in Wuhan, from the daily reports of the National Health Commission of China (http://www.nhc.gov.cn/) and published data during December 22, 2019 to February 11, 2020 (10). Considering that intra- and inter-city transmissions had occurred before the Wuhan lockdown, we also retrieved daily numbers of COVID-19 cases in Wuhan and 50 other cities in mainland China with more reported cases than other cities/regions by the end of January 2020. A map of these cities is shown in Figure S1. The daily numbers of hospital beds designated for COVID-19 cases, as well as the proportion of inpatient beds in isolation wards for severe patients and ICU beds for critical patients, were retrieved from the published data and the Wuhan Municipal Health Commission (WMHC) (9,11) (Figure S2). Detailed descriptions of data sources can be found in the Supplementary material. The dates cited hereafter in this paper were all in 2020.
Statistical analysis
We built a susceptible-exposed-infected-recovered (SEIR) model to simulate the inter-city transmission of SARS-CoV-2 viruses, considering of underreport and test delay in the early stage of the epidemic. We also incorporated into the model the implementation of individual and governmental control measures, the population inflow/outflow between Wuhan and 50 other cities in mainland China, and a higher transmission risk in public transportation. Details of the model’s structure, parameters, descriptions and ranges can be found in the Supplementary material and Table S1.
We assumed that the lockdown and traffic restrictions had reduced 99% of the population flow from and within Wuhan since January 23. The daily number of diagnosed cases of SARS-CoV-2 infection in China was assumed to follow a Poisson distribution. Underreport of COVID-19 cases in the early pandemic has been widely reported but it is nearly impossible to get the true reporting rates. We therefore estimated the reporting rates according to previous studies (12-16) and the public available data: 1.8% on January 3, 3.0% on January 18, 14.0% on January 23, 34.0% on February 8 and 35.3% on February 18 (Table 1). The daily reporting rates between these dates were interpolated by a linear regression.
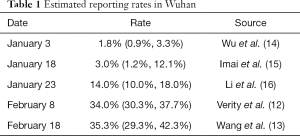
Full table
We built the second model to simulate the occupancy of hospital beds designated for COVID-19 cases, based on the clinical data of mild, severe and critical cases from previous clinical and epidemiological studies (Table S2). The daily hospital bed shortage for COVID-19 patients was calculated from the daily number of COVID-19 patients in the mild, severe and critical stages deducted by the total number of designated inpatient and ICU beds. Details of estimation for hospital bed shortages of non-COVID-19 patients are shown in Tables S3,S4.
During the lockdown, most hospitals in Wuhan suspended non-emergency services due to manpower shortages and allocation of inpatient hospital beds to COVID-19 patients. However, there are no official reports about the numbers of non-COVID-19 patients or hospital bed availability during the Wuhan lockdown. Previous work has demonstrated the feasibility of using social media posts and news to monitor and assess unexpected disease outbreaks (17,18). Here we adopt a simple model utilizing social media posts to estimate hospital bed shortages of non-COVID-19 patients. We first retrieved posts on the social media platform Weibo and then sort for posts relevant to online medical consultations, or complaints about hospital bed shortages and suspension of non-emergency healthcare services (19). We retrieved all relevant posts before February 29 and found that the earliest date for such posts was February 3. We then deleted repeated posts from the same Weibo users, and narrowed down the search to Weibo users located in Wuhan to increase specificity. After screening the contents, we retrieved the posts by COVID-19 patients (i.e., mentioned they had confirmed and suspected SARS-CoV-2 infection), and by non-COVID-19 patients (mentioned they did not have SARS-CoV-2 infection). Daily numbers of these posts are plotted in Figure S3. We assumed that COVID-19 and non-COVID-19 patients had the same probability of posting messages on social media. Hence, the daily ratio of hospital beds needed by COVID-19 patients to those needed by non-COVID-19 patients was estimated in proportion to the daily ratio of the posts by these two groups. Given the higher attention and perceived risk to COVID-19, we also repeated the estimation by assuming that COVID-19 patients were 2 or 10 times more likely to post messages. Because the earliest post about hospital bed shortages appeared on February 3 and most hospitals in Wuhan had gradually resume normal services by early March, we only calculated the hospital bed shortage for non-COVID-19 patients in February.
Simulation scenarios
The Wuhan lockdown began on January 23 and nearly all inter- and intra-city traffic was suspended. To study the impact of traffic restrictions, we simulated three additional scenarios: 80% and 50% decrease of traffic volume, and no traffic restriction (0% decrease) under different assumptions of reporting rates. In addition, the Wuhan lockdown was implemented in the exponential phase of this outbreak, therefore it would be interesting to examine the change in efficacy if the implementation date were 20 days earlier/later than the actual date (January 3 or February 12). We simulated the transmission of COVID-19 under these different scenarios, and subsequently calculated the cumulative number of cases by February 11 and the final epidemic size of the first wave. We quantified the relative efficiency of different traffic restriction levels and timing by comparing these estimates to the estimated real cumulative cases in Wuhan and 50 other Chinese cities. We also estimated the shortage of hospital beds in Wuhan under these scenarios.
The experience of the 2009 influenza pandemic suggested that infection control measures and human behaviour change could disrupt the rapid spread of novel viruses, but the gradual relaxation of these measures could result in multiple waves of epidemics over months or years (20-22). Similarly, during the lockdown, aggressive social distancing measures and traffic restrictions could have gradually waned as well. Hence, we also investigated the possibility of the second wave based on different assumptions of gradual relaxation of personal mitigation measures in Wuhan.
All data analyses were conducted using the R version 3.6.1. The datasets and codes used in this study can be found at https://github.com/Larryzza/The-shortage-of-hospital-beds-for-COVID-19-Wuhan.
IRB approval
The study was conducted in accordance with the Declaration of Helsinki (as revised in 2013). The IRB approval is exempted since this study used only the public available data.
Results
The model well fits the daily numbers of newly reported cases and cumulative numbers by February 11 in Wuhan (Figure 1). After adjustment for underreport and diagnosis delay, we estimated that there were a total of 88,083 [95% confidence interval (CI): 38,929, 139,796] symptomatic COVID-19 cases in Wuhan as of February 11. These numbers are much higher than the reported number of 19 601 laboratory-confirmed cases, suggesting that 77.7% (95% CI: 49.6%, 86.0%) of cases were unreported. Daily numbers of newly onset cases peaked on February 2 and February 3, and gradually declined afterwards. Correspondingly, the daily hospital bed shortages were estimated to have peaked on February 6, February 9 and February 11, with estimates of 43,960 (95% CI: 35,246, 52,929), 2,779 (95% CI: 1,395, 4,163) and 196 (95% CI: 143, 250) beds for mild, severe and critical cases, respectively (Figure 2). The daily average shortage of hospital beds for mild, severe and critical cases was 41,241 (95% CI: 32,852, 49,797), 1,498 (95% CI: 553, 2,563) and 160 (95% CI: 108, 215) in early February, 18,238 (95% CI: 13,824, 23,100), 1,553 (95% CI: 451, 3,072) and 143 (95% CI: 100, 187) in mid-February, and 0 (95% CI: 0, 92), 0 (95% CI: 0, 0) and 39 (95% CI: 16, 69) in late February (Table S5).
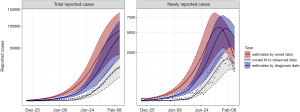
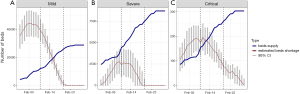
We retrieved a total of 1,215 Weibo posts relevant to hospital bed demands for COVID-19 and non-COVID-19 patients. More than 90% of the posts were about the hospital bed needs of COVID-19 cases, with the first found on February 3. The daily numbers of posts about COVID-19 peaked on February 5 and gradually decreased until February 29 (Figure S3). This pattern coincides with the gradual increase of designated hospital beds and reveals that healthcare services in Wuhan nearly broke down in early February. A small number of posts about the hospital bed needs of non-COVID-19 patients were retrieved, and the maximal daily number was observed on February 18, nearly 4 weeks after the start of the lockdown.
From the model, we estimated that the proportion of mild, severe and critical cases in COVID-19 patients who posted social media messages of seeking help was 1.6% (95% CI: 1.4%, 2.2%) and 0.09% (95% CI: 0.01%, 0.1%), and 20% (95% CI: 10%, 30%), respectively. On average, the daily numbers of hospital bed shortages for mild cases of non-COVID-19 patients were 919 (95% CI: 63, 5,087), 1,838 (95% CI: 127, 10,177) and 9,187 (95% CI: 639, 50,899) during February 3 to 10. The numbers slightly increased during February 11 to 20, and decreased during February 21 to 29 (Table S6). The corresponding numbers of severe and critical non-COVID-19 cases were 13 (95% CI: 1, 336), 26 (95% CI: 3, 675) and 126 (95% CI: 15, 3,408) in early February, doubled in mid-February and then decreased to the similar level of early February (Table S6).
The estimates of the total cases and relative changes under 5 different traffic restriction scenarios in Wuhan are shown in Figure S4. Compared to the real situation (lockdown since January 23 and 99% traffic restriction), if there were no traffic restriction, by April 8 the number of COVID-19 cases would have increased by 9,381 (95% CI: 4,064, 15,889) in Wuhan, 5,283 (95% CI: 2,396, 9,655) in the other 50 cities, and 14,663 (95% CI: 6,460, 25,544) in all cities combined, respectively (Table 2). The increased numbers correspond to 11.1%, 26.4% and 13.9% of the actual observed numbers. Earlier implementation of traffic restriction on January 3 would have increased the total number of cases in Wuhan to 12,848 (95% CI: 8,115, 19,524) (14.6% of the actual cases), but dramatically reduced cases in other cities by 14,476 (95% CI: 7,142, 26,512) (70.3% of the actual cases). The total number of cases in all 51 cities would have decreased by 1,628 (95% CI: −3,633, 8,044) (1.4% of the actual number) by February 11. If there were later implementation of restriction on February 12, 9,150 (95% CI: 3,988, 15,376) more cases would have been observed in Wuhan, 5,275 (95% CI: 2,397, 9,645) in other cities, and 14,425 (95% CI: 6,386, 25,022) in all 51 cities by April 8.
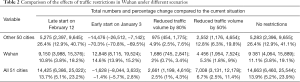
Full table
Compared to the real scenario, the hospital bed shortage for mild, severe and critical COVID-19 patients would have increased under all the five simulated scenarios (Figure 3 and Table S5). The greatest increase would have occurred if the lockdown and traffic restrictions were enforced on January 3, or if there were neither traffic restrictions nor lockdown. Earlier implementation would have increased the shortage of hospital beds earlier than February 21, whereas completely no lockdown would have delayed the ICU shortage peak, compared to the real scenario.
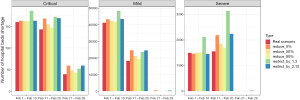
We simulated the second wave based on the assumption that social distancing measures had gradually waned by the daily ratio of 1/30, 1/40 after February 11. The larger the waning weight, the earlier the second wave would have started. The onset date of the second wave would have been as early as in June if social distancing measures were relaxed soon after the end of the lockdown (Figure S5). We estimated that the second wave would have reached the maximum (2,894, 95% CI: 523, 3,635) that were nearly half of the peak numbers in the first wave (5,812, 95% CI: 2,531, 7,911, when the waning weight was assumed 1/30). But the second wave would have lasted longer than the first wave. A shortage of ICU beds would have occurred in critical cases under the scenario that social distancing lasted for 30 and 40 days (Table S7). Overall, if the same number of hospital beds were designated to COVID-19 as in the first wave, it would have met the needs of mild and severe cases in the second wave.
Discussion
In response to the COVID-19 outbreak, Wuhan had been locked down for 67 days from January 23 to April 8. In line with other studies, we demonstrated the Wuhan lockdown and stringent control measures had effectively mitigated the regional and national COVID-19 epidemics (3,23-25). In this study, we did a further investigation on the shortage of inpatient and ICU beds for COVID-19 patients across the spectra of mild, severe and critical cases. We also adopted a simple approach to estimate non-COVID-19 patients hospital bed shortage by retrieving social media posts of seeking help with medical consultations and hospital beds.
The health authority in Wuhan recently updated the death toll of COVID-19, bringing the number of cases during the first wave to 50,333 and the death toll to 3,869, which were 325 and 1,290 more than the previously reported numbers (26). Our model estimates demonstrated that the serious underreport of the deaths had most likely occurred in February, when the reporting rate was low and there was a great gap of hospital beds for severe and critical cases (Figure 2 and Figure S6). According to our estimates, as of April 8, when Wuhan reopened, there had been a total of 4,037 (95% CI: 3,465, 4,642) deaths, which is largely consistent with the total number of reported deaths after adjustment for temporal change in reporting rates. In addition, we estimated a final epidemic size of 95,336 (95% CI: 41,762, 158,606) infected cases, twice as high as the officially reported number. This result is consistent with the underreporting rate estimated by Wang et al. (59% as of February 18) (13). The daily shortage of ICU beds for critical COVID-19 patients remained at the level of 100 beds during the entire first wave, although the shortage was nearly resolved for severe cases in the late stage. This could explain why such a large number of deaths were not captured in surveillance.
Previous studies have suggested a high risk of virus transmission while traveling in buses, trains and airplanes, due to increased direct and indirect human-to-human contact (27,28). Compared to the real situation of city lockdown and stringent traffic restrictions, no traffic restrictions could have increased the total number of cases by 11.1% (95% CI: 3.8%, 19.1%) and 26.4% (95% CI: 12.9%, 41.1%) in Wuhan and 50 other mainland Chinese cities, respectively. This is not surprising, as in the early stages of the COVID-19 epidemic, most of the cases in other cities were imported from Wuhan (29). Our results support that traffic restrictions in Wuhan were effective in containing the spread of COVID-19 to other cities, despite that the outbreak within Wuhan slightly escalated soon after the restriction. This immediate surge could have been explained by two reasons. First, many people rushed to the designated hospitals and waited for a few hours to get throat swabs for lab tests. This could have increased the transmissions within the city. Second, all the infected cases in their latent period were forced to stay in the city due to traffic restrictions, leading to more sources of transmission. However, it is of note that complete suspension of public and private traffic creates problems in daily life and delays access to critical and routine healthcare services. This is particularly of concern for vulnerable populations, such as the elderly and those with disabilities or chronic conditions who need regular medication. To date, a few countries and regions outside of China have been locked down in order to mitigate the COVID-19 outbreak, and most have adopted voluntary home quarantines. Hence, we investigated the scenarios of partial traffic restrictions (80% and 50% reduction), which would have only slightly increased the final epidemic size and the hospital bed shortage compared to the complete lockdown. Taken together, partial traffic restrictions might have achieved a better balance between the social and medical needs of different populations.
Our results demonstrate that the timing of the lockdown had a greater effect than the intensity of the traffic restrictions. It is interesting to find that January 23 now appears to have been a better time point to lock down Wuhan than January 3 and February 12, according to our estimates. The implementation of traffic restrictions as early as January 3 would have been more effective in reducing the regional spread of the COVID-19 epidemic, but significantly increased the case numbers inside Wuhan. This would have overwhelmed the healthcare system in Wuhan to a greater extent. Ai et al. (30) estimated that 1,420 (95% CI: 1,059, 1,833) cases would have been prevented if the city lockdown policy had been implemented 2 days earlier. Nevertheless, our simulation shows early implementation on January 3 would also have increased case numbers in Wuhan by 14.6% (95% CI: 13.9%, 15.2%) of total cases estimated under the real scenario (as of April 8). This would have placed even more unbearable burden on the local healthcare system. Our results further suggest that an earlier traffic restriction in Wuhan would have not changed the local and regional epidemics, but later implementation on February 12 would probably have significantly increased the final size of the epidemic by 13.7% (95% CI: 5.1%, 23.2%) as of April 8, 2020.
The daily number of posts on social media that sought for online medical consultations or complained about the shortage of hospital beds or suspension of clinics, had reached a peak in early February. This pattern was similar to that of the hospital bed shortage for COVID-19 patients in Wuhan. It is of note that our estimates of hospital bed shortages for non-COVID-19 patients might need a cautious interpretation. We assumed that the probability of sending posts to seek for help via social media in non-COVID-19 patients was the same as that in COVID-19 cases, which might not hold due to different risk perceptions and media attention to the COVID-19 outbreak and chronic diseases. Also, high-risk populations such as the elderly might not be familiar with social media. Nevertheless, we observed that a large proportion of posts were posted by relatives and friends for older people. As suggested by previous studies (31,32), crowdsourcing from online sources and social media could be an alternative approach in the absence of reliable and timely data.
Conclusions
The overwhelmed healthcare system in Wuhan might have delayed the provision of health care to both COVID-19 and non-COVID-19 patients during the lockdown. However, earlier or later lockdowns of Wuhan would have caused more serious shortages. Although the city lockdown is an effective mitigation measure to control the regional and national COVID-19 epidemics, such stringent control measures require considerable manpower and economic costs, making them unlikely to be sustainable or affordable in many cities or countries. As we demonstrated in this study, the suspension of routine healthcare services might have seriously delayed the hospitalization of patients without SARS-CoV-2 infection. The decision to lock down a city in future should ensure equality of access to healthcare services among vulnerable populations, with careful consideration of societal and economic costs.
Acknowledgments
Funding: DH and LY were supported by the Alibaba (China) Co. Ltd. Collaborative Research Fund. PC was supported by the National Natural Science Foundation of China.
Footnote
Reporting Checklist: The authors have completed the MDAR reporting checklist. Available at http://dx.doi.org/10.21037/atm-20-5248
Peer Review File: Available at http://dx.doi.org/10.21037/atm-20-5248
Conflicts of Interest: All authors have completed the ICMJE uniform disclosure form (Available at http://dx.doi.org/10.21037/atm-20-5248). DH and LY report that both are supported by the Alibaba (China) Collaboration Fund. PC reports grants from National Natural Science Foundation of China during the conduct of the study. The other authors have no conflicts of interest to declare.
Ethical Statement: The authors are accountable for all aspects of the work in ensuring that questions related to the accuracy or integrity of any part of the work are appropriately investigated and resolved. The study was conducted in accordance with the Declaration of Helsinki (as revised in 2013). The IRB approval is exempted since this study used only the public available data.
Open Access Statement: This is an Open Access article distributed in accordance with the Creative Commons Attribution-NonCommercial-NoDerivs 4.0 International License (CC BY-NC-ND 4.0), which permits the non-commercial replication and distribution of the article with the strict proviso that no changes or edits are made and the original work is properly cited (including links to both the formal publication through the relevant DOI and the license). See: https://creativecommons.org/licenses/by-nc-nd/4.0/.
References
- Wang C, Horby PW, Hayden FG, et al. A novel coronavirus outbreak of global health concern. Lancet 2020;395:470-3. [Crossref] [PubMed]
- World Health Organization. Novel Coronavirus(2019-nCoV) Situation Report – 4. Available online: 24 January 2020.https://www.who.int/docs/default-source/coronaviruse/situation-reports/20200124-sitrep-4-2019-ncov.pdf?sfvrsn=9272d086_8
- Leung K, Wu JT, Liu D, et al. First wave COVID-19 transmissibility and severity in China outside Hubei after control measures and second wave scenario planning: a modelling impact assessment. Lancet 2020;395:1382-93. [Crossref] [PubMed]
- Hui DS, I, Azhar E, Madani TA, et al. The continuing 2019-nCoV epidemic threat of novel coronaviruses to global health - The latest 2019 novel coronavirus outbreak in Wuhan, China. Int J Infect Dis 2020;91:264-6. [Crossref] [PubMed]
- Ni MY, Yang L, Leung CMC, et al. Mental Health, Risk Factors, and Social Media Use During the COVID-19 Epidemic and Cordon Sanitaire Among the Community and Health Professionals in Wuhan, China: Cross-Sectional Survey. JMIR Ment Health 2020;7:e19009. [Crossref] [PubMed]
- Falagas ME, Karamanidou C, Kastoris AC, et al. Psychosocial factors and susceptibility to or outcome of acute respiratory tract infections. Int J Tuberc Lung Dis 2010;14:141-8. [PubMed]
- Prem K, Liu Y, Russell TW, et al. The effect of control strategies to reduce social mixing on outcomes of the COVID-19 epidemic in Wuhan, China: a modelling study. Lancet Public Health 2020;5:e261-70. [Crossref] [PubMed]
- Team TICC-R. Impact of non-pharmaceutical interventions (NPIs) to reduce COVID-19 mortality and healthcare demand 2020.
- Li J, Yuan P, Heffernan J, et al. Observation wards and control of the transmission of COVID-19 in Wuhan. Bull World Health Organ 2020. [Crossref] [PubMed]
- Zhu N, Zhang D, Wang W, et al. A Novel Coronavirus from Patients with Pneumonia in China, 2019. N Engl J Med 2020;382:727-33. [Crossref] [PubMed]
- Wuhan Municipal Health Commission (WMHC) [Available online: http://wjw.wuhan.gov.cn/
- Verity R, Okell LC, Dorigatti I, et al. Estimates of the severity of coronavirus disease 2019: a model-based analysis. Lancet Infect Dis 2020;20:669-77. [Crossref] [PubMed]
- Wang C, Liu L, Hao X, et al. Evolving Epidemiology and Impact of Non-pharmaceutical Interventions on the Outbreak of Coronavirus Disease 2019 in Wuhan, China. medRxiv 2020:2020.03.03.20030593.
- Wu JT, Leung K, Bushman M, et al. Estimating clinical severity of COVID-19 from the transmission dynamics in Wuhan, China. Nat Med 2020;26:506-10. [Crossref] [PubMed]
- Imai N, Dorigatti I, Cori A, et al. Report 2: Estimating the potential total number of novel Coronavirus cases in Wuhan City, China 2020.
- Li R, Pei S, Chen B, et al. Substantial undocumented infection facilitates the rapid dissemination of novel coronavirus (SARS-CoV-2). Science 2020;368:489. [Crossref] [PubMed]
- Shan S, Zhao F, Wei Y, et al. Disaster management 2.0: A real-time disaster damage assessment model based on mobile social media data—A case study of Weibo (Chinese Twitter). Safety Sci 2019;115:393-413. [Crossref]
- Chunara R, Andrews JR, Brownstein JS. Social and news media enable estimation of epidemiological patterns early in the 2010 Haitian cholera outbreak. Am J Trop Med Hyg 2012;86:39-45. [Crossref] [PubMed]
- wuhancrisis, hanfu. Help seeking posts on Weibo platform: GitHub repository; 2020 Available online: https://github.com/wuhancrisis/wuhancrisis.github.io
- Poletti P, Ajelli M, Merler S. The effect of risk perception on the 2009 H1N1 pandemic influenza dynamics. PLoS One 2011;6:e16460. [Crossref] [PubMed]
- Wessel L, Hua Y, Wu J, et al. Public health interventions for epidemics: implications for multiple infection waves. BMC Public Health 2011;11 Suppl 1:S2. [Crossref] [PubMed]
- He D, Dushoff J, Day T, et al. Inferring the causes of the three waves of the 1918 influenza pandemic in England and Wales. Proc Biol Sci 2013;280:20131345. [Crossref] [PubMed]
- Kraemer MUG, Yang CH, Gutierrez B, et al. The effect of human mobility and control measures on the COVID-19 epidemic in China. Science 2020;368:493-7. [Crossref] [PubMed]
- Long Q, Deng H, Chen J, et al. Antibody responses to SARS-CoV-2 in COVID-19 patients: the perspective application of serological tests in clinical practice. medRxiv 2020.
- Lin Q, Zhao S, Gao D, et al. A conceptual model for the coronavirus disease 2019 (COVID-19) outbreak in Wuhan, China with individual reaction and governmental action. Int J Infect Dis 2020;93:211-6. [Crossref] [PubMed]
- France-Presse A. Coronavirus: others will revise death tolls like China, World Health Organisation says. South China Morning Post 2020 18 Apr, 2020.
- Goeijenbier M, van Genderen P, Ward BJ, et al. Travellers and influenza: risks and prevention. J Travel Med 2017;24:taw078. [Crossref] [PubMed]
- Foxwell AR, Roberts L, Lokuge K, et al. Transmission of influenza on international flights, may 2009. Emerg Infect Dis 2011;17:1188-94. [Crossref] [PubMed]
- Du Z, Wang L, Cauchemez S, et al. Risk for Transportation of 2019 Novel Coronavirus (COVID-19) from Wuhan to Cities in China. medRxiv 2020:2020.01.28.20019299.
- Ai S, Zhu G, Tian F, et al. Population movement, city closure and spatial transmission of the 2019-nCoV infection in China. medRxiv 2020:2020.02.04.20020339.
- Leung GM, Leung K. Crowdsourcing data to mitigate epidemics. Lancet Digit Health 2020;2:e156-7. [Crossref] [PubMed]
- Sun K, Chen J, Viboud C. Early epidemiological analysis of the coronavirus disease 2019 outbreak based on crowdsourced data: a population-level observational study. Lancet Digit Health 2020;2:e201-8. [Crossref] [PubMed]