Aberrant amplitude low-frequency fluctuation (ALFF) and regional homogeneity (ReHo) in generalized anxiety disorder (GAD) and their roles in predicting treatment remission
Introduction
Of the psychiatric disorders, anxiety disorders are the most common, with its prevalence being as high as 7.3% (4.8% to 10.9%) (1). Furthermore, the prevalence of anxiety disorders varies greatly across different countries, and within countries depending on the study conducted. This variability is particularly pronounced in generalized anxiety disorder (GAD); for example, the lifetime prevalence of GAD according to the Diagnostic and Statistical Manual of Mental Disorders (DSM)-IV criteria ranges from 0.1% in Nigeria to 6.2% in New Zealand (2), and the lifetime prevalence of GAD in China ranges from 0.3% (3) to 4.1–4.6% (4). GAD is a mental disorder characterized by comprehensive anxiety, unreasonable worries, nervous and high muscle tension, and autonomic hyperactivity (5). Excessive, uncontrollable worries concerning comprehensive areas with no respective stimuli or an inappropriate sensation of potential risk is the most important diagnostic item of GAD (6). Compared to other mental diseases such as mood disorders, the diagnostic criterion of GAD is considerably ambiguous which makes the diagnosis and prognosis of GAD challenging. Thus, the exploration for, and definition of objective criterion or biomarkers in identifying disease risk and treatment response in GAD has become an urgent need for psychiatrists.
In order resolve this issue, considerable work has been done to uncover the pathophysiological mechanisms of GAD, including gene studies, protein biomarker studies, as well as functional and structural neuroimaging studies (7-10). As a noninvasive method, functional magnetic resonance imaging (fMRI) utilizes intensity changes in a type of magnetic resonance (MR) signal to track hemodynamic changes in the brain. The hemodynamic changes can be reflected with blood oxygenation level-dependent (BOLD) signals, and BOLD can be utilized as a proxy measure for neuronal function (including the degree of these activations and the synchrony of brain function across two or more regions) (11). fMRI has been applied to reflect the functional brain abnormalities in GAD, while resting-state (Rs)-fMRI has been applied to assess functional abnormalities in individuals’ resting state (12).
Regional aberrant amplitude low-frequency fluctuation (ALFF) is calculated as the averaged square root at each frequency (0.01–0.08 Hz) of the power spectrum at each voxel. It represents the spontaneous neuronal activity of the regional brain area (11). Compared to healthy controls (HC), GAD patients have been found to show higher ALFF in the bilateral dorsomedial prefrontal and dorsolateral prefrontal cortex, along with higher ALFF in the left precuneus and posterior cingulate cortex (13).
Finally, regional homogeneity (ReHo) can reflect the local synchronization of the time courses of the nearest neighboring voxels (usually 27 voxels) based on a voxel-wise measure. ReHo can thus indicate the changes of temporal neuronal activity in specific regional areas. The ReHo method has been used to investigate neural activity change in depression and GAD (11,14).
Thus far, however, few studies have combined ALFF and ReHo methods to examine GAD, and whether or not ALFF combined with ReHo can predict the treatment remission, is still unknown. We therefore conducted this study to examine the abnormal ALFF and ReHo brain regions between a GAD patient group and an HC group in order to identify the potential brain regions related to the pathophysiology of GAD and evaluate their ability to predict treatment remission. We present the following article in accordance with the MDAR reporting checklist (available at http://dx.doi.org/10.21037/atm-20-6598).
Methods
Participants
All participants were patients who met the diagnostic criteria for GAD according to DSM-IV (15), and all participants were recruited from Huzhou Third Hospital between June 2015 and June 2017. The inclusion criteria for patients were the following: (I) first episode and no treatment history; (II) age range, 18–50 years; (III) Han nationality and right-handed; (IV) education level over 9 years; (V) Hamilton Anxiety Rating Scale (HAM-A) score ≥17 and 17-item Hamilton Depression Rating Scale (HAM-D17) ≤14 (5). The exclusion criteria were as follows: (I) any other mental illness requiring medical intervention including schizophrenia, bipolar disorder, major depression disorder, etc.; (II) MR scanning contraindications; (III) structural abnormalities after routine brain MR scanning; (IV) received any psychotropic drug or psychotherapy within 2 weeks before admission. We screened 120 cases, 78 cases met the criteria of this study, and 40 GAD cases participated in this study. We recruited 32 HCs who were well matched for age and gender from the local community, and all subjects were assessed by a single psychiatrist using the Structural Clinical Interview for DSM-IV Disorders (SCID). The HAM-A scores of all HC subjects were <7. Those subjects with any psychiatric disorder history were excluded. The protocol was permitted by the Ethics Committee of Huzhou Third Hospital. The protocol was permitted by the Ethics Committee of Huzhou Third Hospital (Ethical approval number: 2018-010,036). After a complete description of the research scheme, written informed consent was obtained from all participants before the study. All procedures performed in this study involving human participants were in accordance with the Declaration of Helsinki (as revised in 2013).
Treatment
GAD patients received anti-anxiety treatment with escitalopram or venlafaxine for 8 weeks. The drug choice and change was determined by doctors based on the symptomatic characteristics and the side effects in the treatment. In all 30 GAD cases, 23 cases were treated by escitalopram while 7cases were treated by venlafaxine. The dosing of drugs was flexible and conducted according to the anxiety symptom severity and the side effects assessed by the clinician. Other anxiolytics and sedative-hypnotics were permitted.
The average dosage of escitalopram was 16.2±3.6 mg/d, and the average dosage of venlafaxine was 186.2±23.7 mg/d after 2 weeks of treatment and lasted for 8 weeks.
Clinical assessment
The severity and efficacy of the GAD symptoms was rated with HAM-A by the same trained rater throughout the treatment. HAM-A score on week 8 was applied as the primary efficacy endpoint. Remission was defined as HAM-A ≤7 after 8 weeks of treatment. GAD patients were classified as remitters if their HAM-A score ≤7; otherwise, they were classified as nonremitters. The raters were blind to the purpose of the rating scales. To maintain the blindness of this study, an experienced research coordinator was trained to manage the schedules and all data in this study.
MRI data acquisition
Rs-fMRI scan was performed using a GE Signa HDx 1.5T MRI scanner (GE Healthcare, Chicago, IL, USA) with an eight-channel head coil by a trained operator. All participants were asked to keep their eyes opened and to relax without falling asleep or thinking about specific things. To minimize the head motion in the course, a special pad was used. Resting-state MR images (BOLD) were acquired using the follow parameters: repetition time (TR)/echo time (TE) =2,000/40 ms; flip angle =83°; resolution =64×64; field of view (FOV) =240×240 mm2; voxel size =3×3×3 mm3; slice thickness =3.0 mm; interspace =1.0 mm; slices =28; duration =8 min 04 s. All subjects received a conventional high-resolution structural T1 scan for spatial normalization and localization using the follow parameters: flip angle =20°; resolution =250×250; thickness =1.2 mm; no interspace; slices =128; duration =4 min 04 s.
MRI data preprocessing
DEPASF 4.3 Advanced Edition software (http://rfmri.org/dpabi) based on Matlab 2013b was used to conduct the MRI data preprocessing and statistical analyses. First, Digital Imaging and Communications in Medicine (DICOM MRI) data files were converted into neuroimaging informatics technology initiative (NIFTI) images, and then the first 10 volumes were discarded as being the subjects’ adaptation to the scan. For slice timing and head motion correction, the remaining MRI images were slice-time–corrected and realigned to the first volume. For spatial normalization, realigned MRI images were spatially normalized according to each subject’s T1 structural images, and then all images were resampled to 3-mm isotropic voxels. Smoothing was completed using a 6-mm full-width-half-maximum (FWHM) Gaussian kernel, and the resampled images were spatially smoothed in order decrease spatial noise. The head motion of all subjects in the scanning was checked to identify whether the translation exceeded 2 mm or if the rotation exceeded 2°, if either occurred, the data were discarded for quality control. We found no significant differences of head motion and rotation between GAD patients and HC participants.
ALFF and ReHo value calculation
ALFF and ReHo analysis was performed using DEPASF4.3 Advanced Edition (http://rfmri.org/DPARSF). The ALFF value was calculated as follows: each voxel of the time series was in the power spectrum range of 0.01 to 0.08 Hz, and the average value of the square root was calculated by fast Fourier transform to calculate the ALFF; after subtracting the average value and dividing by the whole brain voxel deviation, the ALFF value was converted to z-distribution to achieve standardization; finally, the result obtained was filtered through a 0.01–0.08 Hz bandpass filter for band-pass filtering.
The ReHo value was calculated as follows: the spatially standardized data were passed through a 0.01–0.08 Hz bandpass filter to reduce low-frequency drift and high-frequency noise; Kendall’s Coefficient of Concordance (KCC) was then applied to calculate the similarity between a single voxel and the surrounding 27 voxels based on ReHo to measure the similarity of the time series in the functional cluster. Then, the individual’s ReHo image was divided by the average ReHo value of the whole brain of all the participants in this group. Finally, the ReHo brain map was spatially smoothed with a smooth core of 6 mm.
Statistical analysis
The study endpoint for all analyses was week 8. The demographic and clinical data were processed with SPSS 19.0 for Windows. Data were recorded as mean ± standard deviation (SD). All variables showed normal and near-normal distribution as checked by the Kolmogorov-Smirnov test. Student’s t-tests were used to compare the difference in demographic and clinical data. An analysis of two-sample t-test based on DEPASF 4.3 Advanced Edition Statistical Analysis (http://rfmri.org/DPARSF) was performed to identify brain areas which showed significant differences between the GAD and HC groups, and between remitters and nonremitters, with age and gender as covariates. The results were corrected with a permutation correction (voxel-level, P<0.01; cluster size, >20 voxels; number, 5,000; no acceleration; two tailed). The two-sample t-test on the voxel-based analysis were used to compare ALFF and ReHo maps between GAD and HC as well as between remitters and nonremitters (see details above). Pearson’s correlation analysis was performed to explore the relationship between baseline HAMA scores/illness duration and mean ALFF/ReHo values (see details above). A significant difference was defined as a two-tailed P value <0.05.
Results
Demographic information of the GAD vs. HC groups and remitters vs. nonremitters
In all 40 GAD patients and 32 HC who participated in the study, 10 GAD patients and 2 HC were excluded from the statistical analysis: 4 GAD patients and 2 HC for excessive head motion in quality control (translation >2 mm or rotation >2°) and 6 GAD patients for exceeding the age limit (age not matched). The final analysis consisted of 30 GAD patients (age, 18–50) and 30 HC (age, 20–48), and after 8 weeks of treatment, there were 9 remitters and 21 non-remitters in the GAD group.
There was no significant difference in the male-to-female ratio or in age between the GAD and HC groups. There was no obvious difference in gender (male-to-female ratio), age, illness duration, and education status between remitters and non-remitters. The baseline HAM-A/HAMD scores showed no significant difference, but the 8-week HAM-A/HAMD scores in the remitters were lower than those in the nonremitters. The demographic data and main clinical characteristics of all subjects are listed in Tables 1 and 2.

Full table
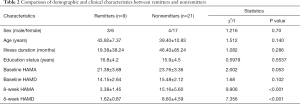
Full table
ALFF and ReHo values between the GAD and HC groups
ALFF values of the GAD and HC groups
The ALFF values in GAD patients in the right postcentral gyrus, right supramarginal cortex, and right precentral gyrus were lower than those of the HC group (P<0.05, permutation corrected) (Figure 1 and Table 3).
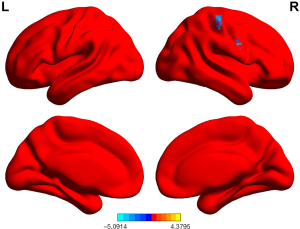
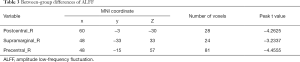
Full table
ReHo values of the GAD and HC groups
The ReHo values in the GAD group in the right precentral gyrus, right postcentral gyrus, and left precentral gyrus were lower than those in the HC group. The ReHo values in the GAD group for the left posterior cingulate cortex, left angular gyrus, and left precuneus were higher than those in the HC group (P<0.05, permutation corrected) (Figure 2 and Table 4).
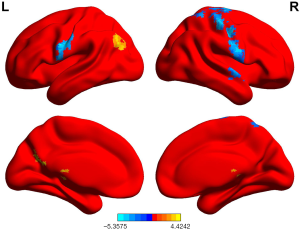
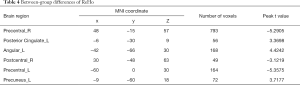
Full table
Correlations between ALFF and ReHo values and clinical features (baseline HAMA and illness duration) in brain regions exhibiting significant difference
ALFF was associated with baseline HAMA
The ALFF value in the left postcentral gyrus was negatively correlated with baseline HAMA, while that of the right frontal cortex was positively correlated with baseline HAMA (P<0.05, permutation corrected) (Table 5).

Full table
ReHo was associated with baseline HAMA
The ReHo value in right fusiform cortex, left postcentral gyrus, and right rolandic operculum cortex was negatively correlated with baseline HAMA, while that of the right angular gyrus, right frontal-mid cortex was positively correlated with baseline HAMA (P<0.05, permutation corrected) (Table 6).
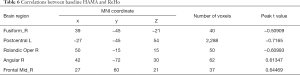
Full table
ALFF was associated with illness duration
The ALFF value in the right frontal-superior-orbital cortex and right frontal-medial-orbital cortex was positively correlated with illness duration (P<0.05, permutation corrected) (Table 7).

Full table
ReHo was associated with illness duration
The ReHo value of the left supplementary motor area cortex was negatively correlated with illness duration, while those in the right frontal-superior-orbital cortex, left hippocampus, and frontal lobe were positively correlated with illness duration (P<0.05, permutation corrected) (Table 8).
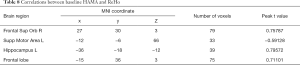
Full table
ALFF and ReHo values between remitters and nonremitters
ALFF value between remitters and non-remitters
ALFF values in GAD patients who achieved remission were higher in the left fusiform cortex, left hippocampus, right thalamus, and left rolandic operculum cortex than in those who did not achieve remission (P<0.05, permutation corrected) (Figure 3 and Table 9).
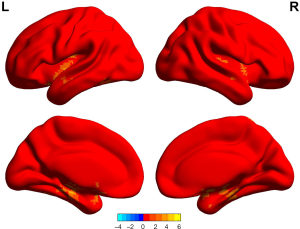
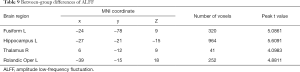
Full table
ReHo value between remitters and non-remitters
ReHo values in GAD patients who achieved remission were higher in the left angular gyrus and right postcentral gyrus than those who did not achieve remission (P<0.05, permutation corrected) (Figure 4 and Table 10).

Full table
Discussion
It is commonly accepted that ALFF can reflect regional brain activity (11,13) while ReHo can reflect local synchronization with nearby brain areas (11,14). Both ALFF and ReHo values have been used to reveal the neuropathology of psychiatric disease, including that of GAD (13,16). This study combined ALFF and ReHo values to investigate the characteristics of ALFF and ReHo in GAD patients in order to examine ALFF and ReHo as possible biomarkers for GAD, and to evaluate the ability of ALFF and ReHo values to predict treatment outcome in GAD patients. In this study, GAD patients showed several functional abnormalities in ALFF and ReHo. Compared to the HC, the GAD patients had lower ALFF values in the right postcentral gyrus and right precentral gyrus; lower ReHo values in the right precentral gyrus, right postcentral gyrus, and left precentral gyrus; and higher ReHo values in the left posterior cingulate cortex. ALFF of the left postcentral gyrus was negatively correlated with baseline HAMA, while that of the right frontal middle cortex was positively correlated with HAMA. ReHo values in the left postcentral gyrus was negatively correlated with baseline HAMA, while that of the right frontal middle cortex was positively correlated with HAMA. ALFF values in the right frontal-superior-orbital cortex and right frontal med-orbital-cortex was positively correlated with illness duration. ReHo value of the right superior-orbital cortex, left hippocampus, and frontal lobe was positively correlated with illness duration respectively. The remitters showed higher ALFF values in the left hippocampus and higher ReHo values in the right postcentral cortex compared to nonremitters.
The prevalence of GAD varies greatly depending on the country (3) and the study conducted (4,5). This is largely due to the pathological mechanism underlying GAD remaining obscure and the lack of objective diagnostic criteria. And there are no reliable markers which can predict the treatment outcome effectively, so it takes at least 2 weeks for a GAD patient to respond to anti-anxiety drug treatment while remission may take even longer (17). It is thus urgent that reliable biomarkers be found in order to guide clinicians in the diagnosis and the treatment of GAD. Accumulated evidence has proven the value of Rs-fMRI indices as biomarkers in GAD, as anxiety is the result of brain dysfunction in some brain regions (17). A core symptom of anxiety disorder, fear, (including panic) is regulated by an amygdala-centered neural circuit. The other core symptom of anxiety disorder, worry, (including anxiety pain, anticipated anxiety, and compulsion) is regulated by the cortic-striatal-thalamic-cortical (CSTC) neural circuit (18). However, anxiety disorder comprises many subtypes, with GAD being one among many, and thus it remains unclear whether these changes are specific to GAD or are instead related to anxiety in general.
In the present study, the GAD group exhibited lower ALFF and ReHo values in the right postcentral and precentral gyrus compared to the HC group, and higher ReHo values in the left posterior cingulate cortex, left angular gyrus (13), and left precuneus compared to the HC group. The GAD group also had higher ALFF values in the dorsomedial prefrontal cortex (DMPFC) and left posterior cingulate cortex compared to the HC group (19). These results indicate a hyperactivation of left posterior cingulate cortex in GAD patients. The precentral gyrus is a part of the prefrontal cortex and postcentral cortex, and is closely linked to the prefrontal cortex. The dysfunction of the prefrontal cortex in GAD has been explained in many studies (20), and emotional dysfunction due to hyperactivity of the amygdala and damage to the prefrontal cortex and hippocampal structures caused by chronic stress plays a key part in the pathological mechanism of anxiety and fear (21). Besides the abnormalities in the prefrontal cortex and left posterior cingulate cortex, we also found abnormal ReHo values in the left angular gyrus and left precuneus. Findings of studies that use rs-fMRI on GAD patients rarely report congruent results because GAD involves many brain regions and may consist of different subtypes; we thus need further large sample studies to confirm the pathological mechanism of GAD.
GAD is a chronic disease with recurrent episodes (6), and there are no reliable markers to evaluate its illness duration and the severity. The present study also found that ALFF and ReHo values in the left postcentral gyrus were negatively correlated with baseline HAMA, while those in the right frontal middle cortex were positively correlated with HAMA. This suggests that the presence of hypoactivity of the left postcentral gyrus and hyperactivity of the right frontal mid cortex may indicate more serious symptoms in GAD patients. ALFF and ReHo values in the right frontal-superior-orbital cortex were positively correlated with illness duration, suggesting that the hyperactivity of the frontal-superior-orbital cortex may indicate a longer illness duration in GAD patients. ReHo value in the left hippocampus and frontal lobe was also positively correlated with disease duration which means the dysfunction of these brain regions may also have a close correlation with the course of GAD. Andreescu et al. (18) also found that longer illness duration was positively correlated with functional connectivity (FC) between the posterior cingulate cortex and the insula in anxiety patients. Meanwhile, worry severity was negatively correlated with FC between the posterior cingulate cortex and the medial prefrontal cortex. These results stress the key importance of the postcentral cortex and prefrontal cortex in GAD. We used ALFF and ReHo values in our study while Andreescu et al. (18) used FC to evaluate brain function, which may explain the discrepancy in results between the studies. The results of rs-fMRI studies in adolescents and elderly GAD patients are usually inconsistent and difficult to duplicate (21,22), which suggests that future studies need to improve the sampling homogeneity, including that of age, course of disease, and disease severity, and this once again suggests that GAD may have different subtypes.
Antidepressants including escitalopram are the first-line drugs in the treatment of GAD (23). However, antidepressants often take weeks to take effect, and, as about half GAD patients cannot achieve remission (2), impairs patient compliance, markers which can predict treatment outcome before the treatment would be highly valuable. According to our findings, compared to nonremitters, remitters showed higher ALFF values in the left fusiform cortex, left hippocampus, right thalamus cortex, and left rolandic operculum cortex; and higher ReHo values in the left angular gyrus and right postcentral cortex. Previous research reported that elevated neural activation in the anterior cingulate cortex, hippocampus, insula, dorsolateral prefrontal cortex, amygdala, and inferior frontal gyrus predicted response to psychotherapy in panic disorder and GAD (24); naturally, as this study was focused on treatment via psychotherapy, the results are different to ours. Meanwhile, Andreescu et al. (18) found that after 12 weeks of treatment, GAD patients showed increased connectivity between the dorsolateral prefrontal cortex and prefrontal regions. Andreescu et al.’s work further highlighted the importance of the postcentral cortex in GAD, and suggested that the hyperactivity of the right postcentral cortex indicates a better outcome for patients treated with escitalopram. However, whether the baseline function of the fusiform cortex, left hippocampus, and right thalamus can predict treatment outcome requires further study.
Previous studies indicate spatiotemporal mechanisms play a key role in the psychopathological symptoms in anxiety disorders, such as dysregulation of synchronization in the neural activity of networks, and based on clinical finding in GAD and rsfMRI observations in healthy subjects, these network abnormalities in the resting state underlie core psychopathological symptoms of GAD such as unstable self, increased anxiety, increased interoceptive and somatic symptoms (25,26). So, the abnormalities of neural network activities and synchronization can be applied to assist the diagnosis of GAD. In our study, we only investigated reginal brain area but not the network of brain so the value of clinical application is limited, and in the future study, we will pay more attention on these areas.
In conclusion, the results of this study indicate that altered regional brain activity and local synchronization may be related to the pathophysiology of GAD and have certain value in predicting remission in treatment. Special attention should be paid to the precentral and postcentral gyrus, as aberrant ALFF and ReHo values in these two regions could be used as a biomarker for GAD.
There are a few limitations to our study. First, our 1.5 T scanner did not duplicate the results of abnormal amygdala function in GAD patients. Sample and scanning imprecision may account for this result, and we should improve the quality of our MRI data acquisition. Second, although we have considered the effects of depression on GAD pathophysiology and brain function, the comorbidity of GAD with major depressive disorder (MDD) is so high that we could not eliminate the effect of depression in this study, and stricter inclusion/exclusion criteria for depression, or perhaps the addition of an MDD control group, will be important for future studies. We also did not eliminate the effects of anti-anxiety drugs on brain function; in fact, brain image of f-MRI has been used to select anti-anxiety drugs in precision medicine of GAD. A larger sample size and longer study duration could also potentially reveal more significant characteristics of rs-fMRI in GAD.
Acknowledgments
The authors would like to thank all participants who took part in this study.
Funding: This study was partly supported by funds from the Huzhou Public Welfare Research Project Social Development (Key Medical and Health) Category (2018GZ39, Xinhua Shen), the Social Development Project of Public Welfare Technology Application in Zhejiang Province in 2019 (LGF19H090003, Xinhua Shen), and the Social Development Project of Public Welfare Technology Application in Zhejiang Province in 2019 (LGF19H090002, Zhongxia Shen).
Footnote
Reporting Checklist: The authors have completed the MDAR reporting checklist. Available at http://dx.doi.org/10.21037/atm-20-6598
Data Sharing Statement: Available at http://dx.doi.org/10.21037/atm-20-6598
Conflicts of Interest: All authors have completed the ICMJE uniform disclosure form (available at http://dx.doi.org/10.21037/atm-20-6598). JZ, ZS, XS, YS, LR, and MQ report grants from Huzhou Public Welfare Research Project Social Development (Key Medical and Health) Category (2018GZ39, Xinhua Shen), grants from Social Development Project of Public Welfare Technology Application in Zhejiang Province in 2019 (LGF19H090003, Xinhua Shen), grants from Social Development Project of Public Welfare Technology Application in Zhejiang Province in 2019 (LGF19H090002, Zhongxia Shen), during the conduct of the study. The other authors have no conflicts of interest to declare.
Ethical Statement: The authors are accountable for all aspects of the work in ensuring that questions related to the accuracy or integrity of any part of the work are appropriately investigated and resolved. The protocol was permitted by the Ethics Committee of Huzhou Third Hospital (Ethical approval number: 2018-010,036). After a complete description of the research scheme, written informed consent was obtained from all participants before the study. All procedures performed in this study involving human participants were in accordance with the Declaration of Helsinki (as revised in 2013).
Open Access Statement: This is an Open Access article distributed in accordance with the Creative Commons Attribution-NonCommercial-NoDerivs 4.0 International License (CC BY-NC-ND 4.0), which permits the non-commercial replication and distribution of the article with the strict proviso that no changes or edits are made and the original work is properly cited (including links to both the formal publication through the relevant DOI and the license). See: https://creativecommons.org/licenses/by-nc-nd/4.0/.
References
- Aronow WS, Shamliyan TA. Effects of atypical antipsychotic drugs on QT interval in patients with mental disorders. Ann Transl Med 2018;6:147. [Crossref] [PubMed]
- Tempesta D, Mazza M, Serroni N, et al. Neuropsychological functioning in young subjects with generalized anxiety disorder with and without pharmacotherapy. Prog Neuropsychopharmacol Biol Psychiatry 2013;45:236-41. [Crossref] [PubMed]
- Huang Y, Wang Y, Wang H, et al. Prevalence of mental disorders in China: a cross-sectional epidemiological study. Lancet Psychiatry 2019;6:211-24. [Crossref] [PubMed]
- Phillips MR, Zhang J, Shi Q, et al. Prevalence, treatment, and associated disability of mental disorders in four provinces in China during 2001-05: an epidemiological survey. Lancet 2009;373:2041-53. [Crossref] [PubMed]
- Shen Z, Zhu J, Yuan Y, et al. The roles of brain-derived neurotrophic factor (BDNF) and glial cell line-derived neurotrophic factor (GDNF) in predicting treatment remission in a Chinese Han population with generalized anxiety disorder. Psychiatry Research 2019;271:319-24. [Crossref] [PubMed]
- Stein MB, Sareen J. Clinical practice. Generalized Anxiety Disorder. N Engl J Med 2015;373:2059-68. [Crossref] [PubMed]
- Dunn EC, Sofer T, Gallo LC, et al. Genome-wide association study of generalized anxiety symptoms in the Hispanic Community Health Study/Study of Latinos. Am J Med Genet B Neuropsychiatr Genet 2017;174:132-43. [Crossref] [PubMed]
- Gottschalk MG, Domschke K. Genetics of generalized anxiety disorder and related traits. Dialogues Clin Neurosci 2017;19:159-68. [Crossref] [PubMed]
- Okbay A, Baselmans BM, De Neve JE, et al. Genetic variants associated with subjective well-being, depressive symptoms, and neuroticism identified through genome-wide analyses. Nat Genet 2016;48:624-33. [Crossref] [PubMed]
- Hettema JM, Kettenmann B, Ahluwalia V, et al. Pilot multimodal twin imaging study of generalized anxiety disorder. Depress Anxiety 2012;29:202-9. [Crossref] [PubMed]
- Tian X, Wei D, Du X, et al. Assessment of trait anxiety and prediction of changes in state anxiety using functional brain imaging: A test–retest study. Neuro Image 2016;133:408-16. [Crossref] [PubMed]
- Klumpp H, Keutmann MK, Fitzgerald DA, et al. Resting state amygdala-prefrontal connectivity predicts symptom change after cognitive behavioral therapy in generalized social anxiety disorder. Biol Mood Anxiety Disord 2014;4:14. [Crossref] [PubMed]
- Wang W, Hou J, Qian S, et al. Aberrant regional neural fluctuations and functional connectivity in generalized anxiety disorder revealed by resting-state functional magnetic resonance imaging. Neurosci Lett 2016;624:78-84. [Crossref] [PubMed]
- Zang Y, Jiang T, Lu Y, et al. Regional homogeneity approach to fMRI data analysis. Neuroimage 2004;22:394-400. [Crossref] [PubMed]
- Carter RM, Wittchen HU, Pfister H, et al. One-year prevalence of subthreshold and threshold DSM-IV generalized anxiety disorder in a nationally representative sample. Depress Anxiety 2001;13:78-88. [Crossref] [PubMed]
- Oathes DJ, Patenaude B, Schatzberg AF, et al. Neurobiological signatures of anxiety and depression in resting-state functional magnetic resonance imaging. Biol Psychiatry 2015;77:385-93. [Crossref] [PubMed]
- Strawn JR, Geracioti L, Rajdev N, et al. Pharmacotherapy for generalized anxiety disorder in adult and pediatric patients: an evidence-based treatment review. Expert Opin Pharmacother 2018;19:1057-70. [Crossref] [PubMed]
- Andreescu C, Sheu LK, Tudorascu D, et al. Emotion reactivity and regulation in late-life generalized anxiety disorder: functional connectivity at baseline and post-treatment. Am J Geriatr Psychiatry 2015;23:200-14. [Crossref] [PubMed]
- Coplan JD, Webler R, Gopinath S, et al. Neurobiology of the dorsolateral prefrontal cortex in GAD: Aberrant neurometabolic correlation to hippocampus and relationship to anxiety sensitivity and IQ. J Affect Disord 2018;229:1-13. [Crossref] [PubMed]
- Mah L, Szabuniewicz C, Fiocco AJ. Can anxiety damage the brain? Curr Opin Psychiatry 2016;29:56-63. [Crossref] [PubMed]
- Roy AK, Fudge JL, Kelly C, et al. Intrinsic functional connectivity of amygdala-based networks in adolescent generalized anxiety disorder. J Am Acad Child Adolesc Psychiatry 2013;52:290-9.e2. [Crossref] [PubMed]
- Cui H, Zhang J, Liu Y, et al. Differential alterations of resting-state functional connectivity in generalized anxiety disorder and panic disorder. Hum Brain Mapp 2016;37:1459-73. [Crossref] [PubMed]
- Northoff G. Spatiotemporal Psychopathology II: How does a psychopathology of the brain's resting state look like? Spatiotemporal approach and the history of psychopathology. J Affect Disord 2016;190:867-79. [Crossref] [PubMed]
- Santos VA, Carvalho DD, Van Ameringen M, et al. Neuroimaging findings as predictors of treatment outcome of psychotherapy in anxiety disorders. Prog Neuropsychopharmacol Biol Psychiatry 2019;91:60-71. [Crossref] [PubMed]
- Northoff G. The brain’s spontaneous activity and its psychopathological symptoms – spatiotemporal binding and integration Prog Neuropsychopharmacol Biol Psychiatry 2018;80:81-90. [Crossref] [PubMed]
- Tompson S, Chua HF, Kitayama S. Connectivity between mPFC and PCC predicts post-choice attitude change: the self-referential processing hypothesis of choice justification. Hum Brain Mapp 2016;37:3810-20. [Crossref] [PubMed]