A CT-based radiomics signature for evaluating tumor infiltrating Treg cells and outcome prediction of gastric cancer
Introduction
Regulatory T (Treg) cells are a subset of CD4+ T cells which distinguished by expression of the transcription factor forehead box protein P3 (FoxP3). Accumulating evidence demonstrated that a large number of Treg cells were infiltrated in tumor microenvironment (1). In addition, most of Treg cells within tumors had high proliferative activity and could lead to profound immunosuppression within tumors. A number of studies showed that higher fraction of Treg cells was associated with worse prognosis of breast cancer (2), non-small-cell lung cancer (3), pancreatic cancer (4), renal cell cancer (5), head and neck squamous cell cancer (6) and gastric cancer (GC) (7). Rapid progression referred to as hyper progression disease (HPD) had been observed in up to 10% of patients received immune checkpoint inhibitor (ICI) of several types of tumors (8,9). Recently, Kamada et al. reported that Treg cells may contribute to the HPD in proportion of GC patients treated with anti-PD-1 therapy (8). These results suggested a potential role for tumor infiltrating Treg (TITreg) cells in prediction of outcome and treatment response of immunotherapies.
Computational medical imaging, known as Radiomics, are processes that translate medical images into high-dimensional quantitative data and thus enable the characterization of microscopic features of tumor tissues such as cellular, molecular and even gene expression properties. In previous studies, radiomics had successful applicated in the differential diagnosis, prognosis or lymph node metastasis prediction of GC patients (10-12). Therefore, the radiomics-based biomarkers could be a novel non-invasive biomarker for diagnosis, prognosis and treatment response of cancer patients and promising complimentary to other gene-driven tumor markers or biopsies.
To our knowledge, there is no previous study that had investigated the value of radiomics for assessing TITreg cells. Thus, the aim of current study is to develop and validate a CT-based radiomics signature for evaluation of TITreg cells in GC. The prognostic value of the radiomics signature was also evaluated.
Methods
Patients and study design
This study was conducted in three independent cohorts. One hundred and thirty-five GC patients who had undergone radical gastrectomy at Tianjin Medical University Cancer Institute and Hospital between June 2012 and April 2016 were enrolled. The patients were randomly divided into training cohort (n=90) and validation cohort (n=45). The inclusion criteria: (I) patients received radical gastrectomy with D2 lymphadenectomy; (II) GC diagnosis was histologically confirmed; (III) contrast-enhanced abdominal CT images were acquired within 14 days before operation; (IV) Image quality was satisfactory for analysis. The exclusion criteria: (I) patients received chemotherapy or radiotherapy before surgery; (II) with other malignant tumors; (III) incomplete clinical information.
The Cancer Genome Atlas (TCGA) Stomach Adenocarcinoma (STAD) dataset was used as an external testing cohort. The CT images of TCGA-STAD dataset were obtained from The Cancer Imaging Archive (TCIA). The RNA-sequencing data and clinical information of patients were acquired from the TCGA portal. The abundance of TITreg cells of each patient was calculated using CIBERSORT web tools (13). All patients of TCGA dataset had undergone radical gastrectomy and the diagnosis of GC were also histologically confirmed.
This study was approved by ethics committee of our institute and informed consent was obtained from all the patients. The flowchart of study design is showed in Figure 1.
CT scanning protocol
Contrast-enhanced abdominal CT was performed by using Discovery CT750 HD (GE Medical Systems, Milwaukee, Wisconsin) or Somatom Sensation 64 scanner (Siemens Medical Solutions, Forchheim, Germany). Oral doses of water (500–1,000 mL) were administered to ensure stomach distension prior to CT examination. The parameters were as follows: tube voltage was 120 kVp, tube current was 150–200 mA; field of view, 350 mm × 350 mm; matrix, 512×512; Images reconstruction section thickness: 1.25 mm. Arterial phase images were obtained following delays of 20 seconds after intravenous injection of contrast media (2.5 mL/s, 1.2 mL/kg; Omnipaque 300, GE Healthcare, Chicago, Illinois) via a syringe pump. The portal venous phase was obtained after delays of 60 seconds.
Multispectral immunohistochemical analysis of TITreg cells
The abundance of TITreg cells was analyzed utilizing Opal 7-Colour Manual IHC Kit (PerkinElmer NEL811001KT) according to the manufacture’s protocol (14). In brief, the slides were incubated with Antibody Diluent blocking buffer (PerkinElmer) at room temperature (RT) for 10 min. The primary antibody for CD4 (Abcam, ab133616, 1:500) and FoxP3 (R&D, MAB8214, 1:400) were incubated at RT for 1 h. Then, a secondary HRP antibody were incubated at RT for 10 min. Signal amplification was performed using Opal 520 TSA (PerkinElmer) and incubated at RT for 10 min. Visualization of the slides was done using the Mantra Quantitative Pathology Imaging System (PerkinElmer) and analyzed using InForm Image Analysis software (PerkinElmer, version 2.1). The TITreg cell fraction in CD4+ cells were calculated and data were presented as mean ± SD.
Estimation of TITreg cells using CIBERSORT algorithm
CIBERSORT is a gene expression-based deconvolution algorithm for assessing immune cell composition. Normalized gene expression data of TCGA-STAD dataset was uploaded to CIBERSORT web portal (13). The proportion of 22 types of intratumoral immune cells, including Treg cells, were evaluated with the default algorithm at 1000 permutations (15).
Tumor segmentation and feature extraction
The portal venous phase of contrast-enhanced CT images was selected for the analysis because most of the lesions had significant enhancement in this phase (16).
The volume of interest (VOI) of tumors were manually drawn along the tumor boundaries by using 3D Slicer software (version 4.8.1). The VOIs were delineated by two radiologists (Reader 1, X Gao; Reader 2, T Ma) with 6 years and 9 years of experience, respectively. Reader 1 performed tumor segmentations for patients of all cohorts. Reader 2 independently segmented tumors of the training cohort. Inter-class correlation coefficients (ICCs) were calculated for evaluation of inter-observer reliability of the extracted features. Feature extraction was performed by using PyRadiomics software (version 2.2.0) (17). Eight hundred and fifty-nine radiomic features were extracted from VOIs. The features were divided into four catalogs including: first-order, shape, texture, and wavelet features. Details of radiomic features are showed in Table S1.
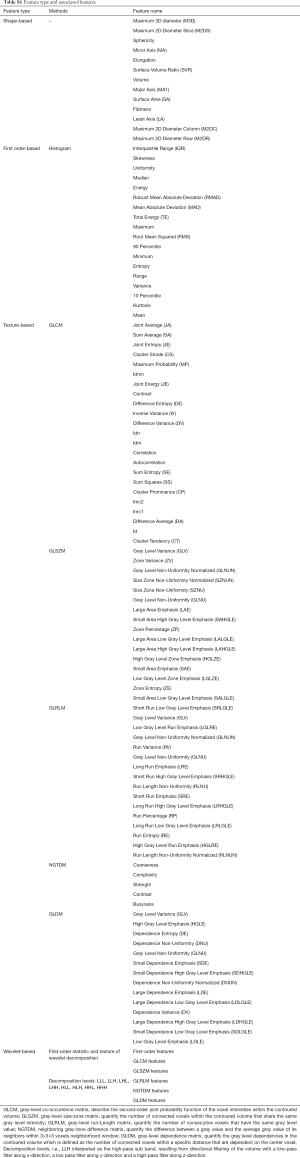
Full table
Feature selection and radiomics signature construction
To evaluate the inter-observer reliability of the extracted features, tumors of the training cohort were independently segmented by two radiologists (X Gao and T Ma) and ICCs were calculated for each feature. Only features with ICC ≥0.8 were considered as highly reproducible and retained. Then, the patients were stratifying into high or low Treg cell group on the basis of median value of Treg cell fraction in CD4+ cells. The Mann-Whitney U test was utilized to compare the value of each radiomics feature between high and low TITreg cell groups. The features with P<0.05 were considered as significant different features between two groups and included for further analysis. Finally, the least absolute shrinkage and selection operator (Lasso) regression model was used for feature selection and signature construction (18). The regularization parameter λ was defined by use of 10-fold cross-validation. Lasso logistic regression analysis was done utilizing “glmnet” package of R software. The workflow of radiomics analysis is showed in Figure 2.
Statistical analysis
The t-test, Mann-Whitney U test or one-way ANOVA test was used for numerical variables. χ2 test or Fisher’s exact test was used for categorical variables. The receiver operator characteristic (ROC) curves and the area under the curve (AUC) were utilized to assess the performance of radiomics signature for estimating TITreg cells. Univariable and multivariable Cox regression analysis were used for identifying risk factors of overall survival (OS). Factors with statistical significance at the univariable analysis were analyzed for the multivariable model with step-wise forward approach. The optimal cutoff value of radiomics score was decided with the maximized Youden index from the training cohort and patients were divided into high or low radiomics score group. OS was evaluated using the Kaplan-Meier curve along with log-rank test. P<0.05 was considered as statistical significance. Statistical analysis was performed by using R (version 3.4.2).
Results
Patients characteristics
The clinical characteristics of patients are showed in Table 1. The contrast-enhanced abdominal CT images, RNA-seq data and clinical information of TCGA-STAD dataset were downloaded from TCGA and TCIA data portal. 18 patients were excluded for lacking of RNA-seq data and 30 patients were finally included as the testing cohort. All patients from the three cohorts were pathological diagnosed with gastric adenocarcinoma. No significant difference in clinical covariates was observed among the three cohorts.
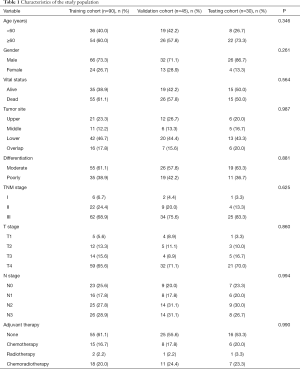
Full table
Evaluation of TITreg cells abundance in the training, validation and testing cohort
The abundance of TITreg cell of 135 patients of the training and the validation cohort were estimated using multispectral immunohistochemical analysis. The TITreg cell fraction in CD4+ cells of the training cohort and the validation cohort were 22.41%±17.53% and 21.38%±13.72%, respectively. As showed in Figure 3, the relative fraction of 22 tumor-infiltrating immune cell of 30 GC patients of the testing cohort were estimated utilizing CIBERSORT. The TITreg cell fraction in CD4+ cells of the testing cohort were 23.21%±13.28%. The fraction of TITreg cells were not significantly differed among three cohorts (P=0.879, Figure 3A).
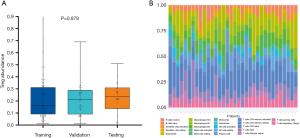
Selection of features and establishment of radiomics signature
Of 859 features extracted from the selected ROIs of the training cohort, 70 features with ICC <0.8 were excluded. Then, 145 of the remained 789 features were identified as significant differed features between high and low Treg groups (P<0.05) and enrolled into LASSO regression analysis. Finally, the radiomics signature was built with 6 features. The calculation formula radiomics score is provided in supplementary materials.
The performance of radiomics signature for estimating TITreg cells
The ability of radiomics signature for estimating TITreg cells abundance was evaluated by using ROC curves. The AUCs of radiomics signature for the training and the validation cohorts were 0.884 (95% CI: 0.816–0.952, Figure 4A) and 0.869 (95% CI: 0.760–0.978, Figure 4B), respectively. To verify the predictive ability of the radiomics signature in different ethnic population, TCGA-STAD dataset which enrolled non-Asian patients was used as an external testing cohort. The radiomics signature also showed a good predictive performance in the testing cohort with an AUC of 0.847 (95% CI: 0.698–0.997, Figure 4C).
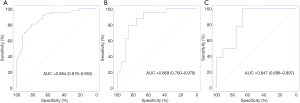
Prognostic value of TITreg cells and radiomics signature
As shown in Table 2, univariable and multivariable Cox regression analysis showed that the radiomics signature [hazard ratio (HR) 2.018, 95% CI: 1.133–3.721, P=0.012], T-stage (HR 1.012, 95% CI: 1.008–1.875, P=0.042) and TNM stage (HR 1.226, 95% CI: 1.101–2.007, P=0.033) were independent predictors of OS. Kaplan-Meier analysis revealed that higher radiomics score were associated with poorer OS in the training (P=0.011, Figure 5A), validation (P=0.016, Figure 5B) and the testing cohort (P=0.022, Figure 5C). The higher abundance of TITreg cells were also related to unfavorable OS in the training (P=0.006, Figure 5D), validation (P=0.021, Figure 5E) and the testing cohort (P=0.039, Figure 5F).
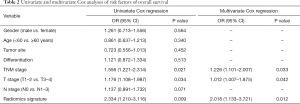
Full table
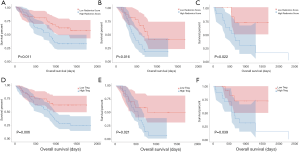
Discussion
In our study, six robust image features were identified and utilized to build a radiomics signature for assessing TITreg cells of GC patients. The AUCs of radiomics signature for the training and the validation cohort were 0.884 and 0.869, respectively. Then, by linking image features and gene expression signature of Treg cells, the performance of the radiomics signature was confirmed in an independent cohort with an AUC of 0.847. In addition, survival analysis showed that the radiomics signature could also sever as a predictor of OS in GC patients.
Treg cells are a subset of CD4+ T cells with immunosuppressive function. An increasing body of evidence demonstrated that Treg cells are highly infiltrated in various tumors. A recent meta-analysis showed that higher Treg cells was significantly correlated with poor outcome in most of solid tumors (19). Thus, TITreg cells play a vital role in shaping immunosuppressive tumor microenvironment, leading to the progression, invasion and metastasis of tumor (20,21).
ICIs represented by anti-CTLA-4 and anti-PD-1/L1 agents exhibited encouraging efficacy in various solid tumors and has deeply changed the strategy of cancer treatment. Some studies demonstrated that PD-1 also expressed on a subset of Treg cells (22-24). Furthermore, through interaction with PD-L1 on CD8+ T cells, upregulation of PD-1 on Treg cells could strengthen the suppression of immune response of CD8+ T cells (23). Recently, Kamada et al. reported that PD-1+ Treg cells significantly amplified in GC patients who received anti-PD-1 and contributed to the development of HPD (8). Thus, the radiomics signature established in our study maybe a potential non-invasive biomarker for predicting and monitoring the development of HPD induced by anti-PD-1/PD-L1 agents.
To date, studies about the relationship between radiomics features and intratumoral immune landscape are still limited. Tang et al. identified a radiomics signature consist of four features for prognosis prediction of lung cancer. The signature divided patients into four clusters which associated with OS. Specifically, a favorable outcome cluster with low CT intensity and high heterogeneity had the low level of PD-L1+ tumor cell and the high level of infiltrating CD3+ T cells (25). However, whether CD3+ T cells rather than specific subset of T cells could be a reliable biomarker for immune response is still a controversial problem (26). Sun and colleagues proposed and tested a radiomic signature for assessing tumor-infiltrating CD8+ cells in three datasets. Their results showed that the radiomic signature could be a useful biomarker for assessing CD8+ cell abundance and outcome predication for patients received PD-1/PD-L1 therapy (27). However, Sun et al.’s study enrolled more than 10 types of tumors. Since the patterns of intratumoral immune cell are tremendously different among different types of tumors, their results may still need to be confirmed in specific tumor type.
This study has some limitations. First, the retrospective nature of this study was inherently disadvantageous. Second, although the radiomics signature was established and tested with three independent cohorts, the sample size was relatively small. Third, no patients received anti-PD-1 treatment was enrolled for evaluation the ability of the radiomics signature for predicting treatment response. Fourth, although same CT scan parameters were used in different scanners and cohorts, images acquired with different scanners and at different centers may still introduce bias.
Conclusions
In conclusion, we proposed a CT-based radiomics signature for estimating TITreg cells and outcome prediction of GC. Multicenter studies with larger dataset are warranted to verify our results for clinical practice. GC Patients received anti-PD-1 are also required to further investigate the potential of the radiomics signature for predicting treatment response of anti-PD-1 agents.
Supplementary
Radiomics score calculation formula
Radiomics score = (0.645 × GLSZM.Gray Level Non Uniformity Normalized) – (0.211×GLSZM.Gray Level Variance) – (0.216 × GLSZM.Small Area High Gray Level Emphasis) – (0.137 × Wavelet-WLW.ngtdm.Complexity) + (0.08 × GLCM.MCC − 0.221) × (Wavelet-LLL.firstorder.Maximum).
Acknowledgments
Funding: This work was supported by grants from the Fund of National Natural Science Foundation of China (grant number 81772620), and Tianjin major project for chronic disease prevention and control (grant number 17ZXMFSY0).
Footnote
Conflicts of Interest: All authors have completed the ICMJE uniform disclosure form (available at http://dx.doi.org/10.21037/atm.2020.03.114). The authors have no conflicts of interest to declare.
Ethical Statement: The authors are accountable for all aspects of the work in ensuring that questions related to the accuracy or integrity of any part of the work are appropriately investigated and resolved. This study was approved by the Ethics Committee of Tianjin Medical University Cancer Institute and Hospital (No. E2019082) and informed consent was obtained from all the patients.
Open Access Statement: This is an Open Access article distributed in accordance with the Creative Commons Attribution-NonCommercial-NoDerivs 4.0 International License (CC BY-NC-ND 4.0), which permits the non-commercial replication and distribution of the article with the strict proviso that no changes or edits are made and the original work is properly cited (including links to both the formal publication through the relevant DOI and the license). See: https://creativecommons.org/licenses/by-nc-nd/4.0/.
References
- Tanaka A, Sakaguchi S. Targeting Treg cells in cancer immunotherapy. Eur J Immunol 2019;49:1140-6. [PubMed]
- Li F, Zhao Y, Wei L, et al. Tumor-infiltrating Treg, MDSC, and IDO expression associated with outcomes of neoadjuvant chemotherapy of breast cancer. Cancer Biol Ther 2018;19:695-705. [Crossref] [PubMed]
- Barua S, Fang P, Sharma A, et al. Spatial interaction of tumor cells and regulatory T cells correlates with survival in non-small cell lung cancer. Lung Cancer 2018;117:73-9. [Crossref] [PubMed]
- Wang X, Lang M, Zhao T, et al. Cancer-FOXP3 directly activated CCL5 to recruit FOXP3+ Treg cells in pancreatic ductal adenocarcinoma. Oncogene 2017;36:3048-58. [Crossref] [PubMed]
- Ghatalia P, Gordetsky J, Kuo F, et al. Prognostic impact of immune gene expression signature and tumor infiltrating immune cells in localized clear cell renal cell carcinoma. J Immunother Cancer 2019;7:139. [Crossref] [PubMed]
- Seminerio I, Descamps G, Dupont S, et al. Infiltration of FoxP3+ Regulatory T Cells is a Strong and Independent Prognostic Factor in Head and Neck Squamous Cell Carcinoma. Cancers (Basel) 2019. [Crossref] [PubMed]
- Knegt P, de Jong PC, van der Schans EJ. Pharyngeal diverticula. Clin Otolaryngol Allied Sci 1987;12:319-20. [PubMed]
- Kamada T, Togashi Y, Tay C, et al. PD-1+ regulatory T cells amplified by PD-1 blockade promote hyperprogression of cancer. Proc Natl Acad Sci U S A 2019;116:9999-10008. [Crossref] [PubMed]
- Togasaki K, Sukawa Y, Kanai T, et al. Clinical efficacy of immune checkpoint inhibitors in the treatment of unresectable advanced or recurrent gastric cancer: an evidence-based review of therapies. Onco Targets Ther 2018;11:8239-50. [Crossref] [PubMed]
- Li W, Zhang L, Tian C, et al. Prognostic value of computed tomography radiomics features in patients with gastric cancer following curative resection. Eur Radiol 2019;29:3079-89. [Crossref] [PubMed]
- Wang Y, Liu W, Yu Y, et al. CT radiomics nomogram for the preoperative prediction of lymph node metastasis in gastric cancer. Eur Radiol 2020;30:976-86. [Crossref] [PubMed]
- Ma Z, Fang M, Huang Y, et al. CT-based radiomics signature for differentiating Borrmann type IV gastric cancer from primary gastric lymphoma. Eur J Radiol 2017;91:142-7. [Crossref] [PubMed]
- Newman AM, Liu CL, Green MR, et al. Robust enumeration of cell subsets from tissue expression profiles. Nat Methods 2015;12:453-7. [Crossref] [PubMed]
- Nghiem PT, Bhatia S, Lipson EJ, et al. PD-1 Blockade with Pembrolizumab in Advanced Merkel-Cell Carcinoma. N Engl J Med 2016;374:2542-52. [Crossref] [PubMed]
- Fu H, Zhu Y, Wang Y, et al. Identification and Validation of Stromal Immunotype Predict Survival and Benefit from Adjuvant Chemotherapy in Patients with Muscle-Invasive Bladder Cancer. Clin Cancer Res 2018;24:3069-78. [Crossref] [PubMed]
- Tsurumaru D, Miyasaka M, Nishimuta Y, et al. Differentiation of early gastric cancer with ulceration and resectable advanced gastric cancer using multiphasic dynamic multidetector CT. Eur Radiol 2016;26:1330-7. [Crossref] [PubMed]
- van Griethuysen JJM, Fedorov A, Parmar C, et al. Computational Radiomics System to Decode the Radiographic Phenotype. Cancer Res 2017;77:e104-7. [Crossref] [PubMed]
- Huang YQ, Liang CH, He L, et al. Development and Validation of a Radiomics Nomogram for Preoperative Prediction of Lymph Node Metastasis in Colorectal Cancer. J Clin Oncol 2016;34:2157-64. [Crossref] [PubMed]
- Shang B, Liu Y, Jiang SJ, et al. Prognostic value of tumor-infiltrating FoxP3+ regulatory T cells in cancers: a systematic review and meta-analysis. Sci Rep 2015;5:15179. [Crossref] [PubMed]
- Shi C, Chen Y, Chen Y, et al. CD4+ CD25+ regulatory T cells promote hepatocellular carcinoma invasion via TGF-beta1-induced epithelial-mesenchymal transition. Onco Targets Ther 2018;12:279-89. [Crossref] [PubMed]
- Li X, Ma K, Song S, et al. Tight correlation between FoxM1 and FoxP3+ Tregs in gastric cancer and their clinical significance. Clin Exp Med 2018;18:413-20. [Crossref] [PubMed]
- Zhang B, Chikuma S, Hori S, et al. Nonoverlapping roles of PD-1 and FoxP3 in maintaining immune tolerance in a novel autoimmune pancreatitis mouse model. Proc Natl Acad Sci U S A 2016;113:8490-5. [Crossref] [PubMed]
- Park HJ, Park JS, Jeong YH, et al. PD-1 upregulated on regulatory T cells during chronic virus infection enhances the suppression of CD8+ T cell immune response via the interaction with PD-L1 expressed on CD8+ T cells. J Immunol 2015;194:5801-11. [Crossref] [PubMed]
- Asano T, Meguri Y, Yoshioka T, et al. PD-1 modulates regulatory T-cell homeostasis during low-dose interleukin-2 therapy. Blood 2017;129:2186-97. [Crossref] [PubMed]
- Tang C, Hobbs B, Amer A, et al. Development of an Immune-Pathology Informed Radiomics Model for Non-Small Cell Lung Cancer. Sci Rep 2018;8:1922. [Crossref] [PubMed]
- Jin S, Xu B, Yu L, et al. The PD-1, PD-L1 expression and CD3+ T cell infiltration in relation to outcome in advanced gastric signet-ring cell carcinoma, representing a potential biomarker for immunotherapy. Oncotarget 2017;8:38850-62. [PubMed]
- Sun R, Limkin EJ, Vakalopoulou M, et al. A radiomics approach to assess tumour-infiltrating CD8 cells and response to anti-PD-1 or anti-PD-L1 immunotherapy: an imaging biomarker, retrospective multicohort study. Lancet Oncol 2018;19:1180-91. [Crossref] [PubMed]