Suggestions for designing studies investigating diagnostic accuracy of biomarkers
A diagnostic test accuracy (DTA) study is a special type of clinical research. Traditional observational or interventional research primarily focuses on the efficiency and safety of treatment approaches, or factors affecting the occurrence or outcomes of a target disease. In contrast, DTA studies center around the diagnostic efficiency of index tests, including those of serum biomarkers and imaging parameters. The metrics used to estimate the diagnostic efficiency of an index test include sensitivity, specificity, accuracy, area under curve (AUC), positive/negative likelihood ratio (PLR/NLR), and positive/negative predictive value (PPV/NPV) (1).
The majority of diseases have their own reference standards; however, the clinical application of their reference standards usually has shortcomings which limits their clinical application. In breast cancer diagnosis, for example, the reference standard for breast cancer diagnosis is biopsy, which is invasive and operator dependent. Consequently, researchers usually search for some noninvasive, low-cost, convenient, objective, and shorter turn-around time (TAT) tools for breast cancer diagnosis, like serum or urine biomarkers. Notably, during the past decades, the number of studies investigating diagnostic accuracy of biomarkers has gradually increased. This may be by virtue of biomarkers’ advantages, which include lower costs, a noninvasiveness approach, objective assessment, convenience, and shorter TAT. Before deciding whether to recommend a biomarker in a guideline, it is crucial to evaluate its diagnostic accuracy in various clinical settings. The studies with rigorous design and meaningful statistical analysis can provide strong and straightforward evidence for guideline markers. Therefore, it is essential that studies be conducted scrupulously in order to properly evaluate the diagnostic accuracy of biomarkers.
Here, we review and summarize the key knowledge needed for designing a DTA study that investigates the diagnostic accuracy of biomarkers. Although only biomarkers are discussed in this study, some of the conclusions in this report can also be extended to other diagnostic tools, such as imaging, immunochemistry, or electrocardiograph parameters.
Consecutively enrolling participants with uniform inclusion and exclusion criteria
In clinical practice, the information obtained first for diagnosis is history, symptoms, and signs. Therefore, these are usually listed as inclusion and exclusion criteria in DTA. For example, if a patient with dyspnea visits an emergency department (ED), heart failure (HF) may be suspected by a clinician. However, the current criteria for HF diagnosis is very subjective, and treatment response is needed in some cases. In such cases, some researchers may consider serum biomarkers for HF diagnosis, including N-terminal pro-brain natriuretic peptide (NT-proBNP). When performing a study investigating the diagnostic accuracy of NT-proBNP for HF, the inclusion criteria should be patients with dyspnea who visit the ED. Notably, although some patients visit the ED complaining of dyspnea, their diagnosis is easy to make based on history, signs, or symptoms, trauma induced dyspnea. Therefore, trauma-induced dyspnea should be listed as an exclusion criterion (2). The participants who are enrolled should meet the inclusion criteria but should not meet the exclusion criteria. In some studies, researchers enroll healthy individuals as controls. This type of study design can bias the diagnostic accuracy of an index test, because healthy individuals are obviously not the target population in whom HF is suspected (3,4).
Another design weakness involves the two-gate design (3). In a study investigating the diagnostic accuracy of serum and urine cytokeratin-19 fragments (CYFRA 21-1) for bladder cancer (5), the researchers did not report whether there was a uniform inclusion and exclusion criteria for patient enrollment. They only reported that they enrolled some patients with bladder cancer, and patients with cystitis, urolithiasis, urinary tract infection (UTI), kidney carcinomas, and benign bladder tumor were set as controls. In fact, some of the non-bladder cancer subjects might have had different signs, symptoms, and history when compared with the bladder cancer patients. Thus, they could not be the target population in whom bladder cancer should be suspected. In short, uniform inclusion and exclusion criteria are essential to ensuring the representativeness of participants in DTA studies. The representativeness of subjects is especially important because only studies with a real-world design can guide real-world clinical practice.
In addition to uniform inclusion and exclusion criteria, another key point to ensure the representativeness of participants is consecutive enrollment. That means, unless ethical issues arise, all participants who meet the inclusion criteria and do not meet the exclusion criteria should be enrolled, irrespective of their final diagnosis, social and economic status, disease severity, and complications. In a study investigating the diagnostic accuracy of CYFRA 21-1 for bladder cancer (5), the researchers did not report whether the participants were consecutively enrolled, the ramifications of which were that the prevalence of bladder cancer in the studied cohort might not have been consistent with clinical practice. In a DTA study, prevalence has theoretically little effect on sensitivity, specificity, PLR, and NLR; however, NPV, and PPV are greatly affected by it (6). Therefore, the PPV and NPV in this study are unreliable. Usually, we believe that the level of evidence from prospective studies is higher than that from retrospective studies. This is partly because that, in prospective DTA studies, subjects can be consecutively enrolled, and thus the prevalence of target disease is consistent with clinical settings. In addition, the proportion of missing data is lower in prospective studies. In retrospective studies, meanwhile, some subjects may be excluded because they have some missing value, and the prevalence of target disease is problematic.
I suggest the full title of a DTA report should be PIDTA: P, participants; I, index test; D, study design; T, target disease; A, aims. The two key characteristics of study design are the type of data collection (prospective or retrospective) and whether blinding is performed. The aims of a study include evaluating the diagnostic accuracy of an index test, comparing the diagnostic accuracy of two or more index tests, and evaluating whether a new diagnostic test provides additional diagnostic information beyond conventional diagnostic information.
Here are two examples of DTA report titles:
- Diagnostic accuracy of N-terminal pro-brain natriuretic peptide for heart failure in dyspnea patients: a prospective study (P: dyspnea patients; I: N-terminal pro-brain natriuretic peptide; D: prospective study; T: heart failure; A: only evaluate the diagnostic accuracy of N-terminal pro-brain natriuretic peptide).
- Head-to-head comparison of serum and urine cytokeratin-19 fragments for bladder cancer diagnosis in hematuria patients: a prospective and double-blinded study (P: hematuria patients; I: serum and urine cytokeratin-19 fragments; D: prospective, double-blinded, and head-to-head comparison; T: bladder cancer; A: comparing the diagnostic accuracy of serum and urine cytokeratin-19 fragments).
Blinded testing and interpretation
Blinded design is usually used in interventional studies. In DTA studies, blinded design has two key points. One is that the results of an index test should be unknown by the clinicians who make the final diagnosis (clinician blinded), and the other is that the final diagnosis of subjects should be unknown to operator who perform the index test determination (operator blinded).
For biomarkers or other laboratory tests, operator-blinding may have little effect on the final diagnosis. This is due to the fact that these are objective results obtained from laboratory instruments. However, for some scales or questionnaires, the effect of operators cannot be neglected. If the operator who records the scale knows the final diagnosis of the subjects, they may be more prone to categorize the subjects into the case group, leading to an overestimation of the diagnostic accuracy of the index test.
Clinician-blinding is another critical issue in designing DTA studies. This is especially important in the conditions where the diagnostic criteria for the target disease is subjective. NT-proBNP for HF diagnosis in dyspnea patients is a typical example. If the clinicians who make the final diagnosis already know the results and NT-proBNP before diagnosis, some of the non-HF subjects with higher NT-proBNP may be misclassified as HF, and the diagnostic accuracy of NT-proBNP may be overestimated.
Some of biomarkers have been listed as a component or item of a diagnostic criterion, such as serum anti-cyclic citrullinated peptide (anti-CCP) for rheumatoid arthritis (RA) (7) and D-dimer for disseminated intravascular coagulation (DIC) (8). In these cases, it is unreasonable to evaluate the biomarker’s diagnostic accuracy. In fact, if a biomarker has been recommended as a diagnostic marker for a given disease by a guideline, it means that its diagnostic value is very clear and robust, and the studies investigating the diagnostic accuracy of the biomarker may lack novelty.
Double blinding is very important when comparing the diagnostic marker of two biomarkers in a head-to-head manner. In a study comparing the diagnostic accuracy of BNP, NT-proBNP, and mid-regional pro-atrial natriuretic peptide (MR-proANP) for HF in dyspnea patients visiting ED, NT-proBNP and MR-proANP were masked to clinicians who made the final diagnosis, while the results of the BNP were not masked due to ethical reasons (9). In cases like these, it is reasonable to compare the diagnostic accuracy of NT-proBNP and MR-proANP, while it is unreasonable to compare the diagnostic accuracy of NT-proBNP and BNP, or MR-proANP and BNP. This is because the knowledge of BNP may positively influence the diagnosis of HF and bias the diagnostic accuracy in favor of BNP (9).
Prespecified threshold
If the index test has categorized data with a clear and well-defined threshold, a two-by-two table can be constructed while sensitivity, specificity, NLR, PLR, PPV, and NPV can be easily calculated. However, for continuous data, the situation is more complicated, because there is a trade-off between sensitivity and specificity. Take NT-proBNP and HF diagnosis in dyspnea patients as an example. It is well known that HF patients have significantly higher serum NT-proBNP than non-HF patients. If the threshold is set at a low level, the sensitivity would be high while the specificity would be low. The sensitivity decreases and the specificity increases if the threshold increases. Therefore, it is quite challenging for researchers to establish an optimal threshold. Receiver operator characteristics (ROC) curves with AUC can be used to estimate the overall diagnostic accuracy of an index test with continuous distribution. However, the clinical interpretation of AUC is not straightforward. Some researchers adopt the threshold with the maximum Youden index on a ROC curve as a recommenced threshold. However, this data-driven strategy may overestimate the diagnostic accuracy of an index test (10).
Here, we present two personal opinions concerning threshold selection. The first approach is to adopt the threshold used in previous studies. Because the threshold is prespecified, it has equal probability to positively or negatively bias the diagnostic accuracy of an index test. Another approach is more complicated, depending the clinical settings. If the index test is used for ruling out a target disease (e.g., troponin I for ruling out acute myocardial infarction, D-dimer for ruling out pulmonary embolism), a prespecified NPV should be defined at a high level (e.g., 99.0% or 99.5%). For an index test which aims to decrease the risk of further diagnostic tools [e.g., tumor markers for decreasing the risk of invasive biopsy (11)], the strategy proposed by Pepe et al. is suggested (12). This strategy emphasizes adopting a threshold depending on the prevalence of the target disease in the study’s cohort, and the ratio of benefit associated with the clinical outcomes of a positive test in cases [true positive (TP)] to cost associated with a positive test in controls [false positive (FP)].
A single reference standard for all subjects
A reference standard is a critical component in a DTA study. The basic requirement for a reference is that it should correctly differentiate disease and non-disease. For some diseases, the reference standards are clear and widely accepted, like biopsy for breast cancer. However, for some diseases, the diagnosis is more subjective, such as the diagnosis of pneumonia in dyspnea patients. Under such conditions, an expert committee is usually established to make the final diagnosis.
Usually, the reference used in a DTA study should be performed in all subjects, regardless of the presence of disease. For example, in a study investigating the diagnostic accuracy of methylated septin 9 for breast cancer (13), the researchers reported the following:
“A total of 86 breast tumor tissue samples (59 breast cancer and 27 benign breast tumor patients) confirmed by pathologic examinations were obtained from the needle breast biopsy collections of Liaocheng People’s Hospital between August 2016 and June 2017”.
It is clear that all of the participants, regardless of whether breast cancer was present, received pathologic examinations, and thus this study has no bias in the reference standard. However, for some studies, this issue is not clearly reported or completed correctly. For example, in a study investigating the diagnostic accuracy of prothrombin induced by vitamin K absence-II (PIVKA-II) for chronic hepatitis B (CHB)-related hepatocellular carcinoma (HCC), 134 HCC and 119 CHB patients without HCC (control group) were enrolled. The researcher reported the following:
“The HCC subjects were initially diagnosed by ultrasonography, computed tomography (CT), magnetic resonance imaging (MRI), or selective celiac angiography, and confirmed by pathological examination of the liver biopsies. Chronic HBV infection was defined as the persistent existence of hepatitis B surface antigen (HBsAg) for at least 1 year or HBV DNA concentrations more than 105”.
The statement is unclear and it is unknown whether the authors exclude HCC patients in the control group using the reference standard. CHB patients complicated with HCC is very common in clinical practice. Therefore, liver biopsy, computed tomography (CT), and magnetic resonance imaging (MRI) should also be performed in the control group to exclude HCC (14).
A few meaningful statistical methods
As mentioned above, ROC curve analysis is a popular method to estimate the diagnostic accuracy of an index test. ROC curve is the combination of sensitivity and specificity at various thresholds with the AUC reflecting the global diagnostic accuracy of an index test.
Currently, several novel statistical methods have been developed for DTA studies. Here, we introduce a few of them.
Decision curve analysis (DCA)
A ROC curve with a sensitivity and specificity at a certain threshold reflects the diagnostic accuracy of an index test; however, it cannot conclude whether there is net benefit for a participant nor determine how many patients will benefit from the index test. DCA is a novel statistical method for DTA studies proposed by Vickers et al. in 2006 (15). It graphically depicts the net benefit for all subjects at various thresholds. Take a recent work concerning diagnostic accuracy of urine routine parameters for UTI as an example (16). Urine culture is the standard reference for UTI diagnosis, but it has some limitations in that it is subject to contamination and can be time- and labor-consuming. Therefore, some researchers suggest using urine bacteria for UTI diagnosis. In this study, the prevalence of UTI in studies cohort was 16.8% (156/927). At a probability of 30%, the net benefit of urine bacteria was 0.0419. The reasons for this are explained below.
If the clinicians or patients believe that antibiotic therapy should be initiated or urine culture should be performed when the probability of UTI is higher than 30%, some UTI patients with a probability of more than 30% (TP) would benefit. However, for some non-UTI patients with a probability of more than 30% (FP), antibiotics therapy or urine culture is harmful. Hence, what is key under such circumstance is to ascertain whether the benefit is greater than the harm for subjects with a probability of more than 30%. For an individual, we can calculate the probability of harm and benefit according to a formula proposed by Vickers et al. (15). The mathematic basis of the formula is beyond the focus of this review. What is important is that the net benefit at the probability of 30% is 0.0419. This means that, if the clinicians ask 100 suspected UTI patients to receive bacteria determination and let the patients with a probability of more than 30% receive urine culture or antibiotics therapy, a net 4.19 TP patients (UTI patients) will be identified, without increasing the number of FP (17).
Diagnostic model
For a given disease, there may be several diagnostic markers. Therefore, it is valuable to evaluate whether these diagnostic markers, when used together, can improve the diagnostic accuracy. Some diagnostic models have been proposed to incorporate available diagnostic markers. One of the examples is the Risk of Ovarian Malignancy Algorithm (ROMA) score for ovarian cancer diagnosis in patients with a pelvic mass (18). ROMA score incorporates two widely used ovarian cancer diagnostic markers, cancer antigen 125 (CA125) and human epididymis protein 4 (HE4), with a logistic regression model. The probability of ovarian cancer can be calculated with the logistic regression model, and a ROC curve can be drawn with the probability. If the AUC of the probability is significantly higher than a single test, it means that the diagnostic accuracy of the model is superior to a single test, and combinational use of biomarkers is encouraged.
In addition to a logistic regression model, some novel machine learning approaches can also be used to build a diagnostic model (19-21). However, it should be noted that although machine learning represents an advanced tool in DTA study, it also has some drawbacks, which may limit its clinical applications (22).
Nomograms
A nomogram is usually used to predict the probability of survival in prognostic studies. Some researchers also use it to graphically calculate the probability of a disease for a given patient based on an index test or diagnostic model. In a recently published, prospective, multicenter study regarding the diagnostic accuracy of alpha-fetoprotein (AFP) and PIVKA-II for HCC, the authors constructed a diagnostic nomogram using age, gender, ln(AFP) and ln(PIVKA-II) (23). A nomogram is very straightforward method, and it can be easily interpreted. Put simply, the point of each potential diagnostic parameter and its total points are indicated in the nomogram. The corresponding probability of HCC at a total point can be easily calculated in the nomogram. For example, if a patient is male (approximately 5 points), aged more than 65 years (approximately 20 points), ln(AFP) is 6 (approximately 30 points), and ln(PIVKA-II) is 3 (approximately 20 points), his total point sum is 75, and the corresponding probability of HCC in the nomogram is approximately 80%. An online risk calculator can also be built to facilitate the use of a nomogram, like in the previous study (23).
Net reclassification index (NRI) and integrated discriminatory index (IDI)
NRI and IDI are two widely used statistical methods to estimate whether an investigated diagnostic marker provides additional diagnostic value beyond traditional clinical information (24). For example, in a previous study (25), researchers investigated the diagnostic accuracy of soluble CD146 (sCD146) for HF in acute dyspnea patients visiting ED. Although the researchers proved that sCD146 was a useful diagnostic marker for HF, with an AUC of 0.870 and comparable to that of NT-proBNP, some were skeptical as to whether sCD146 could provide additional diagnostic information beyond NT-proBNP. To address this issue, the researcher used NRI and IDI to analyze the data.
Table 1 is an example of using NRI and IDI to assess the additional diagnostic value of sCD146 beyond NT-proBNP. The methods used to calculate category-NRI, continuous NRI, and IDI were as follows: when NT-proBNP is used alone, the probability of HF can be calculated with a logistic regression model (basic model). Next, another logistic regression model (new model) can be used to incorporate NT-proBNP and sCD146, and another probability can also be calculated. The threshold of the category-NRI is set at 0.20, meaning 0.20 is a threshold to define positive (HF) and negative (non-HF) results. The two probabilities in basic and new models are used to calculate category-NRI, continuous NRI, and IDI in the following fashion.
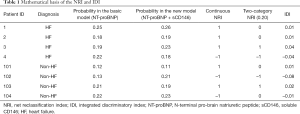
Full table
- IDI is the differential value between these two probabilities. Therefore, for patient 1 with HF, the IDI is 0.26–0.25=0.01. For patient 101 without HF, the IDI is 0.12–0.11=0.01.
- Continuous NRI is used to estimate whether the probability is improved. If the probability has been improved in a new model, continuous NRI is labeled as 1; otherwise, it is labeled as –1. Therefore, for patient 1 with HF, the continuous NRI is 1; while for patient 102 without HF, the continuous NRI is –1.
- Two-category NRI is used to estimate whether the changes of the probabilities exceed the predefined threshold, which here is 0.20. For patient 1 with HF, although the probability in the new model is increased, both of the probabilities in the basic and new model are more than 0.20, indicating that the new model does not improve the diagnostic accuracy of the basic model. Therefore, the two-category NRI is 0. For patient 103 without HF, the probability decreased from 0.21 (higher than 0.20) to 0.19 (lower than 0.20), indicating that this is a non-HF patient who has been misdiagnosed by the basic model and has been correctly diagnosed by the new model. Therefore, the two-category NRI is 1.
Taking IDI as an example, the overall IDI can be calculated using the following formula:
The methods used to calculate continuous or category NRI is similar to IDI. A Z test can be used to detect whether IDI, continuous NRI, or category NRI is significantly higher than 0. IDI and NRI can be easily calculated with an R package PredictABEL (26).
Diagnostic scales
In DTA studies, it is valuable to determine whether a novel diagnostic marker provides additional diagnostic value beyond available conventional information, including signs, symptoms, laboratory tests, microbiological findings, and imaging characteristics. NRI and IDI can be used to address this issue. A basic model can be constructed with conventional parameters, and a new model can be constructed with a basic model and the index test. With these two models, NRI and IDI can be calculated, and whether an index test provides additional diagnostic information can be verified. However, the basic model has two limitations. The first limitation is that the basic model may miss some conventional parameters, and thus may not reflect the overall diagnostic accuracy of the available parameters. The second limitation is the fact that the clinical setting is more complicated than a mathematic model composed of several parameters or variables. The experience or ability of the physicians should be considered when making diagnosis.
To overcome the limitations of NRI and IDI, some researchers use a diagnostic scale to evaluate whether a new test adds additional information beyond conventional clinical information. For example, in a study investigating NT-proBNP for HF diagnosis in dyspnea patients, the researchers asked two physicians to read the medical records of all subjects, including laboratory tests (except NT-proBNP), history, signs, symptoms, and imaging characteristics. Then, these two physicians estimated the likelihood of HF in each patient with a scale from 0 to 100% (27). Scale 0 indicated that the subject definitely did not have HF, and scale 100 indicated that the subject definitely had HF. This scale could reflect the overall diagnostic ability of all available information, as well as the experience or ability of the common physicians in the clinical setting. NRI and IDI were then used to estimate whether NT-proBNP provided additional diagnostic information beyond the scale. Actually, the researchers could also ask other physicians to read all the medical records including those for NT-proBNP and then estimate the likelihood of HF with a new scale. The AUC of these two scales (with or without NT-proBNP) could also be compared to address whether NT-proBNP can improve the diagnostic accuracy of clinicians.
Conclusions
To ascertain the diagnostic accuracy of a biomarker, it is crucial to estimate the diagnostic accuracy of biomarkers in studies using rigorous design. In this review, we summarized the key information for designing a DTA study of biomarkers, and introduced some useful statistical methods. These suggestions and statistical methods may help researchers in designing better DTA studies.
Acknowledgments
None.
Footnote
Conflicts of Interest: The authors have no conflicts of interest to declare.
Ethical Statement: The authors are accountable for all aspects of the work in ensuring that questions related to the accuracy or integrity of any part of the work are appropriately investigated and resolved.
References
- Millot G, Voisin B, Loiez C, et al. The next generation of rapid point-of-care testing identification tools for ventilator-associated pneumonia. Ann Transl Med 2017;5:451. [Crossref] [PubMed]
- Potocki M, Breidthardt T, Reichlin T, et al. Comparison of midregional pro-atrial natriuretic peptide with N-terminal pro-B-type natriuretic peptide in the diagnosis of heart failure. J Intern Med 2010;267:119-29. [Crossref] [PubMed]
- Rutjes AW, Reitsma JB, Vandenbroucke JP, et al. Case-control and two-gate designs in diagnostic accuracy studies. Clin Chem 2005;51:1335-41. [Crossref] [PubMed]
- Whiting P, Rutjes AW, Reitsma JB, et al. Sources of variation and bias in studies of diagnostic accuracy: a systematic review. Ann Intern Med 2004;140:189-202. [Crossref] [PubMed]
- Fu F, Zeng F, Sun Z, et al. Head-to-head comparison of serum and urine cytokeratin-19 fragments (CYFRA 21-1) for bladder cancer diagnosis. Transl Cancer Res 2018;7:55-9. [Crossref]
- Hu ZD. Circulating biomarker for malignant pleural mesothelioma diagnosis: pay attention to study design. J Thorac Dis 2016;8:2674-76. [Crossref] [PubMed]
- Aletaha D, Neogi T, Silman AJ, et al. 2010 rheumatoid arthritis classification criteria: an American College of Rheumatology/European League Against Rheumatism collaborative initiative. Ann Rheum Dis 2010;69:1580-8. [Crossref] [PubMed]
- Levi M, Toh CH, Thachil J, et al. Guidelines for the diagnosis and management of disseminated intravascular coagulation. British Committee for Standards in Haematology. Br J Haematol 2009;145:24-33. [Crossref] [PubMed]
- Maisel A, Mueller C, Nowak R, et al. Mid-region pro-hormone markers for diagnosis and prognosis in acute dyspnea: results from the BACH (Biomarkers in Acute Heart Failure) trial. J Am Coll Cardiol 2010;55:2062-76. [Crossref] [PubMed]
- Leeflang MM, Moons KG, Reitsma JB, et al. Bias in sensitivity and specificity caused by data-driven selection of optimal cutoff values: mechanisms, magnitude, and solutions. Clin Chem 2008;54:729-37. [Crossref] [PubMed]
- Tang YF, Li W, Yuan JP, et al. Diagnostic values of serum tumor markers CA72-4, SCCAg, CYFRA21-1, NSE, AFU, CA125, CA19-9, CEA and FER in nasopharyngeal carcinoma. Transl Cancer Res 2018;7:1406-12. [Crossref]
- Pepe MS, Janes H, Li CI, et al. Early-Phase Studies of Biomarkers: What Target Sensitivity and Specificity Values Might Confer Clinical Utility? Clin Chem 2016;62:737-42. [Crossref] [PubMed]
- Chen S, Zhou C, Liu W, et al. Methylated septin 9 gene for noninvasive diagnosis and therapy monitoring of breast cancer. Transl Cancer Res 2018;7:587-99. [Crossref]
- Wan J, Su J, Ye Z, et al. Diagnostic performance of protein induced by vitamin K absence II for chronic hepatitis B-related hepatocellular carcinoma. J Lab Precis Med 2019;4:10. [Crossref]
- Vickers AJ, Elkin EB. Decision curve analysis: a novel method for evaluating prediction models. Med Decis Making 2006;26:565-74. [Crossref] [PubMed]
- Han YQ, Zhang L, Wang JR, et al. Net benefit of routine urine parameters for urinary tract infection screening: a decision curve analysis. Ann Transl Med 2019. [Crossref]
- Vickers AJ, van Calster B, Steyerberg EW. A simple, step-by-step guide to interpreting decision curve analysis. Diagn Progn Res 2019;3:18. [Crossref] [PubMed]
- Moore RG, McMeekin DS, Brown AK, et al. A novel multiple marker bioassay utilizing HE4 and CA125 for the prediction of ovarian cancer in patients with a pelvic mass. Gynecol Oncol 2009;112:40-6. [Crossref] [PubMed]
- Hoffmann G, Bietenbeck A, Lichtinghagen R, et al. Using machine learning techniques to generate laboratory diagnostic pathways—a case study. J Lab Precis Med 2018;3:58. [Crossref]
- Luo Y, Szolovits P, Dighe AS, et al. Using machine learning to predict laboratory test results. Am J Clin Pathol 2016;145:778-88. [Crossref] [PubMed]
- Naugler C, Church DL. Automation and artificial intelligence in the clinical laboratory. Crit Rev Clin Lab Sci 2019;56:98-110. [Crossref] [PubMed]
- Lippi G. Machine learning in laboratory diagnostics: valuable resources or a big hoax? Diagnosis (Berl) 2019. [Epub ahead of print].
- Yang T, Xing H, Wang G, et al. A novel online calculator based on serum biomarkers to detect hepatocellular carcinoma among patients with hepatitis B. Clin Chem 2019. [Epub ahead of print]. [Crossref] [PubMed]
- Pencina MJ, D’Agostino RB Sr, D'Agostino RB Jr, et al. Evaluating the added predictive ability of a new marker: from area under the ROC curve to reclassification and beyond. Stat Med 2008;27:157-72; discussion 207-12. [Crossref] [PubMed]
- Gayat E, Caillard A, Laribi S, et al. Soluble CD146, a new endothelial biomarker of acutely decompensated heart failure. Int J Cardiol 2015;199:241-7. [Crossref] [PubMed]
- Kundu S, Aulchenko YS, van Duijn CM, et al. PredictABEL: an R package for the assessment of risk prediction models. Eur J Epidemiol 2011;26:261-4. [Crossref] [PubMed]
- Januzzi JL Jr, Camargo CA, Anwaruddin S, et al. The N-terminal Pro-BNP investigation of dyspnea in the emergency department (PRIDE) study. Am J Cardiol 2005;95:948-54. [Crossref] [PubMed]