Association of air pollution and fine particulate matter (PM2.5) exposure with gestational diabetes: a systematic review and meta-analysis
Highlight box
Key findings
• AP and PM exposure are associated with GDM incidence.
What is known and what is new?
• Environmental pollution is associated with many chronic diseases of pregnancy.
• Our study confirmed that there is a significant correlation between environmental pollution exposure before and during pregnancy and the occurrence of diabetes in pregnancy
What is the implication, and what should change now?
• Pregnant women or women with birth plans should consider the value of a favorable living environment.
Introduction
Gestational diabetes mellitus (GDM) refers to diabetes diagnosed during the second or third trimester of pregnancy, with no previous diabetic history (1). GDM is a dangerous and often-ignored condition that endangers the health of pregnant women and their children. It is closely associated with significant increases in neonatal and maternal mortality, with a morbidity of approximately 16.7% (1). Pregnancy-related complications, such as hypertension, an overweight baby, and difficult delivery, are prevalent among women with GDM (2). Type-2 diabetes occurs in nearly 50% of women with GDM history within 5–10 years after delivery, especially after the age 35 years (3,4). In addition, GDM increases the risk for cardiovascular diseases and renal diseases (5), and to some extent increases the risk of gestational hyperglycemia and postpartum depression (6). When the blood sugar levels of pregnant women rise, excess sugar easily crosses the placenta, inducing hyperglycemia in the fetus, which can lead to delays in fetal lung maturation and dyspnea syndrome after birth. At the same time, the fetus becomes prone to accumulate too much sugar and consume more oxygen, which can lead to fetal hypoxia. Excess sugar will be converted to fat in the fetus, causing the fetus to gain weight and develop macrosomia (7-9). Studies have shown that various factors contribute to the development of GDM, such as body mass index (BMI) during pregnancy, low education levels, low family socioeconomic status, and so on. In recent years, the association between GDM and environmental exposure (PM2.5 and noise) during pregnancy has attracted wide attention from researchers (10).
Fine particulate matter (PM2.5) refers to inhalable particulate matter in the atmosphere, with an aerodynamic equivalent diameter less than 2.5 µg. It represents a current hotspot in the research of air pollution (AP). Its major constituents include various pollutants, water-soluble salt ions, heavy metals, and so on (11). Due to the small volume, PM2.5 in the bronchiole deposited on the wall influences gas exchange in the lungs, and some finer PM2.5 alveolar components can penetrate the blood, overflow distribution, and damage other parts of the body. It is also capable of inducing oxidative stress, endothelial dysfunction, and inflammatory response, and these pathogenic processes subsequently cause insulin resistance, a link that is especially significant in women (12).
A previous systematic review has demonstrated the association between AP and diabetes (13). Nevertheless, the association between AP and GDM, especially the link between different pollutants and GDM, remains highly contentious. Recent reviews reported controversial findings, probably due to the limited number of included studies (14,15). In the conclusions of these two studies, there are contradictions about the correlation between PM2.5, NOx and GDM. In addition, for O3, BC and CO, due to the limited amount of literature, no corresponding conclusions have been given. Therefore, we conducted this systematic review and meta-analysis to evaluate the association of AP and PM2.5 exposure with GDM, so as to provide an evidence-based reference for its clinical prevention. We present the following article in accordance with the PRISMA reporting checklist (available at https://atm.amegroups.com/article/view/10.21037/atm-22-6306/rc).
Methods
This study has been pre-registered on International Platform of Registered Systematic Review and Meta-analysis Protocols (registration No. INPLASY202290123).
Search strategy
The databases of PubMed, Cochrane Library, Embase, and Web of Science were systematically searched from inception to 1 April 2022, using the combination of medical subject headings (MeSH). Search items mainly contained Diabetes, Gestational (MeSH), Air Pollution (MeSH), Particulate Matter (MeSH).
Inclusion and exclusion criteria
Studies meeting the following criteria were considered eligible for inclusion:
- P (Populations): pregnant women.
- E (Exposure): exposure to air pollution during pregnancy, including different air pollution components, such as PM2.5, PM10, NOx, O3, etc.
- C (Control): pregnant women not exposed to air pollution.
- O (Outcome): after controlling the confounding factors, the OR value of the correlation between air pollution and GDM calculated by logistic regression or multifactor logistic regression.
- S (Study design): the types of included studies were cohort study, cross-sectional study, case control study and propensity matching study.
The exclusion criteria were as follows:
- P (Populations): pregnant women who did not record the outcome of GDM in detail.
- E (Exposure): there are no studies on environmental exposure components during pregnancy.
- C (Control): none.
- O (Outcome): only univariate analysis of the relationship between environmental exposure and GDM, and studies only evaluating the association of reduced exposure with changed diabetes status and improved birth outcomes.
- S (Study design): (I) when analyzing the relationship between air exposure and GDM, the logistic regression of multiple factors or the logistic regression that controls the confounding factors is used. However, when the number of samples is too small, the stability of the regression coefficient is questioned. Therefore, we need to exclude studies with less than 50 sample descriptions. (II) No full text of the meeting summary.
Study selection and data extraction
Study selection and data extraction were processed by 2 researchers independently, and disagreements were resolved via group discussion. All retrieved articles were imported into Endnote (Clarivate, London, UK). Duplicates were removed, titles and abstracts were browsed, and full-texts of the potential eligible studies were read.
Data were extracted using a pre-designed standardized extraction that included a basic linear table and different pollutants and the concentration of fine particulate matter on the influence of the GDM data extraction table, which included the following information: (I) authors, publication year, the author’s area and study period; (II) sampling mechanism; (III) study design; (IV) study location; (V) exposure (study of pollutants and fine particles and their concentrations); (VI) sample size; (VII) type of exposure (continuous or categorical); (VIII) covariate adjustment and the OR value of logistic regression for the association between AP and GDM after covariate adjustment; and (IX) diagnostic criteria for GDM.
The literature screening and data extraction were performed by two independent researchers, and the results were cross-checked. If there were any dissents, a third researcher was consulted to assist in the final decision.
Quality assessment
The Newcastle-Ottawa Scale (NOS) was adopted for the quality assessment of included cohort studies and case-control studies. The NOS scale is designed by Wells et al. (16). and is used for methodological quality assessment of non-randomized studies. The NOS scale scores articles according to 8 questions in 3 domains, with a total score of 9. A study with a score of 0–3 was rated as low quality; a study with a 4–6 score was considered as moderate quality; and a study with a 7–9 score was graded as high quality (17). In contrast, the risk of bias in the included cross-sectional studies was assessed using the Joana Brigg’s Institute (JBI) critical appraisal checklist, with a total score of nine. This scale contains nine signaling questions, and each question can be answered as yes, no, unclear, or not applicable. Based on this, individual studies were assigned a score according to the review objectives. The responses were scored 0 for “not appropriate, not reported, or unclear” and 1 for “yes” (18).
Two researchers independently performed the quality assessment of the included studies and cross-checked their results. If there were any dissents, a third researcher was consulted to assist in the final decision.
Statistical analysis
Effect size selection
The included studies comprised several cohort studies, 1 cross-sectional study, and 1 case-control study. Although cohort studies were in the majority, these studies adopted logistic regression or multivariate logistic regression after covariate adjustment to analyze the association between AP and GDM, and calculated the OR value as the effect size. Thus, the effect size in our systematic review was the OR value.
Model selection
Model selection was chosen based on the heterogeneity. Data were combined using a random-effect model if an I2 was greater than 50%, otherwise, a fixed-effect model would be used.
Combined effect size results
The effect estimate would be aggregated if 2 studies in the extracted data reported the same pollutants and fine particles at the same gestational stage as the exposure window group. In this meta-analysis, we calculated 2 types of pooled effect estimates: (I) increased GDM risk per unit of persistent pollutants and fine particulate matter; and (II) combined multiple-effect estimates from the same study at the same gestational stage (1). If exposure estimates were not in mass/volume units, they were converted (PPB and PPM) to uniform units µg/m3 using the method provided by Malmqvist.
The heterogeneity
- Heterogeneity (I2): mixed OR values were also presented at different exposure windows, and the heterogeneity of result analysis for each effective pollutant and fine particulate matter was assessed using Cochran’s q-test and Higgins I2 statistic.
- Exploring the sources of heterogeneity: Subgroup analysis: The included literature reported the effect estimates of different pregnancy stages, different pollutants, and fine particles. In terms of time, subgroup analysis was conducted for the same pollutant and different periods of fine particles; in terms of pollutant types, subgroup analysis was conducted for all pollutants except for PM2.5. We believed that multiple concentrations might be the source of heterogeneity based on the inclusion of 35 studies with a large volume of data and inconsistent concentrations, therefore we performed meta-regression.
Publication bias
- Publication bias was tested via Begg’s and Egger’s test, and a funnel plot was provided.
- To deal with generated publication bias, we planned to use the shear and complement method.
A P<0.05 indicated a statistical difference.
Results
Study selection
Our search strategy yielded 714 studies, of which 656 were excluded after initial screening and removing duplicates. The remaining 58 studies were evaluated in accordance with the summary. Following that, 54 full-text studies were retrieved for detailed evaluation. Within these articles, 11 studies had assessed the effects of GDM on the fetus, including traffic-related AP and fine particulate matter indicators, 6 assessments of fasting blood sugar and glucose stable/metabolic results, 1 study provided results from the same number of results, and 1 study assessed the impact of green space on gestational diabetes. Therefore, we report the remaining 35 studies on AP and fine particles in the meta-analysis. Detailed study selection process is provided in Figure 1.
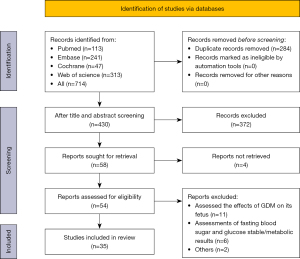
Study characteristics and quality assessment
A total of 17 studies were conducted in China (19-35), 12 studies in the United States (11,36-46), 1 study in Korea (47), 1 study in Sweden (48), and 2 studies in Australia (49,50) , and 2 studies in Denmark (10,51). Almost all of the studies were cohort studies with 35 studies, 1 was a cross-sectional study (34), and the remaining 1 was a case control (35).
The included studies involved pregnant women who were exposed to AP and fine particulate matter before and during their pregnancy, but the review only included studies on gestational diabetes. In terms of GDM diagnostic criteria, the World Health Organization (WHO) approach was utilized in the majority of research, and the WHO and the authors’ own diagnostic procedures for GDM were employed in a few others (10).
All included studies used data from professional institutions’ medical records or birth assessments and follow-up records, except for 1 that used self-reported results (37).
Furthermore, the methodological quality of included studies was assessed using NOS (17), and all were scored over 7, indicating these studies were of high-quality. Detailed study characteristics and quality assessment are provided in https://cdn.amegroups.cn/static/public/atm-22-6306-1.xlsx.
Outcome measurements and prevalence: GDM
The definition of GDM is shown in the basic linear table (https://cdn.amegroups.cn/static/public/atm-22-6306-1.xlsx). The definition of GDM varied according to the study area. A total of 15 studies used the diagnostic standard of GDM of American Diabetes Association (10,11,25,36-47): GDM was diagnosed according to a serum glucose level greater than 200 mg/dL in a glucose challenge test, or at least 2 measurements of serum glucose level reaching or exceeding the following values in 100 g or 75 g oral glucose tolerance test (OGTT): fasting, 95 mg/dL; 1 hour, 180 mg/dL; 2 hours, 155 mg/dL; and 3 hours, 140 mg/dL, as previously described.
A total of 8 studies used the GDM diagnostic criteria released by the Chinese Diabetes Association (19-24,33,34), in which the diagnosis was mainly based on OGTT: (I) fasting blood glucose over 5.1 mmol/L, (II) blood glucose over 5.1 mmol/L at baseline, 1-hour blood glucose over 10.0 mmol/L, or 2-hour blood glucose over 8.5 mmol/L (17). A total of 9 studies used the standard of international Gestational Diabetes Association (18,23,25,27,29,30,33,41,42), in which GDM is defined as fasting blood glucose greater than 5.1 mmol/L, blood glucose greater than 10 mmol/L after 1 hour, or blood glucose greater than 8.5 mmol/L after 2 hours (20).
One study conformed with the diagnostic criteria released by World Health Organization (34): a fasting venous blood glucose over 7.0 mmol/L with or without a 120-minute value over 7.8 mmol/L in 75 g OGTT.
One study conformed with the Danish criteria (10): more than 1 measurement of venous blood glucose exceeded 6.2 mmol/L at 0 minutes, 10.9 mmol/L at 30 minutes, 11.1 mmol/L at 60 minutes, 9.2 mmol/L at 90 minutes, 8.9 mmol/L at 120 minutes, 8.2 mmol/L at 150 minutes, and 7.3 mmol/L at 180 minutes in OGTT.
AP exposure
Most studies had used the routine monitoring data of air as a measurement basis, then used the environment model [12 studies used the regression model of land use (19,22,25,30,36-38,41,43,45,47,48), 8 studies used the satellite remote sensing model (20,21,23,24,30,31,49,50), and the remaining 15 used discrete or novel models] to assign data to maternal residential address during delivery, and 6 studies defined exposure based on patients’ residential address at the city/town level (27,28,33,34,39,40). A total of 5 studies further considered residential mobility (10,11,32,44,46) and collected historical residential address information during pregnancy. Exposure indices usually varied from study to study based on the distance between the nearest monitoring station and the current residential address and the number and density of monitors. A total of 35 studies investigated PM2.5 and PM10, as well as nitrogen dioxide (NO2), nitrogen oxides (NOX), ozone (O3), black carbon (BC), carbon monoxide (CO), sulfur dioxide (SO2), and nitric oxide (NO) (https://cdn.amegroups.cn/static/public/atm-22-6306-1.xlsx), and 4 studies required us to extract the results in a graph (30,34,40,50).
Combined effects of AP and fine particulate matter on the risk of GDM
Table 1 presents a pooled effect estimate for air pollutants and fine particulate matter. Most included studies assessed the association between repeated exposure to air pollutants and fine particulate matter and GDM. In terms of fine particulate matter exposure, exposure to PM2.5, PM10, and BC all had an effect on the occurrence of GDM. PM10 and BC had little effect in the first trimester, but the estimated effect of BC exposure on GDM in the first trimester also reached a critical level of statistical significance [OR =1.05, 95% confidence interval (CI): 0.99–1.11]. Exposure to air pollutants, including SO2, NOX, and NO, has been shown to influence the incidence of GDM. Due to the lack of research on NOX and NO2 in the included studies, it could only be stated that exposure to NOX during the first and second trimesters had some influence on GDM, and exposure to NO during the first trimester had an effect on GDM.
Table 1
Pollutant and exposure window combination | Number of studies | OR (95% CI) | I2 (%) |
---|---|---|---|
PM2.5 | 1.06 (1.05–1.08) | 88.8 | |
Preconception | 6 | 1.09 (1.05–1.14) | 93.0 |
Trimester 1 | 17 | 1.05 (1.01–1.08) | 88.8 |
Trimester 2 | 20 | 1.07 (1.03–1.10) | 85.9 |
Entire pregnancy | 10 | 1.06 (1.02–1.11) | 80.6 |
PM10 | 1.06 (1.01–1.11) | 90.3 | |
Preconception | 4 | 1.09 (1.00–1.19) | 91.6 |
Trimester 1 | 6 | 0.99 (0.95–1.03) | 63.7 |
Trimester 2 | 5 | 1.10 (0.89–1.37) | 88.7 |
Entire pregnancy | 3 | 1.13 (1.03–1.23) | 40.1 |
NO2 | 0.99 (0.96–1.03) | 93.9 | |
Preconception | 3 | 1.05 (0.95–1.16) | 90.6 |
Trimester 1 | 8 | 1.00 (0.96–1.04) | 81.7 |
Trimester 2 | 7 | 0.96 (0.90–1.02) | 87.4 |
Entire pregnancy | 5 | 0.97 (0.86–1.09) | 98.3 |
SO2 | 1.18 (1.10–1.26) | 92.7 | |
Preconception | 4 | 1.19 (1.03–1.39) | 95.2 |
Trimester 1 | 6 | 1.19 (1.06–1.35) | 94.0 |
Trimester 2 | 5 | 1.18 (0.98–1.42) | 92.6 |
Entire pregnancy | 3 | 1.11 (0.92–1.34) | 73.0 |
O3 | 1.00 (0.94–1.05) | 98.5 | |
Preconception | 4 | 0.99 (0.94–1.05) | 89.6 |
Trimester 1 | 7 | 1.03 (0.97–1.09) | 95.9 |
Trimester 2 | 5 | 1.00 (0.91–1.09) | 95.0 |
Entire pregnancy | 4 | 0.92 (0.73–1.15) | 99.6 |
BC | 1.08 (1.06–1.10) | 83.8 | |
Trimester 1 | 3 | 1.05 (0.99–1.11) | 69.2 |
Trimester 2 | 4 | 1.06 (1.01–1.12) | 62.6 |
Entire pregnancy | 3 | 1.13 (1.05–1.20) | 92.0 |
NO | 1.04 (1.03–1.06) | 0.0 | |
Trimester 2 | 2 | 1.05 (1.02–1.08) | 0.0 |
NOX | 1.07 (1.04–1.11) | 89.3 | |
Trimester 1 | 2 | 1.03 (1.00–1.07) | 64.1 |
Trimester 2 | 3 | 1.35 (1.05–1.75) | 93.3 |
CO | 1.00 (0.96–1.04) | 77.7 | |
Preconception | 2 | 1.02 (0.96–1.08) | 34.6 |
Trimester 1 | 3 | 0.99 (0.91–1.07) | 87.8 |
Trimester 2 | 3 | 1.01 (0.89–1.15) | 84.6 |
OR, odds ratio; CI, confidence interval; NO2, nitrogen dioxide; SO2, sulfur dioxide; O3, ozone; BC, black carbon; NO, nitric oxide; NOx, nitrogen oxides; CO, carbon monoxide.
Overall impact estimates for certain pollutants (O3, CO, NO2) did not show any association with GDM. When analyzing data, we used the random effect model to unify pollutant concentration units. The main research object (PM2.5) and funnel plot were cut and supplemented in meta-regression to compensate for possible publication bias owing to concentration differences. A supplementary method was employed (see Figure 2) and after the supplement of 13 literatures, the result of adjustment was OR =1.03 (95% CI: 1.01–1.04), which still had an impact on GDM.
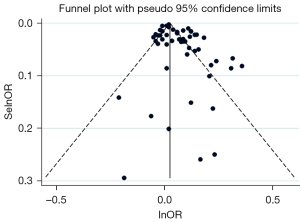
Subgroup analysis
The 35 included studies were divided into 9 groups based on different fine particles and pollutants, whereas pregnant women were separated into 4 groups based on pregnancy stage. The results are shown in Table 1. The subgroup analysis was performed when the number of studies exposed to the same pollutants and at the same stage of pregnancy was ≥2.
Sensitivity analysis
Sensitivity analysis was processed via removing included studies one by one. Given the significant heterogeneity existing among the studies, the random-effect model was applied. Removal of each study failed to reverse the results indicating the robustness of the results.
Publication bias
Begg’s funnel plot was produced to reveal the potential publication bias, which was tested by Egger’s linear regression. The results showed that publication bias should be considered (P<0.05), which might be unavoidable due to the considerable variation in the concentration of the included studies. Therefore, funnel plot (PM2.5) and meta-regression (see Figure 2) were conducted for concentration, and the results showed that the adjusted result of the shear-complement method was OR =1.03 (95% CI: 1.01–1.05).
Discussion
With rapid economic development, air pollutants and fine particulate matter have become serious threats to human health. AP and fine particulate matter are believed to cause multiple metabolic disturbances, such as autonomic nervous dysfunction, cellular oxidative stress, inflammation response, endoplasmic reticulum stress, cellular apoptosis, and glucose metabolic disorder (52,53). Decreased insulin sensitivity, impaired insulin secretion, and elevated serum lipid concentration provide a biological rationale for the association between pollutant exposure and diabetes risk. GDM is related to impaired glucose tolerance (IGT) and has many similarities with diabetes (54,55), suggesting that many of the mechanisms underlying diabetes can be equally applied to GDM.
Previous epidemiological studies have investigated the effect of AP on chronic diseases like diabetes and hypertension (13), and some articles have also explored the association of air pollutants with GDM, but the results have been inconsistent and controversial. Outdoor AP and GDM: there were only 1 or 2 studies for each type of air pollutant in a systematic review and meta-analysis of 8 studies. The authors concluded that exposure to SO2, NO, and NOx was associated with an increased risk of GDM. However, the pooled effect estimates were derived from effect estimates reported separately at 3 months in the original study and did not consider different stages of pregnancy (14). Epidemiological evidence regarding the effect of AP exposure on GDM occurrence: systematic review and meta-analysis: a total of 11 articles were included in this paper. The authors concluded that the association between PM2.5 and GDM was explained to some extent, while other pollutants (NO2, O3, NO, CO, NOx, SO2) failed to reveal any association with GMD. Compared with the previous paper, this study compared the effect estimates of high pollution exposure and low pollution exposure to air pollutants (15). Departures from linearity in the association between air pollutant-exposure and GDM have been reported in previous studies (41,48), Therefore, in this study, pollution concentration of all studies was analyzed and meta-regression was performed based on concentration as a variable. Concentration variables were not evaluated in the previous meta-analysis.
We searched 4 major databases for this analysis, and 35 studies with varying characteristics were included, yielding a total sample size of 6,939,725. Limited sample size is usually statistically sensitive to extremums, which might lead to statistical inferences that differ from the actual situation. The sampling error would decrease with the increase of sample size, making the inference more reliable. Therefore, all the studies included in this meta-analysis had large sample, and most of the studies initially excluded women with chronic metabolic disease, kidney disease, or had a history of diabetes, which helped to assess the net-effects. On the other hand, subgroup analysis could identify the potential sources of heterogeneity so as to improve the statistical performance. The final results showed that exposure to PM2.5, PM10, SO2, O3, BC, NO, and NOX had some correlation with GDM, but did not show any correlation for populations exposed to NO2, O3, and CO. Different adjustment models used in the included studies led to inconsistent OR values, with statistically significant differences, suggesting that these factors (BMI, race, education level, age, smoking, etc.) may be potential confounding factors.
The heterogeneity among included studies might be caused by variances in study-design, exposures, and outcome definitions. First, most of the studies were retrospective-design, leading to recall bias, and several studies used classified exposure as an independent variable, while only the ORs and 95% CIs of sustained exposures were extracted and analyzed. Second, each study used different methods and instruments to measure exposure to air pollutants. Most of the land-use models used in the study used data from central AP monitoring stations, which has some limitations. Due to the obvious limited spatial coverage, monitoring data mostly reflect changes in pollutant levels over time, resulting in selection bias. Third, considering the exposure to different concentrations of AP, although the final result revealed some pollutants related to GDM, exposure to varying concentrations and risk of GDM will result in a deviation. As a result, we propose that future researchers should concentrate on the study of AP and fine particulate matter concentrations that can cause GDM. Fourth, there were differences in confounding factors. Different studies used different adjustment models and included different populations. The inadequate adjustment might contribute to bias if not correctly assessed. The data of each study were adjusted for factors such as the mean age, BMI, education level, race, and smoking history of the participants. The adjustment factors varied from study to study, which could lead to information bias. Studies have shown that living in a green space with higher environmental standards may reduce the incidence of GDM (45), whereas living near the main road, traffic density, or population density, may heighten the risk of GDM. Although not directly addressing the GDM and the relationship between AP, it is usually considered a substitute for AP and fine particulate matter (56,57). However, traffic pollution, as a rough estimate of air quality, cannot determine the precise level of individual exposure. In addition, exposure to household air pollution (HAP) from cooking with biomass fuels and burning garbage at home has been linked to an increase in GDM in pregnant women. Cooking and burning waste with biomass fuel can also be seen as another way to release harmful gases and fine particles (58).
Our results suggest that exposure to air pollutants is a significant risk factor for GDM. However, given the differences in AP and fine particulate concentrations in the study, the conclusion needs to be interpreted prudently, since AP is more severe in developing countries, where most of the GDM cases were reported. It is advised that further cohort studies be undertaken in developing countries to explore further associations.
Conclusions
This meta-analysis suggests that exposure to certain air pollutants and fine particulate matter in pregnant women may be associated with an increased risk of GDM. Although the effect of air pollutants and fine particulate matter on GDM is uncertain, it cannot be ignored considering the large sample size included in this meta-analysis and many studies conducted in developing countries. In addition, more studies, more comprehensive interventions, and better research methods are needed in subsequent studies because some pollutant studies are unable to identify GDM exposure.
In this regard, we recommend that pregnant women should avoid direct exposure to AP, protect themselves outside, and avoid oil smoke and gas produced by the burning of biomass materials at home. At the same time, we feel that continuing efforts to protect the environment are necessary for the entire society.
Acknowledgments
Funding: None.
Footnote
Reporting Checklist: The authors have completed the PRISMA reporting checklist. Available at https://atm.amegroups.com/article/view/10.21037/atm-22-6306/rc
Conflicts of Interest: All authors have completed the ICMJE uniform disclosure form (available at https://atm.amegroups.com/article/view/10.21037/atm-22-6306/coif). The authors have no conflicts of interest to declare.
Ethical Statement: The authors are accountable for all aspects of the work in ensuring that questions related to the accuracy or integrity of any part of the work are appropriately investigated and resolved.
Open Access Statement: This is an Open Access article distributed in accordance with the Creative Commons Attribution-NonCommercial-NoDerivs 4.0 International License (CC BY-NC-ND 4.0), which permits the non-commercial replication and distribution of the article with the strict proviso that no changes or edits are made and the original work is properly cited (including links to both the formal publication through the relevant DOI and the license). See: https://creativecommons.org/licenses/by-nc-nd/4.0/.
References
-
.Diabetes Atlas IDF 2021 . Available online: https://diabetesatlas.org/ - Moe K, Sugulle M, Dechend R, et al. Risk prediction of maternal cardiovascular disease one year after hypertensive pregnancy complications or gestational diabetes mellitus. Eur J Prev Cardiol 2020;27:1273-83. [Crossref] [PubMed]
- He J, Chen X, Wang Y, et al. The experiences of pregnant women with gestational diabetes mellitus: a systematic review of qualitative evidence. Rev Endocr Metab Disord 2021;22:777-87. [Crossref] [PubMed]
- Diaz-Santana MV, O'Brien KM, Park YM, et al. Persistence of Risk for Type 2 Diabetes After Gestational Diabetes Mellitus. Diabetes Care 2022;45:864-70. [Crossref] [PubMed]
- Rawal S, Olsen SF, Grunnet LG, et al. Gestational Diabetes Mellitus and Renal Function: A Prospective Study With 9- to 16-Year Follow-up After Pregnancy. Diabetes Care 2018;41:1378-84. [Crossref] [PubMed]
- Clark CE, Rasgon NL, Reed DE 2nd, et al. Depression precedes, but does not follow, gestational diabetes. Acta Psychiatr Scand 2019;139:311-21. [Crossref] [PubMed]
- Koos BJ, Gornbein JA. Early pregnancy metabolites predict gestational diabetes mellitus: implications for fetal programming. Am J Obstet Gynecol 2021;224:215.e1-7. [Crossref] [PubMed]
- Liu B, Cai J, Xu Y, et al. Early Diagnosed Gestational Diabetes Mellitus Is Associated With Adverse Pregnancy Outcomes: A Prospective Cohort Study. J Clin Endocrinol Metab 2020;105:dgaa633. [Crossref] [PubMed]
- Wu Y, Liu B, Sun Y, et al. Association of Maternal Prepregnancy Diabetes and Gestational Diabetes Mellitus With Congenital Anomalies of the Newborn. Diabetes Care 2020;43:2983-90. [Crossref] [PubMed]
- Pedersen M, Olsen SF, Halldorsson TI, et al. Gestational diabetes mellitus and exposure to ambient air pollution and road traffic noise: A cohort study. Environ Int 2017;108:253-60. [Crossref] [PubMed]
- Rammah A, Whitworth KW, Symanski E. Particle air pollution and gestational diabetes mellitus in Houston, Texas. Environ Res 2020;190:109988. [Crossref] [PubMed]
- Eze IC, Hemkens LG, Bucher HC, et al. Association between ambient air pollution and diabetes mellitus in Europe and North America: systematic review and meta-analysis. Environ Health Perspect 2015;123:381-9. [Crossref] [PubMed]
- Bai W, Li Y, Niu Y, et al. Association between ambient air pollution and pregnancy complications: A systematic review and meta-analysis of cohort studies. Environ Res 2020;185:109471. [Crossref] [PubMed]
- Elshahidi MH. Outdoor Air Pollution and Gestational Diabetes Mellitus: A Systematic Review and Meta-Analysis. Iran J Public Health 2019;48:9-19. [Crossref] [PubMed]
- Hu CY, Gao X, Fang Y, et al. Human epidemiological evidence about the association between air pollution exposure and gestational diabetes mellitus: Systematic review and meta-analysis. Environ Res 2020;180:108843. [Crossref] [PubMed]
- Wells G, editor. The Newcastle-Ottawa Scale (NOS) for Assessing the Quality of Non-Randomised Studies in Meta-Analyses. Symposium on Systematic Reviews: Beyond the Basics; 2014.
- Chen Q, Zhu K, Liu X, et al. The Protection of Naturally Acquired Antibodies Against Subsequent SARS-CoV-2 Infection: A Systematic Review and Meta-Analysis. Emerg Microbes Infect 2022;11:793-803. [Crossref] [PubMed]
- Di Toro F, Gjoka M, Di Lorenzo G, et al. Impact of COVID-19 on maternal and neonatal outcomes: a systematic review and meta-analysis. Clin Microbiol Infect 2021;27:36-46. [Crossref] [PubMed]
- Chen G, Sun X, Wang J, et al. The association between maternal exposure to fine particulate matter (PM2.5) and gestational diabetes mellitus (GDM): a prospective birth cohort study in China. Environ Res Lett 2021;16:055004. [Crossref]
- Cheng X, Ji X, Yang D, et al. Associations of PM(2.5) exposure with blood glucose impairment in early pregnancy and gestational diabetes mellitus. Ecotoxicol Environ Saf 2022;232:113278. [Crossref] [PubMed]
- Hehua Z, Yang X, Qing C, et al. Dietary patterns and associations between air pollution and gestational diabetes mellitus. Environ Int 2021;147:106347. [Crossref] [PubMed]
- Hu Q, Wang D, Yue D, et al. Association of ambient particle pollution with gestational diabetes mellitus and fasting blood glucose levels in pregnant women from two Chinese birth cohorts. Sci Total Environ 2021;762:143176. [Crossref] [PubMed]
- Kang J, Liao J, Xu S, et al. Associations of exposure to fine particulate matter during pregnancy with maternal blood glucose levels and gestational diabetes mellitus: Potential effect modification by ABO blood group. Ecotoxicol Environ Saf 2020;198:110673. [Crossref] [PubMed]
- Liao J, Chen X, Xu S, et al. Effect of residential exposure to green space on maternal blood glucose levels, impaired glucose tolerance, and gestational diabetes mellitus. Environ Res 2019;176:108526. [Crossref] [PubMed]
- Lin Q, Zhang S, Liang Y, et al. Ambient air pollution exposure associated with glucose homeostasis during pregnancy and gestational diabetes mellitus. Environ Res 2020;190:109990. [Crossref] [PubMed]
- Liu WY, Lu JH, He JR, et al. Combined effects of air pollutants on gestational diabetes mellitus: A prospective cohort study. Environ Res 2022;204:112393. [Crossref] [PubMed]
- Mai D, Xu C, Lin W, et al. Association of abnormal-glucose tolerance during pregnancy with exposure to PM(2.5) components and sources. Environ Pollut 2022;292:118468. [Crossref] [PubMed]
- Pan SC, Huang CC, Lin SJ, et al. Gestational diabetes mellitus was related to ambient air pollutant nitric oxide during early gestation. Environ Res 2017;158:318-23. [Crossref] [PubMed]
- Yao M, Liu Y, Jin D, et al. Relationship betweentemporal distribution of air pollution exposure and glucose homeostasis during pregnancy. Environ Res 2020;185:109456. [Crossref] [PubMed]
- Ye B, Zhong C, Li Q, et al. The Associations of Ambient Fine Particulate Matter Exposure During Pregnancy With Blood Glucose Levels and Gestational Diabetes Mellitus Risk: A Prospective Cohort Study in Wuhan, China. Am J Epidemiol 2020;189:1306-15. [Crossref] [PubMed]
- Zhang H, Dong H, Ren M, et al. Ambient air pollution exposure and gestational diabetes mellitus in Guangzhou, China: A prospective cohort study. Sci Total Environ 2020;699:134390. [Crossref] [PubMed]
- Zhang H, Zhao Y. Ambient air pollution exposure during pregnancy and gestational diabetes mellitus in Shenyang, China: a prospective cohort study. Environ Sci Pollut Res Int 2021;28:7806-14. [Crossref] [PubMed]
- Zhang M, Wang X, Yang X, et al. Increased risk of gestational diabetes mellitus in women with higher prepregnancy ambient PM(2.5) exposure. Sci Total Environ 2020;730:138982. [Crossref] [PubMed]
- Yu G, Ao J, Cai J, et al. Fine particular matter and its constituents in air pollution and gestational diabetes mellitus. Environ Int 2020;142:105880. [Crossref] [PubMed]
- Shen HN, Hua SY, Chiu CT, et al. Maternal Exposure to Air Pollutants and Risk of Gestational Diabetes Mellitus in Taiwan. Int J Environ Res Public Health 2017;14:1604. [Crossref] [PubMed]
- Choe SA, Eliot MN, Savitz DA, et al. Ambient air pollution during pregnancy and risk of gestational diabetes in New York City. Environ Res 2019;175:414-20. [Crossref] [PubMed]
- Choe SA, Kauderer S, Eliot MN, et al. Air pollution, land use, and complications of pregnancy. Sci Total Environ 2018;645:1057-64. [Crossref] [PubMed]
- Fleisch AF, Gold DR, Rifas-Shiman SL, et al. Air pollution exposure and abnormal glucose tolerance during pregnancy: the project Viva cohort. Environ Health Perspect 2014;122:378-83. [Crossref] [PubMed]
- Fleisch AF, Kloog I, Luttmann-Gibson H, et al. Air pollution exposure and gestational diabetes mellitus among pregnant women in Massachusetts: a cohort study. Environ Health 2016;15:40. [Crossref] [PubMed]
- Jo H, Eckel SP, Chen JC, et al. Associations of gestational diabetes mellitus with residential air pollution exposure in a large Southern California pregnancy cohort. Environ Int 2019;130:104933. [Crossref] [PubMed]
- Padula AM, Yang W, Lurmann FW, et al. Prenatal exposure to air pollution, maternal diabetes and preterm birth. Environ Res 2019;170:160-7. [Crossref] [PubMed]
- Papatheodorou S, Gold DR, Blomberg AJ, et al. Ambient particle radioactivity and gestational diabetes: A cohort study of more than 1 million pregnant women in Massachusetts, USA. Sci Total Environ 2020;733:139340. [Crossref] [PubMed]
- Rammah A, Whitworth KW, Amos CI, et al. Air Pollution, Residential Greenness and Metabolic Dysfunction during Early Pregnancy in the INfancia y Medio Ambiente (INMA) Cohort. Int J Environ Res Public Health 2021;18:9354. [Crossref] [PubMed]
- Robledo CA, Mendola P, Yeung E, et al. Preconception and early pregnancy air pollution exposures and risk of gestational diabetes mellitus. Environ Res 2015;137:316-22. [Crossref] [PubMed]
- Sun Y, Li X, Benmarhnia T, et al. Exposure to air pollutant mixture and gestational diabetes mellitus in Southern California: Results from electronic health record data of a large pregnancy cohort. Environ Int 2022;158:106888. [Crossref] [PubMed]
- Zheng Y, Wen X, Bian J, et al. Associations between the chemical composition of PM(2.5) and gestational diabetes mellitus. Environ Res 2021;198:110470. [Crossref] [PubMed]
- Kim JH, Choi YY, Yoo SI, et al. Association between ambient air pollution and high-risk pregnancy: A 2015-2018 national population-based cohort study in Korea. Environ Res 2021;197:110965. [Crossref] [PubMed]
- Malmqvist E, Jakobsson K, Tinnerberg H, et al. Gestational diabetes and preeclampsia in association with air pollution at levels below current air quality guidelines. Environ Health Perspect 2013;121:488-93. [Crossref] [PubMed]
- Melody SM, Ford JB, Wills K, et al. Maternal exposure to fine particulate matter from a large coal mine fire is associated with gestational diabetes mellitus: A prospective cohort study. Environ Res 2020;183:108956. [Crossref] [PubMed]
- Melody SM, Wills K, Knibbs LD, et al. Maternal Exposure to Ambient Air Pollution and Pregnancy Complications in Victoria, Australia. Int J Environ Res Public Health 2020;17:2572. [Crossref] [PubMed]
- Hu H, Ha S, Henderson BH, et al. Association of Atmospheric Particulate Matter and Ozone with Gestational Diabetes Mellitus. Environ Health Perspect 2015;123:853-9. [Crossref] [PubMed]
- American Diabetes Association. In: Standards of medical care in diabetes—2008. Diabetes Care 2008;31:S12-S54. [Crossref] [PubMed]
- Bowe B, Xie Y, Li T, et al. The 2016 global and national burden of diabetes mellitus attributable to PM(2·5) air pollution. Lancet Planet Health 2018;2:e301-12. [Crossref] [PubMed]
- Johns EC, Denison FC, Norman JE, et al. Gestational Diabetes Mellitus: Mechanisms, Treatment, and Complications. Trends Endocrinol Metab 2018;29:743-54. [Crossref] [PubMed]
- Pathirana MM, Lassi Z, Ali A, et al. Cardiovascular risk factors in women with previous gestational diabetes mellitus: A systematic review and meta-analysis. Rev Endocr Metab Disord 2021;22:729-61. [Crossref] [PubMed]
- Li D, Wang JB, Yu ZB, et al. Air pollutants concentration and variation of blood glucose level among pregnant women in China: A cross-sectional study. Atmos Environ 2020;223:117191. [Crossref]
- Najafi ML, Zarei M, Gohari A, et al. Preconception air pollution exposure and glucose tolerance in healthy pregnant women in a middle-income country. Environ Health 2020;19:131. [Crossref] [PubMed]
- Amegah AK, Sewor C, Obeng AA, et al. Vitamin D intake modifies the association of household air pollution exposure with maternal disorders of pregnancy. Indoor Air 2022;32:e12963. [Crossref] [PubMed]
(English Language Editor: J. Jones)