Overview of triple negative breast cancer prognostic signatures in the context of data science-driven clinico-genomics research
Background
Triple-negative breast cancer (TNBC) is a highly aggressive tumor with inherent heterogeneity leading to a variety of outcomes, including early relapse, metastatic spread, and poor survival. Chen et al.’s (1) proposed a prognostic signature to improve risk stratification in TNBC patients obtained by combining expressions from The Cancer Genome Atlas (TCGA), with 1,090 primary tumors and 113 normal, and RNA-seq data FUSCCTNBC (Sequence Read Archive, SRP15). As the samples were classified according to subtypes (HR+/HER2-, HR+/HER2+, HR-/HER2+, TNBC, and normal samples with status of ER, PR, and HER2), the differentially expressed gene (DEG) profiles were computed from pairwise comparisons between TNBC and the other subtypes.
In general, signatures may inherently determine stratifications based on groups of patients sharing similar tumor characteristics and biological properties. Ideally, a signature should be linked to some novel mechanism, a pathway or a biological process or a drug mechanism. Algorithmic or semi-algorithmic identifications of (non-redundant) groups call for validation at both biological and clinical levels. For instance, prognostic validity may be established because of its association with overall survival. One usually relies on the ‘big data evidence’ that omics are expected to support. However, the reliability assigned to these and other types of signatures is a controversial matter. First, many cancer signatures are not fully justified. Signatures may fail to show biological value with reference to the specific cancer they address. This is typically proven algorithmically, by enabling gene signature replacement and showing that surrogate genes have measurable prognostic prediction power not affecting the overall signature, but with opposite biological interpretation compared to the replaced genes with respect to mechanisms. Therefore, the problem becomes lack of provable causality regarding outcome prediction, depending on the usable surrogate gene space.
Second, reproducibility implies the ability to assess the robustness of a signature relatively to specific methods or platforms. A demonstration for association with outcomes should be generally valid across independent groups and replicate experiments, and with no role played by factors excluded from the model generating the signature, including noise. Gene expression signatures (GES) connecting phenotypes to mRNA suffer from quite limited reproducibility. Therefore, one possible strategy is to combine significantly enhanced GES across datasets and experiments covering various phenotypes. Another strategy is to correlate gene expressions with other measurements to increase the signature’s prediction power, for instance through co-expression networks.
The strategy followed by Chen et al. [2022] to obtain the prognostic GES involves multiple steps (Figure 1A). Chen et al.’s results are now contextualized (Figures 1B,2) with reference to a selected non-comprehensive list of recent signature results retrieved from PubMed (query: “TNBC prognostic signatures”, 164 results).
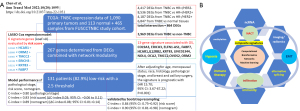
Contextualization
NACT and EMT
TNBC patients respond better to neoadjuvant therapy compared to other BC subtypes, though some patients still have a poor prognosis (2). A study represented the transcriptional portrait associated with neoadjuvant chemotherapy (NACT) resistance and found candidate genes as prognostic markers (3). In Blaye et al.’s study (4), an immunologic transcriptomic signature was found using 115 post-NACT samples to predict relapse in TNBC patients by profiling 770 TME-related genes using the NanoString PanCancer IO360 panel. The 8 genes linked to immunity (BLK, GZMM, CXCR6, LILRA1, SPIB, CCL4, CXCR4, SLAMF7) were predictive of intra-cohort relapse (validated externally in KMplot and METABRIC), indicating that lack of immune activation after NACT is associated with a high risk of distant relapse. Another study (5) revealed a 6-epithelial-mesenchymal transition (EMT) gene signature (LUM, SFRP4, COL6A3, MMP2, CXCL12, and HTRA1) at diagnosis potentially predictive for metastasis in TNCB patients who did not achieve pathological complete response to NACT and in patients treated with surgery in combination with adjuvant therapy. The EMT pathway was significantly enriched in pre- and post-NACT patients with metastasis after NACT. The 6-EMT gene signature was validated in a GEO dataset (HR =0.36, P=0.0008, 95% CI: 0.200–0.658) and in another GEO dataset of TNBC patients who received surgery followed by adjuvant chemotherapy (HR =0.46, 95% CI: 0.225–0.937). Other results (6) informing on cell plasticity relatively to EMT were centered on a signature of circulating tumor cells (CTCs) from 32 patients aimed to identify markers associated with TNBC outcome and aggressiveness, especially tissue inhibitor of metalloproteinases 1 (TIMP1) and androgen receptor (AR), whose blockade was further analyzed in vitro and in vivo in relation to proliferation and dissemination.
Immunotherapy & hypoxia-related
Immunotherapy (ImT) research and TNBC prognosis present signature gaps. A hypoxia-immune-based cross-cohort classifier predictive of prognosis was developed and validated in (7) to potentially guide TNBC ImT. In study of Yang et al. (8), six gene cross-cohort prognostic hypoxia-immune related signatures were identified to stratify patients into high-/low-risk groups, with the former group showing poorer prognosis, and correlation with hypoxia status in tumor microenvironment (TME). Interestingly, there were relatively less activated immune cells and lower expression levels in immune checkpoint inhibitors (ICI). In study of Sun et al. (9), a robust predictive 3-gene signature (PEKL, ALDOA, PGK1) associated with the glycolytic process was identified based on 48 hypoxia-related prognostic genes and a risk score model was set as a prognostic biomarker for TNBC. Future ImT developments will involve ICI toward TNBC treatment, as in Islam et al.’s study (10) (PI3K, MEK, PARP, EGFR, VEGF, and AR). Immune prognostic signatures were investigated (11) based on 66 prognostic genes, showing predictive power of the response to ICI (PD-1, PD-L1, PD-L2 and CTLA4 gene expressions higher in the low-risk group). ICI expression (and clinical) profiles of TNBC samples from TCGA and METABRIC were used to build 1-, 2-, 3-year OS-related GES (PDCD1, PDCD1LG2 and KIR3DL2, with receiver operating characteristic (ROC) curves showing AUC values of 0.925, 0.822 and 0.835, respectively) from potentially favorable OS predictors (IDO1, CD274, PDCD1LG2, PDCD1, CTLA4, ICOS, KIR3DL2, HLA-B, HLAF, LGA3) (12).
Studies of underlying mechanisms will include the TME and the immune infiltration (ImI). In study of Qin et al. (13), the TNBC TME landscape was elucidated (GEO, N=107, training: METABRIC, N=299, validation cohorts). Two distinct TME clusters were identified, one characterized by low ImI with poor prognosis, the other with high ImI and better survival probability. These clusters showed differential expression (37 upregulated and 778 downregulated genes) delivering then a final 8-gene TME signature (PPFIA4, TNFRSF1B, ARHGAP9, ZNF831, CTLA4, BLK, ANKRD22 and CLEC4E) in which TNFRSF1B and BLK were risk factors for poor prognosis in TNBC patients. In (14), from the study of 1,145 patients a tumor immune risk score (TIRS) was obtained with 8 biomarkers stratifying patients in high- and low-risk groups. Significant survival and ImI pattern differences were found at both transcriptome and protein levels indicating 4 different tumor immune microenvironment types (TIMT): one associated with the best prognosis and immune status, another with the opposite effect, the other two associated with highly unstable genomes and stem-cell-related characteristics along with high stromal scores.
Bioinformatics composite signatures
TNBC prognostic signatures may involve hub genes and pathways (15), without establishing genes as drivers of network causality and directionality (16). For example, in (17) a 5-gene prognostic signature (CD79A, CXCL13, IGLL5, LHFPL2, and PLEKHF1) was found based on co-expressed hub genes associated with macrophages and related to gemcitabine resistance in TNBC patients. Then, a survival-related 5-gene hub signature (TOP2A, CCNA2, PCNA, MSH2, CDK6) was combined with ImI analysis to show relevance for immune monitoring across GEO cancer data. Tumor-infiltrating lymphocytes (TILs) are a robust prognostic factor for improved patient survival, with T cells being predominant, but without resolving the uncertainty about the relationship with patient prognosis (18).
The real predictive power of cancer-related immune prognostic markers was investigated in (19) through a bioinformatic approach identifying a 6-gene signature (IL18R1, CD53, TRIM, JAW1, LTB, and PTPRCAP) positively correlated with disease-free survival (DFS) in TNBC patients and showing significant immune expression profiles in cancer cells and in intra- and peri-TILs. In Kim et al.’s study (20), it was identified the associations between gene expression and distant recurrence-free survival (DRFS) from a 13-gene expression profile (CD1B, CD53, CT45A1, GTF3C1, IL11RA, IL1RN, LRRN3, MAPK1, NEFL, PRKCE, PTPRC, SPACA3 and TNFSF11) associated with patient prognosis (P<0.05) (AUC =0.923). Combined with stage, it was predictive of distant recurrence of early TNBC, with 3 genetic risk groups classifying DRFS rate significantly even intra-stage (P<0.001) and informing on treatment with new possible ImT targets. Finally, cell differentiation together with grade- and tumor mutational burden-related DEGs were identified using integrated single-cell and bulk RNA-seq data analysis, delivering a composite 10-gene prognostic signature (RMND5A, ZNF829, KDM5B, NCBP2, GPI, BGN, CCND2, PLBD1, ZYG11A, IL17RD), closely related to tumor ImI (21).
Epigenetics
Despite its relevance, aberrant DNA methylation is still underutilized in prognostic models for TNBC. For instance, the N6-methyladenosine (m6A) modification is relevant in cancer development, but not enough evidenced in TNBC. Based on the TCGA transcriptome profiles of the 13 m6A methylation regulators measured from 98 TNBC tumor and normal tissue samples, two expression levels were emphasized, specifically ALKBH5 (unfavorable prognostic factor, HR =3.327, P=0.006) and METTL14 (favorable, HR =0.425, P=0.009), both contributing to improve the accuracy of a prognostic model of risk prediction when combined with TNM stage (AUC =0.791) (22). Aberrant DNA methylation was also investigated in study of Zhang et al. (23) from TCGA paracancer samples (PCS). Differential methylation-DEG correlation revealed 1,525 DEGs and 150 differentially methylated genes between TNBC and PCS, with a signature including prognostic markers such as ABCC9 (cg06951626), NKAPL (cg18675097, cg01031101, and cg17384889), and TMEM132C (cg03530754).
In Gao et al.’s study (24), methylation and expression data from TCGA were combined, and 743 differentially methylated sites (DMS) were identified corresponding to 332 genes, with 357 hypermethylated sites and 386 hypomethylated sites, of which 103 were prognosis related. A LASSO-driven 5-DMSs prognostic signature was found to classify TNBC patients with significant survival difference (log-rank P=4.97E-03), then validated in GSE78754 (HR =2.42, 95% CI: 1.27–4.59, log-rank P=0.0055) and verified for DFS in GSE141441 (HR =2.09, 95% CI: 1.28–3.44, log-rank P=0.0027). Two DMS were related to high risk (cg21234506 and cg21580376; HR >1), and three DMS were protective (cg15724876, cg17887364, and cg19419246; HR <1). Interestingly, a recent study (25) on TME of TNBC associated m6A modification and hypoxia status, identifying 26 genes related to both regulation types and characterizing two clusters, one being with significantly worse prognosis. A 6-gene prognostic signature was identified (PIM2, PET117, SMARCA5, TAF9, ABCB10, MKP1) among the m6A modification-hypoxia genes to evaluate risk and predict ImT response of patients.
Network- and machine learning (ML)-driven signatures
Key DEGs (CENPW, HORMAD1, APOD, PIP, and ZNF703) were found as candidate prognostic markers associated with poor OS through integrated bioinformatics performed on GEO microarray TNBC data (26). Network analysis focused on 147 co-DEGs in TNBC vs non-cancerous tissue samples, identifying a 15-gene signature inclusive of BUB1 and CENPF significantly correlated with OS, while BUB1, CCNA2, and PACC1 showed significant poor DFS (27). In study of Liu et al. (28), 105 heterogeneous DEGs were identified between TNBC and other subtypes. Two prognostic signatures were significant: a 4-gene (FAM83B, KITLG, CFD and RBM24) in disease-free interval, and a 5-gene (FAM83B, EXO1, S100B, TYMS and CFD) in progression-free interval. The multivariate Cox regression models showed predictive performance by time-dependent ROC analysis and survival analysis of TNBC subtypes (mesenchymal stem-like and mesenchymal) emphasized the FAM83B prognostic role.
In study of Liu et al. (29), bioinformatics and network analyses explored the role of alternative splicing events (ASE) events and their correlation with TNBC prognostic DEGs, delivering ASE profiles, prognostic interaction networks, and splice factor-AS interaction networks. Finally, the use of ML was central to (30) where first a Recursive Feature Elimination (RFE) algorithm identified signatures (20, 25, 30, 35, 40, 45, and 50 genes) differentiating TNBC from other subtypes, then XGBoost was found the best performer for 45 genes (mixed signatures), of which 34 genes differentially regulated, 4 specifically relevant for distant metastasis free survival, and 2 potentially prognostic (POU2AF1 and S100B) associated with MAPK, PI3-AkT, Wnt, TGF-β, and other signal transduction pathways involved in metastasis. In study of Gong et al. (31), 151 TNBC patients obtained from the TCGA SpliceSeq database showed relevance for the Exon Skip (ES) type of AS events, more robust in predicting performance in TNBC prognosis. The ES AS signature confers a strong oncogenic phenotypic enrichment in a high-risk group (cell cycle and SUMOylating pathways of tumorigenesis), and a low-risk group (programmed cell death and metabolism pathways).
Non-coding RNAs
Many ncRNAs are likely dysregulated in TNBC, some being promising biomarkers and prognostic too. For instance, an 8-miRNAs signature (miR-139-5p, miR-10b-5p, miR-486-5p, miR-455-3p, miR-107, miR-146b-5p, miR-324-5p and miR-20a-5p) supported an accurate predictive model of relapse in TNBC patients highly correlated with prognosis (AUC of 0.80), independently validated twice (AUCs of 0.89 and 0.90) (32). In study of Zaka et al. (33), Cox analysis was employed to identify 25 miRNAs associated with prognosis with both risky and protective outcomes. Some miRNAs were associated with OS (hsa-miR-342-3p, hsa-miR-195, hsa-miR-155, and hsa-miR-497) and others with distant metastases-free survival (hsa-miR-29c, hsa-miR-342-3p, hsa-let-7c, hsa-let-7b, and hsa-miR-497). Based on both TCGA and GEO, and supported by ImmPort relatively to immune-related mRNAs, of 62 identified immune-related lncRNAs a signature of 4 (RP11-890B15.3, RP11-1024P17.1, MFI2-AS1 and RP11-180N14.1) showed regulation power mediated by miRNAs and prognostic value stratifying TNBC patients into high- and low-risk groups. The high-risk group had unfavorable outcomes together with significant immune response to tumor cell and high infiltrating abundance of regulatory T cell (34). In study of Wu et al. (35), a 10-m6A-related lncRNA signature predictive of the prognostic risk in TNBC (SAMD12-AS1, BVES-AS1, LINC00593, MIR205HG, LINC00571, ANKRD10-IT1, CIRBP-AS1, SUCLG2-AS1, BLACAT1, and HOXB-AS1) was found, establishing a prognostic score risk model (AUC of 0.997 and 0.864 in TCGA and GSE76250 datasets, respectively) and showing patients with lower score associated to better OS.
Radiomics & integrated signatures
A TNBC radiogenomic study (N=202) generated quantitative radiomic features (n=860) from contrast-enhanced magnetic resonance images (CE-MRI). Notably, a radiomic feature capturing peritumoral heterogeneity (PH) was an established prognostic factor for RFS (P=0.01) and OS (P=0.004). Combined with matching transcriptomic and metabolomic data, the PH was associated with immune suppression and upregulated fatty acid synthesis (36). A role for radiomics with reference images to achieve consistent assessments is linked to the assessment of stromal TILs to be incorporated into clinical practice scores based on the reproducibility of multiple factors central to the heterogeneity in lymphocyte distribution (slide-related issues; outside tumor boundary; minimal assessable stroma; inflammatory cells etc.) (37). In study of Wu et al. (38), 17 dynamic CE-MRI features and TILs were combined to characterize tumor and parenchyma in a TGCA cohort (n=126). A LASSO imaging signature for TILs was found and a prediction model built with imaging signature and molecular features, resulting in 4 imaging features significantly associated with TILs: tumor volume, cluster shade of signal enhancement ratio (SER), mean SER of tumor-surrounding background parenchymal enhancement (BPE), and proportion of BPE. The imaging signature during validation allowed the predicted TILs to separate TNBC patients into two groups with distinct RFS (log-rank P=0.042), with TNBC showing no/minimal TILs associated with a worse prognosis.
To conclude, TNBC research offers room for further improvement. Especially longitudinal studies based on patients with long or more prolonged survival life periods (5–10 years) and involving a multitude of data/marker types including imaging, will be valuable, bringing future challenges for integrative data science approaches.
Acknowledgments
Funding: The author acknowledges support from JAX Computational Sciences, JAX Cancer Center (JAXCC) and NCI CCSG (P30CA034196) and support from grant NSF 19-500, DMS 1918925/1922843.
Footnote
Provenance and Peer Review: This article was commissioned by the editorial office, Annals of Translational Medicine. The article did not undergo external peer review.
Conflicts of Interest: The author has completed the ICMJE uniform disclosure form (available at https://atm.amegroups.com/article/view/10.21037/atm-22-5477/coif). The author has no conflicts of interest to declare.
Ethical Statement: The author is accountable for all aspects of the work in ensuring that questions related to the accuracy or integrity of any part of the work are appropriately investigated and resolved.
Open Access Statement: This is an Open Access article distributed in accordance with the Creative Commons Attribution-NonCommercial-NoDerivs 4.0 International License (CC BY-NC-ND 4.0), which permits the non-commercial replication and distribution of the article with the strict proviso that no changes or edits are made and the original work is properly cited (including links to both the formal publication through the relevant DOI and the license). See: https://creativecommons.org/licenses/by-nc-nd/4.0/.
References
- Chen C, Lin CJ, Li SY, et al. Identification of a novel signature with prognostic value in triple-negative breast cancer through clinico-transcriptomic analysis. Ann Transl Med 2022;10:1095. [Crossref] [PubMed]
- Sporikova Z, Koudelakova V, Trojanec R, et al. Genetic Markers in Triple-Negative Breast Cancer. Clin Breast Cancer 2018;18:e841-50. [Crossref] [PubMed]
- Vishnubalaji R, Alajez NM. Transcriptional landscape associated with TNBC resistance to neoadjuvant chemotherapy revealed by single-cell RNA-seq. Mol Ther Oncolytics 2021;23:151-62. [Crossref] [PubMed]
- Blaye C, Darbo É, Debled M, et al. An immunological signature to predict outcome in patients with triple-negative breast cancer with residual disease after neoadjuvant chemotherapy. ESMO Open 2022;7:100502. [Crossref] [PubMed]
- Wei LY, Zhang XJ, Wang L, et al. A Six-Epithelial-Mesenchymal Transition Gene Signature May Predict Metastasis of Triple-Negative Breast Cancer. Onco Targets Ther 2020;13:6497-509. [Crossref] [PubMed]
- Abreu M, Cabezas-Sainz P, Pereira-Veiga T, et al. Looking for a Better Characterization of Triple-Negative Breast Cancer by Means of Circulating Tumor Cells. J Clin Med 2020;9:353. [Crossref] [PubMed]
- Zheng S, Zou Y, Liang JY, et al. Identification and validation of a combined hypoxia and immune index for triple-negative breast cancer. Mol Oncol 2020;14:2814-33. [Crossref] [PubMed]
- Yang X, Weng X, Yang Y, et al. A combined hypoxia and immune gene signature for predicting survival and risk stratification in triple-negative breast cancer. Aging (Albany NY) 2021;13:19486-509. [Crossref] [PubMed]
- Sun X, Luo H, Han C, et al. Identification of a Hypoxia-Related Molecular Classification and Hypoxic Tumor Microenvironment Signature for Predicting the Prognosis of Patients with Triple-Negative Breast Cancer. Front Oncol 2021;11:700062. [Crossref] [PubMed]
- Islam R, Lam KW. Recent progress in small molecule agents for the targeted therapy of 454 triple-negative breast cancer. Eur J Med Chem 2020;207:112812. [Crossref] [PubMed]
- Wang C, Feng G, Zhu J, et al. Developing an immune signature for triple-negative breast cancer to predict prognosis and immune checkpoint inhibitor response. Future Oncol 2022;18:1055-66. [Crossref] [PubMed]
- Liu J, Ling Y, Su N, et al. A novel immune checkpoint-related gene signature for predicting overall survival and immune status in triple-negative breast cancer. Transl Cancer Res 2022;11:181-92. [Crossref] [PubMed]
- Qin Y, Deng J, Zhang L, et al. Tumor microenvironment characterization in triple-negative breast cancer identifies prognostic gene signature. Aging (Albany NY) 2021;13:5485-505. [Crossref] [PubMed]
- Liu C, Li Y, Xing X, et al. Immunogenomic landscape analyses of immune molecule signature-based risk panel for patients with triple-negative breast cancer. Mol Ther Nucleic Acids 2022;28:670-84. [Crossref] [PubMed]
- Ma J, Chen C, Liu S, et al. Identification of a five genes prognosis signature for triple-negative breast cancer using multi-omics methods and bioinformatics analysis. Cancer Gene Ther 2022;29:1578-89. [Crossref] [PubMed]
- Dill CD, Dammer EB, Griffen TL, et al. A network approach reveals driver genes associated with survival of patients with triple-negative breast cancer. iScience 2021;24:102451. [Crossref] [PubMed]
- Su P, Peng Z, Xu B, et al. Establishment and validation of an individualized macrophage-related gene signature to predict overall survival in patients with triple negative breast cancer. PeerJ 2021;9:e12383. [Crossref] [PubMed]
- Savas P, Virassamy B, Ye C, et al. Single-cell profiling of breast cancer T cells reveals a tissue-resident memory subset associated with improved prognosis. Nat Med 2018;24:986-93. [Crossref] [PubMed]
- Marchetti P, Antonov A, Anemona L, et al. New immunological potential markers for triple negative breast cancer: IL18R1, CD53, TRIM, Jaw1, LTB, PTPRCAP. Discov Oncol 2021;12:6. [Crossref] [PubMed]
- Kim JY, Jung HH, Sohn I, et al. Prognostication of a 13-immune-related-gene signature in patients with early triple-negative breast cancer. Breast Cancer Res Treat 2020;184:325-34. [Crossref] [PubMed]
- Wang X, Chen H. Prognosis Prediction Through an Integrated Analysis of Single-Cell and Bulk RNA-Sequencing Data in Triple-Negative Breast Cancer. Front Genet 2022;13:928175. [Crossref] [PubMed]
- Wang S, Zou X, Chen Y, et al. Effect of N6-Methyladenosine Regulators on Progression and Prognosis of Triple-Negative Breast Cancer. Front Genet 2020;11:580036. [Crossref] [PubMed]
- Zhang X, Kang X, Jin L, et al. ABCC9, NKAPL, and TMEM132C are potential diagnostic and prognostic markers in triple-negative breast cancer. Cell Biol Int 2020;44:2002-10. [Crossref] [PubMed]
- Gao Y, Wang X, Li S, et al. Identification of a DNA Methylation-Based Prognostic Signature for Patients with Triple-Negative Breast Cancer. Med Sci Monit 2021;27:e930025. [Crossref] [PubMed]
- Shen X, Zhong J, He J, et al. Identification of m6A modification patterns and development of m6A-hypoxia prognostic signature to characterize tumor microenvironment in triple-negative breast cancer. Front Immunol 2022;13:978092. [Crossref] [PubMed]
- Bissanum R, Kamolphiwong R, Navakanitworakul R, et al. Integrated bioinformatic analysis of potential biomarkers of poor prognosis in triple-negative breast cancer. Transl Cancer Res 2022;11:3039-49. [Crossref] [PubMed]
- Chen DL, Cai JH, Wang CCN. Identification of Key Prognostic Genes of Triple Negative Breast Cancer by LASSO-Based Machine Learning and Bioinformatics Analysis. Genes (Basel) 2022;13:902. [Crossref] [PubMed]
- Liu Y, Teng L, Fu S, et al. Highly heterogeneous-related genes of triple-negative breast cancer: potential diagnostic and prognostic biomarkers. BMC Cancer 2021;21:644. [Crossref] [PubMed]
- Liu Q, Wang X, Kong X, et al. Prognostic Alternative mRNA Splicing Signature and a Novel Biomarker in Triple-Negative Breast Cancer. DNA Cell Biol 2020;39:1051-63. [Crossref] [PubMed]
- Thalor A, Kumar Joon H, Singh G, et al. Machine learning assisted analysis of breast cancer gene expression profiles reveals novel potential prognostic biomarkers for triple-negative breast cancer. Comput Struct Biotechnol J 2022;20:1618-31. [Crossref] [PubMed]
- Gong S, Song Z, Spezia-Lindner D, et al. Novel Insights Into Triple-Negative Breast Cancer Prognosis by Comprehensive Characterization of Aberrant Alternative Splicing. Front Genet 2020;11:534. [Crossref] [PubMed]
- Hong HC, Chuang CH, Huang WC, et al. A panel of eight microRNAs is a good predictive parameter for triple-negative breast cancer relapse. Theranostics 2020;10:8771-89. [Crossref] [PubMed]
- Zaka M, Sutton CW, Peng Y, et al. Model-Based Integration Analysis Revealed Presence of Novel Prognostic miRNA Targets and Important Cancer Driver Genes in Triple-Negative Breast Cancers. Cancers (Basel) 2020;12:632. [Crossref] [PubMed]
- Li YX, Wang SM, Li CQ. Four-lncRNA immune prognostic signature for triple-negative breast cancer Running title: Immune lncRNAs predict prognosis of TNBC. Math Biosci Eng 2021;18:3939-56. [Crossref] [PubMed]
- Wu J, Cai Y, Zhao G, et al. A ten N6-methyladenosine-related long non-coding RNAs signature predicts prognosis of triple-negative breast cancer. J Clin Lab Anal 2021;35:e23779. [Crossref] [PubMed]
- Jiang L, You C, Xiao Y, et al. Radiogenomic analysis reveals tumor heterogeneity of triple-negative breast cancer. Cell Rep Med 2022;3:100694. [Crossref] [PubMed]
- Kos Z, Roblin E, Kim RS, et al. Pitfalls in assessing stromal tumor infiltrating lymphocytes (sTILs) in breast cancer. NPJ Breast Cancer 2020;6:17. [Crossref] [PubMed]
- Wu J, Li X, Teng X, et al. Magnetic resonance imaging and molecular features associated with tumor-infiltrating lymphocytes in breast cancer. Breast Cancer Res 2018;20:101. [Crossref] [PubMed]