Adding heart rate n-variability (HRnV) to clinical assessment potentially improves prediction of serious bacterial infections in young febrile infants at the emergency department: a prospective observational study
Introduction
Serious bacterial infections (SBIs) [including meningitis, bloodstream infections and urinary tract infections (UTIs)] in young febrile infants (<90 days old) pose a diagnostic dilemma to emergency department (ED) physicians and paediatricians (1-3). Misdiagnosis and delayed time-to-antibiotics may result in death and long-term disability (4-6). Physicians therefore subject many of these young febrile infants to invasive investigations [blood, urine and cerebrospinal fluid (CSF)] and often, broad-spectrum empirical antibiotics (7,8).
Over-investigation and liberal use of antibiotics are costly. In the United States of America (USA), the cost of hospitalisation and discharge after no more than 3 days of antibiotics for these febrile infants added to more than USD 76 million dollars over seven years (9). These invasive procedures are also painful for the infants and cause caregivers additional anxiety (10). Recognising the need to reduce unnecessary testing, researchers have derived algorithms to define a low-risk population that might benefit from a less aggressive approach (11,12). Generalisability of these algorithms has been limited by variable performance among different patient populations (13). Moreover, among infants at non-low risk of SBIs, these algorithms do not provide guidance on which infants should receive priority for time-critical interventions. This lack of prioritisation causes delays in time-to-antibiotics for febrile infants who are at high risk for SBIs (14). Researchers continue to pursue predictive models for SBIs using clinical and biochemical predictors (15-17).
We previously demonstrated that heart rate variability (HRV) adds to triage performance in predicting for SBIs among febrile infants (18). HRV analyses beat-to-beat (R-R) oscillation and is a measure of autonomic nervous system (ANS) regulation (19). ANS dysfunction is a maladaptive response in injury and critical illness, including sepsis states (20-22). In the adult ED sepsis population, HRV parameters have been demonstrated to correlate well with disease severity and impending shock (23-25). Heart rate n-variability (HRnV), constructs new signals based on the R-to-R peak intervals (RRI) used in the conventional HRV analysis. It was more recently invented as a novel tool to augment the number of calculated parameters from the same segment of signals, with the potential to enhance the prognostic information provided by traditional HRV parameters (26,27).
We therefore sought to explore the potential of HRV and HRnV measures to predict for SBIs in young febrile infants. We hypothesized that the addition of HRV and HRnV measures to existing triage tools will enhance the discriminative ability of models to identify SBIs at the time of ED triage. We present the following article in accordance with the TRIPOD reporting checklist (28) (available at https://atm.amegroups.com/article/view/10.21037/atm-22-3303/rc).
Methods
Study design, population and recruitment
We performed a prospective observational study of febrile infants (<90 days old) presenting to the ED of KK Women’s and Children’s Hospital in Singapore, between December 2017 and November 2021. As the larger of 2 paediatric hospitals in the country, we serve children <18 years old with an annual ED attendance of about 150,000 children. In this study, we recruited a convenience sample of infants <90 days old who had an axillary or rectal temperature of 38 ℃ and above. For infants who were over-wrapped, ED triage nurses were trained to unwrap these infants, keeping on a single layer of clothing, and recheck their temperatures. Infants who still had an axillary temperature of 38 ℃ and above were eligible for recruitment.
Recruitment took place during office hours with the support of dedicated research personnel. We excluded infants with a history of non-sinus rhythm due to potential confounding on the HRV analysis, and preterm infants <35 weeks’ gestation who would innately constitute a higher risk profile who should receive more urgent investigations. Recruitment was stopped in the ED during the COVID-19 pandemic (February 7th 2020–February 17th 2021, May 6th–July 12th 2021) due to hospital infection control policies.
In our hospital, all febrile infants <90 days are hospitalised for further investigations and monitoring. Neonates (infants <28 days old) receive a comprehensive workup, including blood, urine and CSF analysis, and routinely receive broad-spectrum antibiotics. Infants between 28–90 days old receive blood and urine analysis, with the medical team’s discretion on whether or not to obtain CSF for analysis. The majority of these receive antibiotics until culture results are known (8).
The study was conducted in accordance with the Declaration of Helsinki (as revised in 2013). The study was approved by the SingHealth Centralised Institutional Review Board in Singapore (No. CIRB 2017/2680) and informed consent was taken from all individual participants. We registered this study (Clinical Trial registration No. NCT04103151).
Data variables and data collection
At triage, we collected data on patient demographics and vital signs as follows: heart rate (HR) and oxygen saturation were measured using pulse oximetry, respiratory rate (RR) was obtained via manual counting by the triage nurse, and blood pressure (BP) was measured by the non-invasive Dinamap ProCare 300 (GE Medical Systems, Milwaukee, WI, USA) (8). Our hospital uses the Severity Index Score (SIS) at ED triage which assesses the child’s respiratory status, activity, colour and play (29). During the consultation, we obtained information on gestation and presence of maternal group B streptococcus (GBS). Laboratory investigations such as the total white blood cell count, the absolute neutrophil count (ANC), haemoglobin, platelets, C-reactive protein (CRP) and procalcitonin were recorded. If the infants are stable, these laboratory investigations are carried out in the ward after hospitalisation. If they are considered to be high risk for SBIs (by vital signs or clinician assessment), the investigations and administration of antibiotics are administered expeditiously in the ED. The diagnostic cascade is detailed in Figure 1.
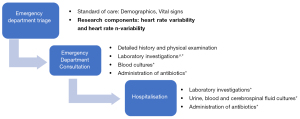
Outcome definitions
We chose the primary outcome as presence of SBIs, defined as culture-proven (I) bacterial meningitis, (II) bacteraemia and (III) UTIs (12). We chose to align our definition with the updated literature (12) and did not include pneumonia or lower respiratory tract infections stated in our original protocol (NCT04103151) because there were concerns over inconsistencies in final diagnoses when their records were reviewed. SBIs are confirmed via testing of the CSF, blood and urine, respectively. Bacterial meningitis was defined as pure growth of a single pathogen in the CSF. Bacteraemia was defined as pure growth of a single pathogen in the bloodstream. We did not include common skin contaminants like coagulase-negative staphylococcus. The definition of UTI requires both the presence of pyuria and the pure growth of a single pathogen (30). Culture positive UTI was defined as growth of a single pathogen of either >100,000 colony-forming units (CFU)/mL in a clean catch urine specimen, or >50,000 CFU/mL in a catheterized specimen, or 10,000–50,000 CFU/mL with either nitrite or leucocyte esterase positive findings in the urinalysis, in a catheterized specimen.
We also documented the clinical outcomes of mortality, admission to the paediatric intensive care unit (ICU) or high dependency (HD), and length of hospital stay. We documented the need for fluid resuscitation, mechanical ventilation and inotrope use.
Members of the study team who documented outcomes were blinded to the HRV results. The patient outcomes (SBI versus no SBI) were not known to the research personnel who performed the HRV.
HRV and HRnV analyses
We obtained HRV parameters in time-, frequency- and non-linear domains; 5-minute single lead electrocardiogram (ECG) tracings were obtained using a paediatric-friendly device, with a sampling frequency of 250 Hz. Infants were placed either supine in the cot bed or held supine in the caregivers’ arms, with our priority being the child’s comfort and to minimise movement artefacts. We enhanced the adult-derived HRV prototype and established a comprehensive PC-based software to handle the frequent motion artefacts. Our software used the Physionet HRV toolkit where R-R peaks were automatically identified (31,32). In the infant population, the QRS peaks were correctly and automatically identified in most cases. However, there were a few cases with motion artefacts and abrupt baseline drift. In these instances, our software allowed manual editing to add or delete the QRS peaks so that the HRV parameters could be derived more accurately. Also, the frequency range for frequency domain parameters was fine-tuned to accommodate the infants’ faster HR. This enabled us to perform and complete the HRV analysis on most of these young infants.
HRnV is a novel complementary method to the conventional HRV analysis (26). HRnV constructs new signals based on the RRI used in the conventional HRV analysis, called RRnIs. As illustrated in Figure S1 (27), when n≤3, we can generate 6 new RRnI signals from the original RRI by combining n consecutive RRIs, with or without overlapping signals, controlled by parameter m. Subsequently, standard HRV parameters (i.e., HRnV parameters) can be calculated using RRnIs, which share similar properties with RRIs but contain additional physiological information such as the long-term correlations within the original RRI. We obtained HRnV parameters in the same domains as the conventional HRV analysis mentioned above. We excluded from the analysis HRV and HRnV data that could not be processed due to movement artefacts.
Statistical analysis
We presented categorical variables using frequencies and percentages, and continuous variables using median and interquartile range (IQR) due to non-normality. We performed univariate Chi-squared test or Mann-Whitney U test depending on the variable type. We presented a predictive model generated by backward stepwise logistic regression. The variables in the predictive model were based on patient demographics, vital signs, HRV, HRnV parameters and laboratory investigations. We only included patients with complete data for the predictive model. We presented unadjusted and adjusted odds ratios (aOR) together with their 95% confidence intervals (CIs). Variables were entered into the multivariable regression based on their univariate P values (P<0.4) as well as clinical discretion. The variables were determined after reviewing known predictors in the literature, as well as availability of these data at ED triage and then at consultation. Collinearities (squared Pearson’s correlation coefficient bigger than 0.85) were removed before conducting multivariable regression, due to known correlations among HRV and HRnV parameters (26). We described performance of the model using area under curve (AUC) analysis, with corresponding 95% CIs. We first assessed the AUC of vital signs and clinical assessment in the prediction of SBIs, then added on HRV, HRnV and laboratory investigations, incrementally. We chose to do so because HRV and HRnV are non-invasive biomarkers and have potential at triage to provide early discrimination, before subsequent laboratory investigations (which require turnaround time) yield results.
Results
We recruited a total of 330 febrile infants, among whom 18 (5.5%) patients did not complete the study because movement artefacts rendered the HRV and HRnV parameters unsuitable for analysis (Figure 2). Among the 18 excluded infants, the median age was 11.0 days (IQR 3.0–50.5 days) and 4 (22.2%) had SBIs, all of which were UTIs. Among 312 infants analysed, the median age was 35.5 days (IQR 13.0–61.0 days) and 137 (43.9%) were females; 74/312 infants (23.7%) had SBIs with the most common being UTIs (66/72, 91.7%) and bacteraemia (6/72, 8.3%). Among the remaining 2 infants, 1 infant had meningitis and UTI, and another infant had bacteraemia and UTI.
Table 1 shows the demographics and vital signs of febrile infants, stratified by the presence of SBI. In our study population, there were significantly fewer neonates among infants with SBIs compared to those without SBIs (17/74, 23.0% vs. 103/238, 43.3%, P=0.002). Infants with SBIs were less likely to be females (14/74, 18.9% vs. 123/238, 51.7%, P<0.001), had a higher temperature (median 38.6 ℃, IQR 38.2–39.1 vs. 38.4 ℃, IQR 38.1–38.8, P=0.029) and a faster HR (median 173/min, IQR 152–188 vs. 165/min, IQR 151–178, P=0.014).
Table 1
Variables | Infants with SBIs (N=74) | Infants without SBIs (N=238) | P value |
---|---|---|---|
Age, days, median [IQR] | 49 [28–72] | 32 [10–55] | <0.001 |
Neonates <28 days, n (%) | 17 (23.0) | 103 (43.3) | 0.002 |
Female sex, n (%) | 14 (18.9) | 123 (51.7) | <0.001 |
Late pre-terms‡, n (%) | 3 (4.1) | 14 (5.9) | 0.545 |
Presence of maternal GBS, n (%) | 0.443 | ||
Yes | 16 (21.6) | 45 (18.9) | |
No | 46 (62.2) | 138 (58.0) | |
Unknown | 12 (16.2) | 55 (23.1) | |
If maternal GBS present, treated, n (%) | 14/16 (87.5) | 39/45 (86.7) | 0.932 |
Day of illness, median [IQR] | 1 [1–2] | 1 [1–1] | 0.002 |
Temperature, ℃, median [IQR] | 38.6 [38.2–39.1] | 38.4 [38.1–38.8] | 0.029 |
Heart rate, /min, median [IQR] | 173 [152–188] | 165 [151–178] | 0.014 |
Respiratory rate, /min, median [IQR] | 40 [40–48] | 40 [38–45] | 0.390 |
Pulse oximetry, %, median [IQR] | 100 [98–100] | 99 [98–100] | 0.186 |
Severity index score, median [IQR] | 9 [8–10] | 9 [8–10] | 0.785 |
Blood investigations, median [IQR] | N=74 | N=223 | |
Total white blood cell count | 14.6 [10.4–19.5] | 11.8 [8.9–14.4] | <0.001 |
Absolute neutrophil count | 7.6 [4.3–10.9] | 4.4 [2.7–6.6] | <0.001 |
Absolute lymphocyte count | 5.1 [3.7–6.6] | 4.6 [3.2–6.4] | 0.389 |
Neutrophil to lymphocyte ratio | 1.5 [0.9–2.0] | 1.0 [0.6–1.6] | <0.001 |
Absolute monocyte count | 1.5 [1.2–2.2] | 1.4 [1.0–1.8] | 0.083 |
Hemoglobin | 11.0 [10.1–12.7] | 12.2 [10.7–16.4] | 0.001 |
Platelets | 469 [400–544] | 412 [346–488] | 0.002 |
C-reactive protein, median [IQR] | N=73 | N=218 | |
28.6 [12.4–61.2] | 2.9 [0.1–9.2] | <0.001 | |
Procalcitonin, median [IQR] | N=38 | N=80 | |
0.59 [0.14–4.3] | 0.11 [0.05–0.18] | <0.001 |
‡, defined as gestation 35–36 weeks. SBIs, serious bacterial infections; IQR, interquartile range; GBS, group B streptococcus.
There were significant differences in the full blood count indices, CRP and procalcitonin (Table 1). We found time-, frequency- and non-linear HRV parameters that were significantly different between febrile infants with SBIs and those without (Table 2). A large number of HRnV parameters were potentially discriminatory between the 2 groups and are ranked by statistical significance in Table S1. Infants with SBIs had lower variability than those in the non SBI group. There were no adverse events that occurred from application of HRV and HRnV.
Table 2
Variables | SBI, median (IQR) | No SBI, median (IQR) | P value |
---|---|---|---|
LF (ms) | 160.03 (104.30, 291.21) | 248.17 (105.19, 561.19) | 0.046 |
Poincare’ SD2 (ms) | 25.33 (18.34, 42.01) | 31.46 (21.93, 49.14) | 0.046 |
TP (ms) | 778.19 (426.80, 2,223.23) | 1,252.98 (660.01, 2,975.05) | 0.049 |
SDNN (ms) | 18.58 (13.10, 30.49) | 23.21 (15.66, 36.91) | 0.062 |
VLF (ms) | 538.19 (259.64, 1,728.27) | 916.98 (413.62, 2,243.64) | 0.068 |
Kurtosis | 0.14 (0.00, 0.53) | 0.30 (0.00, 2.04) | 0.101 |
Poincare’ SD1 (ms) | 5.58 (3.81, 8.41) | 6.57 (4.02, 12.17) | 0.112 |
RMSSD (ms) | 9.29 (5.68, 17.21) | 9.29 (5.68, 17.21) | 0.112 |
Skewness | 2.00 (0.00, 5.00) | 3.00 (0.00, 17.00) | 0.125 |
SDHR (bpm) | 8.04 (6.35, 13.07) | 9.56 (6.91, 13.14) | 0.144 |
RR triangular index | 4.70 (3.70, 7.60) | 5.40 (3.60, 8.50) | 0.147 |
HF (ms) | 34.62 (16.43, 99.04) | 48.18 (18.88, 118.61) | 0.167 |
Mean NN (ms) | 370.14 (350.97, 405.55) | 370.14 (350.97, 405.55) | 0.284 |
AVHR (bpm) | 162.66 (148.03, 171.40) | 160.97 (144.04, 171.00) | 0.297 |
NN50 | 0.12 (−0.40, 0.51) | 0.24 (−0.23, 0.71) | 0.374 |
HRV parameters are listed in ascending P value, up to P<0.4. SBIs, serious bacterial infections; HRV, heart rate variability; IQR, interquartile range; LF, low frequency; TP, total power; SDNN, standard deviation of R-R intervals; VLF, very low frequency; RMSSD, square root of the mean squared differences between R-R intervals; SDHR, standard deviation of mean heart rate; HF, high frequency; mean NN, average of R-R intervals between 2 consecutive Rs; AVHR, average heart rate; NN50, the number of times that the absolute difference between 2 successive R-R intervals exceeds 50 ms.
Majority of our febrile infants were admitted to the general ward (299/312, 95.8%) with only 6/312 (1.9%) requiring higher acuity care in the ICU or HD. There were no deaths in this study population. More infants with SBIs received fluid resuscitation (12/74, 16.2%) compared to those without SBIs (20/238, 8.4%), although not statistically significant (P=0.053) (Table S2). Only 1 infant required non-invasive ventilatory support, and none required endotracheal intubation or inotropic support.
The multivariable model is presented in Table 3, derived from 290 infants with complete data. Age, sex, day of fever, and RR performed with an AUC of 0.765 (95% CI: 0.705–0.825). When adding on HRV and then HRnV parameters, the AUC improved to 0.776 (95% CI: 0.718–0.835) and 0.807 (95% CI: 0.752–0.861), respectively (Figure 3). By adding laboratory results that became available after ED consultation (absolute neutrophil count, haemoglobin, and CRP), the performance of the model improved to 0.874 (95% CI: 0.828–0.921) (Figure 3).
Table 3
Variables | Odds ratio (95% CI) | Adjusted odds ratio (95% CI) |
---|---|---|
Patient demographics | ||
Female sex | 0.192 (0.1–0.37) | 0.119 (0.049–0.289) |
Age | 1.017 (1.007–1.027) | 1.018 (1.003–1.034) |
Day of fever | 1.937 (1.235–3.039) | 1.663 (0.932–2.966) |
Vital signs | ||
Respiratory rate | 1.021 (0.98–1.063) | 1.051 (0.993–1.112) |
HRV parameters | ||
Mean NN | 0.996 (0.991–1.002) | 1.02 (1.007–1.033) |
Kurtosis | 0.925 (0.836–1.024) | 1.424 (1.086–1.869) |
NN50 | 1.023 (0.833–1.255) | 1.545 (1.09–2.19) |
Poincare’ SD1 | 0.948 (0.903–0.995) | 0.558 (0.412–0.755) |
HRnV parameters | ||
HR3V SDHR | 0.893 (0.751–1.062) | 1.439 (1.071–1.933) |
HR3V Skewness | 0.994 (0.986–1.002) | 1.024 (1.002–1.047) |
HR3V2 DFA α1 | 0.387 (0.095–1.571) | 0.002 (0.0–0.05) |
Blood investigations | ||
Absolute neutrophil count | 1.179 (1.098–1.267) | 1.108 (1.008–1.217) |
Haemoglobin | 1.002 (1.0–1.005) | 1.003 (1.0–1.006) |
C-reactive protein | 1.035 (1.023–1.047) | 1.025 (1.012–1.038) |
HRV, heart rate variability; HRnV, heart rate n-variability; CI, confidence interval; mean NN, average of R-R intervals between 2 consecutive Rs; NN50, the number of times that the absolute difference between 2 successive R-R intervals exceeds 50 ms; SDHR, standard deviation of mean heart rate; DFA α1, detrended fluctuation analysis α1.
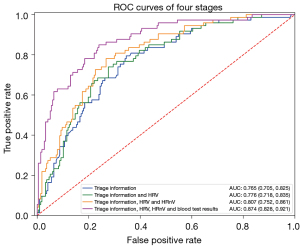
Discussion
We found discriminatory parameters using the novel HRnV method over and above conventional HRV parameters, which improved the ability to predict for SBIs when added to existing clinical parameters. When used at ED triage, HRnV and HRV in addition to demographics and vital signs improved the AUC from 0.765 (95% CI: 0.705–0.825) to 0.807 (95% CI: 0.752–0.861). Adding blood biomarkers (when the laboratory results became available) further improved the AUC to 0.874 (95% CI: 0.828–0.921).
We described a high prevalence of SBIs at 23.7%, compared to the existing literature of 10% (12). We postulate that this could be due to differences in patient population. Infants with transient fever and who were assessed to be stable at primary care centres may not have been referred to our facility. The vast majority of our SBIs were UTIs, while the prevalence of bacteraemia and meningitis (2.6%) was comparable with that of other centres (11). Unlike another study which found higher risk among neonates (11), we found a significant proportion of SBIs (77%) in the group 29–90 days old, highlighting the need for careful assessment and monitoring for all young infants <90 days old.
An earlier study among adults presenting to the ED with sepsis demonstrated that HRnV in addition to HRV parameters and vital signs, was superior in predicting for mortality when compared to traditional scores including the acute physiology and chronic health evaluation II (APACHE II) and sequential organ failure assessment (SOFA) (27). The authors propose that HRnV may contribute to rapid, objective and accurate risk stratification for sepsis severity and risk of death. We demonstrated that HRnV, in addition to HRV, adds value for risk stratification among young infants at risk of SBIs. In our study, the ROC improved from 0.776 (95% CI: 0.718–0.835) to 0.807 (95% CI: 0.752–0.861) after HRnV was added to both HRV and existing triage information.
HRnV holds promise in the young infant age group due to the non-invasive nature of this technology (18). Importantly, we demonstrated that HRnV provides additional information at triage, early in the infants’ ED journey. HRnV has the potential to reduce recognition delays and contribute to prioritisation of at-risk infants for early antibiotics (14). Future studies should focus on deriving actionable thresholds using HRV and HRnV, together with other clinical predictors, similar to an automated triage algorithm derived for chest pain in adults, providing real time risk stratification for febrile infants at risk of SBIs (26,33). Recommendations for change in clinical practice can only be made after studying the performance metrics of these thresholds. In implementing an actionable algorithm, we can then study if the time-to-antibiotics is indeed improved for infants at high risk of SBIs. An effective risk stratification approach, once validated, may address the current burdens of diagnostic inefficiencies (34,35).
There are several strengths of this study. The prospective cohort design ensured that HRV/HRnV was performed prior to knowledge of patient outcomes thereby reducing bias. In order to accommodate the infants’ physiologically faster HRs, the study team refined the prototype. These changes improved accuracy and reduced the number of infants who could not be analysed due to movement artefacts (pre-enhancement 16/168, 9.5% vs. 2/162, 1.2% post-enhancement in October 2019). By overcoming these barriers, the technology can be used for future prospective validation studies.
We recognise a number of limitations to the study. Our cohort was largely haemodynamically stable. This affects generalisability of our findings to more critically ill septic infants. Some infants did not have a complete septic workup (including a lumbar puncture) performed, therefore allowing a theoretical risk of missed SBI. However, all febrile infants are monitored in our institution until they are well and afebrile for 24 hours before discharge. We also recognise that the derivation and validation of clinical prediction models may potentially result in cases of missed SBIs, and the safety profiles of these should be evaluated carefully before recommendations for clinical implementation (36,37). We excluded 18 infants (5.5%) because their HRV could not be analysed. However, the SBI rate was comparable between the excluded infants and the analysed study population (22.2% vs. 23.7%). Although we designed the study to use a discrete 5-minute ECG recording for HRV, we recognise that continuous ECG monitoring may yield richer information for diagnostic and prognostic purposes. We did not account for possible diurnal variation in our HRV analyses. Finally, we recognise that single-centre research will need validation in other study populations.
Apart from diagnostic assessment, ANS dysregulation determined by abnormal HRV has been reported to predict for organ dysfunction and death in critically ill children (38). Children who recover from critical illness also have an improvement in time-domain HRV parameters (39). Future studies should investigate if HRnV can provide additional discrimination and stability to these prognostic models. The addition of HRnV to HRV may enrich the longitudinal physiological information made available to clinicians as they care for ill children (40).
Conclusions
We found that the addition of HRnV and HRV to demographics and vital signs improved model performance at ED triage. Further research on febrile infants in other populations is required for validation.
Acknowledgments
We would like to thank Ms Charis Lim Shu En for her assistance in the recruitment of patients in this study.
Funding: This work was funded by the National Medical Research Council (NMRC) (NMRC/MOH-CNIG18 May 0005 to SLC). NL is supported by the Duke-NUS Signature Research Programme funded by the Ministry of Health, Singapore. The funders had no role in the study design, data collection and analysis, decision to publish, or preparation of the manuscript.
Footnote
Reporting Checklist: The authors have completed the TRIPOD reporting checklist. Available at https://atm.amegroups.com/article/view/10.21037/atm-22-3303/rc
Data Sharing Statement: Available at https://atm.amegroups.com/article/view/10.21037/atm-22-3303/dss
Peer Review File: Available at https://atm.amegroups.com/article/view/10.21037/atm-22-3303/prf
Conflicts of Interest: All authors have completed the ICMJE uniform disclosure form (available at https://atm.amegroups.com/article/view/10.21037/atm-22-3303/coif). SLC reports that this work was funded by the National Medical Research Council (NMRC) (NMRC/MOH-CNIG18 May 0005 to SLC) and the funders had no role in the study design, data collection and analysis, decision to publish, or preparation of the manuscript. MEHO and NL are inventors of two patents (US10299689B2 and 10202114423W) relevant to this study. ZXK, DG, MEHO and NL own stock in TIIM Healthcare that produces devices relevant to this study. The other authors have no conflicts of interest to declare.
Ethical Statement:
Open Access Statement: This is an Open Access article distributed in accordance with the Creative Commons Attribution-NonCommercial-NoDerivs 4.0 International License (CC BY-NC-ND 4.0), which permits the non-commercial replication and distribution of the article with the strict proviso that no changes or edits are made and the original work is properly cited (including links to both the formal publication through the relevant DOI and the license). See: https://creativecommons.org/licenses/by-nc-nd/4.0/.
References
- Aronson PL, Thurm C, Alpern ER, et al. Variation in care of the febrile young infant <90 days in US pediatric emergency departments. Pediatrics 2014;134:667-77. Erratum in: Pediatrics 2015;135:775. [Crossref] [PubMed]
- Greenhow TL, Hung YY, Pantell RH. Management and Outcomes of Previously Healthy, Full-Term, Febrile Infants Ages 7 to 90 Days. Pediatrics 2016;138:e20160270. [Crossref] [PubMed]
- Chong SL, Ong GY, Chin WYW, et al. A retrospective review of vital signs and clinical outcomes of febrile infants younger than 3 months old presenting to the emergency department. PLoS One 2018;13:e0190649. [Crossref] [PubMed]
- Bargui F, D'Agostino I, Mariani-Kurkdjian P, et al. Factors influencing neurological outcome of children with bacterial meningitis at the emergency department. Eur J Pediatr 2012;171:1365-71. [Crossref] [PubMed]
- de Jonge RC, van Furth AM, Wassenaar M, et al. Predicting sequelae and death after bacterial meningitis in childhood: a systematic review of prognostic studies. BMC Infect Dis 2010;10:232. [Crossref] [PubMed]
- Huynh BT, Kermorvant-Duchemin E, Chheang R, et al. Severe bacterial neonatal infections in Madagascar, Senegal, and Cambodia: A multicentric community-based cohort study. PLoS Med 2021;18:e1003681. [Crossref] [PubMed]
- Cantey JB. The Spartacus Problem: Diagnostic Inefficiency of Neonatal Sepsis. Pediatrics 2019;144:e20192576. [Crossref] [PubMed]
- Yao SHW, Ong GY, Maconochie IK, et al. Analysis of emergency department prediction tools in evaluating febrile young infants at risk for serious infections. Emerg Med J 2019;36:729-35. [Crossref] [PubMed]
- Oliver EA, Reagan PB, Slaughter JL, et al. Patterns of Empiric Antibiotic Administration for Presumed Early-Onset Neonatal Sepsis in Neonatal Intensive Care Units in the United States. Am J Perinatol 2017;34:640-7. [PubMed]
- Lerwick JL. Minimizing pediatric healthcare-induced anxiety and trauma. World J Clin Pediatr 2016;5:143-50. [Crossref] [PubMed]
- Mintegi S, Bressan S, Gomez B, et al. Accuracy of a sequential approach to identify young febrile infants at low risk for invasive bacterial infection. Emerg Med J 2014;31:e19-24. [Crossref] [PubMed]
- Kuppermann N, Dayan PS, Levine DA, et al. A Clinical Prediction Rule to Identify Febrile Infants 60 Days and Younger at Low Risk for Serious Bacterial Infections. JAMA Pediatr 2019;173:342-51. [Crossref] [PubMed]
- Vos-Kerkhof E, Gomez B, Milcent K, et al. Clinical prediction models for young febrile infants at the emergency department: an international validation study. Arch Dis Child 2018;103:1033-41. [Crossref] [PubMed]
- Yang J, Ong WJ, Piragasam R, et al. Delays in Time-To-Antibiotics for Young Febrile Infants With Serious Bacterial Infections: A Prospective Single-Center Study. Front Pediatr 2022;10:873043. [Crossref] [PubMed]
- Yaeger JP, Jones J, Ertefaie A, et al. Refinement and Validation of a Clinical-Based Approach to Evaluate Young Febrile Infants. Hosp Pediatr 2022;12:399-407. [Crossref] [PubMed]
- Pelkonen T, Urtti S, Cardoso O, et al. Accuracy of Clinical and Cerebrospinal Fluid Indicators in the Diagnosis of Bacterial Meningitis in Infants <90 Days of Age in Luanda, Angola. Pediatr Infect Dis J 2021;40:e462-5. [Crossref] [PubMed]
- Tan VSR, Ong GY, Lee KP, et al. Pyrexia in a young infant - is height of fever associated with serious bacterial infection? BMC Pediatr 2022;22:188. [Crossref] [PubMed]
- Chong SL, Ong GY, Allen JC, et al. Early prediction of serious infections in febrile infants incorporating heart rate variability in an emergency department: a pilot study. Emerg Med J 2021;38:607-12. [Crossref] [PubMed]
- Heart rate variability: standards of measurement, physiological interpretation and clinical use. Task Force of the European Society of Cardiology and the North American Society of Pacing and Electrophysiology. Circulation 1996;93:1043-65. [Crossref] [PubMed]
- Badke CM, Marsillio LE, Weese-Mayer DE, et al. Autonomic Nervous System Dysfunction in Pediatric Sepsis. Front Pediatr 2018;6:280. [Crossref] [PubMed]
- Wee BYH, Lee JH, Mok YH, et al. A narrative review of heart rate and variability in sepsis. Ann Transl Med 2020;8:768. [Crossref] [PubMed]
- de Castilho FM, Ribeiro ALP, Nobre V, et al. Heart rate variability as predictor of mortality in sepsis: A systematic review. PLoS One 2018;13:e0203487. [Crossref] [PubMed]
- Chiew CJ, Wang H, Ong MEH, et al. Serial Heart Rate Variability Measures for Risk Prediction of Septic Patients in the Emergency Department. AMIA Annu Symp Proc 2019;2019:285-94. [PubMed]
- Arbo JE, Lessing JK, Ford WJH, et al. Heart rate variability measures for prediction of severity of illness and poor outcome in ED patients with sepsis. Am J Emerg Med 2020;38:2607-13. [Crossref] [PubMed]
- Barnaby DP, Fernando SM, Herry CL, et al. Heart Rate Variability, Clinical and Laboratory Measures to Predict Future Deterioration in Patients Presenting With Sepsis. Shock 2019;51:416-22. [Crossref] [PubMed]
- Liu N, Guo D, Koh ZX, et al. Heart rate n-variability (HRnV) and its application to risk stratification of chest pain patients in the emergency department. BMC Cardiovasc Disord 2020;20:168. [Crossref] [PubMed]
- Liu N, Chee ML, Foo MZQ, et al. Heart rate n-variability (HRnV) measures for prediction of mortality in sepsis patients presenting at the emergency department. PLoS One 2021;16:e0249868. [Crossref] [PubMed]
- Collins GS, Reitsma JB, Altman DG, et al. Transparent Reporting of a multivariable prediction model for Individual Prognosis or Diagnosis (TRIPOD): the TRIPOD statement. Ann Intern Med 2015;162:55-63. [Crossref] [PubMed]
- Nelson KG. An index of severity for acute pediatric illness. Am J Public Health 1980;70:804-7. [Crossref] [PubMed]
- Reaffirmation of AAP Clinical Practice Guideline. The Diagnosis and Management of the Initial Urinary Tract Infection in Febrile Infants and Young Children 2-24 Months of Age. Pediatrics 2016;138:e20163026. [Crossref] [PubMed]
- Available online: https://archive.physionet.org/tutorials/hrv-toolkit/
- Behar J, Johnson A, Clifford GD, et al. A comparison of single channel fetal ECG extraction methods. Ann Biomed Eng 2014;42:1340-53. [Crossref] [PubMed]
- Heldeweg ML, Liu N, Koh ZX, et al. A novel cardiovascular risk stratification model incorporating ECG and heart rate variability for patients presenting to the emergency department with chest pain. Crit Care 2016;20:179. [Crossref] [PubMed]
- Noorbakhsh KA, Ramgopal S, Rixe NS, et al. Risk-stratification in febrile infants 29 to 60 days old: a cost-effectiveness analysis. BMC Pediatr 2022;22:79. [Crossref] [PubMed]
- Coyle C, Brock G, Wallihan R, et al. Cost Analysis of Emergency Department Criteria for Evaluation of Febrile Infants Ages 29 to 90 Days. J Pediatr 2021;231:94-101.e2. [Crossref] [PubMed]
- Aronson PL, Wang ME, Shapiro ED, et al. Risk Stratification of Febrile Infants ≤60 Days Old Without Routine Lumbar Puncture. Pediatrics 2018;142:e20181879. [Crossref] [PubMed]
- Bonadio W. In Search of an Ideal Protocol to Distinguish Risk for Serious Bacterial Infection in Febrile Young Infants. J Pediatr 2021;231:32-4. [Crossref] [PubMed]
- Badke CM, Marsillio LE, Carroll MS, et al. Development of a Heart Rate Variability Risk Score to Predict Organ Dysfunction and Death in Critically Ill Children. Pediatr Crit Care Med 2021;22:e437-47. [Crossref] [PubMed]
- Marsillio LE, Manghi T, Carroll MS, et al. Heart rate variability as a marker of recovery from critical illness in children. PLoS One 2019;14:e0215930. [Crossref] [PubMed]
- Johnston BW, Barrett-Jolley R, Krige A, et al. Heart rate variability: Measurement and emerging use in critical care medicine. J Intensive Care Soc 2020;21:148-57. [Crossref] [PubMed]