The development of a nomogram model for predicting left recurrent laryngeal nerve lymph node metastasis in esophageal cancer based on radiomics and clinical factors
Highlight box
Key findings
• The radiomics features combined with clinical factors can effectively predict the metastasis of left RLNN in ESCC and can be used to guide the dissection of lymph nodes before surgery.
What is known and what is new?
• The extent of lymph node dissection in esophageal cancer is controversial. The dissection of left RLNN brings some postoperative complications while obtaining certain benefits. However, there is still a lack of a better noninvasive way to predict left RLNN metastasis.
• Radiomics features combined with clinical factors are used to construct a nomogram to predict left RLNN metastasis in ESCC.
What is the implication, and what should change now?
• This nomogram provides clinicians with a non-invasive auxiliary method to predict left RLNN metastasis in esophageal cancer to guide the formulation of precise and personalized preoperative lymph node dissection plans for clinical patients. More accurate and effective radiomics feature extraction methods need to be further developed.
Introduction
Esophageal cancer is a malignant digestive tract tumor. Progressive dysphagia is a typical symptom of esophageal cancer. Worldwide, esophageal cancer is the 7th most common cancer and ranks 6th in overall mortality (1). In China, esophageal cancer is the 6th most common cancer and the 4th leading cause of cancer-related death (2). According to the latest expert consensus, surgical resection is currently the most common and effective treatment for esophageal cancer (3), and lymph node metastasis (LNM) is the most critical factor affecting the prognosis of esophageal cancer patients. Thus, lymph node dissection is an essential part of radical surgery for esophageal cancer (4). However, the use of lymph node dissection in esophageal cancer is controversial (5,6).
Three-field lymph node dissection can effectively control local lesions, remove undetectable micro-metastases, help obtain accurate pathological staging, and improve the 5-year overall survival (OS) rate of patients with esophageal cancer. However, the surgical trauma is greater than two-field lymph node dissection, and it increases the risk of related complications and the incidence of postoperative mortality (4).
Due to the lymphatic drainage system of esophageal cancer, LNM of esophageal cancer can be upward, downward, or skipped. Upper esophageal cancer tends to metastasize upward, lower esophageal cancer tends to metastasize downward, and LNM of middle esophageal cancer can spread either upward or downward (7). Recurrent laryngeal nerve lymph nodes (RLNNs) are the common sites for esophageal cancer LNM. Metastatic lymph nodes can spread along the recurrent laryngeal nerve (RLN), from the chest to the neck. The particularity of the anatomical location of the left RLNNs adjacent to the aorta and the RLN and the long surgical path increases the difficulty of lymph node dissection and increases postoperative complications, such as hoarseness and the pulmonary infection incidence rate. The selection of the appropriate lymph node for dissection according to preoperative pathological results or preoperative examinations can enable the trauma caused by dissection of the left RLNNs to be avoided, improve the cure rate of esophageal cancer, and reduce surgical trauma and thus improve patients’ postoperative quality of life. Thus, the accurate preoperative determination of whether tumor metastasis occurs in the left RLNNs determines the extent of lymph node dissection in patients with esophageal cancer.
The gold standard for diagnosing LNM is pathological findings. A lymph node biopsy is an invasive procedure that can cause a certain degree of trauma to the patient, such as bleeding, pneumothorax, and even tumor dissemination. Invasive examinations, such as endoscopic ultrasonography and endoscopic ultrasonography fine needle aspiration place demanding requirements on the operator and carry certain risks, so it has limited application in the diagnose of LNMs in patients with esophageal cancer.
At present, the main non-invasive methods for preoperative lymph node evaluations in esophageal cancer patients include ultrasound, contrast-enhanced computed tomography (CT), magnetic resonance imaging, and positron emission tomography-CT (PET-CT). In previous studies, contrast-enhanced CT and PET-CT have shown certain sensitivity and specificity in the clinical prediction of LNM in patients with esophageal cancer (8-10). However, there are still some limitations in predicting LNM in esophageal cancer.
A study has shown that CT has a lower accuracy in predicting lymph node involvement than endoscopic ultrasound (EUS) at 46–58%, compared to 72–80%. The sensitivity of 2-fluoro-2-deoxy-D-glucose positron emission tomography (FDG-PET) was 57% (range, 43–70%) and the specificity was 85% (range, 76–95%) (10). Some prediction models based on clinical data to predict LNM of esophageal squamous cell carcinoma (ESCC) mostly use the short diameter of lymph nodes as a predictor. Although they can achieve certain accuracy, the short diameter of lymph nodes as a threshold for predicting LNM is still controversial, and reactive lymph node enlargement easily causes false positives
CT is a traditional non-invasive method for obtaining tumor-related information. With continuous developments in medical imaging, Radiomics focuses on improvements of image analysis through the automatic analysis of a given imaging method. Medical imaging data can be quantitatively analyzed using automated or semi-automated software for a given imaging modality to identify additional tumor features that are difficult to identify or quantify with the naked eye, such as shape and heterogeneity (11). The tumor region of interest (ROI) is delineated by automated segmentation methods or an experienced radiologist, and the radiomics features are subsequently extracted from the ROI. These features include the shape feature, first-order feature, grayscale co-occurrence matrix feature (GLCM), grayscale region size matrix feature (GLSZM), grayscale distance region matrix feature (GLDZM), grayscale run-length matrix feature (GLRLM), neighborhood grayscale difference matrix feature (NGTDM), grayscale correlation matrix, and wavelet feature (12). The extracted radiomics features can be further screened, and the relationship between the selected features can then be analyzed (11).
In this study, we segmented the ROI of the left RLNN from the contrast-enhanced CT scans of patients with ESCC, extracted and then filtered the radiomics features of the left RLNNs, and finally included the patient’s clinical data in the prediction model to predict the metastasis of the left RLNNs and analyzed its accuracy through internal verification. We present the following article in accordance with the TRIPOD reporting checklist (available at https://atm.amegroups.com/article/view/10.21037/atm-22-5628/rc).
Methods
Study design
The sample size of this study was initially set based on 10 events per variable (EPV). Due to the large number of radiomics features, the sample size required according to this principle is very large. However, the amount of data that patients diagnosed with ESCC who underwent radical resection of esophageal cancer in our hospital is limited. There were 511 patients with ESCC who underwent radical resection of esophageal cancer in our hospital from 2015 to 2020. Excluding patients who had received preoperative anti-tumor treatment (104 cases), combined with other malignant tumors (18 cases), and missing clinical data (39 cases), the clinical data of 350 patients were finally collected and the radiomics features were segmented and extracted. Among them, 55 patients had left RLNN metastasis. The postoperative pathology confirmed that the left RLNN metastasis rate is about 11.7%, which is consistent with the study of Kanemura et al. (13). In order to solve the problem of too many independent variables and small sample size, we used least absolute shrinkage and selection operator (LASSO) analysis and stepwise regression to preprocess the radiomics features, and finally screened out 6 radiomics features to be included in the construction of the model. Due to the small sample size, this study did not divide the training set and validation set at the beginning but used 10-fold cross-validation and bootstrap sampling verification for internal validation after the model was established.
Patient information
The study was conducted in accordance with the Declaration of Helsinki (as revised in 2013). The study was approved by Ethics Committee-Scientific Research Subcommittee of Zhongshan Hospital Affiliated to Xiamen University (No. 2022-256). Individual consent for this retrospective analysis was waived. This study included 350 patients who were diagnosed with ESCC and underwent surgical treatment at the Zhongshan Hospital Affiliated to Xiamen University from 2015 to 2020. Of the patients, 282 were male and 68 were female. The patients were aged 36–82 years, and had an average age of 61.55±8.41 years. Further, 55 patients tested positive for metastasis to the left RLNN, and 295 patients tested negative for metastasis to the left RLNN.
To be eligible for inclusion in this study, patients had to meet the following inclusion criteria: (I) have been diagnosed with ESCC and treated with surgery; (II) have a gold standard postoperative pathology report; (III) have undergone a contrast-enhanced CT examination within 1 month of surgery, and have image data that could be exported; and (IV) have complete clinical data. Patients were excluded from the study if they met any of the following exclusion criteria: (I) had other concurrent malignant tumors; (II) had undergone preoperative radiotherapy, chemotherapy, and other anti-tumor treatments.
In this study, age, gender, smoking history, drinking history, the contrast-enhanced CT value of the primary lesion, the contrast-enhanced CT value of the left RLNN, the difference between the contrast-enhanced CT value and the non-enhanced CT value of the left RLNN, the difference of contrast-enhanced CT value between the left RLNN and the primary lesion, tumor location, lesion length, and degree of tumor differentiation were included.
CT image
All the patients received routine and contrast-enhanced CT of the thorax using a multi-detector CT system (Brilliance ICT; Philips Medical Systems). The scanning range was from the mandible to the costophrenic angle level. The contrast-enhanced CT scan parameters were 120 kVp, with automatic tube current modulation, and a matrix size of 512×512. An injection of Iopamidol (300 mg/mL) was administered as a contrast medium at a dose of 70 mL and a rate of 4.0 mL/s.
Image processing and radiomics feature extraction and screening
The chest contrast-enhanced CT scans (venous-phase scans with a slice thickness of 2.5 mm) of the patients were exported in the Digital Imaging and Communications in Medicine (DICOM) format and then imported into the 3D Slicer 4.11, an open-source radiomics extraction toolkit, and the ROI of the left RLNNs was segmented manually by 2 experienced thoracic surgeons (Figure 1). Next, 3D reconstruction was performed, and the radiomics plugin was used to automatically calculate and extract the radiomics of the left RLNNs, including the shape features, first-order feature, GLCM, GLSZM, GLDZM, GLRLM, and NGTDM. The extracted radiomics features were included in the LASSO regression for variable screening, and the variables corresponding to the optimal λ were selected.
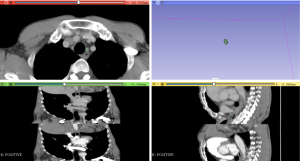
Construction of the nomogram and evaluation
The radiomics features screened by LASSO-logistic regression and the variables with P values <0.05 among clinical indicators were included in the multivariate logistic regression model, and the variables were screened using the stepwise method, after which, the nomogram for predicting the metastasis of the left RLNNs was finally established. The receiver operator characteristic (ROC) curve was used to evaluate the predictive ability of the model. The 10-fold cross-validation was used for the internal validation of the model. The Bootstrap method was repeatedly used 2,000 times to draw the calibration curve. Harrell’s C statistic was used to calculate the concordance index (C-index) to evaluate the calibration of the nomogram model.
Statistical analysis
SAS 9.4 was used for the statistical analysis. The counting data are expressed as the rates, and the comparisons between groups were performed using the chi-square test. The normally distributed measurement data are described as the mean plus or minus the standard deviation, and the comparisons between groups were performed by the 2 independent samples t-test. P value <0.05 (two sides) was considered statistically significant. The radiomics features were screened by LASSO-logistic regression. The variables were screened by the stepwise method, and the nomogram was constructed by the multivariate logistic regression. The R 3.6.1 software “rms” package was used to establish the nomogram. ROC curves were used to evaluate the predictive ability of the model. The 10-fold cross-validation was used for the internal validation of the model. The Bootstrap method was used to draw the calibration curve. Harrell’s C statistic was used to evaluate the calibration of the model.
Results
Analysis of clinical indicators
The clinical indicators of the 350 patients were included in this study, and the results are shown in Table 1. According to the univariate analysis results, the enhanced CT value of the left RLNNs, the difference between the contrast-enhanced CT values between the left recurrent nerve and the primary lesion, and the tumor location were statistically significant (all P<0.05).
Table 1
Variables | Total | Negative (n=295) | Positive (n=55) | t/Z/χ2 | P |
---|---|---|---|---|---|
Age, mean ± SD (years) | 61.55±8.41 | 61.43±8.47 | 62.16±8.11 | –0.591 | 0.555 |
Gender, n (%) | |||||
Male | 282 (80.57) | 236 (80.00) | 46 (83.64) | 0.392 | 0.531 |
Female | 68 (19.43) | 59 (20.00) | 9 (16.36) | ||
Smoking history, n (%) | |||||
No | 124 (35.43) | 105 (35.59) | 19 (34.55) | 0.022 | 0.881 |
Yes | 226 (64.57) | 190 (64.41) | 36 (65.45) | ||
Drinking history, n (%) | |||||
No | 242 (69.14) | 199 (67.46) | 43 (78.18) | 2.499 | 0.114 |
Yes | 108 (30.86) | 96 (32.54) | 12 (21.82) | ||
Contrast-enhanced CT value of primary lesion, mean ± SD | 86.09±14.06 | 85.7±14.06 | 88.16±14.05 | –1.191 | 0.234 |
Contrast-enhanced CT value of left RLNN, mean ± SD | 37.86±30.26 | 35.87±32.38 | 48.52±8.61 | –5.712 | <0.001* |
Difference between the contrast-enhanced CT value and the non-enhanced CT value of left RLNN, mean ± SD | 25.53±16.23 | 26.26±16.48 | 21.62±14.29 | 1.955 | 0.051 |
Difference of contrast-enhanced CT value between left RLNN and the primary lesion, mean ± SD | 48.22±30.29 | 49.83±32.35 | 39.64±11.94 | 4.112 | <0.001* |
Tumor location, n (%) | 13.333 | 0.001* | |||
Upper | 34 (9.71) | 32 (10.85) | 2 (3.64) | ||
Middle | 246 (70.29) | 196 (66.44) | 50 (90.91) | ||
Low | 70 (20.00) | 67 (22.71) | 3 (5.45) | ||
Lesion length, n (%) | 2.038 | 0.361 | |||
<3 cm | 68 (19.43) | 57 (19.32) | 11 (20.00) | ||
3–5 cm | 206 (58.86) | 170 (57.63) | 36 (65.45) | ||
>5 cm | 76 (21.71) | 68 (23.05) | 8 (14.55) | ||
Degree of tumor differentiation, n (%) | 0.581 | 0.748 | |||
G1 | 14 (4.00) | 11 (3.73) | 3 (5.45) | ||
G2 | 279 (79.71) | 237 (80.34) | 42 (76.36) | ||
G3 | 57 (16.29) | 47 (15.93) | 10 (18.18) |
*, P<0.05 was considered statistically significant. RLNN, recurrent laryngeal nerve lymph node; CT, computed tomography.
Screening of radiomics features
The 3D slicer 4.11 extracted 107 radiomics features. The extracted radiomics features were included in the LASSO-logistic regression for variable screening, and the curve of the regression coefficient changes with log (λ) is shown in Figure 2A. The results showed that the optimal λ value with log (λ) =−3.22 was chosen when the deviation was the smallest. The 6 variables with non-zero coefficients were selected, and the area under the curve (AUC) value was 0.884, which was a small distance from the maximum AUC, as shown in Figure 2B. The 6 variables identified were the least axis length, maximum 2D diameter column, maximum 2D diameter row, surface volume ratio, difference entropy, and run-length non-uniformity.
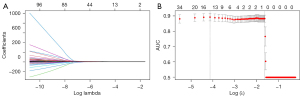
Analysis results of the logistic regression model
The statistically significant variables (P<0.05) among the clinical indicators (i.e., the contrast-enhanced CT value of the left RLNNs, the difference of contrast-enhanced CT value between the left RLNNs and the primary lesion, and tumor location) and the variables identified by the LASSO-logistic regression (i.e., the least axis length, maximum 2D diameter column, maximum 2D diameter row, surface volume ratio, difference entropy, and run-length non-uniformity) were included in the multivariate logistic regression model, and the stepwise method was used to screen the variables. Finally, we identified the most meaningful predictors, including the tumor location, surface volume ratio, and run-length non-uniformity. The results are shown in Table 2.
Table 2
Variables | β | Standard error | Wald χ2 | P | OR (95% CI) |
---|---|---|---|---|---|
Constant | –3.651 | 1.32 | 7.65 | 0.006 | |
Tumor location | |||||
Upper | –0.341 | 1.367 | 0.062 | 0.803 | 0.711 (0.049, 10.352) |
Middle | 1.923 | 0.718 | 7.182 | 0.007 | 6.841 (1.676, 27.921) |
Low | 1.0 (reference) | ||||
Surface volume ratio | –1.084 | 0.498 | 4.732 | 0.03 | 0.338 (0.127, 0.898) |
Run-length non-uniformity | 0.036 | 0.007 | 27.828 | <0.001 | 1.037 (1.023, 1.051) |
RLNN, recurrent laryngeal nerve lymph node.
Model construction and evaluation
Nomogram construction and use
According to the results of the multivariate logistic regression analysis, an individualized and visualized nomogram model for predicting left RLNNs metastasis in patients with ESCC was constructed. As Figure 3 shows, the scores (points) corresponding to the individual indicators of the patient in the model were added to obtain the total score (total points), and a vertical curve was drawn to the predicted probability axis to obtain the probability of metastasis of left RLNN. The result suggests that the patient has left RLNNs metastasis when the total points are ≥32 points [P (metastasis) ≥0.10; the cut-off value].
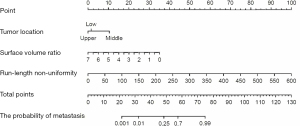
Evaluation of the model
The ROC curve was plotted, and the AUC value was used to assess model prediction performance. The AUC value of the nomogram model in this study was 0.903 [95% confidence interval (CI): 0.861–0.945; P<0.001; Figure 4], indicating that the model had an excellent ability to predict left RLNN metastasis. The Brier score of this model was 0.074, which was <0.25, indicating that the model’s predicted values were close to the actual values. The accuracy of the model was evaluated by 10-fold cross-validation, and the of are shown in Table 3. The validation set had an accuracy of 76.60%, a sensitivity of 85.08%, and a specificity of 75.49%. The results of the training set and the validation set were similar, indicating that the model was stable. After the Bootstrap sampling verification of the nomogram model (n=2,000), the C-index was 0.904, indicating good calibration. The correction curve (Figure 5) shows that the prediction results obtained by the model were in good agreement with the actual observations, with a mean absolute error of 0.028.
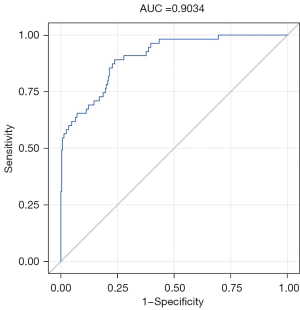
Table 3
Correct rate, % | Sensitivity, % | Specificity, % | |
---|---|---|---|
Training set | 77.74 | 88.08 | 75.81 |
Validation set | 76.60 | 85.08 | 75.49 |
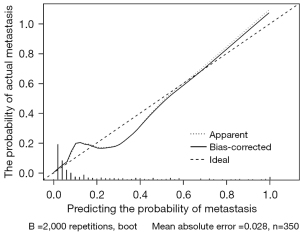
Discussion
Esophageal cancer is one of the most aggressive types of cancer, and due to the high incidence of LNM, patients have a poor prognosis. LNM is the most critical factor affecting the prognosis of esophageal cancer patients, and lymph node dissection is an integral part of radical surgery for esophageal cancer. However, the use of lymph node dissection in esophageal cancer is still controversial. According to a meta-analysis, a 3-field lymphadenectomy is associated with a significantly improved 5-year OS, but no significant differences between 3-field lymphadenectomy and 2-field lymphadenectomy were found in terms of postoperative mortality, anastomotic leak, pulmonary complications, chylothorax, and recurrent nerve palsy (14). Conversely, Koterazawa’s meta-analysis showed that OS did not differ significantly between the 3- and 2-field lymphadenectomy groups, but the incidence of RLN palsy was significantly higher in the 3-field lymphadenectomy than the 2-field lymphadenectomy group (15).
Regional lymph node stations of metastasis are most frequently observed in the RLNN; however, the rates of metastasis to the to the left and right RLNN are similar. The left RLNNs, whose anatomical pathway is longer that its right counterpart, runs over a broad region, along the RLN, from the upper mediastinum to the neck, and thus can be more difficult to remove surgically. Moreover, it is located in front of the trachea, which increases the difficulty of the right thoracotomy operation (13). Additionally, left RLNN adjacent to the aorta and a left RLN lymphadenectomy also increase postoperative respiratory complications (including dyspnea, pneumonia, aspiration, and acute respiratory distress syndrome), increase the incidence of esophagogastric anastomotic fistulas, and prolong postoperative hospital stays. Left RLN palsy is more common than right RLN because of its longer path and deeper location (16). According to Pai’s study of patients with clinical positive RLN lymph node involvement before treatment, there were significant differences in the OS and disease-free survival (DFS) between patients with and without RLN lymphadenectomy; however, no significant differences were found in the 3-year OS and DFS between patients with and without RLN lymphadenectomy in the entire cohort or the neoadjuvant chemoradiotherapy group (17).
Preoperative examinations of the left RLNN metastasis determine the choice of lymph node dissection in patients with esophageal cancer. Thus, predicting the left RLNNs metastasis using a non-invasive method would enable clinicians to formulate individualized lymph node dissection plans for patients with ESCC before surgery. This study sought to establish a prediction model for left RLNN metastasis in ESCC to guide clinicians in formulating personalized and precise lymph node dissection plans for patients with esophageal cancer before surgery.
Radiomics focuses on improving image analysis, using an automated high-throughput extraction of large amounts of quantitative features of medical images, and is a recent innovation in medical imaging analysis. Radiomics, as the advanced image analysis of novel medical imaging, can capture additional information not currently used, and more specifically, genomics and proteomic patterns that can be expressed in terms of macroscopic image-based features (9). Thus, this study constructed a predictive model for left RLNN metastasis in ESCC patients based on radiomics and clinical data.
Relevant study has shown that LNM of esophageal cancer is associated with the tumor location, lesion length, depth of invasion, degree of differentiation, and the presence of vascular tumor thrombus (18). A previous study showed that PIM-1, a serine/threonine protein kinases of the PIM (Proviral Integration of Moloney virus) family, is a promising molecular marker for predicting LNM in early esophagus cancer (19). However, as only 67 samples were included in that research, and a single data source was used, the study lacked generalisability. As clinical data for molecular marker was lacking at our research center, it was not included in the univariate analysis of the clinical data in this study. In clinical practice, the short diameter of the lymph node ≥1.0 cm is often used as the diagnostic criterion for positive LNM, but the clinical diagnostic criterion for the short diameter of metastatic lymph nodes is still controversial (20). Additionally, as lymph node enlargement cannot exclude other possibilities, such as inflammatory cell infiltration and tissue hyperplasia, this indicator was not included in the univariate analysis of the clinical data in this study.
The following preoperative clinical indicators were included in this study: age, gender, smoking history, drinking history, the contrast-enhanced CT value of the primary lesion, the contrast-enhanced CT value of the left RLNNs, the difference between the contrast-enhanced CT value and the non-enhanced CT value of the left RLNNs, the difference between the contrast-enhanced CT value between the left RLNNs and the primary lesion, tumor location, lesion length, and degree of tumor differentiation. According to the univariate analysis results, the contrast-enhanced CT value of the left RLNNs, the difference of contrast-enhanced CT value between the left recurrent nerve and the primary lesion, and the tumor location were statistically significant (all P<0.05).
LASSO is a widely used data screening method that shrinks the coefficients and changes some coefficients to 0, and LASSO can be used for feature reduction and selection (21). Studies have shown that the method is suitable for processing large numbers of radiomics with relatively small sample sizes and avoiding overfitting (22,23).
The AUC of the prediction model reached 0.903 (95% CI: 0.861–0.945), indicating that the prediction model had excellent prediction performance. Further, 10-fold cross-validation was used to verify the internal validation of the model. The results of the training set and the validation set were similar, indicating that the model was stable. The Bootstrap method was used to draw the calibration curve, and the nomogram model was internally verified. Harrell’s C statistic was used to calculate the C-index to evaluate the calibration of the nomogram model, and the C-index for predicting malignancy was 0.904, indicating that the model had a good degree of calibration, and the prediction results obtained by the model were close to the actual observations.
This study had a number of limitations. First, the data in this study were all obtained from the Zhongshan Hospital Affiliated to Xiamen University, and the sample size was not sufficiently large. In future research, it is hoped that data from multicenter sources can be collected, and the external verification of the model can be further improved. Second, the elevation of some tumor markers, such as abnormal tumor protein PMI-1, is associated with LNM in esophageal cancer. However, as the study lacked complete clinical data for tumor markers, some tumor markers were not included in the univariate analysis. The inclusion of specific tumor markers in subsequent studies may increase the accurate predictive ability and clinical application value of the constructed predictive models. Third, in the process of radiomics extraction in this study, the ROIs of the left RLNNs on chest contrast-enhanced CT scans in patients with ESCC were manually outlined, which took a great deal of time and effort and were affected by some fatty tissues next to the lymph nodes. Further developments need to be made in medical imaging techniques and the accuracy and precision of lymph node segmentation needs to be improved. Thus, in future research, we hope to obtain data from multicenter sources, increase the sample size, include more meaningful clinical indicators, and extract radiomics features in a more scientific and effective way to improve the predictive ability and clinical application value of the predictive model.
Conclusions
A visual nomogram model combining clinical data and radiomics features was established to predict left RLNN metastasis in patients with ESCC in a non-invasive way. This model can provide a reference for clinicians to formulate individualized lymph node dissection plans for patients with ESCC. According to the ROC curve, the AUC of the model was 0.903, which means that the model had an excellent predictive ability. Combined with the internal verification results, our results indicated that the predictive model was stable and had a high degree of calibration.
Acknowledgments
Funding: This work was supported by the National Natural Science Foundation of China (No. 82073405), and the Science and Projects of Xiamen Science and Technology Commission (No. 3502Z20184032).
Footnote
Reporting Checklist: The authors have completed the TRIPOD reporting checklist. Available at https://atm.amegroups.com/article/view/10.21037/atm-22-5628/rc
Data Sharing Statement: Available at https://atm.amegroups.com/article/view/10.21037/atm-22-5628/dss
Conflicts of Interest: All authors have completed the ICMJE uniform disclosure form (available at https://atm.amegroups.com/article/view/10.21037/atm-22-5628/coif). The authors have no conflicts of interest to declare.
Ethical Statement: The authors are accountable for all aspects of the work in ensuring that questions related to the accuracy or integrity of any part of the work are appropriately investigated and resolved. The study was conducted in accordance with the Declaration of Helsinki (as revised in 2013). The study was approved by Ethics Committee-Scientific Research Subcommittee of Zhongshan Hospital Affiliated to Xiamen University (No. 2022-256). Individual consent for this retrospective analysis was waived.
Open Access Statement: This is an Open Access article distributed in accordance with the Creative Commons Attribution-NonCommercial-NoDerivs 4.0 International License (CC BY-NC-ND 4.0), which permits the non-commercial replication and distribution of the article with the strict proviso that no changes or edits are made and the original work is properly cited (including links to both the formal publication through the relevant DOI and the license). See: https://creativecommons.org/licenses/by-nc-nd/4.0/.
References
- Bray F, Ferlay J, Soerjomataram I, et al. Global cancer statistics 2018: GLOBOCAN estimates of incidence and mortality worldwide for 36 cancers in 185 countries. CA Cancer J Clin 2018;68:394-424. [Crossref] [PubMed]
- Cao W, Chen HD, Yu YW, et al. Changing profiles of cancer burden worldwide and in China: a secondary analysis of the global cancer statistics 2020. Chin Med J (Engl) 2021;134:783-91. [Crossref] [PubMed]
- Kakeji Y, Oshikiri T, Takiguchi G, et al. Multimodality approaches to control esophageal cancer: development of chemoradiotherapy, chemotherapy, and immunotherapy. Esophagus 2021;18:25-32. [Crossref] [PubMed]
- Li H, Fang W, Yu Z, et al. Chinese expert consensus on mediastinal lymph node dissection in esophagectomy for esophageal cancer (2017 edition). J Thorac Dis 2018;10:2481-9. [Crossref] [PubMed]
- Yamashita K, Makino T, Yamasaki M, et al. Comparison of short-term outcomes between 2- and 3-field lymph node dissection for esophageal cancer. Dis Esophagus 2017;30:1-8. [Crossref] [PubMed]
- Li B, Hu H, Zhang Y, et al. Three-field versus two-field lymphadenectomy in transthoracic oesophagectomy for oesophageal squamous cell carcinoma: short-term outcomes of a randomized clinical trial. Br J Surg 2020;107:647-54. [Crossref] [PubMed]
- Hagens ERC, van Berge Henegouwen MI, Gisbertz SS. Distribution of Lymph Node Metastases in Esophageal Carcinoma Patients Undergoing Upfront Surgery: A Systematic Review. Cancers (Basel) 2020.
- Lopci E, Kauppi J, Lugaresi M, et al. Siewert type I and II oesophageal adenocarcinoma: sensitivity/specificity of computed tomography, positron emission tomography and endoscopic ultrasound for assessment of lymph node metastases in groups of thoracic and abdominal lymph node stations. Interact Cardiovasc Thorac Surg 2019;28:518-25. [Crossref] [PubMed]
- Li B, Li N, Liu S, et al. Does [18F] fluorodeoxyglucose-positron emission tomography/computed tomography have a role in cervical nodal staging for esophageal squamous cell carcinoma? J Thorac Cardiovasc Surg 2020;160:544-50. [Crossref] [PubMed]
- Schmidlin EJ, Gill RR. New frontiers in esophageal radiology. Ann Transl Med 2021;9:904. [Crossref] [PubMed]
- Rogers W, Thulasi Seetha S, Refaee TAG, et al. Radiomics: from qualitative to quantitative imaging. Br J Radiol 2020;93:20190948. [Crossref] [PubMed]
- Mayerhoefer ME, Materka A, Langs G, et al. Introduction to Radiomics. J Nucl Med 2020;61:488-95. [Crossref] [PubMed]
- Kanemura T, Makino T, Miyazaki Y, et al. Distribution patterns of metastases in recurrent laryngeal nerve lymph nodes in patients with squamous cell esophageal cancer. Dis Esophagus 2017;30:1-7. [PubMed]
- Bona D, Lombardo F, Matsushima K, et al. Three-field versus two-field lymphadenectomy for esophageal squamous cell carcinoma: A long-term survival meta-analysis. Surgery 2022;171:940-7. [Crossref] [PubMed]
- Koterazawa Y, Oshikiri T, Takiguchi G, et al. Prophylactic Cervical Lymph Node Dissection in Thoracoscopic Esophagectomy for Esophageal Cancer Increases Postoperative Complications and Does Not Improve Survival. Ann Surg Oncol 2019;26:2899-904. [Crossref] [PubMed]
- Chen WS, Zhu LH, Li WJ, et al. Novel technique for lymphadenectomy along left recurrent laryngeal nerve during thoracoscopic esophagectomy. World J Gastroenterol 2020;26:1340-51. [Crossref] [PubMed]
- Pai CP, Hsu PK, Chien LI, et al. Clinical outcome of patients after recurrent laryngeal nerve lymph node dissection for oesophageal squamous cell carcinoma. Interact Cardiovasc Thorac Surg 2022;34:393-401. [Crossref] [PubMed]
- Wang A, Lu L, Fan J, et al. Lymph node metastatic patterns and its clinical significance for thoracic superficial esophageal squamous cell carcinoma. J Cardiothorac Surg 2020;15:262. [Crossref] [PubMed]
- Plum PS, Warnecke-Eberz U, Dhaouadi O, et al. Molecular markers predicting lymph node metastasis in early esophageal cancer. Histol Histopathol 2015;30:1193-202. [PubMed]
- Lee HN, Kim JI, Shin SY, et al. Combined CT texture analysis and nodal axial ratio for detection of nodal metastasis in esophageal cancer. Br J Radiol 2020;93:20190827. [Crossref] [PubMed]
- Hepp T, Schmid M, Gefeller O, et al. Approaches to Regularized Regression - A Comparison between Gradient Boosting and the Lasso. Methods Inf Med 2016;55:422-30. [Crossref] [PubMed]
- Peng H, Dong D, Fang MJ, et al. Prognostic Value of Deep Learning PET/CT-Based Radiomics: Potential Role for Future Individual Induction Chemotherapy in Advanced Nasopharyngeal Carcinoma. Clin Cancer Res 2019;25:4271-9. [Crossref] [PubMed]
- Sauerbrei W, Perperoglou A, Schmid M, et al. State of the art in selection of variables and functional forms in multivariable analysis-outstanding issues. Diagn Progn Res 2020;4:3. [Crossref] [PubMed]