Exploring biomarkers and transcriptional factors in type 2 diabetes by comprehensive bioinformatics analysis on RNA-Seq and scRNA-Seq data
Introduction
Diabetes mellitus (DM) is a chronic disease characterized by high blood sugar levels, caused by insufficient insulin production by the pancreas or an inappropriate response of the body's cells to insulin (1). DM is generally classified into two forms: type 1 diabetes (T1D) and type 2 diabetes (T2D). Of these, T2D is the most common type of diabetes, accounting for 90–95% of all diabetes cases, and is primarily characterized by pancreatic beta-cell dysfunction and insulin resistance (2). T2D can lead to serious clinical complications such as diabetic cardiomyopathy, retinopathy, nephropathy, and neuropathy, with high rates of disability and mortality (3). As lifestyle habits have changed, the number of T2D patients globally has increased significantly. According to the World Health Organization (WHO), T2D affected 425 million people worldwide in 2017 and is expected to affect 629 million people by 2045 (4). At present, clinically, the early diagnosis of T2D lacks reliable biomarkers, and its pathogenesis has not been fully studied, and the treatment is still challenging (5). Although some glycemic control drugs are used to treat advanced T2D, complete remission is rare among patients (6). Therefore, the study of early diagnostic markers and molecular pathology of T2D is of great significance for the clinical treatment of T2D.
RNA-sequencing (RNA-seq) is a precise and sensitive technique for examining global gene expression profiles (7). providing new and powerful means to study the pathogenesis of complex diseases such as T2D (8). In the past, studies have tried to find the biomarker genes of T2D through RNA-seq, and proposed the possible molecular mechanism. Che et al. proposed that 10 hub genes including CNOT6L, and CNOT6, etc, are involved in the pathogenesis of T2D (9). Transcriptomic analysis also found that two core genes, SERPING1 and ANPEP, were associated with the development of T2D (10). Whether these genes can work as clinical biomarkers of T2D, there is a lack of modeling verification of large-scale clinical samples. In addition, a limitation of RNA-seq methods is that bulk RNA-seq cannot capture the heterogeneity of each sample (11). Furthermore, heterogeneity may exist even within a single disease (12). Single-cell RNA sequencing (scRNA-seq) is a new technology to reveal cellular heterogeneity, which not only overcomes the limitations of traditional RNA-sequencing techniques in detecting small expression differences between cells (13), but also clearly shows gene expression profiles of various cell types, as well as specific cellular functional alterations.
In this study, we obtained RNA-seq data from healthy and T2D patient cohorts from the Gene Expression Omnibus (GEO) database. Subsequently, we performed Gene Ontology (GO) functional enrichment analysis and Kyoto Encyclopedia of Genes and Genomes (KEGG) enrichment pathway analysis to explore the biological functions and enrichment pathways of differentially expressed genes (DEGs). Importantly, we also applied the Least Absolute Shrinkage and Selection Operator (LASSO) algorithm and Nomogram establishment, demonstrating that SLC2A2, SERPINF1, RASGRP1 and CHL1 are reliable biomarkers of T2D. Finally, we annotated cell clusters by analyzing single-cell sequencing data of human islet cells, and screened key cell clusters using biomarker expression levels, and finally identified biomarker transcription factors (TFs). Our findings suggest that aberrantly expressed SERPINF1 in fibroblasts may be regulated by NR2F2, which provides new insights into the treatment of T2D. We present the following article in accordance with the TRIPOD reporting checklist (available at https://atm.amegroups.com/article/view/10.21037/atm-22-4303/rc).
Methods
Data source
We downloaded the GSE41762 dataset containing RNA-seq data from the islet tissues of 57 healthy participants and 20 T2D patients from the GEO database (https://www.ncbi.nlm.nih.gov/geo/query/acc.cgi?acc=GSE41762). Moreover, the scRNA-seq data of pancreatic tissue and islets of six healthy controls and four T2D donors was downloaded from the ArrayExpress database (registration number: “E-MTAB-5061”) (https://www.ebi.ac.uk/arrayexpress/experiments/E-MTAB-5061/). The design and analysis flow of this study was shown in Figure S1. The study was conducted in accordance with the Declaration of Helsinki (as revised in 2013).
Differential expression analysis
The “limma” R package (https://bioconductor.org/packages/release/bioc/html/limma.html) was applied to screen DEGs between the healthy and T2D samples with a setting of |log2 fold change (FC)| >0.5 and P<0.05 (14). Next, the volcano plot of DEGs was displayed using the “ggplot2” R package, and the heatmap of DEGs was plotted using the “heatmap” R package.
Functional enrichment analysis
The “clusterProfiler” package in R language was used to perform the GO and KEGG enrichment analyses of DEGs (15). P<0.05 was considered to indicate significant enrichment.
Biomarkers screening
The LASSO algorithm in R language was used to screen biomarkers for T2D. Next, the ability of biomarkers to distinguish healthy from T2D patients was analyzed using receiver operating characteristic (ROC) curves of the “pROC” package by calculating the area under curve (AUC) values of the ROC curves. Genes with AUC values greater than 0.8 were defined as biomarkers.
Nomogram establishment
A nomogram based on the biomarkers was constructed using the “lrm” function in the R package to predict the probability of illness. Moreover, calibration curves were displayed using the “rms” package, decision curves were constructed by “ggDCA” package and ROC curves were applied to verify the validity of the nomogram.
Single-cell analysis
The “Seurat” R package was utilized to process the scRNA-seq data. The data were initially filtered according to the following criteria: (I) genes that were only expressed in ≤3 cells; (II) low-quality cells with <100 genes; and (III) cells with >10,000 genes. Next, the retained cells were defined as core cells. Moreover, analysis of variance (ANOVA) as performed on the genes of core cells to screen out the highly variable genes (16). Moreover, the gene expression of core cells was normalized through a linear regression model, and principal components analysis (PCA) was performed to illustrate the distribution of core cells. Next, the top 20 principal components were selected for data dimensionality reduction using the tSNE algorithm in the R language. The “FindAllMarker” function of the “Seurat” R package was employed to identify marker genes and used these marker genes to annotate different cell clusters through the R package “single” and “CellMarker” database (17). Also, the expression levels of biomarkers were utilized to screen the key cell clusters.
The fibroblasts were separated into high and low expression groups according to the expression of biomarkers. Gene set variation analysis (GSVA) was used to analyze the functional enrichment of all genes in the biomarker genome. Differences in the GSVA scores (which we denoted as “t”) between the high and low biomarker expression groups were analyzed using the “limma” R package (18).
SCENIC analysis
Single-cell regulatory network inference and clustering (SCENIC) analysis was performed on the high and low biomarker expression groups using the “SCENIC” package in R language to identify the TFs (19).
Statistical analysis
All analyses were conducted using the R programming language. If not specified above, a P value (two-sided) less than 0.05 was considered statistically significant.
Results
Analysis of T2D-related DEGs
Following analysis using the “limma” package, a total of 111 DEGs between the control and T2D groups were obtained, including 68 up-regulated genes and 43 down-regulated genes (Figure 1A,1B, https://cdn.amegroups.cn/static/public/atm-22-4303-1.xls). The GO analysis results showed that 111 genes were enriched, with a total of 636 GO terms, including the regulation of hormone secretion, positive regulation of the MAPK (mitogen activated protein kinase) cascade, metabolic process of collagen, etc. (Figure 2A, https://cdn.amegroups.cn/static/public/atm-22-4303-2.xls). In addition, these DEGs were involved in diabetes at maturity, pancreatic secretion, the Ras signaling pathway, etc. (Figure 2B, https://cdn.amegroups.cn/static/public/atm-22-4303-3.xls).
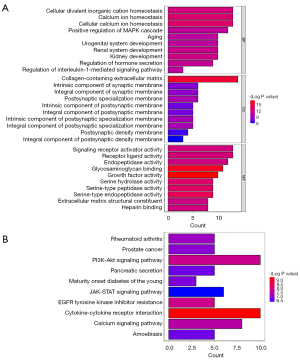
Identification of biomarkers
To further screen out the biomarkers in T2D, the LASSO algorithm was executed based on the 111 DEGs. Based on λmin =0.1169, a total of six genes were extracted, namely SLC2A2, SERPINF1, RASGRP1, CHL1, PGC, and IAPP (Figure 3A,3B, https://cdn.amegroups.cn/static/public/atm-22-4303-4.xls).
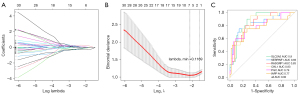
The gene with the highest AUC value was SLC2A2 (AUC =0.9), followed by those of SERPINF1, RASGRP1, and CHL1 (AUC =0.83), and the AUCs of PGC and IAPP were 0.79 and 0.77, respectively. Notably, SLC2A2, SERPINF1, RASGRP1, and CHL1, which had AUCs >0.8, were screened as biomarkers for subsequent analysis (Figure 3C).
Nomogram construction
A nomogram based on SLC2A2, SERPINF1, RASGRP1, and CHL1 was constructed (Figure 4A), and the calibration curve indicated that the diagnostic model nomogram was effective (Figure 4B). The highest benefit rate of the model (SLC2A2 + SERPINF1 + RASGRP1 + CHL1) highlighted the validity of the nomogram (Figure 4C). Furthermore, the AUC value of the nomogram based on SLC2A2 + SERPINF1 + RASGRP1 + CHL1 was 0.902, which verified the effectiveness of the nomogram (Figure 4D).
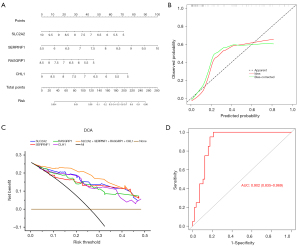
Screening of single-cell data and cell cluster analysis
After filtering, a total of 1,515 and 1,817 cells were retained in the T2D and healthy groups, respectively (Figure 5A). These cells were computed by ANOVA and the top 2,000 most variable genes were selected (Figure 5B).
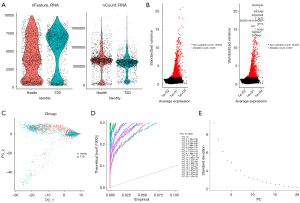
Following the normalization of gene expression, PCA of the variably expressed genes between the T2D and healthy groups was conducted (Figure 5C), and the top 20 principal components were screened for subsequent analysis (Figure 5D,5E).
Through unbiased clustering based on the tSNE analyses, 13 cell clusters were identified (Figure 6A). The results of cell cluster analysis in the healthy and T2D cohorts are shown in Figure 6B. Marker genes were screened using the FindAllMarkers function (https://cdn.amegroups.cn/static/public/atm-22-4303-5.xls) and the top five marker genes are displayed in Figure 6C.
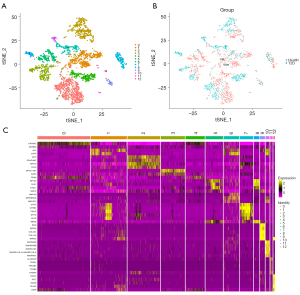
Based on the marker genes, we annotated 13 cell clusters to six different cell types (Figure S2A), including epithelial cells (CELA3A, CTRB1, SPINK1, CLPS, PRSS3, and eGFP), fibroblasts (BGN, SFRP2, COL3A1, COL1A2, IGFBP4, and SPARC), neurons (GPC5-AS1, DLK1, STMN2, SEC11C, G6PC2 and LY6E), endothelial cells (CLDN5, RGCC, IFI27, CD36, PLVAP, and ESAM), macrophages (ALOX5AP, CPA3, S100A4, TPSB2, TPSAB1, and TPSD1), and hepatocytes (CRYBA2, TTR, GPX3, CHGA, SNORD50A, and GCG). The expression levels of marker genes in the six cell types were displayed in violin and scatter plots (Figure S2B-S2M).
Confirmation of the key cell clusters and GSVA analysis
We found that SERPINF1 was mainly expressed in fibroblasts, which indicated that fibroblasts may be more correlated with the occurrence and progression of diabetes, so fibroblasts were used as the target cell for subsequent analysis (Figure S3A,S3B). The expression level of SERPINF1 in fibroblasts in the healthy group was higher than that in fibroblasts in the T2D group (Figure S3C, S3D). Furthermore, we discovered that the proportion of fibroblasts with a high expression of SERPINF1 was higher in the healthy group than in the T2D group (Figure S3E).
To explore the potential role of fibroblasts with high and low expressions in T2D, we analyzed the foundational enrichment by GSVA. Seventeen functional pathways were mainly activated in the high expression group, including phosphatidylinositol-3 kinase/protein kinase B (PI3K-AKT) signaling, interferon α response, reactive oxygen species pathway, and 21 functional pathways including apoptosis, inflammatory response pathway, and myogenesis were activated in the low expression group (Figure 7, https://cdn.amegroups.cn/static/public/atm-22-4303-6.xls).
Analysis of key TFs and target genes
To investigate the specific transcriptional regulators of fibroblasts with high and low expressions of SERPINF1, the TFs of the two cell groups were predicted by SCENIC, and the top 10 TFs of relative activity scores were demonstrated in Figure 8A and Figure 8B (https://cdn.amegroups.cn/static/public/atm-22-4303-7.xls). Both cell groups were regulated by HEYL, HOXB2, HOXA1, ATF5, ZNF467, SMARCB1, CREB3L1, JUNB, and NR1H2, which have already been identified as TFs for fibroblasts. However, NR2F2 and NFE2L2 were specific TFs in the high and low biomarker expression groups, respectively (Figure 8C), and their expression levels were visualized in Figure 8D,8E. The expression level of NR2F2 was significantly different, and therefore, it was considered as a TF of fibroblasts with high SERPINF1 expression (Figure 8F).
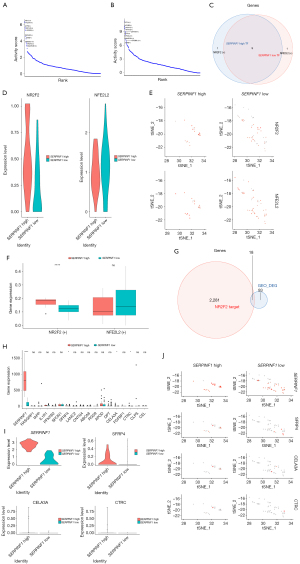
We also extracted the target genes regulated by NR2F2 from the SCENIC analysis results and intersected them with the DEGs. A total of 18 genes were both target genes and DEGs (Figure 8G), and four target genes: SERPINF1, SFRP4, CELA3A, and CTRC had notable differences between the fibroblasts with high and low expressions of SERPINF1 (Figure 8H), and their expression levels are displayed in Figure 8I,8J.
Discussion
Diabetes is an incurable chronic disease with chronic complications that can spread to vital organs of the body, and severe acute complications can be life-threatening. T2D accounts for more than 90% of the total number of diabetic patients (20). At present, the pathogenesis of T2D has not been fully studied, and in-depth research will help to advance the problem of curing T2D. With the development of single-cell sequencing technology, scRNA-seq data analysis has become a current research hotspot. Unlike tissue expression data, scRNA-seq data can mask important transcriptional signals contained in individual cells, and researchers can study genes at higher resolution in different types of cell populations based on scRNA-seq data (21). Using scRNA-seq data to study the gene expression profile changes and pathogenesis of T2D is a promising and effective method.
To further screen the biomarkers in T2D, we applied the LASSO algorithm based on 111 DEGs and identified four biomarker genes, including SLC2A2, SERPINF1, RASGRP1, and CHL1. These four genes have been studied in the past, including those linked to diabetes. In mice, biallelic SLC2A2 inactivation induces neonatal diabetes (22) and biallelic SLC2A2 mutations cause Fanconi-Bickel syndrome (FBS).
A previous study investigated the role of SLC2A2 in transient neonatal diabetes mellitus (TNDM) or permanent neonatal diabetes mellitus (PNDM) using a combination of sequencing and homozygosity mapping to rule out common genetic causes of NDM. Of the 104 included patients, five had SLC2A2 homozygous mutations, including four de novo mutations. Four out of five patients with SLC2A2 mutations developed isolated diabetes followed by Fanconi–Bickel syndrome. Four out of five patients had TNDM, and one patient with PNDM was still on insulin at 28 months. These results suggest that SLC2A2 mutation is an autosomal recessive cause of NDM. Patients with homozygous SLC2A2 mutations may have neonatal diabetes, which emphasizes the role of GLUT2 in human beta cells (23).
Pigment epithelium-derived factor (PEDF), also known as serpin F1 (human gene symbol: SERPINF1), belongs to the serpin family of peptide enzyme inhibitors. SERPINF1 exerts multiple roles in vitro and in mice, including promoting neuronal survival and differentiation and effectively inhibiting angiogenesis (24). The fat mass-increasing allele SNP rs12603825 is significantly associated with increased fasting SERPINF1 concentrations, and SERPINF1 levels are significantly positively correlated with all of the measured body fat parameters and fasting leptin concentrations. A common functional genetic variant at the locus encoding SERPINF1 is associated with overall obesity, obesity-related insulin resistance, and circulating leptin levels in populations that are at increased risk of T2D (25). Impaired endothelial angiogenesis is a hallmark of diabetic vascular complications.
RASGRP1, a member of the RasGRP family, is a nucleotide exchange factor necessary for Ras activation, which in turn stimulates various effector systems (26,27). A study has shown that knockdown of RasGRP1 significantly attenuates vascular endothelial growth factor (VEGF) induced migration and angiogenesis of human umbilical vein endothelial cells (HUVECs) and activation of the AKT pathway. Phosphorylation of VEGF, RASGRP1, and AKT is downregulated in high glucose-exposed HUVECs compared with normal glucose, whereas metformin up-regulates RASGRP1-dependent VEGF signaling and ameliorates impaired angiogenesis induced by high glucose. RASGRP1 is also involved in VEGF-induced angiogenesis and the pro-angiogenic effect of metformin under hyperglycemia (28).
CHL1 is a neural recognition molecule of the immunoglobulin superfamily that is mainly expressed in the nervous system (29). CHL1 regulates neuronal migration, axonal growth, and dendritic projection. In contrast to CHL1 overexpression, silencing of CHL1 induces cell proliferation, decreased apoptosis, prolonged S phase, and shortened G1 phase of the cell cycle. Extracellular signal-regulated kinase (ERK) 1/2-MAPK inhibitors abolish the effect of CHL1 deficiency on MIN6 cell proliferation. Furthermore, a high-fat diet results in increased islet volume and β-cell proliferation in mice, decreased CHL1 expression, and activation of the ERK pathway. Thus, high fat-induced decreased expression of CHL1 in mouse islets leads to cell proliferation via the ERK pathway and regulation of the cell cycle via the p53 pathway. These mechanisms may contribute to obesity-induced compensatory hyperplasia of pancreatic beta cells in prediabetes (30).
In addition, we also investigated specific TFs that were differentially expressed in fibroblasts with high and low expressions of SERPINF1, and finally found that the TFs NFE2L2 and NR2F2 were differently expressed in fibroblasts with low and high expressions of SERPINF1, respectively. NFE2L2, a member of the small family of basic leucine zipper (bZIP) proteins, exerts anti-oxidation and inflammation regulation functions (31). The high expression of NR2F2 is more significant in fibroblasts with high SERPINF1 expression, and its four target genes are differentially expressed in the two groups. NR2F2 is a nuclear receptor family gene and a ligand-induced TF that can regulate multiple genes (32). NR2F2 is thought to play a role in tumor progression and chronic periodontitis (33,34); however, the function of NR2F2 in the pathogenesis of diabetes needs to be further studied.
Our study also has certain limitations that should be noted. The sample size of single-cell data is too small, and there is no clinical sample validation. Also, experiments allowing further functional studies on key genes, such as NR2F2, were lacking. However, our study provides new important clues for the study of the pathogenesis of diabetes and proposes a possible function of fibroblasts in T2D (35). We will also continue to monitor our research findings.
In conclusion, we performed scRNA-seq analysis of T2D in this study. T2D-related DEG analysis yielded a total of 111 DEGs. Then, based on the LASSO algorithm of these DEGs, we identified relevant biomarkers, including SLC2A2, SERPINF1, RASGRP1, and CHL1, which could be used for the biological diagnostic prediction of T2D. Single-cell data screening and cell clustering analysis revealed that 13 cell clusters were annotated into six different cell types, including epithelial cells, fibroblasts, neurons, endothelial cells, macrophages, and hepatocytes. Interestingly, we found that SERPINF1 was predominantly expressed in fibroblasts, and subsequent analysis of fibroblasts revealed that SERPINF1 was expressed at higher levels in healthy constitutive fibroblasts than in T2D constitutive fibroblasts. Analysis of the key TFs and target genes found that NR2F2 and its target genes were differentially expressed in the two groups of fibroblasts, suggesting that NR2F2 is involved in the pathogenesis of T2D. Further research will reveal the specific mechanism of NR2F2 in T2D.
Acknowledgments
Funding: This work was supported by grants from the National Natural Science Foundation of China (No. 82074400), Hunan Provincial Technology Key Research and Development Program (No. 2020SK2101), National Natural Science Foundation of China (No. 82004185), Postgraduate Research and Innovation Project of Hunan Province (No. CX20210692), and Hunan Provincial Key Laboratory of Translational Medicine for TCM Recipe and Syndrome Research (No. 2018TP1021).
Footnote
Reporting Checklist: The authors have completed the TRIPOD reporting checklist. Available at https://atm.amegroups.com/article/view/10.21037/atm-22-4303/rc
Conflicts of Interest: All authors have completed the ICMJE uniform disclosure form (available at https://atm.amegroups.com/article/view/10.21037/atm-22-4303/coif). The authors have no conflicts of interest to declare.
Ethical Statement: The authors are accountable for all aspects of the work in ensuring that questions related to the accuracy or integrity of any part of the work are appropriately investigated and resolved. The study was conducted in accordance with the Declaration of Helsinki (as revised in 2013).
Open Access Statement: This is an Open Access article distributed in accordance with the Creative Commons Attribution-NonCommercial-NoDerivs 4.0 International License (CC BY-NC-ND 4.0), which permits the non-commercial replication and distribution of the article with the strict proviso that no changes or edits are made and the original work is properly cited (including links to both the formal publication through the relevant DOI and the license). See: https://creativecommons.org/licenses/by-nc-nd/4.0/.
References
- Namayandeh SM, Karimi A, Fallahzadeh H, et al. The incidence rate of diabetes mellitus (type II) and its related risk factors: A 10-year longitudinal study of Yazd Healthy Heart Cohort (YHHC), Iran. Diabetes Metab Syndr 2019;13:1437-41. [Crossref] [PubMed]
- Gheibi S, Singh T, da Cunha JPMCM, et al. Insulin/Glucose-Responsive Cells Derived from Induced Pluripotent Stem Cells: Disease Modeling and Treatment of Diabetes. Cells 2020;9:2465. [Crossref] [PubMed]
- Chen YT, Lin WD, Liao WL, et al. NT5C2 methylation regulatory interplay between DNMT1 and insulin receptor in type 2 diabetes. Sci Rep 2020;10:16087. [Crossref] [PubMed]
- Al Arawi WA, Al Shaman US, Albalawi WAM, et al. Association of Demographic Variables with the Awareness of Type 2 Diabetes Mellitus Patients (T2DM) among the Northwest Population in Saudi Arabia. J Diabetes Res 2020;2020:9408316. [Crossref] [PubMed]
- Chen C, Xiang Q, Liu W, et al. Co-expression Network Revealed Roles of RNA m6A Methylation in Human β-Cell of Type 2 Diabetes Mellitus. Front Cell Dev Biol 2021;9:651142. [Crossref] [PubMed]
- Courtney H, Nayar R, Rajeswaran C, et al. Long-term management of type 2 diabetes with glucagon-like peptide-1 receptor agonists. Diabetes Metab Syndr Obes 2017;10:79-87. [Crossref] [PubMed]
- Kim C, Jeong SH, Kim J, et al. Evaluation of the effect of filtered ultrafine particulate matter on bleomycin-induced lung fibrosis in a rat model using computed tomography, histopathologic analysis, and RNA sequencing. Sci Rep 2021;11:22672. [Crossref] [PubMed]
- Afzal M, Alghamdi SS, Migdadi HH, et al. Legume genomics and transcriptomics: From classic breeding to modern technologies. Saudi J Biol Sci 2020;27:543-55. [Crossref] [PubMed]
- Che X, Zhao R, Xu H, et al. Differently Expressed Genes (DEGs) Relevant to Type 2 Diabetes Mellitus Identification and Pathway Analysis via Integrated Bioinformatics Analysis. Med Sci Monit 2019;25:9237-44. [Crossref] [PubMed]
- Ding L, Fan L, Xu X, et al. Identification of core genes and pathways in type 2 diabetes mellitus by bioinformatics analysis. Mol Med Rep 2019;20:2597-608. [Crossref] [PubMed]
- Anene CA, Taggart E, Harwood CA, et al. Decosus: An R Framework for Universal Integration of Cell Proportion Estimation Methods. Front Genet 2022;13:802838. [Crossref] [PubMed]
- Dacquino C, De Rossi P, Spalletta G. Schizophrenia and bipolar disorder: The road from similarities and clinical heterogeneity to neurobiological types. Clin Chim Acta 2015;449:49-59. [Crossref] [PubMed]
- Li RY, Guan J, Zhou S. Boosting scRNA-seq data clustering by cluster-aware feature weighting. BMC Bioinformatics 2021;22:130. [Crossref] [PubMed]
- Ritchie ME, Phipson B, Wu D, et al. limma powers differential expression analyses for RNA-sequencing and microarray studies. Nucleic Acids Res 2015;43:e47. [Crossref] [PubMed]
- Wu T, Hu E, Xu S, et al. clusterProfiler 4.0: A universal enrichment tool for interpreting omics data. Innovation (Camb) 2021;2:100141. [Crossref] [PubMed]
- Gribov A, Sill M, Lück S, et al. SEURAT: visual analytics for the integrated analysis of microarray data. BMC Med Genomics 2010;3:21. [Crossref] [PubMed]
- Zhang X, Lan Y, Xu J, et al. CellMarker: a manually curated resource of cell markers in human and mouse. Nucleic Acids Res 2019;47:D721-8. [Crossref] [PubMed]
- Hänzelmann S, Castelo R, Guinney J. GSVA: gene set variation analysis for microarray and RNA-seq data. BMC Bioinformatics 2013;14:7. [Crossref] [PubMed]
- Aibar S, González-Blas CB, Moerman T, et al. SCENIC: single-cell regulatory network inference and clustering. Nat Methods 2017;14:1083-6. [Crossref] [PubMed]
- Nauck MA, Wefers J, Meier JJ. Treatment of type 2 diabetes: challenges, hopes, and anticipated successes. Lancet Diabetes Endocrinol 2021;9:525-44. [Crossref] [PubMed]
- Luecken MD, Theis FJ. Current best practices in single-cell RNA-seq analysis: a tutorial. Mol Syst Biol 2019;15:e8746. [Crossref] [PubMed]
- Thorens B, Cheng ZQ, Brown D, et al. Liver glucose transporter: a basolateral protein in hepatocytes and intestine and kidney cells. Am J Physiol 1990;259:C279-85. [Crossref] [PubMed]
- Sansbury FH, Flanagan SE, Houghton JA, et al. SLC2A2 mutations can cause neonatal diabetes, suggesting GLUT2 may have a role in human insulin secretion. Diabetologia 2012;55:2381-5. [Crossref] [PubMed]
- Becerra SP, Sagasti A, Spinella P, et al. Pigment epithelium-derived factor behaves like a noninhibitory serpin. Neurotrophic activity does not require the serpin reactive loop. J Biol Chem 1995;270:25992-9. [Crossref] [PubMed]
- Böhm A, Ordelheide AM, Machann J, et al. Common genetic variation in the SERPINF1 locus determines overall adiposity, obesity-related insulin resistance, and circulating leptin levels. PLoS One 2012;7:e34035. [Crossref] [PubMed]
- Ebinu JO, Bottorff DA, Chan EY, et al. RasGRP, a Ras guanyl nucleotide- releasing protein with calcium- and diacylglycerol-binding motifs. Science 1998;280:1082-6. [Crossref] [PubMed]
- Yamashita S, Mochizuki N, Ohba Y, et al. CalDAG-GEFIII activation of Ras, R-ras, and Rap1. J Biol Chem 2000;275:25488-93. [Crossref] [PubMed]
- Xu J, Liu M, Yu M, et al. RasGRP1 is a target for VEGF to induce angiogenesis and involved in the endothelial-protective effects of metformin under high glucose in HUVECs. IUBMB Life 2019;71:1391-400. [Crossref] [PubMed]
- Ottosson-Laakso E, Krus U, Storm P, et al. Glucose-Induced Changes in Gene Expression in Human Pancreatic Islets: Causes or Consequences of Chronic Hyperglycemia. Diabetes 2017;66:3013-28. [Crossref] [PubMed]
- Jiang H, Liu Y, Qian Y, et al. CHL1 promotes insulin secretion and negatively regulates the proliferation of pancreatic β cells. Biochem Biophys Res Commun 2020;525:1095-102. [Crossref] [PubMed]
- Li J, Tian M, Hua T, et al. Combination of autophagy and NFE2L2/NRF2 activation as a treatment approach for neuropathic pain. Autophagy 2021;17:4062-82. [Crossref] [PubMed]
- Arsov T, Kelecic J, Frkovic SH, et al. Expanding the clinical spectrum of pathogenic variation in NR2F2: Asplenia. Eur J Med Genet 2021;64:104347. [Crossref] [PubMed]
- Mauri F, Schepkens C, Lapouge G, et al. NR2F2 controls malignant squamous cell carcinoma state by promoting stemness and invasion and repressing differentiation. Nat Cancer 2021;2:1152-69. [Crossref] [PubMed]
- Li W, Zhang Z, Li Y, et al. Abnormal hsa_circ_0003948 expression affects chronic periodontitis development by regulating miR-144-3p/NR2F2/PTEN signaling. J Periodontal Res 2022;57:316-23. [Crossref] [PubMed]
- Wang X, Ding S. The biological and pharmacological connections between diabetes and various types of cancer. Pathol Res Pract 2021;227:153641. [Crossref] [PubMed]
(English Language Editor: A. Kassem)