This article has an erratum available at: http://dx.doi.org/10.21037/atm-2022-76 the article has been update on 2022-11-09 at here.
Correlation between gut bacteria Phascolarctobacterium and exogenous metabolite α-linolenic acid in T2DM: a case-control study
Introduction
Type 2 diabetes mellitus (T2DM) is a serious and common chronic disease. The main mechanism is an insulin secretion disorder of pancreatic β cells and insulin-sensitive tissues which causes an inappropriate response to insulin, resulting in insufficient insulin secretion and insulin resistance (1). In addition, the occurrence and development of T2DM are also affected by nutrition, physical activity, intestinal flora, and metabolites (2). With the deepening of research, the relationship between intestinal flora and metabolites in T2DM has attracted increasing attention.
Intestinal bacteria are highly dynamic and specific in the human body, and stable and diverse intestinal microecology is essential for human health (3). Firmicutes and Bacteroidetes comprise approximately 90% of all gut microbes, and their abundance and diversity are altered by disease (4,5). Research has shown (6-8) that when the abundance of gram-negative bacteria such as Proteobacteria and Escherichia increase in T2DM patients, lipopolysaccharide in the cell wall can activate toll-like receptor-4 (TLR4), and many pro-inflammatory cytokines are produced, causing low-level systemic inflammation (9). On the other hand, intestinal flora can also metabolize nutrients in vivo, transform and produce some endogenous molecules, including short-chain fatty acids (SCFAs), branched-chain fatty acids (BCFAs), and bile acids (BAs), and alleviate pathological conditions such as insulin resistance and inflammatory cell destruction (10,11). In addition, there are many metabolites from different sources, which play different functions in the occurrence and development of T2DM by interacting with intestinal flora after metabolism and transformation of the ingested nutrients in the body.
Many experiments have studied the regulatory functions of microbial-derived endogenous metabolites in metabolic disorders and the improvement of exogenous nutrients in the richness and diversity of gut microbiota. However, it is not entirely clear whether different gut flora characteristics can affect the absorption of exogenous metabolites and whether gut bacteria are affected by metabolites in improving diabetes and whether they can be used in combination to improve T2DM (12,13). Based on this, a case-control study was carried out to observe the difference in characteristics of intestinal flora and fecal small-molecule metabolites between T2DM patients and normal individuals. A correlation analysis of significantly altered and enriched pathway metabolites with gut microbiota was also carried out. The aim of this study was to explore differences in the content of metabolites in faeces under different intestinal flora abundances between T2DM and normal controls (NC) and to discuss how metabolites coordinate intestinal flora to regulate the immune and energy metabolism pathways of T2DM. Through this experiment, we hoped to provide a theoretical basis for finding a more accurate and efficient early diagnosis and treatment of T2DM. We present the following article in accordance with the STROBE reporting checklist (available at https://atm.amegroups.com/article/view/10.21037/atm-22-3967/rc).
Methods
Subjects
A total of 30 (15 men and 15 women) newly diagnosed T2DM patients with an age range of 30–70 years and different blood glucose levels (without special dietary habits) were selected from the Affiliated Hospital of Chengdu University of Traditional Chinese Medicine from March 2020 to September 2020. The NC group included 21 subjects (10 men and 11 women) from the Physical Examination Center of the Affiliated Hospital of Chengdu University of Traditional Chinese Medicine. All T2DM patients met the early diagnostic criteria of type 2 diabetes after the first update of the World Health Organization in 2019, and were not taking antidiabetic drugs including metformin. T2DM patients had received no previous treatment for their condition, and none had metabolic or infectious disease, serious heart disease, liver disease, kidney injury, or intestinal disease. In addition, none had taken antidiarrheal agents, probiotics, antibiotics, or other health care products within 3 months before participating in the study. Pregnant women, nursing mothers, and menstruating women were excluded (14). The study was conducted in accordance with the Declaration of Helsinki (as revised in 2013). The study was approved by the Medical Ethics Committee of The Affiliated Hospital of Chengdu University of Traditional Chinese Medicine (No. 2020KL-031). Subjects who met the inclusion criteria were informed of the basic information of the study and signed informed consent. The age, height, and weight of all subjects were recorded, as well as T2DM-related reference biochemical indicators.
Untargeted faecal metabolomics analysis
The weight of frozen stool sample from each group was approximately 60 mg. Metabolites were extracted by 1,000 µL of methanol: acetonitrile: water (2:2:1, v/v) and added 2 µg/mL of internal standard L-2-chlorophenylalanine, vortex and mixed for 30 seconds (methanol, Merck; acetonitrile, Merck; L-2-chlorophenylalanine, Shanghai Aladdin). Porcelain beads were added, milled at 45 Hz for 4 min, then ultrasonically for 5 min in an ice-water bath, and repeated three times. After standing at minus 20 ℃ for one hour, the sample was centrifuged at 4 ℃ and 12,000 rpm for 15 min, and the supernatant was placed in an EP tube, with the extract dried in a vacuum concentrator. Next, 150 µL of acetonitrile: water (1:1, v/v) was added to the dried metabolites for reconstitution then vortexed for 30 seconds and sonicated in an ice-water bath for 10 minutes. Samples were then centrifuged at 4 ℃ and 12,000 rpm for 15 min, then 120 µL of supernatant was extracted and placed into a 2-mL injection bottle, before 10 µL of each sample was mixed into QC samples for detection on a liquid chromatography-mass spectrometry (LC-MS) instrument.
The LC-MS consisted of an ACQUITY I-Class PLUS ULTRA high-performance liquid chromatography tandem XevOG2-XSQTof high resolution mass spectrometer (Waters, United States), and an Acquity UPLC HSS T3 column (1.8 µm 2.1×100 mm) was used; The injection volume was 1–3 µL, and the flow rate was 400 µL/min, while the separation gradient was as follows: 0–0.25min98%A, 0.25–10min2%A, 10–13 min2%A, 13–13.1 min98%A, and 13.1–15 min98%A. In both positive and negative ion modes, mobile phase A (0.1% formic acid aqueous solution) and mobile phase B (0.1% formic acid acetonitrile) were used (formic acid, TCI; Acetonitrile, Merck). The Waters XevoG2-XSQTof spectrometer collected primary and secondary mass spectrometry data in MSe mode under the control of the acquisition software (MassLynxV4.2, Waters). In each data acquisition cycle, dual-channel data acquisition for low and high collision energy was carried out simultaneously, which saw the low impact energy as 2 V, the high impact energy range 10–40 V, and the scanning frequency 0.2 seconds. The ESI ion source parameters were as follows: Capillary voltage was set to either 2,000 V (positive ion mode) or −1,500 V (negative ion mode), the taper hole source voltage was 30 volts, the ion source temperature was 150 ℃, the desolventizing gas temperature was 500 ℃, the reverse air flow rate was 50 L/h, and the desolventizing gas flow rate was 900 L/h.
16SrRNA generation full-length detection of intestinal flora
Midstream faecal samples were collected from subjects in the T2DM group and NC group, transported to the laboratory, and stored at −80 ℃. Total DNA was extracted from the samples using a PowerSoil®DNA isolation kit (MoBio, USA), and 16sRNA primers were designed to amplify using the following psrimer: forward primer 27 F: 5'-AGRGTTTGATYNTGGCTCAG-3'; reverse preimer 1492 R: 5'-TASGGHTACCTTGTTASGACTT-3'.
The amplified products were detected by agarose gel electrophoresis, and the qualified samples were mixed. The final reaction product was purified and placed on a Sequel II (Pacbio, USA) sequencer for on-board sequencing, and based on the PacBio sequencing platform, the samples were sequenced by SMRT Cell then filtered by Circular Consensus Sequencing (CCS) sequence. After identification and removal of chimeric sequences, Optimization-Circular Consensus Sequencing (Optimization-CCS) was obtained. Operational taxonomic units (OTUs) clustered the above optimized CCS sequences at the 97% similarity level (USEARCH, Version 10.0) and at bacterial 16S, and a Silva database and RDP Classifier platform was used for species annotation and classification analysis. Species taxonomic analysis was performed to compare the significant differences among groups at the genus and phylum level, and linear discriminant analysis effect size (LEfSe) was used to screen biomarkers with statistical differences among groups (the screening criteria of biomarkers: LDA score >3.5, P<0.05).
Statistical data processing and analysis
SPSS 26.0 (SPSS, Chicago, IL, USA) was used for statistical analysis, and measurement data were expressed as mean ± standard deviation. When the measurement data were normal and the variance was uniform, the t-test was used, and when not, the rank-sum test was used. LC-MS off-machine data was used for normalization and standardization. R studio was used to perform unsupervised principal component analysis (PCA) to distinguish the overall differences in metabolic profiles between groups and to find differential metabolites between groups. The R-3.3.2 language package ropls was used to perform OPLS-DA modeling, and 200 times permutation tests were performed to verify the reliability of the model. The variable importance for the projection (VIP) value of the model was calculated using multiple cross-validation, and the method of combining the difference multiple, the p value, and the VIP value of the OPLS-DA model was adopted to screen the differential metabolites. The screening criteria were P value <0.05 and VIP >1.
The raw data collected by MassLynxV4.2 was processed by ProgenesisQI (Waters Corporation, Milford, USA) software for peak extraction, peak alignment, and other data processing operations, and the mass deviation of fragment ion identification was within 100 ppm. The metabolites were identified using the Human Metabolome Database (HMDB; http://www.hmdb.ca/metabolites) and LIPID MAPS (https://lipidmaps.org) databases, and the R package clusterProfiler package performed enrichment analysis for differential metabolite results. The Kyoto Encyclopedia of Genes and Genomes (KEGG; http://www.kegg.jp/) was used for pathway enrichment analysis, while functional categories and generalists with P<0.05 were considered significant enrichment. The correlation and association analysis between the intestinal flora and target metabolites were conducted by redundancy analysis (RDA) or canonical correspondence analysis (CCA), and Pearson correlation analysis technique was used to analyze the correlation between intestinal flora and the differential metabolites enriched in the pathway.
Results
Demographic characteristics and clinical biochemical indexes
We assessed 30 newly diagnosed T2DM patients and 21 NC, and their demographic characteristics and clinical biochemical indexes appear in Table 1. While there was no statistical difference between the two groups in the distribution of gender (P>0.05), there was in age (P<0.001) and body mass index (BMI) (P<0.001). Among basic biochemical indexes, glycated hemoglobin (HbAlc) (P<0.001), fasting blood glucose (FBG) (P<0.001), total triglycerides (TG) (P=0.002), high-density lipoprotein (HDL) (P=0.006) and fasting serum insulin (FINS) (P=0.026) were significantly higher in the T2DM group compared with the NC group and were statistically significant. Renal parameters such as urea and creatinine were within the normal range.
Table 1
Clinical parameters | T2DM (n=30) | Control (n=21) | P |
---|---|---|---|
Gender (M/F) | 15/15 | 10/11 | 0.867 |
Age (years) | 55.30±10.19 | 42.75±10.62* | 0.000 |
BMI (kg/m2) | 25.66±2.99 | 22.10±1.84* | 0.000 |
TC (mg/dL) | 4.73±0.90 | 4.57±1.06 | 0.575 |
TG (mg/dL) | 1.98±1.08 | 1.16±0.70* | 0.002 |
FBG (mg/dL) | 8.16±2.36 | 5.28±0.78* | 0.000 |
HbA1c (%) | 7.48±1.82 | 5.40±0.46* | 0.000 |
Urea (mmol/L) | 4.79±1.17 | 4.86±1.97 | 0.885 |
Creatinine (μmoI/L) | 59.87±12.73 | 64.50±12.43 | 0.203 |
HDL (mg/dL) | 1.10±0.21 | 1.40±0.43* | 0.006 |
LDL (mg/dL) | 3.01±0.87 | 2.68±0.78 | 0.306 |
FINS (μU/mL) | 12.78±6.07 | 9.47±3.91* | 0.026 |
All parameters are mean ± SD. *, significant P value. BMI, body mass index; TC, total cholesterol; TG, triglyceride; FBG, fasting blood glucose; HbA1c, glycated hemoglobin; HDL, high-density lipoprotein; LDL, low-density lipoprotein; FINS, fasting serum insulin; T2DM, type 2 diabetes mellitus.
Multivariate statistical analysis of metabolites
After all samples were detected in positive and negative ion modes by UPLC-MS, respectively, multivariate statistical analysis was performed on the processed data, and according to the results of the PCA score plot, there were different characteristics of faeces in the T2DM group and NC group (Figure 1A,1B). Orthogonal partial least squares discriminant analysis (OPLS-DA) was also performed (15). In the permutation verification diagram, the horizontal axis represents the similarity with the original model, the vertical axis represents the value of R2Y or Q2, the blue and red dots represent the R2Y and Q2 of the model after Y replacement, respectively, and the dotted line is the fitted regression line. If both R2Y and Q2 are smaller than R2Y and Q2 of the original model, and all the points on the left side of the figure (permutation test) are lower than the point where the abscissa is 1 (original model), the model is meaningful (Figure 2A-2D).
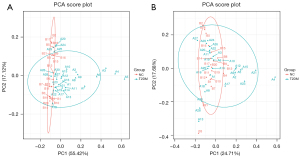
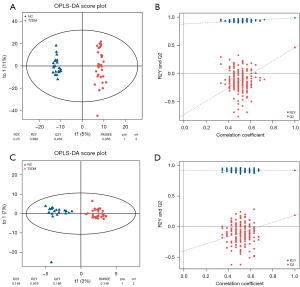
Screening of differential metabolites and KEGG pathway enrichment analysis
VIP >1 and P<0.05 were set as the screened criteria, and we found 355 differentially abundant metabolites in both groups, with 43 increased in T2DM, while 312 were decreased. The size of the scattered points in the volcano plot represents the VIP value of the OPLS-DA model, and the larger the scatter point, the larger the VIP value, and the more reliable the differentially expressed metabolites screened. The blue dots in the figure represent down-regulated differentially expressed metabolites, the red dots represent up-regulated differentially expressed metabolites, and the gray dots represent metabolites detected but not significantly different (Figure 3A). After sorting by P value, the top five qualitative metabolites were selected and labeled in the figure, and were 2-decaprenyl-3-methyl-6-methoxy-1,4-benzoquinone (VIP =3.6, P<0.05), N-glycoloylganglioside GM2 (VIP =2.9, P<0.05), PC(o-16:0/18:0) (VIP =2.8, P<0.05), PC[18:3(6Z,9Z,12Z)/24:1(15Z)] (VIP =2.8, P<0.05), and 3-O-sulfogalactosylceramide (d18:1/22:0) (VIP =2.8, P<0.05).
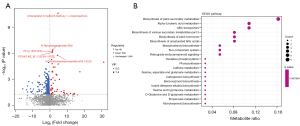
KEGG pathway enrichment point plot showed the enrichment of different metabolites in metabolic pathways (Figure 3B). Compared with the NC group, the levels of ornithine (P=0.04), L-lysine (P=0.03), glutamate (P=0.01), alpha-linolenic acid (P=0.004), traumatin (P=0.05), and erucic acid (P=0.004) in the T2DM group decreased significantly, while PC[18:3(6Z,9Z,12Z)/24:1(15Z)] (P<0.001) levels increased. Alpha-linolenic acid, PC[18:3(6Z,9Z,12Z)/24:1(15Z)], and traumatin were enriched in alpha-linolenic acid metabolism (P=0.014), and alpha-linolenic acid and erucic acid were enriched in biosynthesis of unsaturated fatty acids (P=0.035) (Table 2). The correlation was analyzed and compared with two groups of different intestinal bacteria.
Table 2
Pathway | Name | P value | Q value | Metabolite |
---|---|---|---|---|
ko00997 | Biosynthesis of various secondary metabolites—part 3 | 0.008759 | 0.36276 | Ornithine, L-lysine, and glutamate |
ko00592 | Alpha-linolenic acid metabolism | 0.014258 | 0.36276 | Alpha-linolenic acid, PC[18:3(6Z,9Z,12Z)/24:1(15Z)], and traumatin |
ko01060 | Biosynthesis of plant secondary metabolites | 0.017843 | 0.36276 | Protoporphyrinogen IX, alpha-linolenic acid, L-lysine, glutamate, and brassinolide |
ko01070 | Biosynthesis of plant hormones | 0.019808 | 0.36276 | Alpha-linolenic acid and brassinolide |
ko01040 | Biosynthesis of unsaturated fatty acids | 0.03585 | 0.36276 | Alpha-linolenic acid and erucic acid |
Species distribution of intestinal bacteria between the two groups
We evaluated the composition of intestinal flora in each group. At the genus level, the genera of Escherichia and Megamonas were significantly increased in T2DM, while Phascolarctobacterium were decreased (Figure 4A). We identified 21 differentially expressed biomarkers using LEfSe analysis (LDA score (log10 >3.5) (Figure 4B), and compared with the NC group, the abundances of Bacteroides_stercoris (P=0.0018), Bacteroides_uniformis (P=0.018), Phascolarctobacterium_calcite (P=0.04) and Phascolarctobacterium (P=0.035) in T2DM patients decreased, while Megamonas_funiformis (P=0.0021) and Ruminococcus_gnavus (P=0.015) increased. These results revealed differences in the composition of intestinal microbiota between the two groups, indicating the unique diversity and distribution characteristics of intestinal microbiota in newly diagnosed T2DM patients.
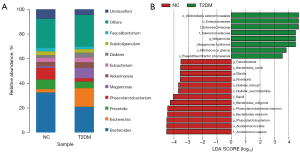
Correlation study of gut microbiota and target metabolites between T2DM and NC groups
RDA/CCA correlation analysis showed there was a certain correlation between intestinal flora and the above metabolites in diabetic patients. Pearson correlation analysis and visual analysis showed nine of the top 20 gut bacteria had positive and negative correlations with four different metabolites at the genus level (Figure 5). Among them, Phascolarctobacterium had significant positive correlation with alpha-linolenic acid (r=0.72, P<0.001).
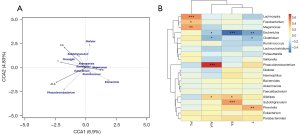
Discussion
The study recruited 51 participants: 30 diabetics and 21 normal controls. Compared with the NC group, the basic clinical characteristics such as FBG, FINS, TG, and BMI in patients with T2DM were significantly increased, and TC and LDL showed an increasing trend. Clinical basic biochemical indicators are important monitoring objects that reflect human metabolism. Naderpoor et al. (16) found in obese people with high BMI, Phascolarctobacterium was significantly positively correlated with insulin sensitivity. In a mouse experiment, Muller et al. (17) found a decrease in intestinal bacterial levels after antibiotic treatment led to a reduction in the number of neurons in the intestine of mice, and the blood glucose in an intervention group was higher than in a control group, indicating the intestinal neurons regulated by microbial groups can independently regulate blood glucose and other metabolism in the body. This further indicates the uniqueness and great potential of intestinal flora in the independent regulation of metabolism.
In recent years, fecal metabolomics has received increasing attention for microbial metabolic functions. Fecal samples are easy to obtain and are of proven value in studying hosts, microorganisms, and their co-regulated metabolic activities (18). In the current study, LC-MS was used to detect small molecular metabolites within 1,500 Da in the faeces of the NC group and T2DM group. We found 355 differentially abundant metabolites in both groups, with 43 of these increased in the T2DM group, while 312 were decreased. 16SrRNA third-generation full-length sequencing was used to evaluate the changed characteristics of intestinal microbials of different groups. At the genus level, the abundances of Bacteroides, Prevotella, and Phascolarctobacterium decreased in intestinal flora of the T2DM group, while the abundance of Escherichia increased, which was consistent with previous research (19-21). In this experiment, the fecal metabolites alpha-linolenic acid, PC[18:3(6Z,9Z,12Z)/24:1(15Z)], and traumatin were enriched in the alpha-linolenic acid metabolic pathway, and alpha-linolenic acid and erucic acid were enriched in the biosynthesis of unsaturated fatty acids, in which the former had a significant positive relationship with Phascolarctobacterium.
In the between-group difference results determined by LEfSe, the abundance of Phascolarctobacterium in T2DM decreased. Phascolarctobacterium is prevalent in the gastrointestinal tract, and can produce SCFAs, and a previous study has shown it as a genus that can have beneficial effects on the human body (22). SCFAs include acetic acid, propionic acid, isobutyric acid, butyric acid, and isovaleric acid, with butyric acid playing a central role in regulating intestinal metabolic balance. Propionic acid is closely related to important metabolic pathways such as the liver gluconeogenesis pathway (23). When SCFAs bind to free fatty acid receptor 2 (FFAR2) receptors in L cells, they can promote the increase of Ca2+ concentration in intestinal L cells and activate important intracellular signal transduction factors, including classical G protein signaling molecules such as protein kinase A (PKA) and protein kinase C (PKC), promote the secretion of glucagon-like peptide-1 (GLP-1), and accelerate the release of insulin (24). Alpha-linolenic acid is an omega-3 polyunsaturated fatty acid (n-3 PUFAs) with the first double bond at the third carbon atom starting from the methyl side of the fatty acid carbon chain (25). As a plant-derived essential fatty acid, it has research value because of its anti-inflammatory activity (25), can interfere with arachidonic acid (AA) metabolism, inhibit the prostaglandin biosynthesis pathway, and reduce the concentration of proinflammatory cytokines (26). In addition, alpha-linolenic acid can improve mitochondrial damage and endoplasmic stress, reduce oxygen species (ROS) and other peroxide production, induce 5-AMP-activated protein kinase (AMPK) activation, regulate multiple key transcription factors, and affect liver fat metabolism (27). Hosomi et al. found the differences in intestinal flora in different experimental groups were mainly reflected in the levels of Phascolarctobacterium, Turicibacter, and Eubacterium after four weeks of feeding soybean oil, EPA, and DHA. The content of Phascolarctobacterium in DHA-fed rats was lower than in soybean oil-fed rats, but its abundance in EPA-fed rats was significantly higher (28). EPA and DHA can be further converted by ingestion of alpha-linolenic acid and are important secondary metabolites of it in vivo. Due to different conversion efficiencies, the metabolic yields are also different (25). However, in a double-blind randomized controlled trial, after feeding n-3 PUFAs to patients with hyperlipidemia in the intervention group for three months, the abundances of Phascolarctobacterium and Veillonella in vivo were reduced (29). In summary, the relationship between alpha-linolenic acid and Phascolarctobacterium requires further investigation. The correlation heat plot showed erucic acid had a certain degree of negative correlation with Escherichia. When Gong et al. studied the feasibility evaluation of phenolic acids as potential probiotics in different whole wheat varieties, they proposed the residual phenolic acid content in chyme was related to the abundance of at least one bacterial genus (30). The correlation heat map showed erucic acid had a certain degree of negative correlation with Escherichia (r=−0.645), and erucic acid may also have different degrees of regulation with Escherichia in vivo.
Thus, in this experiment, it was found that the abundance of Phascolarctobacterium and alpha-linolenic acid concentration in the NC group were higher than those in the T2DM group. The positive correlation between the two was obtained by correlation analysis and indicates their combination may better promote the repair of metabolic disorders and the increase of intestinal probiotics abundance in patients with T2DM. However, to further understand the specific mechanism of the two in the human body, further investigation is required.
Limitation of the study
Due to the problems in this experiment, such as fewer specimens and no in vivo experimental verification, the conclusions are only the preliminary data for future experiments. Regardless, this also indirectly reflects the changes and connections of intestinal bacteria and exogenous metabolites in vivo, providing preliminary information for future in vivo experiments.
Conclusions
According to this experiment and other references, it is speculated that through their interaction the change of Phascolarctobacterium abundance may affect the absorption of alpha-linolenic acid. Alpha-linolenic acid is further metabolized in the body by increasing the abundance of Phascolarctobacterium bacteria, enhancing the transformation of SCFAs, improving the inflammatory pathway, and alleviating insulin resistance. In summary, there is a close and complex relationship between intestinal bacteria and metabolites. The intestinal flora with different characteristic distributions will affect the absorption of nutrients in the intestine, and the transformed metabolites will coordinate the intestinal flora to participate in the metabolic pathway in vivo. However, the relationship between Phascolarctobacterium and alpha-linolenic acid requires further research.
Acknowledgments
Funding: This work was supported by the Key Project of Sichuan Provincial Department of Science and Technology (No. 2020YFS0375).
Footnote
Reporting Checklist: The authors have completed the STROBE reporting checklist. Available at https://atm.amegroups.com/article/view/10.21037/atm-22-3967/rc
Data Sharing Statement: Available at https://atm.amegroups.com/article/view/10.21037/atm-22-3967/dss
Conflicts of Interest: All authors have completed the ICMJE uniform disclosure form (available at https://atm.amegroups.com/article/view/10.21037/atm-22-3967/coif). The authors have no other conflicts of interest to declare.
Ethical Statement: The authors are accountable for all aspects of the work in ensuring that questions related to the accuracy or integrity of any part of the work are appropriately investigated and resolved. The study was conducted in accordance with the Declaration of Helsinki (as revised in 2013). The study was approved by the Medical Ethics Committee of The Affiliated Hospital of Chengdu University of Traditional Chinese Medicine (No. 2020KL-031) and informed consent was taken from all the patients.
Open Access Statement: This is an Open Access article distributed in accordance with the Creative Commons Attribution-NonCommercial-NoDerivs 4.0 International License (CC BY-NC-ND 4.0), which permits the non-commercial replication and distribution of the article with the strict proviso that no changes or edits are made and the original work is properly cited (including links to both the formal publication through the relevant DOI and the license). See: https://creativecommons.org/licenses/by-nc-nd/4.0/.
References
- Roden M, Shulman GI. The integrative biology of type 2 diabetes. Nature 2019;576:51-60. [Crossref] [PubMed]
- Galicia-Garcia U, Benito-Vicente A, Jebari S, et al. Pathophysiology of Type 2 Diabetes Mellitus. Int J Mol Sci 2020;21:6275. [Crossref] [PubMed]
- Faith JJ, Guruge JL, Charbonneau M, et al. The long-term stability of the human gut microbiota. Science 2013;341:1237439. [Crossref] [PubMed]
- Arslan N. Obesity, fatty liver disease and intestinal microbiota. World J Gastroenterol 2014;20:16452-63. [Crossref] [PubMed]
- Cunningham AL, Stephens JW, Harris DA. Gut microbiota influence in type 2 diabetes mellitus (T2DM). Gut Pathog 2021;13:50. [Crossref] [PubMed]
- Song MJ, Kim KH, Yoon JM, et al. Activation of Toll-like receptor 4 is associated with insulin resistance in adipocytes. Biochem Biophys Res Commun 2006;346:739-45. [Crossref] [PubMed]
- Vatanen T, Kostic AD, d'Hennezel E, et al. Variation in Microbiome LPS Immunogenicity Contributes to Autoimmunity in Humans. Cell 2016;165:1551. [Crossref] [PubMed]
- Klein G, Raina S. Regulated Assembly of LPS, Its Structural Alterations and Cellular Response to LPS Defects. Int J Mol Sci 2019;20:356. [Crossref] [PubMed]
- Mohammad S, Thiemermann C. Role of Metabolic Endotoxemia in Systemic Inflammation and Potential Interventions. Front Immunol 2020;11:594150. [Crossref] [PubMed]
- Smith PM, Howitt MR, Panikov N, et al. The microbial metabolites, short-chain fatty acids, regulate colonic Treg cell homeostasis. Science 2013;341:569-73. [Crossref] [PubMed]
- Vinolo MA, Ferguson GJ, Kulkarni S, et al. SCFAs induce mouse neutrophil chemotaxis through the GPR43 receptor. PLoS One 2011;6:e21205. [Crossref] [PubMed]
- Schoeler M, Caesar R. Dietary lipids, gut microbiota and lipid metabolism. Rev Endocr Metab Disord 2019;20:461-72. [Crossref] [PubMed]
- Agus A, Clément K, Sokol H. Gut microbiota-derived metabolites as central regulators in metabolic disorders. Gut 2021;70:1174-82. [Crossref] [PubMed]
- Classification of diabetes mellitus. Geneva: World Health Organization; 2019. License: CC BY-NC-SA 3.0 IGO.
- Worley B, Powers R. PCA as a practical indicator of OPLS-DA model reliability. Curr Metabolomics 2016;4:97-103. [Crossref] [PubMed]
- Naderpoor N, Mousa A, Gomez-Arango LF, et al. Faecal Microbiota Are Related to Insulin Sensitivity and Secretion in Overweight or Obese Adults. J Clin Med 2019;8:452. [Crossref] [PubMed]
- Muller PA, Matheis F, Schneeberger M, et al. Microbiota-modulated CART+ enteric neurons autonomously regulate blood glucose. Science 2020;370:314-21. [Crossref] [PubMed]
- Karu N, Deng L, Slae M, et al. A review on human fecal metabolomics: Methods, applications and the human fecal metabolome database. Anal Chim Acta 2018;1030:1-24. [Crossref] [PubMed]
- Xia F, Wen LP, Ge BC, et al. Gut microbiota as a target for prevention and treatment of type 2 diabetes: Mechanisms and dietary natural products. World J Diabetes 2021;12:1146-63. [Crossref] [PubMed]
- Salamon D, Sroka-Oleksiak A, Kapusta P, et al. Characteristics of gut microbiota in adult patients with type 1 and type 2 diabetes based on next-generation sequencing of the 16S rRNA gene fragment. Pol Arch Intern Med 2018;128:336-43. [Crossref] [PubMed]
- Zhao L, Lou H, Peng Y, et al. Comprehensive relationships between gut microbiome and faecal metabolome in individuals with type 2 diabetes and its complications. Endocrine 2019;66:526-37. [Crossref] [PubMed]
- Wu F, Guo X, Zhang J, et al. Phascolarctobacterium faecium abundant colonization in human gastrointestinal tract. Exp Ther Med 2017;14:3122-6. [Crossref] [PubMed]
- Louis P, Flint HJ. Formation of propionate and butyrate by the human colonic microbiota. Environ Microbiol 2017;19:29-41. [Crossref] [PubMed]
- Reimann F, Gribble FM. Glucose-sensing in glucagon-like peptide-1-secreting cells. Diabetes 2002;51:2757-63. [Crossref] [PubMed]
- Shahidi F, Ambigaipalan P. Omega-3 Polyunsaturated Fatty Acids and Their Health Benefits. Annu Rev Food Sci Technol 2018;9:345-81. [Crossref] [PubMed]
- Hwang DH, Carroll AE. Decreased formation of porstaglandins derived from arachidonic acid by dietary linolenate in rats. Am J Clin Nutr 1980;33:590-7. [Crossref] [PubMed]
- Wang M, Ma LJ, Yang Y, et al. n-3 Polyunsaturated fatty acids for the management of alcoholic liver disease: A critical review. Crit Rev Food Sci Nutr 2019;59:S116-29. [Crossref] [PubMed]
- Hosomi R, Matsudo A, Sugimoto K, et al. Dietary Eicosapentaenoic Acid and Docosahexaenoic Acid Ethyl Esters Influence the Gut Microbiota and Bacterial Metabolites in Rats. J Oleo Sci 2021;70:1469-80. [Crossref] [PubMed]
- Liu H, Li X, Zhu Y, et al. Effect of Plant-Derived n-3 Polyunsaturated Fatty Acids on Blood Lipids and Gut Microbiota: A Double-Blind Randomized Controlled Trial. Front Nutr 2022;9:830960. [Crossref] [PubMed]
- Gong L, Chi J, Zhang Y, et al. In vitro evaluation of the bioaccessibility of phenolic acids in different whole wheats as potential prebiotics. Lwt 2019;100:435-43. [Crossref]