Identification of hub genes and infiltrating immune cells in skeletal muscle in obesity
Introduction
Metabolic syndrome (MetS) refers to a cluster of metabolic disorders, including insulin resistance, abdominal obesity, dyslipidaemia, and elevated blood pressure, and is associated with complications, such as diabetes mellitus and cardiovascular disease (1). Obesity is the leading cause of MetS and is rapidly increasing in both developing and developed countries (2). Therefore, exacerbation of the MetS epidemic due to the growing prevalence of obesity is a matter of concern.
Skeletal muscle, as the largest insulin-sensitive tissue, is a central part of systemic metabolism (3). It is responsible for approximately 20−30% of resting oxygen consumption and 75−95% of insulin-stimulated systemic glucose processing (3). Therefore, any changes in the metabolic rate, mass (4), or circulating factor response of the skeletal muscle will significantly affect systematic metabolism (5).
Obesity involves increased perimuscular immune cell infiltration and proinflammatory activation of intermyocytes and adipose tissue (6). Immune cells secrete proinflammatory molecules that induce myocyte inflammation, thereby impairing myocyte metabolism (7). In obesity, mast cells (MCs) have been reported to accumulate in white adipose tissue (8), and recruit inflammatory cells through interaction between cells and the release of proinflammatory cytokines, chemokines, and proteases (9). Furthermore, the infiltration of dendritic cells (DCs) in adipose tissue and the liver increases, which promotes macrophage infiltration (10) and plays an important role in innate and adaptive immunity (11).
Obesity alters the expression of genes that are closely related to metabolic diseases. For example, serum amyloid A (SAA) levels are higher in patients with obesity (12) and type 2 diabetes (13), and have also been found to share a positive correlation with 2-hour glucose levels (14). SAA1 and SAA2 are called acute-phase SAAs because they are highly inducible during the acute-phase response. SAA has a chemotactic effect on leukocytes, including monocytes, MCs, and T lymphocytes (15). Insulin-like growth factor 2 (IGF2) is a widely expressed polypeptide hormone that is mainly synthesized and secreted by the liver (16). As a promyogenic molecule, IGF2 plays a key role in muscle development and growth in the fetus and after birth, and assists in muscle recovery after injury (17). It is also closely related to metabolism, with studies showing that serum IGF2 levels are higher in patients with obesity (18), and that those with high IGF2 levels are more likely to develop diabetes than those with low IGF2 expression (19). Furthermore, IGF2 expression in porcine skeletal muscle is positively correlated with intramuscular lipid content (20), and IGF2 overexpression promotes endoplasmic reticulum stress, resulting in β cell dysfunction (21); these may be the mechanisms through which IGF2 induces metabolic disorders.
However, the specific genetic alterations and inflammatory state of skeletal muscle in obesity remain unclear. Therefore, in this study, we analyzed changes in gene expression and immune cell infiltration in the skeletal muscle of obese mice to screen for potential early biomarkers for the prevention and treatment of MetS. We present the following article in accordance with the MDAR reporting checklist (available at https://atm.amegroups.com/article/view/10.21037/atm-22-1010/rc).
Methods
Data source
The raw data of the GSE85439 dataset (22) were downloaded from the Gene Expression Omnibus (GEO) database (https://www.ncbi.nlm.nih.gov/geo/). From the microarray datasets, four groups of male mice were selected, with four gastrocnemius samples in each group. Each group of co-housed mice had been randomly assigned to an experimental group of age- and weight-matched mice. From 6 weeks of age, the mice in groups 1 and 2 were fed a normal diet [ND group; 14% kcal from fat, 62% kcal from carbohydrate, and 24% kcal from protein, the National Institutes of Health (NIH)-31, Harlan Teklad] and a high-fat diet (HFD group; 60% kcal from fat, 20% kcal from carbohydrate, and 20% kcal from protein; D12492, Research Diets Inc., USA), respectively, for 12 weeks. The other two groups, obtained from the Jackson Laboratory, comprised 10-week-old ob/ob male mice and their lean male controls, which were fed an ND and water ad libitum. The mice were kept in a clean room on a light/dark cycle for 12 hours. Before the experiments, the mice were acclimatized to living conditions for at least 10–14 days. Before the gastrocnemius samples were harvested, all mice were subjected to 4−5 hours of food withdrawal.
Data processing
On the R platform (version 4.1.0; https://www.r-project.org/), the raw data were read using the read.maimages function of the limma package (23). Next, the raw data were background-subtracted using the backgroundCorrect function and normalized using the normalizeBetweenArrays function of the limma package. Quality reports were then generated using the arrayQualityMetrics package (24). Data outliers were detected using the distance between the arrays, and one sample, GSM2267025, was considered an outlier and therefore removed. The normalized data were further processed using the modelmatrix, makeContrasts, lmFit, eBayes, and topTable functions of the limma R package to obtain differentially expressed genes (DEGs) between the ND and HFD groups and between the wild-type (WT) and ob/ob mice. Genes with a fold change >1.5 and P value <0.05 were defined as DEGs.
Enrichment analysis
Gene set enrichment analysis (GSEA) is an enrichment analysis tool for genome-wide expression profile data which sorts genes according to their level of differential expression to create a ranking table and then assesses whether pre-set gene sets are enriched at the top or bottom of the table. This method avoids missing genes that are not significantly differentially expressed but have important biological significance. Also, because GSEA detects gene sets rather than individual gene expression changes, it can identify subtle expression changes and thus produces more accurate results. Therefore, we imported the processed raw data (from four HFD, three ND, four ob/ob, and four WT mice) into the R platform and then used the gseGO and gseKEGG functions in the clusterProfiler package (25) to identify enriched gene sets with a false detection rate (FDR) <0.25, a normalized enrichment score >1, and P value <0.05, and conduct Gene Ontology (GO) enrichment analysis and Kyoto Encyclopedia of Genes and Genomes (KEGG) enrichment analysis.
Based on functional enrichment analysis of genes with significant expression differences, the significance of functions or pathways can be highlighted. Thus, the intersecting DEGs between the ND and HFD groups and between the WT and ob/ob mice were identified. The biological processes (BPs) of the intersecting DEGs were determined using the enrichGO function in the clusterProfiler package (25).
Immune infiltration analysis
CIBERSORTx (https://rdrr.io/github/singha53/amritr/src/R/supportFunc_cibersort.R) was downloaded and used to determine the proportion of each immune cell in the various samples. The signature matrix file for 25 types of immune cell compositions in mice was downloaded from the supplementary materials of Chen et al.’s article (26). The signature matrix file was obtained using ImmuCC, a computational algorithm that infers the relative compositions of 25 immune cell types in mouse tissues using microarray-based mRNA expression data.
The expression values of all genes or DEGs in each sample in the four groups (four HFD, three ND, four ob/ob, and four WT mice) were organized into expression matrix files. Next, the CIBERSORT function of the CIBERSORT R script was used to compare the genes in the signature matrix file and the expression matrix files to obtain the results for 25 infiltrating immune cell types in each sample. The Student’s t-test was used to analyze the different proportions of immune cells with statistical significance set at P<0.05.
Protein-protein interaction (PPI) network analysis
Intersecting DEGs between the ND (three mice) and HFD (four mice) groups and between WT (four mice) and ob/ob (four mice) mice were identified. The PPI network of the intersecting DEGs was established using STRING (https://www.string-db.org/), with a combined score >0.4 as the cut-off. The interaction file was downloaded and then uploaded to Cytoscape (version 3.8.1; https://cytoscape.org), and gene clusters were identified using the Cytoscape plug-in unit MCODE. The thresholds were set as follows: MCODE score ≥4, node score cut-off =0.2, max depth =100, and k-score =2.
Animals
Male C57BL/6J mice aged 7 weeks old were housed in the Shanghai Medical College of Fudan University. Age- and weight-matched mice were randomly assigned to two groups: HFD (60% kcal from fat, 20% kcal from carbohydrate, 20% kcal from protein; D12492, Research Diets Inc., USA) or ND (14% kcal from fat, 62% kcal from carbohydrate, and 24% kcal from protein, NIH-31, Harlan Teklad), with five mice in each group. The mice were kept in a clean room with a 12-hour light/dark cycle and fed an HFD or ND and water ad libitum for 8 weeks. At 15 weeks of age, the mice were weighed and their gastrocnemii were harvested. Animal care was conducted in accordance with the animal experimental guidelines formulated by the Shanghai Animal Care and Use Committee on Animals. This study was approved by the Ethics Committee of Huashan Hospital of Fudan University (No. 2019-285).
Real-time quantitative reverse transcription-polymerase chain reaction (qRT-PCR)
Hub genes were confirmed by real-time qRT-PCR. From 7 weeks of age, five mice were fed an HFD and five mice were fed an ND. The gastrocnemii of the mice were harvested after 8 weeks on the diet. Total RNA from each sample was extracted using TRIzol (Invitrogen, USA) and then subjected to reverse transcription into complementary DNA (cDNA Synthesis Kit, YEASEN, China). The mRNA concentrations of hub genes were measured by qRT-PCR using an RT-qPCR Kit (YEASEN, China). Glyceraldehyde-3-phosphate dehydrogenase (GAPDH) was used as the internal reference gene. The primer sequences used in this study are listed in Table S1.
Immunofluorescence
The gastrocnemius muscle sections (five ND and five HFD) were fixed in 4% formaldehyde at room temperature for 30 minutes. After being washed with phosphate-buffered solution, the cells were incubated in 0.1% Triton X-100 for 10 minutes. Next, the sections were blocked with 2% horse serum for 1 hour and incubated with tryptase antibody (MC marker; Proteintech Group, China) (27), CD11c antibody (Servicebio, China), and CD86 antibody (Abcam, England) (28) at 4 ℃ overnight.
The sections were then washed with phosphate-buffered saline (PBS) three times and incubated with a secondary antibody conjugated with Alexa Fluor Cy3, 488, and Cy5 (1:500, Beyotime, China) for 1 hour. Afterward, the sections were washed with PBS three times, stained with DAPI for 10 minutes, and then the fluorescence images were captured using a confocal microscope (Leica, Germany). Three different regions with an area of 1 mm2 were randomly selected in every sample slice. The average number of MCs in these three regions was calculated, and the average was used as the number of MCs in each sample (29).
Statistical analysis
Functional enrichment analysis of the DEGs and GSEA analysis were performed using the ClusterProfiler package on the R platform to obtain P values, and these P values were statistically corrected using the Benjamini-Hochberg method. The P values of the DEGs were calculated using the topTable function in the limma package, and these P values were also statistically corrected using the Benjamini-Hochberg method. The CIBERSORT was used to calculate the P values for the immune cell composition. The P values of the correlation analyses between genes and between genes and immune cells were performed using the cor function in the stats R package. The Student’s t-test was used to compare differences in body weight and gene expression between the HFD and ND mice.
Results
The workflow of this study is shown in Figure 1.
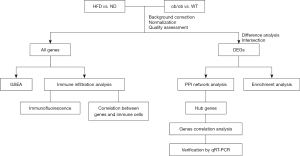
Results of GSEA
GO enrichment analysis indicated that genome-wide genes in the HFD group were enriched in acute inflammatory responses, antimicrobial humoral responses, leukocyte-mediated cytotoxicity, phenol-containing compound metabolic processes, and response to pheromones (Figure 2A). Additionally, genes in the ob/ob mice were significantly more enriched in acute inflammatory responses, endocrine processes, ribosome biogenesis, ribosomal RNA (rRNA) metabolic processes, and rRNA processing than were genes in the control group (Figure 2B). Next, KEGG enrichment analysis showed that the pathways of cell adhesion molecules and cytokine-cytokine receptor interaction were activated in the HFD group, while some metabolic processes, such as the AMP-activated protein kinase (AMPK) signaling pathway, were inhibited (Figure 2C). In the ob/ob mice, more genes (all downregulated) were enriched in the calcium and phosphatidylinositol 3-kinase (PI3K)-protein kinase B (PKB/Akt) signaling pathways than were in the control group (Figure 2D).
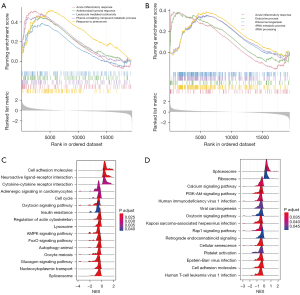
DEGs and their functional enrichment
A total of 2,082 protein-coding genes (1,094 upregulated and 988 downregulated) were differentially expressed in the skeletal muscle between the HFD and ND mice (Figure 3A). A total of 1,646 protein-coding genes (664 upregulated and 982 downregulated) were differentially expressed between the ob/ob and WT mice (Figure 3B). Intersection of these DEGs yielded 374 shared genes (Figure 3C). Functional enrichment analysis showed that these shared genes were mainly associated with the regulation of transcription (Figure 3D).
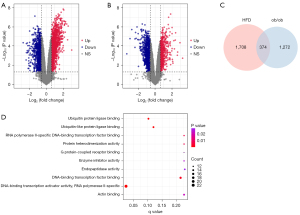
The PPI network and module
To explore the molecular interactions between the shared DEGs, we first transmitted all 374 of them to the STRING database, with a combined score >0.4 serving as the cut-off. Next, the results were exported to Cytoscape for the construction of an interaction network (Figure 4A). Clusters were screened out using the MCODE plug-in (Figure 4). Gene cluster 1 mainly included the GO terms ‘acute-phase response’ and ‘cell chemotaxis’.
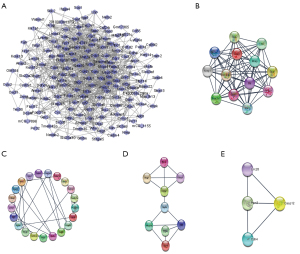
Immune cell infiltration
The GSEA and cluster 1 gene enrichment analysis indicated that the genes were closely related to the acute-phase response. We therefore analyzed infiltration by all 25 types of immune cells in the skeletal muscle.
Analysis using genome-wide genes revealed no difference in immune cell infiltration between the HFD and ND groups (Table S2), whereas the ob/ob mice exhibited a significantly higher proportion of MCs than did the WT mice (Table S3). Next, we analyzed 190 shared DEGs (90 upregulated and 100 downregulated) with consistent trends (Figure S1). The HFD mice had higher proportions of MCs and active DCs, but smaller proportions of memory B, naïve B, active CD8+ T, and memory CD8+ T cells, than did the ND mice (Figure 5, Table S4). In the ob/ob mice compared to the WT mice, the proportions of MCs and active DCs were increased markedly, whereas those of memory B, naïve B, and other immune cells were decreased (Figure 5, Table S5).
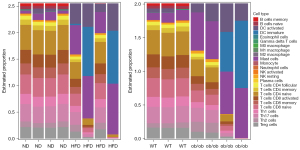
Immunofluorescence experiments were performed to detect infiltration by MCs and active DCs in the skeletal muscle. For fluorescent staining of MCs, we used a tryptase antibody as a specific marker (30). The skeletal muscle of HFD mice had more MCs than that of ND mice (P<0.05) (Figure 6A,6B). Next, to detect active DCs, we analyzed CD86 and CD11c co-localization as a marker (28). However, neither ND nor HFD skeletal muscle exhibited CD86 or CD11c co-localized cells, indicating an absence of active DCs (Figure S2).
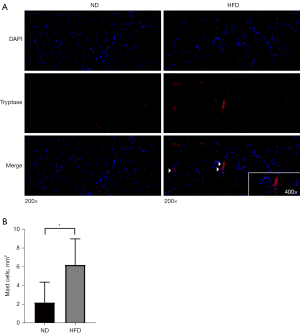
Correlations between the hub genes across the two test phenotypes and with immune cells
The shared DEGs were related to transcription; thus, we analyzed the correlations between gene clusters involved in the acute-phase response and genes that positively regulate transcription. Four acute-phase-response genes (SAA1, SAA2, ORM1, and HP) were all significantly correlated with transcription-regulating genes (SHH, IGF2, and CELA1) (P<0.05) (Table 1, Figure 7A-7C). The gene clusters of the PPI network were enriched in positive regulation of transcription, proteolysis, positive regulation of the apoptotic process, and axon guidance (Figure 7C). Furthermore, the expression of these seven hub genes was significantly higher in HFD and ob/ob mice than in the control mice (Figure 7D).
Table 1
Gene | HFD vs. ND | ob/ob vs. WT | |||||||
---|---|---|---|---|---|---|---|---|---|
SAA1 | SAA2 | ORM1 | HP | SAA1 | SAA2 | ORM1 | HP | ||
SHH | 0.021679 | 0.037605 | 0.009771 | 0.014893 | 0.045027 | 0.078461 | 0.055559 | 0.038506 | |
MAFB | 0.122827 | 0.186904 | 0.069383 | 0.027884 | 0.118143 | 0.202819 | 0.033867 | 0.132701 | |
MECOM | 0.578370 | 0.759932 | 0.185669 | 0.424563 | 0.406863 | 0.505385 | 0.342975 | 0.670521 | |
SIN3A | 0.278942 | 0.347226 | 0.091424 | 0.177620 | 0.815112 | 0.877007 | 0.972791 | 0.912114 | |
IGF2 | 0.026663 | 0.036096 | 0.007606 | 0.010150 | 0.043434 | 0.087859 | 0.004584 | 0.020718 | |
NR1H3 | 0.645306 | 0.737784 | 0.506240 | 0.410363 | 0.846728 | 0.758220 | 0.384628 | 0.640547 | |
RORA | 0.229494 | 0.187485 | 0.391920 | 0.201011 | 0.312522 | 0.341297 | 0.199811 | 0.434619 | |
CELA1 | 0.003424 | 0.007910 | 0.057371 | 0.001004 | 0.015725 | 0.039290 | 0.000162 | 0.003046 | |
SOX4 | 0.389868 | 0.432718 | 0.066716 | 0.277109 | 0.056704 | 0.096250 | 0.065182 | 0.073140 |
HFD, high-fat diet; ND, normal diet; WT, wild-type.
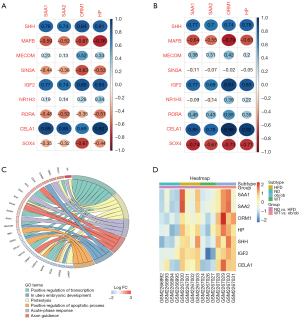
We then analyzed the correlation between immune cells and hub genes involved in the acute-phase response. SAA1 expression was positively correlated with the proportions of MCs and active DCs but negatively correlated with those of memory B, naïve B, gamma-delta T, and active natural killer cells. SAA2 expression was positively correlated with the proportion of active DCs but negatively correlated with that of naïve B and active natural killer cells (Figure 8). The expression of HP and ORM1 was positively correlated with the proportions of MCs and active DCs but negatively correlated with those of memory B and 12 other immune cell types (Figure S3 and Figure S4, respectively).
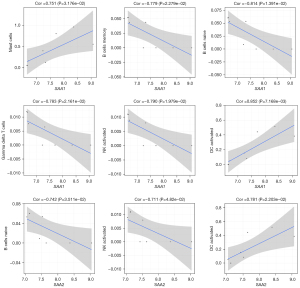
Verification of the hub genes by qRT-PCR
Mice fed an HFD had significantly higher body weights than did the control mice (Figure 9A). Results of qRT-PCR showed that the expression of SAA1, SAA2, IGF2, and CELA1 was markedly higher in the HFD group than in the ND group. However, the expression of HP, ORM1, and SHH did not differ significantly between the HFD and control mice (Figure 9, Figure S5).
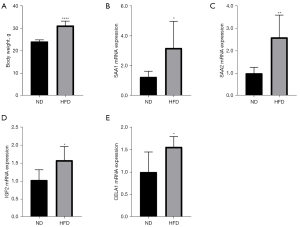
Discussion
In this study, we analyzed the changes in gene expression and inflammatory cell infiltration in the skeletal muscle in obesity. Our main finding was that the skeletal muscle of obese mice exhibited elevated MC infiltration, along with increased expression of SAA1, SAA2, CELA1, and IGF2.
Enrichment analysis suggested that the genes expressed in obese mice were mainly associated with the acute inflammatory response. Additionally, CIBERSORT analysis showed that the proportions of MCs and active DCs were significantly higher in obese mice than in the control group. Immunofluorescence analysis confirmed that more MCs were present in the skeletal muscle of HFD mice than in that of ND mice; however, no difference was observed in the active DC count. Our findings on MCs contrast with those of previous research, which observed no difference in the MC count of skeletal muscle between individuals with and without obesity (31). These apparently contradictory results highlight the need for more data, as sufficient studies clarifying the relationship between MCs and skeletal muscle are currently lacking.
Skeletal muscle inflammation increases in obesity (7), mainly manifesting as elevated immune cell infiltration and proinflammatory activation of the perimuscular adipose tissue that promotes muscle insulin resistance (7). In this study, we confirmed previously reported results by demonstrating the presence of MC infiltration in obese mice. MCs are involved in numerous inflammatory, neurological, and functional diseases (32). Depletion or inactivation of MCs improves diabetes mellitus, and MC stabilizers enhance the treatment of diabetic patients (33). The mechanism of action of MCs in disease appears to be the recruitment of inflammatory cells through their interaction with proinflammatory cytokines, chemokines, and proteases (34). MCs are also involved in DC maturation and their recruitment to tissues. Given the role of DCs in innate and adaptive immunity (11), our and others’ findings together suggest that MCs induce inflammation in the skeletal muscle, thereby affecting its metabolic function.
We found that obesity alters the expression of some genes that are closely related to metabolic disease. Specifically, qRT-PCR confirmed that four of the seven hub genes (SAA1, SAA2, CELA1, and IGF2) had significantly higher expression in HFD mice than in ND mice. Previous studies have reported that SAA concentrations are higher in patients with obesity (12) or type-2 diabetes mellitus (14), and are positively correlated with 2-hour glucose levels (14). Additionally, low concentrations of high-density lipoprotein cholesterol and apolipoprotein AI are associated with significantly high SAA levels in patients with active sarcoidosis (35). In patients with obesity, the serum IGF2 level is elevated, and patients with high IGF2 levels are more likely to develop type 2 diabetes mellitus (36). Alzhanov and Rotwein found that IGF2 promotes the growth and development of the skeletal muscle (17), which is the most important organ for glucose uptake to prevent metabolic disorders (3); however, this result seems to contradict those of the above studies. Overexpression of IGF2 promotes endoplasmic reticulum stress, leading to pancreatic β-cell dysfunction, proliferation, hyperinsulinemia, and hyperglycaemia (19,21). In addition, the IGF2 level in porcine skeletal muscle is positively correlated with the intramuscular lipid content (20). Therefore, it is necessary to further investigate the relationship between IGF2 and skeletal muscle metabolism.
Finally, CELA1 is a potential mediator of elastin remodeling, and silencing of CELA1 impairs lung angiogenesis (37). The expression of CELA1 increases during the development of lung epithelial cells (37) and macrophages, as well as during lung regeneration after pneumonectomy (38). The lungs of CELA1−/− mice exhibit an abnormal elastin structure and high elasticity (39). Taken together, our results are consistent with previous findings that link SAA and IGF2 expression with obesity. However, this is the first empirical demonstration of a potential role for CELA1 in obesity, and there is a need for further research.
Other than IGF2, little is currently known about the relationship between the four hub genes and skeletal muscle. IGF2 is a promyogenic protein that is important for muscle development as well as fetal and post-natal growth, and it also functions in muscle recovery and nerve regeneration after injury (18). IGF2 deletion in skeletal muscle cells reduces mitochondrial biogenesis, mitochondria-associated protein content, and mitochondrial function (40). Studies have also shown that increased IGF2 expression promotes the intramuscular lipid content of porcine skeletal muscle (20). Although the exact mechanisms underlying the effects of IGF2 on skeletal muscles are unknown, it is thought that the protein may regulate skeletal muscle mitochondria via the silent information regulator 2 homolog 1 (SIRT1)-peroxisome proliferator-activated receptor γ coactivator-1, alpha (PGC-1α) signaling pathway (40). Moreover, liver-specific deletion of the IGF2 mRNA-binding protein reduces fatty acid oxidation and increases hepatic triglyceride accumulation (41). Regarding SAA, some studies have reported that it promotes preadipocyte proliferation, increases tumor necrosis factor-α and interleukin-6 release, and inhibits glucose uptake by mature adipocytes (42). Furthermore, SAA has a chemotactic effect on leukocytes, including monocytes, MCs, and T lymphocytes (43).
Polymorphisms in SAA1 can alter the plasma SAA1 levels (44). In particular, the rs4638289 TT and rs12218 CC genotypes are more common in patients with obesity than in non-obese controls, although only the former is associated with SAA levels (45). Allelic variation in IGF2 is associated with body weight and body mass index (BMI). Patients with minor allele homozygotes at 6,815 A/T exhibit lower BMI, whereas those with minor allele homozygotes at 1,156 T/C show higher BMI, than do WT homozygotes (46). To the best of our knowledge, no CELA1 polymorphism studies are currently available; addressing this gap in the research should be a focus for future studies. Until more data on CELA1 polymorphisms are collected, researchers should at least include SAA and IGF2 genotyping when examining the relationship of SAA and IGF2 with obesity.
Other factors may also modify the expression of SAA and IGF2. For example, in a study on children, SAA levels were elevated only in male patients with obesity (12). A study in mice found that while an HFD increased the levels of circulating IGF2, female mice had higher overall IGF2 levels than did male mice (47). Furthermore, research on women with obesity has demonstrated that lower SAA levels were significantly associated with weight loss (48). In mice, hepatic IGF2 expression has been found to decrease significantly under feeding with a branched-chain amino acid diet versus a casein diet (49). In this study, we used only male mice to eliminate the potential effects of sex. Additionally, we fed mice with HFD and ND adjusted for lipid content. Nevertheless, the relationship between the ingested lipid content and gene expression remains unclear. Future studies should investigate the effects of dietary composition on obesity-related gene expression.
This study has several limitations that should be noted. Firstly, since we used an animal model, the relationship between our selected biomarkers and skeletal muscle may not be generalizable to humans. Secondly, few studies have examined the effects of dietary components on the tested genes; thus, we were unable to assess whether dietary components interfere with their influence in obesity. Moreover, due to the coronavirus disease 2019 (COVID-19) pandemic, we could not optimize the experiment by using membrane or extracellular matrix markers to verify the location of immune cells; however, we will supplement this experiment in the future. Finally, we cannot comment on the mechanisms underlying the effects of hub genes and immune cells on skeletal muscle. Nonetheless, our study provides a basis for a new direction in obesity research. By combining bioinformatics technology and basic experiments, we have confirmed that there is elevated MC infiltration and increased expression of SAA1, SAA2, CELA1, and IGF2 in the skeletal muscle of obese mice. These four potential markers may play an important role in skeletal muscle metabolism and in the prevention and treatment of MetS.
Conclusions
In this study, using bioinformatics analysis and fundamental experiments, we have found that the skeletal muscle of obese mice exhibits elevated MC infiltration along with increased SAA1, SAA2, CELA1, and IGF2 expression levels. No previous study has reported increased infiltration of MCs in skeletal muscle in obesity. Moreover, previous studies have found that MC elimination or inactivation can improve diabetes, and MC stabilizers can improve blood sugar; therefore, MC stabilization or elimination in skeletal muscle may be an effective way to treat metabolic diseases.
Our results are consistent with previous findings that link SAA and IGF2 with obesity. However, the increased expression of these two molecules in the skeletal muscle in obesity has never been reported; thus, they may represent new targets for the regulation of skeletal muscle metabolism. Our findings also suggest a potential role of CELA1 in obesity, but further exploration is required. Our study provides a basis for a new direction in obesity research, and the potential markers we have identified may play an important role in skeletal muscle metabolism and the prevention and treatment of metabolic diseases in the future.
Acknowledgments
We are very grateful to Prof. Cao for providing the original data of the GSE85439 dataset.
Funding: This work was funded by the National Natural Science Foundation of China (No. 81873645).
Footnote
Reporting Checklist: The authors have completed the MDAR reporting checklist. Available at https://atm.amegroups.com/article/view/10.21037/atm-22-1010/rc
Data Sharing Statement: Available at https://atm.amegroups.com/article/view/10.21037/atm-22-1010/dss
Peer Review File: Available at https://atm.amegroups.com/article/view/10.21037/atm-22-1010/prf
Conflicts of Interest: All authors have completed the ICMJE uniform disclosure form (available at https://atm.amegroups.com/article/view/10.21037/atm-22-1010/coif). The authors have no conflicts of interest to declare.
Ethical Statement: The authors are accountable for all aspects of the work in ensuring that questions related to the accuracy or integrity of any part of the work are appropriately investigated and resolved. This study was approved by the Ethics Committee of Huashan Hospital of Fudan University (No. 2019-285). Animal care was performed in accordance with the animal experimental guidelines formulated by the Shanghai Animal Care and Use Committee on Animals.
Open Access Statement: This is an Open Access article distributed in accordance with the Creative Commons Attribution-NonCommercial-NoDerivs 4.0 International License (CC BY-NC-ND 4.0), which permits the non-commercial replication and distribution of the article with the strict proviso that no changes or edits are made and the original work is properly cited (including links to both the formal publication through the relevant DOI and the license). See: https://creativecommons.org/licenses/by-nc-nd/4.0/.
References
- Fahed G, Aoun L, Bou Zerdan M, et al. Metabolic Syndrome: Updates on Pathophysiology and Management in 2021. Int J Mol Sci 2022;23:786. [Crossref] [PubMed]
- Salah RO, Ghandour R, Husseini A. Prevalence of overweight, obesity, and associated factors among adolescents in the occupied Palestinian territory: a cross-sectional study. Lancet 2021;398:S46. [Crossref] [PubMed]
- Baskin KK, Winders BR, Olson EN. Muscle as a "mediator" of systemic metabolism. Cell Metab 2015;21:237-48. [Crossref] [PubMed]
- Kim G, Lee SE, Jun JE, et al. Increase in relative skeletal muscle mass over time and its inverse association with metabolic syndrome development: a 7-year retrospective cohort study. Cardiovasc Diabetol 2018;17:23. [Crossref] [PubMed]
- Argilés JM, Campos N, Lopez-Pedrosa JM, et al. Skeletal Muscle Regulates Metabolism via Interorgan Crosstalk: Roles in Health and Disease. J Am Med Dir Assoc 2016;17:789-96. [Crossref] [PubMed]
- Wu H, Ballantyne CM. Metabolic Inflammation and Insulin Resistance in Obesity. Circ Res 2020;126:1549-64. [Crossref] [PubMed]
- Wu H, Ballantyne CM. Skeletal muscle inflammation and insulin resistance in obesity. J Clin Invest 2017;127:43-54. [Crossref] [PubMed]
- Elieh Ali Komi D, Shafaghat F, Christian M. Crosstalk Between Mast Cells and Adipocytes in Physiologic and Pathologic Conditions. Clin Rev Allergy Immunol 2020;58:388-400. [Crossref] [PubMed]
- Cinkajzlová A, Mráz M, Haluzík M. Adipose tissue immune cells in obesity, type 2 diabetes mellitus and cardiovascular diseases. J Endocrinol 2021;252:R1-R22. [Crossref] [PubMed]
- Stefanovic-Racic M, Yang X, Turner MS, et al. Dendritic cells promote macrophage infiltration and comprise a substantial proportion of obesity-associated increases in CD11c+ cells in adipose tissue and liver. Diabetes 2012;61:2330-9. [Crossref] [PubMed]
- Wu L, Liu YJ. Development of dendritic-cell lineages. Immunity 2007;26:741-50. [Crossref] [PubMed]
- Simoes E, Correia-Lima J, Sardas L, et al. Sex dimorphism in inflammatory response to obesity in childhood. Int J Obes (Lond) 2021;45:879-87. [Crossref] [PubMed]
- Griffiths K, Pazderska A, Ahmed M, et al. Type 2 Diabetes in Young Females Results in Increased Serum Amyloid A and Changes to Features of High Density Lipoproteins in Both HDL2 and HDL3. J Diabetes Res 2017;2017:1314864. [Crossref] [PubMed]
- Marzi C, Huth C, Herder C, et al. Acute-phase serum amyloid A protein and its implication in the development of type 2 diabetes in the KORA S4/F4 study. Diabetes Care 2013;36:1321-6. [Crossref] [PubMed]
- De Buck M, Gouwy M, Wang JM, et al. The cytokine-serum amyloid A-chemokine network. Cytokine Growth Factor Rev 2016;30:55-69. [Crossref] [PubMed]
- Kadakia R, Josefson J. The Relationship of Insulin-Like Growth Factor 2 to Fetal Growth and Adiposity. Horm Res Paediatr 2016;85:75-82. [Crossref] [PubMed]
- Alzhanov D, Rotwein P. Characterizing a distal muscle enhancer in the mouse Igf2 locus. Physiol Genomics 2016;48:167-72. [Crossref] [PubMed]
- Livingstone C, Borai A. Insulin-like growth factor-II: its role in metabolic and endocrine disease. Clin Endocrinol (Oxf) 2014;80:773-81. [Crossref] [PubMed]
- Devedjian JC, George M, Casellas A, et al. Transgenic mice overexpressing insulin-like growth factor-II in beta cells develop type 2 diabetes. J Clin Invest 2000;105:731-40. [Crossref] [PubMed]
- Aslan O, Hamill RM, Davey G, et al. Variation in the IGF2 gene promoter region is associated with intramuscular fat content in porcine skeletal muscle. Mol Biol Rep 2012;39:4101-10. [Crossref] [PubMed]
- Casellas A, Mallol C, Salavert A, et al. Insulin-like Growth Factor 2 Overexpression Induces β-Cell Dysfunction and Increases Beta-cell Susceptibility to Damage. J Biol Chem 2015;290:16772-85. [Crossref] [PubMed]
- Yang L, Li P, Yang W, et al. Integrative Transcriptome Analyses of Metabolic Responses in Mice Define Pivotal LncRNA Metabolic Regulators. Cell Metab 2016;24:627-39. [Crossref] [PubMed]
- Ritchie ME, Phipson B, Wu D, et al. limma powers differential expression analyses for RNA-sequencing and microarray studies. Nucleic Acids Res 2015;43:e47. [Crossref] [PubMed]
- Kauffmann A, Gentleman R, Huber W. arrayQualityMetrics--a bioconductor package for quality assessment of microarray data. Bioinformatics 2009;25:415-6. [Crossref] [PubMed]
- Yu G, Wang LG, Han Y, et al. clusterProfiler: an R package for comparing biological themes among gene clusters. OMICS 2012;16:284-7. [Crossref] [PubMed]
- Chen Z, Huang A, Sun J, et al. Inference of immune cell composition on the expression profiles of mouse tissue. Sci Rep 2017;7:40508. [Crossref] [PubMed]
- Maun HR, Jackman JK, Choy DF, et al. An Allosteric Anti-tryptase Antibody for the Treatment of Mast Cell-Mediated Severe Asthma. Cell 2019;179:417-431.e19. [Crossref] [PubMed]
- Wang L, Xiao W, Zheng Y, et al. High dose lipopolysaccharide triggers polarization of mouse thymic Th17 cells in vitro in the presence of mature dendritic cells. Cell Immunol 2012;274:98-108. [Crossref] [PubMed]
- Yokota M, Suzuki K, Tokoyoda K, et al. Roles of mast cells in the pathogenesis of inflammatory myopathy. Arthritis Res Ther 2014;16:R72. [Crossref] [PubMed]
- Barbara G, Stanghellini V, De Giorgio R, et al. Activated mast cells in proximity to colonic nerves correlate with abdominal pain in irritable bowel syndrome. Gastroenterology 2004;126:693-702. [Crossref] [PubMed]
- Boon MR, Bakker LE, Haks MC, et al. Short-term high-fat diet increases macrophage markers in skeletal muscle accompanied by impaired insulin signalling in healthy male subjects. Clin Sci (Lond) 2015;128:143-51. [Crossref] [PubMed]
- Galli SJ, Gaudenzio N, Tsai M. Mast Cells in Inflammation and Disease: Recent Progress and Ongoing Concerns. Annu Rev Immunol 2020;38:49-77. [Crossref] [PubMed]
- Shi MA, Shi GP. Different roles of mast cells in obesity and diabetes: lessons from experimental animals and humans. Front Immunol 2012;3:7. [Crossref] [PubMed]
- Mukai K, Tsai M, Saito H, et al. Mast cells as sources of cytokines, chemokines, and growth factors. Immunol Rev 2018;282:121-50. [Crossref] [PubMed]
- Salazar A, Maña J, Fiol C, et al. Influence of serum amyloid A on the decrease of high density lipoprotein-cholesterol in active sarcoidosis. Atherosclerosis 2000;152:497-502. [Crossref] [PubMed]
- Frystyk J, Skjaerbaek C, Vestbo E, et al. Circulating levels of free insulin-like growth factors in obese subjects: the impact of type 2 diabetes. Diabetes Metab Res Rev 1999;15:314-22. [Crossref] [PubMed]
- Liu S, Young SM, Varisco BM. Dynamic expression of chymotrypsin-like elastase 1 over the course of murine lung development. Am J Physiol Lung Cell Mol Physiol 2014;306:L1104-16. [Crossref] [PubMed]
- Joshi R, Liu S, Brown MD, et al. Stretch regulates expression and binding of chymotrypsin-like elastase 1 in the postnatal lung. FASEB J 2016;30:590-600. [Crossref] [PubMed]
- Joshi R, Heinz A, Fan Q, et al. Role for Cela1 in Postnatal Lung Remodeling and Alpha-1 Antitrypsin-Deficient Emphysema. Am J Respir Cell Mol Biol 2018;59:167-78. [Crossref] [PubMed]
- Zhu Y, Gui W, Tan B, et al. IGF2 deficiency causes mitochondrial defects in skeletal muscle. Clin Sci (Lond) 2021;135:979-90. [Crossref] [PubMed]
- Regué L, Minichiello L, Avruch J, et al. Liver-specific deletion of IGF2 mRNA binding protein-2/IMP2 reduces hepatic fatty acid oxidation and increases hepatic triglyceride accumulation. J Biol Chem 2019;294:11944-51. [Crossref] [PubMed]
- de Oliveira EM, Ascar TP, Silva JC, et al. Serum amyloid A links endotoxaemia to weight gain and insulin resistance in mice. Diabetologia 2016;59:1760-8. [Crossref] [PubMed]
- Abouelasrar Salama S, De Bondt M, De Buck M, et al. Serum Amyloid A1 (SAA1) Revisited: Restricted Leukocyte-Activating Properties of Homogeneous SAA1. Front Immunol 2020;11:843. [Crossref] [PubMed]
- Yamada T. Analysis of serum amyloid A1 exon 4 polymorphism in Japanese population. Amyloid 2000;7:118-20. [Crossref] [PubMed]
- Zhang X, Tang QZ, Wan AY, et al. SAA1 gene variants and childhood obesity in China. Lipids Health Dis 2013;12:161. [Crossref] [PubMed]
- Souren NY, Paulussen AD, Steyls A, et al. Parent-of-origin specific linkage and association of the IGF2 gene region with birth weight and adult metabolic risk factors. Int J Obes (Lond) 2009;33:962-70. [Crossref] [PubMed]
- Guerra-Cantera S, Frago LM, Jiménez-Hernaiz M, et al. Impact of Long-Term HFD Intake on the Peripheral and Central IGF System in Male and Female Mice. Metabolites 2020;10:462. [Crossref] [PubMed]
- Yang RZ, Blumenthal JB, Glynn NM, et al. Decrease of circulating SAA is correlated with reduction of abdominal SAA secretion during weight loss. Obesity (Silver Spring) 2014;22:1085-90. [Crossref] [PubMed]
- Iwasa J, Shimizu M, Shiraki M, et al. Dietary supplementation with branched-chain amino acids suppresses diethylnitrosamine-induced liver tumorigenesis in obese and diabetic C57BL/KsJ-db/db mice. Cancer Sci 2010;101:460-7. [Crossref] [PubMed]
(English Language Editors: A. Kassem and J. Reynolds)